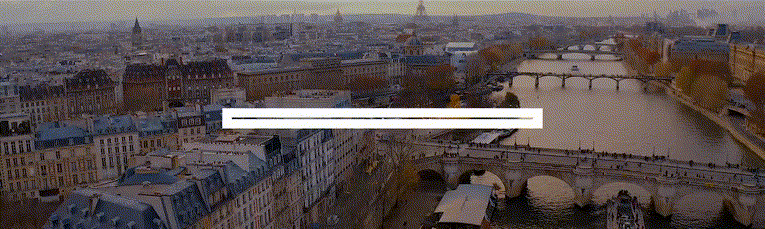
Wandering Minds and Aging Cells
- Epel, Elissa S ;
- Puterman, Eli ;
- Blackburn, Elizabeth ;
- Lazaro, Alanie ;
- Mendes, Wendy Berry
Published Web Location
Many ancient contemplative traditions believe presence of mind promotes greater longevity, a belief that is hard to test. Scientific evidence suggests that mind wandering predicts unhappiness, whereas presence in the moment predicts wellbeing. It is important to test whether a tendency toward mind wandering is associated with biological measures of longevity beyond self-reported measures of well-being. Telomere length has recently emerged as a proxy measure of biological aging and correlate of severe stress. We assessed the association between telomere length and tendency to be present versus tendency to mind wander in 239 healthy women. Those who reported high mind wandering had shorter telomeres, consistently across immune cell types (granulocytes, lymphocytes), than did those who reported low mind wandering, even after adjusting for stress. Telomere length varies widely between adults, and these findings suggest that presence of mind may explain some of these differences. A present attentional state may promote a healthy biochemical milieu and, in turn, cell longevity. © The Author(s) 2013.
Many UC-authored scholarly publications are freely available on this site because of the UC's open access policies . Let us know how this access is important for you.
Enter the password to open this PDF file:
- Alzheimer's disease & dementia
- Arthritis & Rheumatism
- Attention deficit disorders
- Autism spectrum disorders
- Biomedical technology
- Diseases, Conditions, Syndromes
- Endocrinology & Metabolism
- Gastroenterology
- Gerontology & Geriatrics
- Health informatics
- Inflammatory disorders
- Medical economics
- Medical research
- Medications
- Neuroscience
- Obstetrics & gynaecology
- Oncology & Cancer
- Ophthalmology
- Overweight & Obesity
- Parkinson's & Movement disorders
- Psychology & Psychiatry
- Radiology & Imaging
- Sleep disorders
- Sports medicine & Kinesiology
- Vaccination
- Breast cancer
- Cardiovascular disease
- Chronic obstructive pulmonary disease
- Colon cancer
- Coronary artery disease
- Heart attack
- Heart disease
- High blood pressure
- Kidney disease
- Lung cancer
- Multiple sclerosis
- Myocardial infarction
- Ovarian cancer
- Post traumatic stress disorder
- Rheumatoid arthritis
- Schizophrenia
- Skin cancer
- Type 2 diabetes
- Full List »
share this!
November 16, 2012
Wandering minds associated with aging cells
by University of California, San Francisco
Scientific studies have suggested that a wandering mind indicates unhappiness, whereas a mind that is present in the moment indicates well-being. Now, a preliminary UCSF study suggests a possible link between mind wandering and aging, by looking at a biological measure of longevity.
In the study, telomere length, an emerging biomarker for cellular and general bodily aging, was assessed in association with the tendency to be present in the moment versus the tendency to mind wander, in research on 239 healthy, midlife women ranging in age from 50 to 65 years.
Being present in the moment was defined as an inclination to be focused on current tasks, while mind wandering was defined as the inclination to have thoughts about things other than the present or being elsewhere.
According to the findings, published online on Nov. 15 in the new Association for Psychological Science journal Clinical Psychological Science , those who reported more mind wandering had shorter telomeres , while those who reported more presence in the moment, or having a greater focus and engagement with their current activities, had longer telomeres, even after adjusting for current stress.
Telomeres are the DNA -caps that protect the ends of chromosomes, preventing them from deteriorating or fusing with neighboring chromosomes . Telomeres typically shorten with age and in response to psychological and physiological stressors. In research pioneered at UCSF, scientists have discovered that telomere shortness predicts early disease and mortality .
As the study assessed mind wandering and telomeres at the same time, the researchers don't yet know whether mind wandering leads to shorter telomeres, whether the reverse occurs, or some common third factor is contributing to both.
Mindful meditation interventions, which promote attention on the present with a compassionate attitude of acceptance, lead to increases in some aspects of health. Previous studies have found that they are associated with increased activity of an enzyme known as telomerase, which is responsible for protecting and in some cases, replenishing telomeres.
Along with the new UCSF study, these findings support the possibility that a focus on the present may be part of what promotes health measurable at the cellular level, the researchers said.
"Our attentional state—where our thoughts rest at any moment – turns out to be a fascinating window into our well-being. It may be affected by our emotional state as well as shape our emotional state," said Elissa Epel, PhD, associate professor of psychiatry and lead author on the study. "In our healthy sample, people who report being more engaged in their current activities tend to have longer telomeres. We don't yet know how generalizable or important this relationship is."
Moving forward, Epel, along with Eli Puterman, PhD, a psychologist in the UCSF Department of Psychiatry, and colleagues are developing a series of classes to promote more mindful presence, to see if this intervention protects telomere maintenance or even lengthens telomeres.
In the current study, participants self-reported a tendency to mind wander, and were measured for aspects of psychological distress and well-being. The sample was highly educated and had a narrow range of both chronological age and psychological stress (most were low stress), all of which might have contributed to the ability to detect this relationship, Epel said.
The study is the first to link attentional state to telomere length and to control for stress and depression, Epel said. Previous studies have shown links between telomere length and particular types of stress and depression. Since this study relied on self-reported attentional state, she said, further studies directly measuring presence and mind wandering will be needed.
"This study was a first step and suggests it's worth delving into understanding the link between mind wandering and cell health to get a better understanding of whether there is causality and reversibility," said Epel. "For example, does reducing mind wandering promote better cell health? Or are these relationships just reflective of some underlying long-standing characteristics of a person?"
"Results suggest the possibility that the attitude of acceptance of negative experiences might be one of the factors that promotes greater ability to be more present - to be okay with one's current experience and not avoid the unpleasant aspects of everyday experiences," she said.
"A number of emotion theories suggest that greater attentional control leads to less suppression of negative emotions, and thus less of the rebound effect of unsuccessful suppression," said Wendy Berry Mendes, PhD, associate professor and Sarlo/Ekman Endowed Chair of Emotion at UCSF and co-author on this study. "Alternatively, attentional control may help us interpret emotions in a more constructive way, what we call 'positive reappraisals.' Such styles of thinking have been associated with healthy physiological states."
In addition to Epel, Mendes and Puterman, co-authors on this study include Jue Lin, UCSF research biochemist in the Department of Biochemistry and Biophysics; Elizabeth Blackburn, PhD, UCSF molecular biologist and Alanie Lazaro, UCSF laboratory manager in the Department of Psychiatry.
Research on telomeres, and the enzyme that makes them, was pioneered by three Americans, including Blackburn, who co-discovered the telomerase enzyme in 1985. The scientists received the Nobel Prize in Physiology or Medicine in 2009 for this work.
Epel, Blackburn and Lin are co-founders of Telome Health Inc, a telomere measurement company.
Explore further
Feedback to editors

Study highlights increased risk of second cancers among breast cancer survivors
50 minutes ago

Opioids during pregnancy not linked to substantially increased risk of psychiatric disorders in children

Research identifies pitfalls and opportunities for generative AI in patient messaging systems

A closed-loop drug-delivery system could improve chemotherapy
3 hours ago

It's easier now to treat opioid addiction with medication—but use has changed little, study finds

Solving the riddle of the sphingolipids in coronary artery disease

New AI technology estimates brain age using low-cost EEG device

Alteration of brain network condition could predict painful vaso-occlusive crisis in patients with sickle cell disease
5 hours ago

Trials reveal that internet-based conversations help sustain brain function in older adults

Study finds that a dash of exercise can help students focus and enjoy university lectures
Related stories, exercise may prevent stress on telomeres, a measure of cell health.
Apr 4, 2011
Study finds link between chronic depression and accelerated immune cell aging
Apr 5, 2011
Anticipation of stressful situations accelerates cellular aging
Feb 21, 2012
Brief exercise reduces impact of stress on cell aging, study shows
May 26, 2010
Does depression contribute to the aging process?
Anxiety linked to shortened telomeres, accelerated aging: research.
Jul 11, 2012
Recommended for you

Large study finds children with skin diseases suffer stigma, bullying and depression
9 hours ago

Study suggests that living near green spaces reduces the risk of depression and anxiety
Apr 23, 2024

Magic mushrooms can treat medication-resistant depression—but are they safe?

Image viewing experiments challenge theory of universal internal clock

Charting brain synchronization patterns during social interactions
Let us know if there is a problem with our content.
Use this form if you have come across a typo, inaccuracy or would like to send an edit request for the content on this page. For general inquiries, please use our contact form . For general feedback, use the public comments section below (please adhere to guidelines ).
Please select the most appropriate category to facilitate processing of your request
Thank you for taking time to provide your feedback to the editors.
Your feedback is important to us. However, we do not guarantee individual replies due to the high volume of messages.
E-mail the story
Your email address is used only to let the recipient know who sent the email. Neither your address nor the recipient's address will be used for any other purpose. The information you enter will appear in your e-mail message and is not retained by Medical Xpress in any form.
Newsletter sign up
Get weekly and/or daily updates delivered to your inbox. You can unsubscribe at any time and we'll never share your details to third parties.
More information Privacy policy
Donate and enjoy an ad-free experience
We keep our content available to everyone. Consider supporting Science X's mission by getting a premium account.
E-mail newsletter

Wandering minds associated with aging cells: Attentional state linked to length of telomeres
Scientific studies have suggested that a wandering mind indicates unhappiness, whereas a mind that is present in the moment indicates well-being. Now, a preliminary UCSF study suggests a possible link between mind wandering and aging, by looking at a biological measure of longevity.
In the study, telomere length, an emerging biomarker for cellular and general bodily aging, was assessed in association with the tendency to be present in the moment versus the tendency to mind wander, in research on 239 healthy, midlife women ranging in age from 50 to 65 years.
Being present in the moment was defined as an inclination to be focused on current tasks, while mind wandering was defined as the inclination to have thoughts about things other than the present or being elsewhere.
According to the findings, published online on Nov. 15 in the new Association for Psychological Science journal Clinical Psychological Science , those who reported more mind wandering had shorter telomeres, while those who reported more presence in the moment, or having a greater focus and engagement with their current activities, had longer telomeres, even after adjusting for current stress.
Telomeres are the DNA-caps that protect the ends of chromosomes, preventing them from deteriorating or fusing with neighboring chromosomes. Telomeres typically shorten with age and in response to psychological and physiological stressors. In research pioneered at UCSF, scientists have discovered that telomere shortness predicts early disease and mortality.
As the study assessed mind wandering and telomeres at the same time, the researchers don't yet know whether mind wandering leads to shorter telomeres, whether the reverse occurs, or some common third factor is contributing to both.
Mindful meditation interventions, which promote attention on the present with a compassionate attitude of acceptance, lead to increases in some aspects of health. Previous studies have found that they are associated with increased activity of an enzyme known as telomerase, which is responsible for protecting and in some cases, replenishing telomeres.
Along with the new UCSF study, these findings support the possibility that a focus on the present may be part of what promotes health measurable at the cellular level, the researchers said.
"Our attentional state -- where our thoughts rest at any moment -- turns out to be a fascinating window into our well-being. It may be affected by our emotional state as well as shape our emotional state," said Elissa Epel, PhD, associate professor of psychiatry and lead author on the study. "In our healthy sample, people who report being more engaged in their current activities tend to have longer telomeres. We don't yet know how generalizable or important this relationship is."
Moving forward, Epel, along with Eli Puterman, PhD, a psychologist in the UCSF Department of Psychiatry, and colleagues are developing a series of classes to promote more mindful presence, to see if this intervention protects telomere maintenance or even lengthens telomeres.
In the current study, participants self-reported a tendency to mind wander, and were measured for aspects of psychological distress and well-being. The sample was highly educated and had a narrow range of both chronological age and psychological stress (most were low stress), all of which might have contributed to the ability to detect this relationship, Epel said.
The study is the first to link attentional state to telomere length and to control for stress and depression, Epel said. Previous studies have shown links between telomere length and particular types of stress and depression. Since this study relied on self-reported attentional state, she said, further studies directly measuring presence and mind wandering will be needed.
"This study was a first step and suggests it's worth delving into understanding the link between mind wandering and cell health to get a better understanding of whether there is causality and reversibility," said Epel. "For example, does reducing mind wandering promote better cell health? Or are these relationships just reflective of some underlying long-standing characteristics of a person?"
"Results suggest the possibility that the attitude of acceptance of negative experiences might be one of the factors that promotes greater ability to be more present -- to be okay with one's current experience and not avoid the unpleasant aspects of everyday experiences," she said.
"A number of emotion theories suggest that greater attentional control leads to less suppression of negative emotions, and thus less of the rebound effect of unsuccessful suppression," said Wendy Berry Mendes, PhD, associate professor and Sarlo/Ekman Endowed Chair of Emotion at UCSF and co-author on this study. "Alternatively, attentional control may help us interpret emotions in a more constructive way, what we call 'positive reappraisals.' Such styles of thinking have been associated with healthy physiological states."
In addition to Epel, Mendes and Puterman, co-authors on this study include Jue Lin, UCSF research biochemist in the Department of Biochemistry and Biophysics; Elizabeth Blackburn, PhD, UCSF molecular biologist and Alanie Lazaro, UCSF laboratory manager in the Department of Psychiatry.
Research on telomeres, and the enzyme that makes them, was pioneered by three Americans, including Blackburn, who co-discovered the telomerase enzyme in 1985. The scientists received the Nobel Prize in Physiology or Medicine in 2009 for this work.
Epel, Blackburn and Lin are co-founders of Telome Health Inc, a telomere measurement company.
This study was funded by the Baumann Foundation and the Barney & Barbro Foundation.
- Mental Health Research
- Healthy Aging
- Workplace Health
- Chronic Illness
- Mental Health
- Brain-Computer Interfaces
- Philosophy of mind
- Psychedelic drug
- Neuropsychology
- Cognitive science
- Yoga (alternative medicine)
Story Source:
Materials provided by University of California, San Francisco (UCSF) . Note: Content may be edited for style and length.
Journal Reference :
- E. S. Epel, E. Puterman, J. Lin, E. Blackburn, A. Lazaro, W. B. Mendes. Wandering Minds and Aging Cells . Clinical Psychological Science , 2012; DOI: 10.1177/2167702612460234
Cite This Page :
Explore More
- Fossil Frogs Share Their Skincare Secrets
- Fussy Eater? Most Parents Play Short Order Cook
- Precise Time Measurement: Superradiant Atoms
- Artificial Cells That Act Like Living Cells
- Affordable and Targeted Anticancer Agent
- This Alloy Is Kinky
- Giant Galactic Explosion: Galaxy Pollution
- Flare Erupting Around a Black Hole
- Two Species Interbreeding Created New Butterfly
- Warming Antarctic Deep-Sea and Sea Level Rise
Trending Topics
Strange & offbeat.
Academia.edu no longer supports Internet Explorer.
To browse Academia.edu and the wider internet faster and more securely, please take a few seconds to upgrade your browser .
Enter the email address you signed up with and we'll email you a reset link.
- We're Hiring!
- Help Center
Wandering Minds and Aging Cells

2013, Clinical Psychological Science
Many ancient contemplative traditions believe presence of mind promotes greater longevity, a belief that is hard to test. Scientific evidence suggests that mind wandering predicts unhappiness, whereas presence in the moment predicts well-being. It is important to test whether a tendency toward mind wandering is associated with biological measures of longevity beyond self-reported measures of well-being. Telomere length has recently emerged as a proxy measure of biological aging and correlate of severe stress. We ...
Related Papers
Clifford Saron
Both theoretical and empirical work support the notion that meditation training can improve telomere regulation, which may ultimately contribute to healthy aging. Yet, the psychological and biological mechanisms underlying these changes remain underspecified, as do the contexts and boundary conditions in which these changes occur. Here we summarize studies investigating the effects of various meditation-based interventions on telomere biology, making suggestions for future research. We then propose a model describing how meditation training may impact acute and habitual stress responses as pathways to improved cell aging.
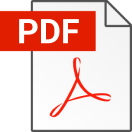
Research Square (Research Square)
Nilakshi Samaranayake
Brooke Huminski
Mindfulness
Marcelo Demarzo, MD, PhD
Roos Van Der Mast
Annals of Behavioral Medicine
Tonya Jacobs
Scientific Reports
Alberto Labarga
Mindfulness and meditation techniques have proven successful for the reduction of stress and improvement in general health. In addition, meditation is linked to longevity and longer telomere length, a proposed biomarker of human aging. Interestingly, DNA methylation changes have been described at specific subtelomeric regions in long-term meditators compared to controls. However, the molecular basis underlying these beneficial effects of meditation on human health still remains unclear. Here we show that DNA methylation levels, measured by the Infinium HumanMethylation450 BeadChip (Illumina) array, at specific subtelomeric regions containing GPR31 and SERPINB9 genes were associated with telomere length in long-term meditators with a strong statistical trend when correcting for multiple testing. Notably, age showed no association with telomere length in the group of long-term meditators. These results may suggest that long-term meditation could be related to epigenetic mechanisms, in...
Biological Psychiatry
richard maser
Systematic Reviews
Background Meditation-based practices have been suggested to result in many biological benefits which include reduction of attrition of telomeres, the protective nucleotide-protein complexes at termini of eukaryotic chromosomes. This systematic review evaluated the effects of meditation on telomere length (TL) in healthy adults. Methods Randomized controlled trials (RCTs) and observational studies conducted to determine the effects of meditation on TL in healthy individuals, published up to July 2020 were retrieved by searching seven electronic databases (PubMed, Scopus, PsycINFO, EMBASE, Cochrane Library, CINAHL and Google Scholar). The methodological quality of RCTs and observational studies was assessed using the Cochrane Collaboration Risk of Bias Tool and Joanna Briggs Institute critical appraisal checklist, respectively. The data was synthesized narratively and the effect estimates of TL in the RCTs were synthesized using alternative methods as a meta-analysis was not conducte...
RELATED PAPERS
Tetrahedron Letters
AURELIO GARCIA CSAKY
Texto & Contexto - Enfermagem
Josefa Delgado
Arbitrium. Zeitschrift für Rezensionen zur germanistischen Literaturwissenschaft 2011,2, pp. 164-170.
Bernhard F. Scholz
Mario GIELEN
Physical review. E
Trifce Sandev
49th IEEE Conference on Decision and Control (CDC)
Sylvain Bremond
JURNAL MANAJEMEN TRANSPORTASI DAN LOGISTIK
ConScientiae Saúde
Carla Rockenbach
Yulök Revista de Innovación Académica
Edward Parra Salazar
Chaos: An Interdisciplinary Journal of Nonlinear Science
Juan Valdivia
2013 IEEE SDN for Future Networks and Services (SDN4FNS)
cagatay buyukkoc
Journal of Radioanalytical and Nuclear Chemistry Articles
Bogusław Rajchel
Journal of Contemporary China
Tse-Kang Leng
Journal of Membrane Science
Francesco Muffato
Fernando Bustamante
CERN European Organization for Nuclear Research - Zenodo
Sita Ramaiah
Adriana Molina
Rizki Putriani
Antioxidants
Filipa Amaro
British Journal of Pharmacology
Janice Koepp
Critical Care Medicine
Sivanthiny Sivanandamoorthy
Revue Roumaine de …
Adrian Trifan
Pakistan Journal of Medical and Health Sciences
Haroon Mansha
American Journal of Transplantation
Mats Bengtsson
Facta universitatis. Series physical education and sport
Igor Vuckovic
- We're Hiring!
- Help Center
- Find new research papers in:
- Health Sciences
- Earth Sciences
- Cognitive Science
- Mathematics
- Computer Science
- Academia ©2024

An official website of the United States government
The .gov means it’s official. Federal government websites often end in .gov or .mil. Before sharing sensitive information, make sure you’re on a federal government site.
The site is secure. The https:// ensures that you are connecting to the official website and that any information you provide is encrypted and transmitted securely.
- Publications
- Account settings
Preview improvements coming to the PMC website in October 2024. Learn More or Try it out now .
- Advanced Search
- Journal List
- HHS Author Manuscripts

Mind-wandering in Healthy Aging and Early Stage Alzheimer’s Disease
Mate gyurkovics.
a Department of Psychology, University of Sheffield, Sheffield, UK
David A. Balota
b Department of Psychology, Washington University in St. Louis, St. Louis, MO, USA
Jonathan D. Jackson
c Department of Neurology, Massachusetts General Hospital, Harvard Medical School, Boston, MA, USA
The frequency of mind-wandering decreases as a function of age in healthy individuals. One possible explanation is that mind-wandering is a resource-dependent process, and cognitive resources decline with age. The present study provides the first investigation of mind-wandering in the earliest stages of Alzheimer’s disease (AD) to further examine the resource model and discontinuities between healthy aging and AD.
Three large cohorts completed the Sustained Attention to Response Task (SART): a healthy middle-aged group (mean age = 61.79 ± 5.84 years; N = 270), a healthy older adult group (mean age = 76.58 ± 5.27 years; N = 282), and a group with early stage AD (mean age = 76.08 ± 7.17; N = 77), comparable in age to the second group.
Self-reports of mind-wandering during the SART decreased as a function of age, and there was a further decrease in the AD group. All three groups produced faster responses on trials before No-Go errors, suggesting mind-wandering occurred in all cohorts. After No-Go errors, healthy older adults slowed disproportionately compared to middle-aged adults. This was not evident in AD individuals who showed post-error slowing comparable to that in the middle-aged group.
Conclusions
The decreased self-reported mind-wandering in older adults and the further decline in AD are consistent with the cognitive resource account of mind-wandering. Behavioral indices suggest that AD is on a continuum with healthy aging, with the exception of post-error slowing which may suggest performance monitoring deficits in early AD individuals (e.g., lack of error awareness).
Mind-wandering (MW) refers to the phenomenon of attention shifting away from mental contents related to the task at hand to unrelated thoughts and feelings ( Christoff, Irving, Fox, Spreng, & Andrews-Hanna, 2016 ; Smallwood & Schooler, 2006 ; 2015 ), and is considered to be a specific mental state within a spectrum of spontaneous thought phenomena ( Christoff et al., 2016 ). There is evidence that people spend a considerable amount of time mind-wandering in their everyday lives and therefore it appears to be a prominent feature of human phenomenology ( Giambra, 1989 ; Killingsworth & Gilbert, 2010 ; Kane et al., 2007 ; McVay, Kane, & Kwapil, 2009 ; Singer & McCraven, 1961 ; Stawarczyk, Majerus, Maj, Van der Linden, & D’Argembeau, 2011 ).
Methods developed to tap MW often include some form of thought sampling ( Smallwood & Schooler, 2006 ). For example, researchers often use an experience sampling approach which probes individuals intermittently during their everyday activities to determine if the participant is thinking about his or her current task or not (see e.g., Kane et al., 2007 ; Killingsworth & Gilbert, 2010 ). In laboratory studies, thought sampling reflects participants responding to a number of probe questions that usually appear randomly or quasi-randomly throughout an experimental task ( Smallwood & Schooler, 2006 , 2015 ). These questions prompt individuals to report their mental contents at the time of the probe. In addition to random sampling, studies sometimes utilize reports of “self-caught mind-wandering” ( Smallwood & Schooler, 2006 ). In this case, participants have to press a key during task performance whenever they detect that their attention has shifted away from the primary task (e.g., Cunningham, Scerbo, & Freeman, 2000 ; Jackson & Balota, 2012 ; Jackson, Weinstein, & Balota, 2013 ; Sayette, Reichle, & Schooler, 2009 ). For example, this might occur when an individual is reading and realizes that he/she has been thinking of something other than the material. This method, however, is unable to detect MW that happens in the absence of meta-awareness (i.e., when participants do not notice that their mind is wandering), even though such meta-awareness is not required for MW to occur ( Schooler, Reichle, & Halpern, 2004 ; Schooler et al., 2011 ; Smallwood, McSpadden, & Schooler, 2007 , 2008; Smallwood & Schooler, 2006 ).
In addition to subjective reports of MW, a common task used to indirectly measure MW is the Sustained Attention to Response Task (SART, e.g., Christoff, Gordon, Smallwood, Smith, & Schooler, 2009 ; Smallwood et al., 2004 ; Smallwood, Beach, Schooler, & Handy, 2008 ; Stawarczyk, Majerus, Maj, et al., 2011 ) in which participants have to respond to frequent non-targets (Go trials), but have to withhold their response to infrequent targets (or No-Go trials; Robertson, Manly, Andrade, Baddeley, & Yiend, 1997 ; Smallwood et al., 2004 ). In addition to self-report data from thought probes embedded within the task, the SART also provides reaction-time (RT) based indices of MW. Two typical RT-based measures of MW in the SART are pre-error speeding and post-error slowing. Pre-error speeding refers to the observation that response latencies on Go trials preceding a No-Go error (i.e., responding to a No-Go target) are generally shorter than on trials preceding correct No-Go trials, presumably reflecting “mindless” rhythmical responding ( Smallwood & Schooler, 2006 ). Post-error slowing refers to the relative slowing of responses following an incorrect No-Go trial compared to responses following a correct No-Go trial. This presumably reflects task re-engagement following the detection of an error caused by a MW episode or attentional lapse ( Cheyne, Carriere, Solman, & Smilek, 2011 ; Cheyne, Solman, Carriere, & Smilek, 2009 ; Jackson & Balota, 2012 ; McVay, Meier, Touron, & Kane, 2013 ). A further behavioral indicator is RT variability as indexed by the coefficient of variation (CoV = SD of Go RTs/Mean Go RTs) ( Cheyne et al., 2009 ). Greater cross-trial intraindividual variability presumably reflects more frequent episodes of transient (partial or complete) disengagement from the task. Importantly, however, RT-based measures may have some limitations. For instance, pre-error speeding could be produced by multiple factors in addition to – or instead of – MW, such as a build-up of a motor habit or a speed-accuracy trade-off ( McVay & Kane, 2012a ). Nevertheless, we still consider behavioral indices important as they are not limited to the time points directly preceding randomly distributed probes and they do not rely on participants’ metacognitive abilities (i.e., participants might misclassify their mental contents).
Mind-wandering and aging
The relationship between healthy aging and MW has been the focus of considerable work utilizing the methods outlined above (for a recent review see Maillet & Schacter, 2016a ). Irrespective of the method used, a relatively consistent, and somewhat surprising, pattern emerges in the literature: older adults tend to report less MW than younger adults ( Giambra, 1989 ; 2000; Jackson & Balota, 2012 ; Jackson et al., 2013 ; Krawietz, Tamplin, & Radvansky, 2012 ; Staub, Doignon-Camus, Bacon, & Bonnefond, 2014 ; Shake, Shulley, & Soto-Freita, 2015 ; Zavagnin, Borella, & De Beni, 2014 ). In addition, older adults do not seem to show differential pre-error speeding, but there is an age-related increase in post-error slowing, which may suggest that task re-engagement following periods of MW require greater effort from older adults than from younger adults, or that older adults devote more attention to evaluating their performance on the task (see for example, Jackson & Balota, 2012 ; McVay et al., 2013 for discussions).
There are a number of factors that can account for the decrease in MW with age. First, according to Smallwood and Schooler (2006) , MW requires cognitive resources because it involves executive control shifting from task performance to personal goals. Because cognitive resources decline with age ( Craik, 1986 ; Salthouse, 2009 ), older adults engage in MW less than younger adults. However, it should be noted that in the study by Shake et al. (2015) cognitive abilities (e.g., working memory capacity) were not associated with MW after controlling for age and interest. This lack of a relationship is somewhat difficult to reconcile with the cognitive resource account of MW. A similar account suggests that older adults may simply find the tasks more engaging and interesting, and the reduction in MW is merely a product of this absorption. Jackson and Balota (2012) , Krawietz et al. (2012) , and Shake et al. (2015) all reported that older adults found the tasks used in these studies (i.e., the SART and reading tasks) more interesting, and that interest was a predictor of MW. Jackson and Balota (2012) also found that older adults were more conscientious than younger adults, and thus less frequent MW in the former group may have been the result of a more disciplined attitude towards task completion. A third account of the age-related decrease in self-reported MW posits that older adults have fewer environmentally-triggered concerns than younger adults, and consequently may have fewer competing thoughts that could draw their attention away from task performance ( Giambra, 1993 ; McVay & Kane, 2010 ; Parks, Klinger, & Perlmutter, 1988 ). Finally, it is also possible that older adults are simply reluctant - or unable - to accurately report their experiences when prompted by the probes (e.g., they misclassify off-task thought as on-task). To investigate this latter account, Frank, Nara, Zavagnin, Touron, and Kane (2015) recently tested the validity of older adult thought reports using eye-tracking during a reading comprehension task. Results showed that eye movements preceding probes differentiated between on-task and off-task thought reports (MW) in both older and younger adults. This pattern was interpreted as indicating that older adults are indeed just as good as younger adults in reporting MW.
Present research
Although there has been considerable work investigating the intriguing finding that older adults consistently report less MW than younger adults, to our knowledge, there has yet to be any study of older adults who are transitioning to the earliest stages of Alzheimer’s disease (AD). This is the focus of the present study. AD pathology involves breakdowns in a number of cognitive processes, most notably episodic memory ( Albert, Moss, Blacker, Tanzi, & McArdle, 2007 ; Storandt, Grant, Miller, & Morris, 2006 ) and executive/attentional processes ( Balota & Faust, 2001 ; Balota et al., 2010 ; Faust & Balota, 2007 ). Consequently, the cognitive resource account of MW would suggest that older individuals with very mild dementia might report even less MW than cognitively healthy older adults because they have fewer resources left over for the executive control of MW. It is worth noting, however, that Duchek, Balota, Storandt, and Larsen (2007 ) found that very mildly demented individuals are also less conscientious than cognitively healthy controls, and so one might expect that individuals in the earliest stages of AD might show more MW than more conscientious healthy controls, if indeed conscientiousness is related to MW reports.
Furthermore, individuals with early stage AD are a particularly intriguing group to study because of the accumulating evidence indicating that there is a break-down in the “default mode network” in early stage AD ( Greicius, Srivastava, Reiss, & Menon, 2004 ; Lee et al., 2016 ; Lustig et al., 2003 ), which may be related to MW reports. The default mode network (DMN) is a set of brain regions that is active during periods of passive rest and becomes deactivated during task performance ( Buckner, 2012 ; Raichle et al., 2001 ; Shulman et al., 1997 ). Importantly, DMN activity is also thought be associated with spontaneous cognition, such as in MW ( Andrews-Hanna, Reidler, Huang, & Buckner, 2010 ; Christoff et al., 2016 ; Fox, Spreng, Ellamil, Andrews-Hanna, & Christoff, 2015 ; Mason et al., 2007 ; for studies specifically focusing on MW during SART see Christoff et al., 2009 ; Durantin, Dehais, & Delorme, 2015 ; Kirschner, Kam, Handy, & Ward, 2012 ; Stawarcyzk, Majerus, Maquet, & D’Argembeau, 2011). Because AD pathology disrupts the functioning of a network implied in MW, individuals showing early signs of AD may produce less MW than healthy controls. On the other hand, because there is evidence suggesting that the DMN is less deactivated during task performance in AD individuals than in healthy control individuals ( Lustig et al., 2003 ), one may actually find more mind-wandering during task performance in the former group. The present study will provide evidence regarding these contrasting predictions of MW in healthy and abnormal aging at the behavioral level.
The present study included three large groups of well-characterized older adults ( N = 629) who actively participate in longitudinal studies: two groups of cognitively healthy older adults (a younger group of subjects, mean age = 61.79, and an older group of participants, mean age = 76.58), and a group of older adults at the earliest detectable stage of AD (comparable in age to the older healthy control group, mean age = 76.08). Participants completed the SART with random thought probes. In addition to the thought probes, we examined the behavioral indices of MW (pre-error speeding, post-error slowing, and the coefficient of variation). Based on Jackson and Balota’s (2012) results, we expected to find larger post-error slowing as a function of age. This would be an important extension of earlier results because the vast majority of the aging studies of mind wandering have compared young college students to healthy older adults. In the present study, we compared a young-old group with an older-old group to examine if one can detect differences in post-error slowing between the two healthy community dwelling older groups that do not have extreme age differences. If we replicate the previous studies in this older sample, we also expected to find fewer MW reports in the old-old participants than the young-old participants.
Importantly, the present study also examined individuals with early stage AD. If AD is on a continuum with healthy aging, then one might expect a further increase in post-error slowing in the AD group as task re-engagement might prove most difficult for participants with attentional control deficits beyond age-related decline. Predictions for pre-error speeding are less clear as age did not disproportionately affect this index in Jackson and Balota’s (2012) original study. In addition, one may expect fewer subjective reports of MW in the early stage AD individuals in accordance with the predictions of the cognitive resource account. However, one could also expect an increased reporting of MW in the early stage AD individuals, compared to healthy older adults, if conscientiousness underlies the decreased reporting of MW in older adults compared to younger adults. This prediction is based on the decrease in conscientiousness in the early stages of AD compared to healthy older adults (e.g., Duchek et al., 2007 ),
Participants
A sample of 629 individuals participated in this study: 552 cognitively healthy adults (Clinical Dementia Rating; CDR = 0, no dementia) and 77 older adults in the earliest stages of AD (CDR higher than 0). Out of these individuals, 49 had a CDR of .5 (very mild dementia) and 28 had a CDR of 1 (mild dementia, see Morris, 1993 ). Healthy participants were further divided into middle-aged adults (age ≤ 69; M age = 61.79, SD = 5.84; range = 43 – 69; n = 270; 63.7% female) and older adults (age > 69; M age = 76.58, SD = 5.27; range = 70 – 94; n = 282; 59.6% female) to examine the effects of healthy aging. The individuals in the early stages of AD were grouped together regardless of age (M age = 76.08, SD = 7.17; range = 63 – 95; n = 77; 44.2% female), but their mean age was not significantly different from that of the older adult group (Welch test, F < .05; p = .569).
All participants were recruited from the Charles and Joanne Knight Alzheimer’s Disease Research Center (Knight ADRC) as part of either the Healthy Aging & Senile Dementia (HASD) project or the Adult Children Study (ACS). Both projects are longitudinal in design and follow individuals who are cognitively healthy upon entering the study. Participants are first screened for other forms of cognitive impairment (e.g., depression, hypertension, etc.) to be consistent with the criteria for “probable AD” as determined by the National Institute of Neurological and Communication Disorders and Stroke—Alzheimer’s Disease and Related Disorders Association ( McKhann et al., 1984 ). Dementia ratings are then assessed using the Washington University CDR scale ( Morris, 1993 ), which assesses dementia severity with CDR 0, 0.5, 1, 2, and 3, corresponding to no dementia, very mild, mild, moderate, and severe dementia, respectively. The CDR assessment encompasses a 90-minute clinical interview that assesses changes in cognition and participant functioning in such domains as memory, orientation, problem solving, community involvement, and personal care; and gathers information from a close collateral source (e.g., family member) too. The reliability and validity of the diagnosis at autopsy have been quite high (93% accuracy), even for those with very mild AD ( Storandt et al., 2006 ). Importantly, only individuals diagnosed with symptomatic AD were included in this study, and so we excluded all individuals with non-AD, uncertain or mixed etiologies, such as frontotemporal contributions to dementia. If a subject completed the SART more than once, only their baseline SART performance was used in the present analyses. Information about CDR status at the time of the SART was also obtained for all participants. Where available, personality data assessed by the NEO-FFI ( Costa & McCrae, 1992 ) was also included.
Table 1 provides demographic data as a function of group. Participants also completed a battery of psychometric tests as part of the project they were enrolled in. These tests were administered during a separate session. The table includes results for the Mini-Mental State Examination (MMSE; Folstein, Folstein, & McHugh, 1975 ), the Selective Reminding Test (SRT; Buschke, 1973 ) and the reading span task ( Daneman & Carpenter, 1980 ). Psychometric data for a given participant was only included if it was collected no later than +/− 1 year from the date of SART completion. As shown in Table 1 , performance significantly declined across groups from younger adults to older adults, and from older adults to early AD (ps < .05 for all group wise comparisons).
Demographic and psychometric data – means (SDs) - as a function of group.
Note: MMSE = Mini-Mental State Examination; SRT = Selective Reminding Test. Higher scores indicate better performance.
In the SART, participants were presented with single numbers 1–9 with the number 3 identified as a target (No-Go) stimulus to which they should withhold their response. If any other digit (non-target, or Go stimulus) appeared, participants were required to press the SPACE bar. The task consisted of 125 trials, and lasted approximately 5 minutes. Each digit was presented for 1250 ms followed by a blank screen of the same duration. Targets were presented on 11.1% of the trials. Throughout the task, 5 thought probes appeared in a random fashion.
SART sessions were preceded by 3 blocks of practice. In the first practice block, 9 trials (1 target) were presented with feedback, without any random thought probes. The second practice block was identical, with the exception that a single thought probe was also presented. In the third practice block, participants no longer received feedback on their performance. With this exception, it was identical to the preceding blocks. This was followed by a test block of 125 trials.
Stimuli were presented centrally, in white against a black background in 44 pt Courier New font. On probe trials, subjects saw the following instruction: “Please choose the one option below which best describes your experience with the task just now”. They then indicated their response by pressing a number key corresponding to one of the following categories: 1) I was thinking about the task; 2) My mind was blank; 3) My mind drifted to things other than the task, but I wasn’t aware of it until you asked me; 4) While doing the task I was aware that thoughts about other things popped into my head. These reflect on-task thought, space outs, zone outs, and tune outs, respectively ( Jackson & Balota, 2012 ; Smallwood, McSpadden, & Schooler, 2007 ). Upon completion of the task, participants also indicated how difficult and how interesting they found the task on a 5-point Likert scale. The experiment was run using E-Prime 1.2 Software (Psychology Software Tools, Pittsburgh, PA).
An alpha of .05 was set to indicate significance in all analyses. Mean response latencies and accuracies as a function of group (middle-aged, healthy older adult, AD) are presented in Table 2 . Trial level RTs below 150 ms and above 2000 ms were removed prior to analyses (.04% of trials were removed due to this screening). Following this, RTs beyond three standard deviations of a participant’s mean were also removed. This resulted in the removal of 1.75% of trials in the middle-aged group, 1.87% in the healthy older adult group, and 1.72% in the AD group 1 . In addition, analyses are also reported on standardized RTs, based on the subject’s mean and standard deviation in order to control for general slowing across groups (see Faust, Balota, Spieler, & Ferraro, 1999 ). Corresponding z-scores are also presented in Table 2 .
Descriptive data – means (SDs) – for middle-aged adults, healthy older adults, and early stage AD individuals for the SART.
Note: Because not all participants committed No-Go errors, reaction times for pre- and post-correct RTs are reported from the subsample of those participants who had pre- or post-error RT data.
Following Jackson and Balota (2012) , thought probes that occurred immediately following a No-Go target due to the randomization were removed from later analyses, since rare No-Go trials may engage task attention. Approximately 11.77% of probes were lost due to this screening. In addition, data from 7 participants (N = 7) who only had 1 or 2 probe responses due to this randomization were not analyzed.
In order to investigate the effects of Age and CDR status on accuracy, a 2 (Go trial accuracy vs. No-Go trial accuracy) × 3 (healthy middle-aged, healthy old, AD) mixed model ANOVA was conducted. Results indicated that subjects were significantly more accurate on Go trials than on No-Go trials (F(1,626) = 140.64, p < .001). A main effect of group was also found (F(2,626) = 35.92, p < .001) indicating that there was no difference in accuracy between the two healthy groups, but AD individuals were significantly less accurate than both other groups (both ps < .001). There was no interaction between group and trial type (F < 1).
Response latencies
A 2 (No-Go response latency vs. Go response latency) × 3 (healthy middle-aged, healthy old, or AD) mixed model ANOVA was conducted to examine the effects of CDR status on reaction times. Subjects were faster on No-Go error trials than on correct Go trials (F(1,301) = 238.00, p < .001). Response latencies varied as a function of group (F(2,301) = 8.10, p < .001): middle-aged adults were significantly faster than both older groups (ps < .01), however, there was no significant difference between healthy and AD older adults. 2 There was a significant group by trial type interaction (F(2,301) = 3.70, p < .05). In follow-up analyses, separate Group × Trial Type ANOVAs indicated that there was a significantly greater difference between No-Go and Go RTs in AD participants, compared to middle-aged adults (F(1,170) = 6.31, p < .05), and in AD participants compared to the age-matched older adults (F(1,175) = 6.53, p < .05). When z-scores were analyzed instead of raw reaction times, No-Go error z-RTs were still significantly faster than Go z-RTs (F(1,301) = 217.69, p < .001), but neither the main effect of group nor the group by trial type interaction was reliable (Fs < 1).
Additional measures from the SART
In order to investigate intra-individual variability across groups, the coefficient of variation (CoV) was calculated for each participant by dividing the standard deviation of their Go RTs by their mean Go RT. Since the assumption of homogeneity of variances was not met, a Welch test was run to examine group differences in CoV. CoV was reliably different across groups (F(2,195.74) = 19.38, p < .001). According to post-hoc analysis, participants in the early stages of AD showed significantly more variable performance (higher CoV) than did those in other two groups (p < .001). The healthy groups did not differ from each other. This result is illustrated in Figure 1 .

Reaction time variability (coefficient of variation) as a function of group. Error bars represent +/− 1 SE.
A skill index ( Seli, 2016 , see also Seli, Jonker, Solman, Cheyne, & Smilek, 2013 ) was also calculated. This index controls for individual differences in speed-accuracy trade-offs, and is calculated by dividing each subject’s mean No-Go accuracy by their mean Go RT. The result is then multiplied by 1000 for interpretive convenience ( Seli, 2016 ). Higher skill index indicates better performance on the SART. Skill index systematically decreased as a function of both healthy aging and early stage AD (Welch test, F(2,200.83) = 27.93, p < .001), with each group being reliably different from each other (all ps < .01).
Pre-error speeding
In the following analyses the mean response latency of four Go trials preceding a No-Go error (i.e., when a response was made to a target) is contrasted against the mean response latency of four Go trials preceding a correct No-Go trial (i.e., where no response was made). If MW is occurring, one might expect trials before an error to be relatively fast because participants are not engaged in fully processing these items, and in some sense are mindlessly pressing the button. Indeed, a 2 (Pre-error RT vs. Pre-correct RT) × 3 (healthy middle-aged, healthy old, or AD) mixed-model ANOVA indicated that trials preceding No-Go errors were faster than trials preceding correctly withheld No-Go responses (F(1,195) = 75.47, p < .001). The main effect of group was also significant (F(2,195) = 10.24, p < .001) as middle-aged adults were faster than both healthy older adults and AD individuals. Results showed no group by trial type interaction (F < 1.5). Turning to the z-transformed RTs, there was a reliable main effect of trial type (F(1,195) = 70.37, p < .001), but neither the main effect of group nor the group by trial type interaction approached significance (Fs < 1.5) in this pre-error speeding analysis.
Post-error slowing
Post-error reaction time refers to the response latency on the Go trial immediately following a No-Go error, whereas post-correct reaction time refers to the response latency on the trial following a correctly withheld No-Go response. It is hypothesized that post-error slowing reflects re-engaging the appropriate task set after an error is made. Reaction time patterns were analyzed in a 2 (Post-error vs. Post-correct) × 3 (healthy middle-aged, healthy old, AD) mixed-model ANOVA. Post-error response latencies were longer than post-correct response latencies (F(1,257) = 138.87, p < .001). Group also produced a significant main effect (F(2,257) = 9.80, p < .001): healthy middle-aged adults were faster than the other two groups. A significant group by trial type interaction was also found (F(2,257) = 3.25, p < .05). To fully explore the nature of this interaction, three separate 2 × 2 mixed-model ANOVAs were conducted. In the first analysis, only the two healthy groups were contrasted. This revealed significant main effects of trial type (F(1,217) = 135.54, p < .001) and age group (F(1,217) = 9.94, p < .01), and, most importantly, a significant group by trial type interaction (F(1,217) = 6.27, p < .05). This indicates that the difference between post-error and post-correct RTs was greater in the older group than in the younger, replicating and extending the pattern observed by Jackson and Balota (2012) when comparing young college students to older adults. In the second ANOVA, only the healthy older adults and the AD individuals were contrasted. A significant main effect of trial type (F(1,149) = 101.03, p < .001) and a marginal main effect of group (F(1,149) = 2.95, p = .088) were found, however, the group by trial type interaction was not reliable (F < 1). In the final ANOVA, the healthy middle-aged group was contrasted with the AD group. This indicated significant main effects of trial type (F(1,148) = 64.34, p < .001) and group (F(1,148) = 16.91, p < .001), but the group by trial type interaction was not reliable in this analysis either (F < 1.5). In sum, the results of the follow-up analyses suggest that healthy older adults were slowing disproportionately compared to younger healthy adults (i.e., the difference between post-error and post-correct RTs was bigger), but this tendency was not evident in AD individuals as there was no reliable difference between middle-aged and AD - or older and AD - adults.
Turning to the z-score analyses, there was a reliable main effect of trial type (F(1,257) = 125.83, p < .001) indicating slowing after errors and a marginal main effect of group (F(2,257) = 2.69, p = .070). Importantly, the group by trial type interaction was significant (F(2,257) = 3.83, p < .05). We conducted follow-up analyses on z-scores in a fashion similar to the analyses for the raw RTs. In the first ANOVA contrasting the healthy groups (middle-aged and older), a significant main effect of trial type (F(1,217) = 136.60, p < .001) and group (F(1,217) = 4.64, p < .05) were found. The group by trial type interaction was also reliable (F(1,217) = 6.11, p < .05). The second ANOVA contrasting the AD participants and the age-matched controls revealed a significant main effect of trial type (F(1,149) = 87.51, p < .001), but the main effect of group did not reach significance (F < 2.5). The group by trial type interaction was marginally significant (F(1,149) = 3.68, p = .057) suggesting that post-error slowing is slightly smaller in the AD individuals compared to their age-matched controls, when controlling for overall processing speed. In the final ANOVA contrasting healthy middle-aged adults and CDR .5 and 1 individuals, only a main effect of trial type was found (F(1,148) = 56.45, p < .001), with neither the main effect of group nor the interaction between group and condition approaching significance (both Fs < .5). These results corroborate the results of the analyses on raw RTs.
Self-reported mind-wandering
Mean proportions of different thought probe responses (on-task thought, space outs, zone outs, and tune outs) in each group are presented in Table 3 .
Mean proportions (SDs) of different thought reports across groups.
Because the distributions of thought report frequencies were skewed, Kruskal-Wallis H tests were used to examine the effect of age/CDR status on mind-wandering. Frequency of on-task thought reports was reliably different across groups (χ 2 (2) = 43.48, p < .001). Pairwise comparisons revealed that each group was significantly different from every other group (ps < .01). In other words, middle-aged adults reported less on-task thoughts than older adults who, in turn, reported less than AD individuals. Frequency of tune outs (mind-wandering with awareness) was also different across groups (χ 2 (2) = 43.94, p < .001). All three pairwise comparisons yielded significant results (ps < .01), thus tune outs decreased in frequency with age, and showed further decrease in the AD group. No compelling significant differences were found in the remaining two thought report categories (space outs and zone outs). 3
Personality, interest, difficulty, and working memory capacity
When the five factors of personality were examined, ANOVAs revealed significant differences between groups in Neuroticism (F(2,559) = 9.48, p < .001), Openness (F(2,559) = 18.31, p < .001), and Conscientiousness (F(2,559) = 8.17, p < .001). See Table 4 for descriptive data.
Descriptive data – means (SDs) – for middle-aged adults, healthy older adults, and early stage AD individuals for personality dimensions, and interest and difficulty ratings.
The significant difference in Neuroticism scores was driven by significant differences between the AD group and the two cognitively healthy groups (ps ≤ .010) with no reliable difference between the latter two groups (p = .095). In the case of Openness, healthy middle-aged adults scored significantly higher than both older groups (ps < .001); there was no significant difference between cognitively healthy older adults and the AD group (p = .111). AD individuals were significantly less conscientious than healthy individuals regardless of age (ps < .01), and there was no reliable difference between the two healthy groups. These patterns replicate Duchek et al. (2007) .
Investigating the relationship between Conscientiousness and SART performance controlling for CDR status, we found that Conscientiousness was negatively correlated with CoV (β = − .16, p < .001), and mean Go RT (β = − .09, p < .05), and positively with skill (β = .11, p < .01). However, conscientiousness was unrelated to self-reported mind-wandering (rho = .07 for on-task thought, and rho = −.06 for tune outs) using Spearman’s rho which does not control for covariates.
Interest ratings differed significantly across groups (χ 2 (2) = 25.21, p < .001). Each group differed significantly from every other group (ps < .05). Middle-aged adults found the task the least interesting, while the AD group found the SART significantly more interesting than the two healthy older adult groups. Difficulty ratings were also significantly different across groups (χ 2 (2) = 18.80, p < .001). The AD group rated the task more difficult than the two healthy groups (ps < .01). Interest and difficulty ratings were positively correlated in the sample (rho = .27, p < .001). Interest was weakly positively correlated with mean Go RT (rho = .09, p < .01), and on-task thought reports (rho = .19, p < .001). It was negatively correlated with tune outs (rho = − .16, p < .001). Perceived difficulty was positively correlated with CoV (rho = .17, p < .001). It was negatively correlated with No-Go accuracy (rho = − .30, p < .001), skill (rho = − .22, p < .001), and tune out frequency (rho = − .09, p < .05).
We also examined the relationship between MW reports and working memory. Across all participants and groups, on-task thought frequency was negatively correlated with reading span (rho = − . 18, p < .001), while tune out frequency was positively correlated with reading span (rho = .21, p < .001). However, neither of the correlations remained significant within groups. When group differences in MW were analyzed with reading span entered as a covariate, significant differences were still found both for on-task thoughts (F(2,403) = 5.45, p < .01) and for tune outs (F(2,403) = 6.50, p < .01).
The goal of the present study was to investigate mind-wandering (MW), as reflected by SART performance and self-reports, as a function of healthy aging and in early stage AD. Self-reports of mind-wandering decreased across groups with the youngest healthy group reporting the most off-task thought, older healthy adults reporting significantly less (replicating previous findings, see Maillet & Schacter, 2016a ), and the AD group reporting the least. With respect to behavioral indices of MW in the SART, no group differences were observed in pre-error speeding after adjusting for processing speed, but there were increases in the older adults compared to younger adults in the coefficient of variation and a skill index. Moreover, there was some evidence of a dissociation between age and AD, such that healthy older participants showed disproportionate slowing after errors compared to middle-aged participants, but the AD individuals did not show a further increase in post-error slowing, but rather showed a small decrease compared to their age-matched controls.
In the following two sections, we discuss two aspects of the present data. First, we discuss the subjective/self-reported MW indices. We then turn to the more objective measures available from the SART. We believe these two dimensions ultimately converge on a useful framework.
Subjective/Self-reported mind-wandering
We formulated two competing hypotheses regarding mind-wandering in early AD. The cognitive resource account of MW ( Smallwood & Schooler, 2006 ) predicts that AD individuals would report less MW because MW is a resource-dependent process and these individuals have depleted cognitive resources. A response style account would, however, predict that since early stage AD individuals are less conscientious than age-matched healthy controls ( Duchek et al., 2007) , they might show a less disciplined response style leading to more mind-wandering (see Jackson & Balota, 2012 ).
Our results support the cognitive resource hypothesis. We found not only an age-related decrease in tune out frequency in healthy participants, but also that AD individuals reported fewer tune outs and more on-task thought than did healthy age-matched controls, suggesting that early AD pathology affects mind-wandering rates above and beyond the effect of age. This could reflect the fact that these individuals have depleted cognitive resources and so need to allocate relatively more attentional resources to the task, and this could have led to more on-task thought reports. This is partly supported by the finding that AD individuals rated the task to be more difficult than both of the healthy groups. (However, it should also be noted that difficulty ratings were only very weakly related to frequency of tune outs, and were not related to on-task thought reports.)
Two further findings of the present study are also broadly consistent with the cognitive resource account. First, working memory capacity as indicated by reading span was found to decrease as a function of age and CDR status suggesting that these groups do indeed differ in the amount of cognitive resources they have at their disposal. Second, there was a positive relationship between MW and working memory capacity, suggesting that the more resources a person has the more likely they are to mind-wander during task performance. Alternatively, because this relationship only holds true for tune outs (i.e., MW with awareness) – this correlation could indicate that working memory capacity merely affects the ability to consciously report episodes of MW. Decline in working memory capacity, however, was only partially responsible for group differences in MW because differences between healthy controls and AD individuals were still present after controlling for working memory capacity. This suggests that some other factor, such as changes in metacognition (as discussed below) is also important in accounting for the AD-related decrease in self-reported MW.
Our results are inconsistent with the idea that episodes of MW reflect failures of executive control ( McVay & Kane, 2009 ; 2012b ) because both healthy and AD older adults would have been expected to report more off-task thoughts if this were the case. Furthermore, the non-negative association between MW and working memory capacity observed in our sample also speaks against this idea. An extension of this account is the “cognitive failure × current concerns” account ( McVay & Kane, 2010 ; 2012b ) which proposes that the occurrence of MW is not determined solely by the functioning of the executive control system but also by the number of competing personal goal-related thoughts a person has that might divert attention away from the primary task. Although this account can explain the typical pattern of age differences in MW comparing college students and older adults ( McVay & Kane, 2010 ; Jackson & Balota, 2012 ), additional assumptions would have to be made to account for the findings of the present study. Specifically, it is unclear why two groups of community dwelling healthy older adults (62 years and 77 years old) differing in age by only 15 years would have different levels of current concerns. Moreover, one would also need to assume that the early stage AD individuals have fewer current concerns or concerns triggered by a laboratory setting than healthy adults of similar age. Given their diagnosis, one might expect the early stage AD individuals to have more current concerns when coming for cognitive testing in a laboratory setting. Although it is possible that current concerns contributed to the current results, further work would need to be conducted to demonstrate a clear link between current concerns and the reports of MW in these groups.
The findings of the present study are in line with previous research suggesting breakdowns in the default mode network in early stages of AD ( Greicius et al., 2004 ; Lee et al., 2016 ; Lustig et al., 2003 ). This network is thought to play an important role in spontaneous cognition, including MW ( Andrews-Hanna et al., 2010 ; Andrews-Hanna, Smallwood, & Spreng, 2014 ; Christoff et al., 2009 , 2016 ; Durantin, Dehais, & Delorme, 2015 ; Mason et al., 2007 ; Stawarcyzk, Majerus, Maquet, & D’Argembeau, 2011). Specifically, it appears that the brain regions of the DMN overlap with those most vulnerable to deposition of the amyloid-β protein, an important pathological marker of AD ( Mormino et al., 2011 ; Ouchi & Kikuchi, 2012 ; Sperling et al., 2009 ), which may appear even in preclinical AD groups ( Sperling, Mormino, & Johnson, 2014 ). Therefore, it is possible that amyloid-β deposition disrupts DMN functioning, and this disruption directly affects the spontaneous generation of mental content, thus providing the neural basis for a decrease in MW frequency (e.g, Sheline et al., 2010 ). Although the present results are consistent with this perspective, it is important to note that the precise role of the DMN and its relation to MW in older adults remains unclear ( Maillet & Schacter, 2016b ). Specifically, DMN disruption might contribute to increased or decreased MW, changes in MW phenomenology ( Andrews-Hanna, Smallwood, & Spreng, 2014) , or perhaps even a change in an individual’s ability to report MW. Clearly, direct measures of DMN functional connectivity or DMN suppression during the SART in these groups would be necessary to directly examine this issue.
In accordance with previous studies ( Duberstein et al., 2011 ; Duchek et al., 2007 ) we found that AD individuals were more neurotic and less conscientious than healthy controls. Contrary to expectations, however, conscientiousness was unrelated to self-reports of MW in the present sample. This personality variable did show correlations with some measures of SART performance: conscientious participants were faster, less variable in their responses, and showed overall better performance. These results suggest that more conscientious individuals do indeed adopt a more disciplined response tendency in the SART as hypothesized by Jackson and Balota (2012) , but this does not affect how frequently they classify their thoughts as task-unrelated in self-report. As such, this cannot account for the decreased self-reported incidence of MW.
It is important to note that in this sample consisting largely of older individuals, participants rarely indicated experiencing that their mind was blank during the task (space out) or that they were unaware that their mind had wandered (zone out). Our results regarding self-reports of off-task thought only include frequency of tune outs, also known as mind-wandering with awareness. This raises the possibility that our findings merely reflect a decline in metacognitive awareness as a function of age and AD pathology, i.e., older adults and AD individuals might have difficulties monitoring their mental contents. Studies focusing on metacognition in AD outside the context of metamemory ( Cosentino, 2014 ) are needed to address this possibility.
As a final note, interest ratings were found to be related to both on-task thought reports and tune outs. Those who found the task more interesting disengaged less frequently. This is in accordance with previous studies ( Krawietz et al., 2012 ; Shake et al., 2015 ). Moreover, interest ratings were affected by both age and AD status – healthy middle-aged participants found the task the least interesting, while AD individuals found it more interesting than any other group. Controlling for interest, however, did not affect the age- and AD-effect in reported MW suggesting that the observed group differences are not simply attributable to greater interest in the task. This finding is important as it provides further support to the view that MW necessitates attentional resources, and that these resources modulate off-task thought, or perhaps metacognitive awareness of MW.
Behavioral performance on the SART
Although the self-reported indices of MW indicate that early stage AD appears to be a progression of healthy aging processes, this was not the case for all of the more objective measures. Reaction time to Go stimuli increased as a function of age in our sample, and there was further slowing in the early stage AD group above and beyond the effect of age. This, however, did not mean that the latter group adopted a more cautious response strategy: No-Go accuracy was the lowest in this group. Furthermore, the skill index ( Seli, 2016 ) similarly showed both an age-related decline and a separate effect of AD pathology. In other words, skill diminished with age, and further diminished as a function of AD status. The general pattern of results is consistent with previous findings that suggest that attentional control tasks can be used to effectively distinguish between healthy older adults and older adults in the earliest stages of AD ( Balota et al., 2010 ; Belleville, Bherer, Lepage, Chertkow, & Gauthier, 2008 ; Hutchison, Balota, & Duchek, 2010 ; Perry & Hodges, 1999 ; Spieler, Balota, & Faust, 1996 ).
We examined the two most commonly analyzed behavioral indices of MW in our sample: pre-error speeding, and post-error slowing. With respect to pre-error speeding, we found that participants were faster on trials preceding an error than on trials preceding a correctly withheld target trial. This has been proposed to reflect becoming disengaged from the task and responding in a mindless, automatic manner, leading to an error ( Smallwood & Schooler, 2006 ). In the present study, no group differences were found in the magnitude of this pre-error speeding. These results are in line with Jackson and Balota’s (2012) finding that there were no age-related changes in pre-error speeding in healthy adults. Furthermore, the data also imply that AD pathology has no effect on pre-error speeding.
Post-error slowing showed a more complex pattern across groups. First, focusing on the two healthy groups, we replicated and extended the results of Jackson and Balota (2012) showing greater post-error slowing in a group of older old adults compared to a slightly younger older adult group. Post-error slowing was present in AD individuals as well, but its magnitude was not reliably different from the younger healthy group and was marginally smaller than the older adult group after processing speed was controlled. In short, instead of showing an increase in post-error slowing, these individuals produced a slight decrease compared to their age-matched controls. If post-error slowing does in fact reflect task re-engagement following periods of inattention ( Cheyne et al., 2009 ; Jackson & Balota, 2012 ), it would seem this process might be deficient in AD older adults. This could be due to a number of reasons. For example, AD individuals may have trouble initiating the re-engagement of the task. In addition, because no feedback was given during the SART, it is possible that AD individuals are less likely to be aware of the errors and hence produce less post-error slowing. To our knowledge, error awareness in AD has not been investigated in a sustained attention paradigm thus far. In other paradigms, however, error awareness has been found to be both relatively intact (in lexical tasks; Ito & Kitagawa, 2005 ; Mathalon et al., 2003 ) and relatively impaired (in metamemory tasks, Cosentino, 2014 ) in AD individuals, and hence, there does not appear to be a clear consensus regarding predictions for the present paradigm. It is also possible that post-error slowing is a marker of a process other than task re-engagement. Notebaert et al. (2009) proposed that infrequent events such as errors lead to longer RTs because they capture attention and orient it away from the task. This re-orienting process may be deficient in the early AD group ( Balota & Faust, 2001 ; Perry & Hodges, 1999 ).
We also investigated behavioral variability across groups, because variability has been proposed to be a marker of mind-wandering ( Bastian & Sackur, 2013 ; Cheyne et al., 2009 ; Henríquez, Chica, Billeke, & Bartolomeo, 2016 ; Seli, Cheyne, & Smilek, 2013 ). Cheyne et al. (2009) suggested that RT variability is an indicator of “occurrent task inattention”, or brief attentional disengagements from the task at hand. In their model, these brief episodes of inattention are similar to tune outs in our study. Replicating previous studies not focusing on MW (e.g., Duchek et al., 2009 ; Hultsch, MacDonald, Hunter, Levy-Bencheton, & Strauss, 2000 ; Jackson, Balota, Duchek, & Head, 2012 ), we found that AD individuals produced a higher coefficient of variation (CoV) than did healthy controls. This would suggest that there is an increase in occurrent task inattention in the earliest stages of AD pathology. This, however, as reported above, was not reflected in higher rates of tune outs in these participants. In fact, the AD group reported tune outs with the lowest frequency. This could either reflect that self-reports on thought probes are not adequate reflections of the actual subjective experiences of early stage AD individuals, or that behavioral variability reflects other processes in addition to transient lapses in attention (e.g., changes in neural signal transmission; Duchek et al., 2009 ; Hultsch et al., 2000 ). The first alternative is closely related to metacognitive monitoring in AD mentioned above.
The present results extended past work showing decreased self-reported mind wandering in older adults, compared to younger adults. In addition, these results replicate the observation that while pre-error speeding does not show an age-related change, post-error slowing shows a disproportionate increase in older adults. Moreover, the older adults reported that they found the task more interesting compared to middle-aged adults, again replicating previous results. Importantly, these patterns were shown in two large cohorts of healthy community dwelling individuals, as opposed to typical aging studies comparing community dwelling older adults to college-aged samples, and hence, these age differences are not due to idiosyncratic characteristics of college-aged students. The self-reported mind wandering indices along with self-reported interest and difficulty changed further in early stage AD compared to age-matched older adults, suggesting AD is on a continuum with aging in these self-reports. However, there were some clear differences in the objective measures of SART performance. Specifically, we demonstrated an AD-specific increase in the CoV, and early stage AD individuals produced more comparable post-error slowing to the young old adults, in comparison to their age-matched older adults. We believe this latter effect, in conjunction with the self-reported increase in interest in the task, is most consistent with a decrease in self-monitoring in early stage AD that is specific to the AD pathophysiological cascade.

Post-target standardized response latencies as a function of group and trial type. Error bars represent +/− 1 SE.
Public significance
The present paper adds to the growing body of evidence that suggests that individuals in the early stages of Alzheimer’s disease show measurable changes in attention, a non-memory domain of cognition, by showing that these individuals report fewer episodes of inattention (mind-wandering) during a sustained attention task than healthy controls. Furthermore, our findings support the notion that self-reports of mind-wandering require cognitive resources, and are not simply a consequence of cognitive failures.
Acknowledgments
This study was funded by the following grants from the National Institute on Aging: P01AG03991 and P01AG026276.
1 We removed 4 additional participants whose data points lied outside the distribution of at least one of the main behavioral outcome variables (Go RT, No-Go RT, Pre-error RT, Pre-correct RT, Post-error RT, Post-correct RT) based on visual inspection of the histograms.
2 Because the group variable was also involved in a significant interaction, the main effect of group on RTs was further investigated in two separate between-subject ANOVAs for Go RT and No-Go RT. When Go RT was the dependent variable, there was a significant slowing in the AD group compared to the healthy older adult group (p < .05). When No-Go RT was the dependent variable, there was no significant difference between these two groups (p = .974).
3 The non-parametric tests also indicated a weakly significant difference between middle-aged adults and AD individuals in zone out frequency, but this effect is not interpreted further because it was not robust (p = .03). Associations between thought reports and behavioral performance (mean Go RT, No-Go accuracy, CV, skill index, pre-error RT z scores, and post-error RT z scores) were also investigated both in the whole sample and within each group. Some weak correlations were observed, all in the expected directions, however these results are not reported in detail because some thought report categories occurred with extremely low frequency thus distributions were heavily skewed. Furthermore, there was no clear age- or dementia status related change in the pattern of the relationships.
- Albert M, Moss MB, Blacker D, Tanzi R, McArdle JJ. Longitudinal change in cognitive performance among individuals with mild cognitive impairment. Neuropsychology. 2007; 21 (2):158–169. [ PubMed ] [ Google Scholar ]
- Andrews-Hanna JR, Reidler JS, Huang C, Buckner RL. Evidence for the default network’s role in spontaneous cognition. Journal of Neurophysiology. 2010; 104 (1):322–335. [ PMC free article ] [ PubMed ] [ Google Scholar ]
- Andrews- Hanna JR, Smallwood J, Spreng RN. The default network and selfgenerated thought: component processes, dynamic control, and clinical relevance. Annals of the New York Academy of Sciences. 2014; 1316 (1):29–52. [ PMC free article ] [ PubMed ] [ Google Scholar ]
- Balota DA, Faust M. Attention in Dementia of the Alzheimer’s Type. In: Boller F, Cappa S, editors. Handbook of Neuropsychology. 2. Vol. 6. New York, NY: Elsevier Science; 2001. pp. 51–80. [ Google Scholar ]
- Balota DA, Tse CS, Hutchison KA, Spieler DH, Duchek JM, Morris JC. Predicting conversion to dementia of the Alzheimer’s type in a healthy control sample: The power of errors in Stroop color naming. Psychology and Aging. 2010; 25 (1):208–218. [ PMC free article ] [ PubMed ] [ Google Scholar ]
- Bastian M, Sackur J. Mind-wandering at the fingertips: automatic parsing of subjective states based on response time variability. Frontiers in Psychology. 2013; 4 :573. [ PMC free article ] [ PubMed ] [ Google Scholar ]
- Belleville S, Bherer L, Lepage É, Chertkow H, Gauthier S. Task switching capacities in persons with Alzheimer’s disease and mild cognitive impairment. Neuropsychologia. 2008; 46 (8):2225–2233. [ PubMed ] [ Google Scholar ]
- Buckner RL. The serendipitous discovery of the brain’s default network. Neuroimage. 2012; 62 (2):1137–1145. [ PubMed ] [ Google Scholar ]
- Buschke H. Selective reminding for analysis of memory and learning. Journal of Verbal Learning and Verbal Behavior. 1973; 12 (5):543–550. [ Google Scholar ]
- Cheyne JA, Carriere JS, Solman GJ, Smilek D. Challenge and error: Critical events and attention-related errors. Cognition. 2011; 121 (3):437–446. [ PubMed ] [ Google Scholar ]
- Cheyne JA, Solman GJ, Carriere JS, Smilek D. Anatomy of an error: A bidirectional state model of task engagement/disengagement and attention-related errors. Cognition. 2009; 111 (1):98–113. [ PubMed ] [ Google Scholar ]
- Christoff K, Gordon AM, Smallwood J, Smith R, Schooler JW. Experience sampling during fMRI reveals default network and executive system contributions to mind wandering. Proceedings of the National Academy of Sciences. 2009; 106 (21):8719–8724. [ PMC free article ] [ PubMed ] [ Google Scholar ]
- Christoff K, Irving ZC, Fox KC, Spreng RN, Andrews-Hanna JR. Mind-wandering as spontaneous thought: a dynamic framework. Nature Reviews Neuroscience. 2016; 17 (11):718–731. [ PubMed ] [ Google Scholar ]
- Cosentino SA. Metacognition in Alzheimer’s disease. In: Fleming SM, Frith CD, editors. The Cognitive Neuroscience of Metacognition. Berlin, Heidelberg: Springer Berlin Heidelberg; 2014. pp. 389–407. [ Google Scholar ]
- Costa PT, McCrae RR. Revised NEO personality inventory (NEO PI-R) and NEO five-factor inventory (NEO FFI): Professional manual. Odessa, FL: Psychological Assessment Resources; 1992. [ Google Scholar ]
- Craik FI. A functional account of age differences in memory. In: Klix F, Hagendorf H, editors. Human memory and cognitive capabilities: Mechanisms and performances. Amsterdam, North-Holland: Elsevier Science Publishing Company; 1986. pp. 409–422. [ Google Scholar ]
- Cunningham S, Scerbo MW, Freeman FG. The electrocortical correlates of daydreaming during vigilance tasks. Journal of Mental Imagery. 2000; 24 (1–2):61–72. [ Google Scholar ]
- Daneman M, Carpenter PA. Individual differences in working memory and reading. Journal of Verbal Learning and Verbal Behavior. 1980; 19 (4):450–466. [ Google Scholar ]
- Duberstein PR, Chapman BP, Tindle HA, Sink KM, Bamonti P, Robbins J, Jerant AF, Franks P. Personality and risk for Alzheimer’s disease in adults 72 years of age and older: a 6-year follow-up. Psychology and Aging. 2011; 26 (2):351–362. [ PMC free article ] [ PubMed ] [ Google Scholar ]
- Duchek JM, Balota DA, Storandt M, Larsen R. The power of personality in discriminating between healthy aging and early-stage Alzheimer’s disease. The Journals of Gerontology, Series B: Psychological Sciences and Social Sciences. 2007; 62 (6):353–361. [ PubMed ] [ Google Scholar ]
- Duchek JM, Balota DA, Tse CS, Holtzman DM, Fagan AM, Goate AM. The utility of intraindividual variability in selective attention tasks as an early marker for Alzheimer’s disease. Neuropsychology. 2009; 23 (6):746–758. [ PMC free article ] [ PubMed ] [ Google Scholar ]
- Durantin G, Dehais F, Delorme A. Characterization of mind wandering using fNIRS. Frontiers in Systems Neuroscience. 2015; 9 :45. [ PMC free article ] [ PubMed ] [ Google Scholar ]
- Faust ME, Balota DA. Inhibition, Facilitation, and Attention Control in Dementia of the Alzheimer Type: The role of unifying principles in cognitive theory development. In: Gorfein DS, MacLeod CM, editors. Inhibition in cognition. Washington, DC: American Psychological Association; 2007. pp. 213–238. [ Google Scholar ]
- Faust ME, Balota DA, Spieler DH, Ferraro FR. Individual differences in information-processing rate and amount: implications for group differences in response latency. Psychological Bulletin. 1999; 125 (6):777–799. [ PubMed ] [ Google Scholar ]
- Folstein MF, Folstein SE, McHugh PR. “Mini-mental state”: a practical method for grading the cognitive state of patients for the clinician. Journal of Psychiatric Research. 1975; 12 (3):189–198. [ PubMed ] [ Google Scholar ]
- Fox KC, Spreng RN, Ellamil M, Andrews-Hanna JR, Christoff K. The wandering brain: Meta-analysis of functional neuroimaging studies of mind-wandering and related spontaneous thought processes. Neuroimage. 2015; 111 :611–621. [ PubMed ] [ Google Scholar ]
- Frank DJ, Nara B, Zavagnin M, Touron DR, Kane MJ. Validating older adults’ reports of less mind-wandering: An examination of eye movements and dispositional influences. Psychology and Aging. 2015; 30 (2):266–278. [ PubMed ] [ Google Scholar ]
- Giambra LM. Task-unrelated-thought frequency as a function of age: A laboratory study. Psychology and Aging. 1989; 4 (2):136–143. [ PubMed ] [ Google Scholar ]
- Giambra LM. The influence of aging on spontaneous shifts of attention from external stimuli to the contents of consciousness. Experimental Gerontology. 1993; 28 (4):485–492. [ PubMed ] [ Google Scholar ]
- Giambra L. Daydreaming characteristics across the life-span: Age differences and seven to twenty year longitudinal changes. In: Kunzendorf RG, Wallace B, editors. Individual differences in conscious experience. Amsterdam, Netherlands: John Benjamins Publishing Company; 2000. pp. 147–206. [ Google Scholar ]
- Greicius MD, Srivastava G, Reiss AL, Menon V. Default-mode network activity distinguishes Alzheimer’s disease from healthy aging: evidence from functional MRI. Proceedings of the National Academy of Sciences. 2004; 101 (13):4637–4642. [ PMC free article ] [ PubMed ] [ Google Scholar ]
- Henríquez RA, Chica AB, Billeke P, Bartolomeo P. Fluctuating minds: spontaneous psychophysical variability during mind-wandering. PLoS One. 2016; 11 (2):e0147174. [ PMC free article ] [ PubMed ] [ Google Scholar ]
- Hultsch DF, MacDonald SW, Hunter MA, Levy-Bencheton J, Strauss E. Intraindividual variability in cognitive performance in older adults: comparison of adults with mild dementia, adults with arthritis, and healthy adults. Neuropsychology. 2000; 14 (4):588–598. [ PubMed ] [ Google Scholar ]
- Hutchison KA, Balota DA, Duchek JM. The utility of Stroop task switching as a marker for early-stage Alzheimer’s disease. Psychology and Aging. 2010; 25 (3):545–559. [ PMC free article ] [ PubMed ] [ Google Scholar ]
- Ito J, Kitagawa J. Error processing in patients with Alzheimer’s disease. Pathophysiology. 2005; 12 (2):97–101. [ PubMed ] [ Google Scholar ]
- Jackson JD, Balota DA. Mind-wandering in younger and older adults: converging evidence from the Sustained Attention to Response Task and reading for comprehension. Psychology and Aging. 2012; 27 (1):106–119. [ PMC free article ] [ PubMed ] [ Google Scholar ]
- Jackson JD, Balota DA, Duchek JM, Head D. White matter integrity and reaction time intraindividual variability in healthy aging and early-stage Alzheimer disease. Neuropsychologia. 2012; 50 (3):357–366. [ PMC free article ] [ PubMed ] [ Google Scholar ]
- Jackson JD, Weinstein Y, Balota DA. Can mind-wandering be timeless? Atemporal focus and aging in mind-wandering paradigms. Frontiers in Psychology. 2013; 4 :742. [ PMC free article ] [ PubMed ] [ Google Scholar ]
- Kane MJ, Brown LH, McVay JC, Silvia PJ, Myin-Germeys I, Kwapil TR. For whom the mind wanders, and when: An experience-sampling study of working memory and executive control in daily life. Psychological Science. 2007; 18 (7):614–621. [ PubMed ] [ Google Scholar ]
- Killingsworth MA, Gilbert DT. A wandering mind is an unhappy mind. Science. 2010; 330 (6006):932. [ PubMed ] [ Google Scholar ]
- Kirschner A, Kam JWY, Handy TC, Ward LM. Differential synchronization in default and task-specific networks of the human brain. Frontiers in Human Neuroscience. 2012; 6 :139. [ PMC free article ] [ PubMed ] [ Google Scholar ]
- Krawietz SA, Tamplin AK, Radvansky GA. Aging and mind wandering during text comprehension. Psychology and Aging. 2012; 27 (4):951–958. [ PubMed ] [ Google Scholar ]
- Lee ES, Yoo K, Lee YB, Chung J, Lim JE, Yoon B, Jeong Y. Default Mode Network Functional Connectivity in Early and Late Mild Cognitive Impairment: Results From the Alzheimer’s Disease Neuroimaging Initiative. Alzheimer Disease and Associated Disorders. 2016; 30 (4):289–296. [ PubMed ] [ Google Scholar ]
- Lustig C, Snyder AZ, Bhakta M, O’Brien KC, McAvoy M, Raichle ME, Morris JC, Buckner RL. Functional deactivations: change with age and dementia of the Alzheimer type. Proceedings of the National Academy of Sciences. 2003; 100 (24):14504–14509. [ PMC free article ] [ PubMed ] [ Google Scholar ]
- Maillet D, Schacter DL. From mind wandering to involuntary retrieval: Age-related differences in spontaneous cognitive processes. Neuropsychologia. 2016a; 80 :142–156. [ PMC free article ] [ PubMed ] [ Google Scholar ]
- Maillet D, Schacter DL. Default network and aging: Beyond the task-negative perspective. Trends in Cognitive Sciences. 2016b; 20 (9):646–648. [ PMC free article ] [ PubMed ] [ Google Scholar ]
- Mason MF, Norton MI, Van Horn JD, Wegner DM, Grafton ST, Macrae CN. Wandering minds: the default network and stimulus-independent thought. Science. 2007; 315 (5810):393–395. [ PMC free article ] [ PubMed ] [ Google Scholar ]
- Mathalon DH, Bennett A, Askari N, Gray EM, Rosenbloom MJ, Ford JM. Response-monitoring dysfunction in aging and Alzheimer’s disease: an event-related potential study. Neurobiology of Aging. 2003; 24 (5):675–685. [ PubMed ] [ Google Scholar ]
- McKhann G, Drachman D, Folstein M, Katzman R, Price D, Stadlan EM. Clinical diagnosis of Alzheimer’s disease: Report of the NINCDS-ADRDA Work Group under the auspices of the Department of Health and Human Services Task Force on Alzheimer’s Disease. Neurology. 1984; 34 :934–939. [ PubMed ] [ Google Scholar ]
- McVay JC, Kane MJ. Conducting the train of thought: working memory capacity, goal neglect, and mind wandering in an executive-control task. Journal of Experimental Psychology: Learning, Memory, and Cognition. 2009; 35 (1):196–204. [ PMC free article ] [ PubMed ] [ Google Scholar ]
- McVay JC, Kane MJ. Does mind wandering reflect executive function or executive failure? Comment on Smallwood and Schooler (2006) and Watkins (2008) Psychological Bulletin. 2010; 136 (2):188–197. [ PMC free article ] [ PubMed ] [ Google Scholar ]
- McVay JC, Kane MJ. Drifting from slow to “d’oh!”: Working memory capacity and mind wandering predict extreme reaction times and executive control errors. Journal of Experimental Psychology: Learning, Memory, and Cognition. 2012a; 38 (3):525–549. [ PMC free article ] [ PubMed ] [ Google Scholar ]
- McVay JC, Kane MJ. Why does working memory capacity predict variation in reading comprehension? On the influence of mind wandering and executive attention. Journal of Experimental Psychology: General. 2012b; 141 (2):302–320. [ PMC free article ] [ PubMed ] [ Google Scholar ]
- McVay JC, Kane MJ, Kwapil TR. Tracking the train of thought from the laboratory into everyday life: An experience-sampling study of mind wandering across controlled and ecological contexts. Psychonomic Bulletin & Review. 2009; 16 (5):857–863. [ PMC free article ] [ PubMed ] [ Google Scholar ]
- McVay JC, Meier ME, Touron DR, Kane MJ. Aging ebbs the flow of thought: Adult age differences in mind wandering, executive control, and self-evaluation. Acta Psychologica. 2013; 142 (1):136–147. [ PMC free article ] [ PubMed ] [ Google Scholar ]
- Mormino EC, Smiljic A, Hayenga AO, Onami SH, Greicius MD, Rabinovici GD, Janabi M, Baker SL, Yen IV, Madison CM, Miller BL, Jagust WJ. Relationships between beta-amyloid and functional connectivity in different components of the default mode network in aging. Cerebral Cortex. 2011; 21 (10):2399–2407. [ PMC free article ] [ PubMed ] [ Google Scholar ]
- Morris JC. The Clinical Dementia Rating (CDR): current version and scoring rules. Neurology. 1993; 43 (11):2412–2414. [ PubMed ] [ Google Scholar ]
- Notebaert W, Houtman F, Van Opstal F, Gevers W, Fias W, Verguts T. Post-error slowing: an orienting account. Cognition. 2009; 111 (2):275–279. [ PubMed ] [ Google Scholar ]
- Ouchi Y, Kikuchi M. A review of the default mode network in aging and dementia based on molecular imaging. Reviews in the Neurosciences. 2012; 23 (3):263–268. [ PubMed ] [ Google Scholar ]
- Parks CW, Klinger E, Perlmutter M. Dimensions of thought as a function of age, gender and task difficulty. Imagination, Cognition and Personality. 1988; 8 (1):49–62. [ Google Scholar ]
- Perry RJ, Hodges JR. Attention and executive deficits in Alzheimer’s disease: A critical review. Brain. 1999; 122 (3):383–404. [ PubMed ] [ Google Scholar ]
- Raichle ME, MacLeod AM, Snyder AZ, Powers WJ, Gusnard DA, Shulman GL. A default mode of brain function. Proceedings of the National Academy of Sciences. 2001; 98 (2):676–682. [ PMC free article ] [ PubMed ] [ Google Scholar ]
- Robertson IH, Manly T, Andrade J, Baddeley BT, Yiend J. ‘Oops!’: performance correlates of everyday attentional failures in traumatic brain injured and normal subjects. Neuropsychologia. 1997; 35 (6):747–758. [ PubMed ] [ Google Scholar ]
- Salthouse TA. When does age-related cognitive decline begin? Neurobiology of Aging. 2009; 30 (4):507–514. [ PMC free article ] [ PubMed ] [ Google Scholar ]
- Sayette MA, Reichle ED, Schooler JW. Lost in the sauce: The effects of alcohol on mind wandering. Psychological Science. 2009; 20 (6):747–752. [ PMC free article ] [ PubMed ] [ Google Scholar ]
- Schooler JW, Reichle ED, Halpern DV. Zoning-out while reading: Evidence for dissociations between experience and meta-consciousness. In: Levin DT, editor. Thinking and seeing: Visual metacognition in adults and children. Cambridge, MA: MIT Press; 2004. pp. 203–226. [ Google Scholar ]
- Schooler JW, Smallwood J, Christoff K, Handy TC, Reichle ED, Sayette MA. Meta-awareness, perceptual decoupling and the wandering mind. Trends in Cognitive Sciences. 2011; 15 (7):319–326. [ PubMed ] [ Google Scholar ]
- Seli P. The Attention-Lapse and Motor Decoupling accounts of SART performance are not mutually exclusive. Consciousness and Cognition. 2016; 41 :189–198. [ PubMed ] [ Google Scholar ]
- Seli P, Cheyne JA, Smilek D. Wandering minds and wavering rhythms: Linking mind wandering and behavioral variability. Journal of Experimental Psychology: Human Perception and Performance. 2013; 39 (1):1–5. [ PubMed ] [ Google Scholar ]
- Seli P, Jonker TR, Solman GJ, Cheyne JA, Smilek D. A methodological note on evaluating performance in a Sustained-Attention-To-Response Task. Behavior Research Methods. 2013; 45 (2):355–363. [ PubMed ] [ Google Scholar ]
- Shake MC, Shulley LJ, Soto-Freita AM. Effects of individual differences and situational features on age differences in mindless reading. Journals of Gerontology, Series B: Psychological Sciences and Social Sciences. 2015; 71 (5):808–820. [ PubMed ] [ Google Scholar ]
- Sheline YI, Raichle ME, Snyder AZ, Morris JC, Head D, Wang S, Mintun MA. Amyloid plaques disrupt resting state default mode network connectivity in cognitively normal elderly. Biological Psychiatry. 2010; 67 (6):584–587. [ PMC free article ] [ PubMed ] [ Google Scholar ]
- Shulman GL, Fiez JA, Corbetta M, Buckner RL, Miezin FM, Raichle ME, Petersen SE. Common blood flow changes across visual tasks: II. Decreases in cerebral cortex. Journal of Cognitive Neuroscience. 1997; 9 (5):648–663. [ PubMed ] [ Google Scholar ]
- Singer J, McCraven V. Some characteristics of adult daydreaming. Journal of Psychology. 1961; 51 :151–164. [ Google Scholar ]
- Smallwood J, Beach E, Schooler JW, Handy TC. Going AWOL in the brain: Mind wandering reduces cortical analysis of external events. Journal of Cognitive Neuroscience. 2008; 20 (3):458–469. [ PubMed ] [ Google Scholar ]
- Smallwood J, Davies JB, Heim D, Finnigan F, Sudberry M, O’Connor R, Obonsawin M. Subjective experience and the attentional lapse: Task engagement and disengagement during sustained attention. Consciousness and Cognition. 2004; 13 (4):657–690. [ PubMed ] [ Google Scholar ]
- Smallwood J, McSpadden M, Schooler JW. The lights are on but no one’s home: Meta-awareness and the decoupling of attention when the mind wanders. Psychonomic Bulletin & Review. 2007; 14 (3):527–533. [ PubMed ] [ Google Scholar ]
- Smallwood J, McSpadden M, Schooler JW. When attention matters: The curious incident of the wandering mind. Memory & Cognition. 2008; 36 (6):1144–1150. [ PubMed ] [ Google Scholar ]
- Smallwood J, Schooler JW. The restless mind. Psychological Bulletin. 2006; 132 (6):946–958. [ PubMed ] [ Google Scholar ]
- Smallwood J, Schooler JW. The science of mind wandering: empirically navigating the stream of consciousness. Annual Review of Psychology. 2015; 66 :487–518. [ PubMed ] [ Google Scholar ]
- Sperling RA, LaViolette PS, O’Keefe K, O’Brien J, Rentz DM, Pihlajamaki M, Marshall G, Hyman BT, Selkoe DJ, Hedden T, Buckner RL, Becker JA, Johnson KA. Amyloid deposition is associated with impaired default network function in older persons without dementia. Neuron. 2009; 63 (2):178–188. [ PMC free article ] [ PubMed ] [ Google Scholar ]
- Sperling R, Mormino E, Johnson K. The evolution of preclinical Alzheimer’s disease: implications for prevention trials. Neuron. 2014; 84 (3):608–622. [ PMC free article ] [ PubMed ] [ Google Scholar ]
- Spieler DH, Balota DA, Faust ME. Stroop performance in healthy younger and older adults and in individuals with dementia of the Alzheimer’s type. Journal of Experimental Psychology: Human Perception and Performance. 1996; 22 (2):461–479. [ PubMed ] [ Google Scholar ]
- Staub B, Doignon-Camus N, Bacon E, Bonnefond A. Investigating sustained attention ability in the elderly by using two different approaches: inhibiting ongoing behavior versus responding on rare occasions. Acta Psychologica. 2014; 146 :51–57. [ PubMed ] [ Google Scholar ]
- Stawarczyk D, Majerus S, Maj M, Van der Linden M, D’Argembeau A. Mind-wandering: phenomenology and function as assessed with a novel experience sampling method. Acta Psychologica. 2011; 136 (3):370–381. [ PubMed ] [ Google Scholar ]
- Stawarczyk D, Majerus S, Maquet P, D’Argembeau A. Neural correlates of ongoing conscious experience: both task-unrelatedness and stimulus-independence are related to default network activity. PloS One. 2011; 6 (2):e16997. [ PMC free article ] [ PubMed ] [ Google Scholar ]
- Storandt M, Grant EA, Miller JP, Morris JC. Longitudinal course and neuropathologic outcomes in original vs revised MCI and in pre-MCI. Neurology. 2006; 67 (3):467–473. [ PubMed ] [ Google Scholar ]
- Zavagnin M, Borella E, De Beni R. When the mind wanders: Age-related differences between young and older adults. Acta Psychologica. 2014; 145 :54–64. [ PubMed ] [ Google Scholar ]
Loading metrics
Open Access
Peer-reviewed
Short Reports
Short Reports offer exciting novel biological findings that may trigger new research directions.
See Journal Information »
Neuronal cell cycle reentry events in the aging brain are more prevalent in neurodegeneration and lead to cellular senescence
Roles Conceptualization, Data curation, Formal analysis, Investigation, Methodology, Writing – original draft
Affiliation School of Life Sciences, Faculty of Science, The Chinese University of Hong Kong, Hong Kong SAR, China
Roles Data curation
Roles Conceptualization, Data curation, Formal analysis, Funding acquisition, Investigation, Methodology, Project administration, Resources, Software, Supervision, Validation, Visualization, Writing – original draft, Writing – review & editing
* E-mail: [email protected]
Affiliations School of Life Sciences, Faculty of Science, The Chinese University of Hong Kong, Hong Kong SAR, China, Gerald Choa Neuroscience Institute, The Chinese University of Hong Kong, Hong Kong SAR, China, Nexus of Rare Neurodegenerative Diseases, The Chinese University of Hong Kong, Hong Kong SAR, China

- Deng Wu,
- Jacquelyne Ka-Li Sun,
- Kim Hei-Man Chow
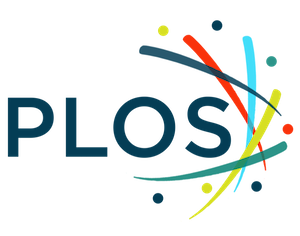
- Published: April 23, 2024
- https://doi.org/10.1371/journal.pbio.3002559
- Peer Review
- Reader Comments
Increasing evidence indicates that terminally differentiated neurons in the brain may recommit to a cell cycle-like process during neuronal aging and under disease conditions. Because of the rare existence and random localization of these cells in the brain, their molecular profiles and disease-specific heterogeneities remain unclear. Through a bioinformatics approach that allows integrated analyses of multiple single-nucleus transcriptome datasets from human brain samples, these rare cell populations were identified and selected for further characterization. Our analyses indicated that these cell cycle-related events occur predominantly in excitatory neurons and that cellular senescence is likely their immediate terminal fate. Quantitatively, the number of cell cycle re-engaging and senescent neurons decreased during the normal brain aging process, but in the context of late-onset Alzheimer’s disease (AD), these cells accumulate instead. Transcriptomic profiling of these cells suggested that disease-specific differences were predominantly tied to the early stage of the senescence process, revealing that these cells presented more proinflammatory, metabolically deregulated, and pathology-associated signatures in disease-affected brains. Similarly, these general features of cell cycle re-engaging neurons were also observed in a subpopulation of dopaminergic neurons identified in the Parkinson’s disease (PD)-Lewy body dementia (LBD) model. An extended analysis conducted in a mouse model of brain aging further validated the ability of this bioinformatics approach to determine the robust relationship between the cell cycle and senescence processes in neurons in this cross-species setting.
Citation: Wu D, Sun JK-L, Chow KH-M (2024) Neuronal cell cycle reentry events in the aging brain are more prevalent in neurodegeneration and lead to cellular senescence. PLoS Biol 22(4): e3002559. https://doi.org/10.1371/journal.pbio.3002559
Academic Editor: Jing Qu, State Key Laboratory of Stem Cell and Reproductive Biology, Chinese Academy of Sciences, CHINA
Received: November 22, 2023; Accepted: February 22, 2024; Published: April 23, 2024
Copyright: © 2024 Wu et al. This is an open access article distributed under the terms of the Creative Commons Attribution License , which permits unrestricted use, distribution, and reproduction in any medium, provided the original author and source are credited.
Data Availability: All original codes and metadata for each individual Figure are available in: https://zenodo.org/doi/10.5281/zenodo.10604562 .
Funding: The work was supported, in part, by grants from the following: The Hong Kong Research Grants Council (RGC)-General Research Fund (GRF) (PI: ECS24107121, GRF16100219 and GRF16100718) (all to K.H-M.C) and the RGC- Collaborative Research Fund (CRF) (Co-I: C4033-19EF) (K.H-M.C); the National NaturalScience Foundation-Excellent Young Scientists Fund 2020 (Ref: 32022087) (K.H-M.C); Alzheimer's Association Research Fellowship (PI: AARF-17-531566) (K.H-M.C). All the funders had no role in study design, data collection and analysis, decision to publish, or preparation of the manuscript.
Competing interests: The authors have declared that no competing interests exist.
Abbreviations: AD, Alzheimer’s disease; CDK, cyclin-dependent kinase; CNA, copy number alteration; CNV, copy number variation; DDR, DNA damage response; ES, early senescent; EST, expressed sequence tag; HMM, hidden Markov model; ND, nondemented; PD, Parkinson’s disease; LBD, Lewy-body demential; LS, late senescence; OPC, oligodendrocyte precursor cell; PC, principal component; PCNA, proliferating cell nuclear antigen; RADC, Rush Alzheimer’s Disease Centre; rPCA, reciprocal principal component analysis; ROS, Religious Order Study; ROSMAP, Rush Memory and Aging Project; snRNA-seq, single-nucleus RNA sequencing; t-SNE, t-distributed stochastic neighbor embedding
Terminally differentiated neurons are believed to have irreversibly exited from the cell division process [ 1 ], and by various means, these cells obtain their postmitotic identity [ 2 , 3 ]. Nonetheless, a large body of work has indicated that as individuals age, a small population of these cells may recommit to a cell cycle-like process [ 4 ], and increasing levels of these events are found in disease-affected brains of patients with late-onset Alzheimer’s disease (AD) [ 4 – 11 ]. While the reprogression and completion of the entire process of mitosis has never been reported, evidence related to the reexpression of cell cycle proteins [ 6 – 11 ] and somatic copy number variations (CNVs) [ 12 ] have been found [ 13 – 15 ], suggesting that a partial duplication of genomic materials might have co-occurred even though this process is likely prematurely halted by the activation of cell cycle checkpoint signaling through the physiological surveillance response [ 16 ]. While some studies have suggested that the rapid reexpression of cell cycle-related genes in neurons immediately leads to cell death and apoptosis [ 17 , 18 ], other reports have indicated that this phenomenon is instead a possible driver of the cellular senescence response [ 19 – 21 ]. To our knowledge, it remains unclear whether these consequences are mutually exclusive or instead a sequela of one another. Due to the randomness of the emerging locations and the limited quantities of these cells in the brain, their full characterization and molecular profiling via traditional histology-based or bulk tissue transcriptomic sequencing techniques have been challenging. To date, it remains unclear how these cell cycle re-engaging neurons may remain viable in the brain for months or even years [ 22 , 23 ] and whether they exhibit any disease-specific properties resulting from the varying brain microenvironments.
By taking advantage of the wealth of human brain single-nucleus RNA sequencing (snRNA-seq) datasets available in public repositories, we developed an analytical pipeline that facilitates the identification and characterization of cell cycle gene reexpressing neurons ( Fig 1A ) to address these questions. For this analysis, the cell cycle gene expression status of each single nucleus was identified to determine whether any postmitotic cell clusters exhibited enhanced expression of these genes. The related findings were subsequently validated by the InferCNV algorithm, as potential DNA duplication or deletion events might be due to aberrant cell cycle activity [ 24 ]. Subsequently, the identities of the target cells of interest were further characterized via cell fate trajectory analysis to uncover their origins and evolutionary relationships. To evaluate the possible roles of these genes in disease pathogenesis and phenotypic heterogeneity, we performed quantitative analysis of their relative numbers, and qualitatively, differential gene expression analysis was conducted for nuclei from control versus disease-affected samples.
- PPT PowerPoint slide
- PNG larger image
- TIFF original image
( A ) Schematic diagram illustrating the general layout and design of the bioinformatics analyses applied in this study. ( B ) t-SNE plot of a total of 34,140 nuclei derived from 24 unaffected, age (mean ± SD = 85.59 ± 4.20), and sex-matched (12 males and 12 females) prefrontal cortex samples from Brodmann area 10 [ 28 ]. The different cell types used are abbreviated as follows: excitatory neurons (Ex), oligodendrocytes (Oli), inhibitory neurons (In), astrocytes (Ast), microglia (Mic), endothelial cells (En), and oligodendrocyte progenitor cells (Opc). ( C ) Normalized expression levels of cell type-specific markers in all cell types mentioned above. ( D ) t-SNE plot of 32,685 postmitotic cell nuclei colored according to cell type (left panel) and subcluster number (right panel). ( E ) Schematic illustration of the cell cycle phase scoring workflow for each nucleus. ( F ) Violin plots showing the distribution of the cell cycle phase scores in all postmitotic cell subclusters. The bold highlights indicate the subcluster with the most significant differences above the average gene expression levels in any particular cell cycle phase. P values against other subclusters are shown on the right. ( G ) Estimation of copy number variants among all excitatory neurons via the InferCNV algorithm. The heatmap on the left shows the CNA regions identified by the HMM, i.e., regions of gain (red) and loss (blue) of expression along each chromosome, at various regions from the p-arm (left side of each box) to the q-arm (right side of each box) in all subclusters. The middle heatmap is an outcome of the Bayesian latent-mixture model implemented to identify the posterior probabilities of alteration status in each cell and whole CNA region. True positive predictions of CNV events were identified only in subcluster 13 (red: gain of copy number; blue: loss of copy number). On the far right are line plots illustrating in detail how gene expression levels on each chromosome are altered in subclusters 13 and 5 compared to a negative control (subcluster 2). The metadata underlying this figure can be found at https://zenodo.org/doi/10.5281/zenodo.10604562 . CNA, copy number alteration; CNV, copy number variation; HMM, hidden Markov model; t-SNE, t-distributed stochastic neighbor embedding.
https://doi.org/10.1371/journal.pbio.3002559.g001
Identification of cell cycle re-engaging neurons in human brain snRNA-seq datasets
The human adult brain is composed of terminally differentiated cells, such as excitatory and inhibitory neurons [ 25 ], myelinated oligodendrocytes [ 26 ], and mature astrocytes [ 27 ], as well as other cells that readily exhibit proliferative propensities [e.g., oligodendrocyte precursor cells (OPCs), microglia, and endothelial cells]. To investigate whether the postmitotic status of these terminally differentiated cells was faithfully attained in aged healthy brains, we analyzed the snRNA-seq data of a discovery cohort [ 28 ] of 24 age- and sex-matched samples (Brodmann area 10 of the prefrontal cortex) harvested from individuals without dementia (ND) ( S1 Table ). Among a total of 34,140 nuclei ( Fig 1B ) in which all the cell types mentioned were identified based on various established markers ( Fig 1C ), 32,685 nuclei belonging to terminally differentiated cell types were selected and divided into 20 smaller subclusters ( Fig 1D ). Among these nuclei, those that had robustly re-expressed cell cycle genes were identified using the AddModuleScore function of Seurat, as each nucleus was assigned a “cell cycle phase score” based on the expression levels of a refined list of 357 cell cycle-related genes ( S2 Table ) collated from the Whitfield and colleagues [ 29 ] and Tirosh and colleagues [ 30 ] studies ( S2 Table ), after which any hypothetical proteins, expressed sequence tags (ESTs) or alias names corresponding to the official gene symbols were screened out. After the corresponding cell cycle phase scores were assigned to each nucleus of all postmitotic cells, significant inductions in cell cycle genes were observed only among subclusters of excitatory neuronal nuclei [e.g., subclusters 5 (G1/S phase) and 13 (S, G2, G2/M and M/G1 phases)] ( Fig 1F ). For other postmitotic cell types, such as inhibitory neurons, myelinated oligodendrocytes, and mature astrocytes, this phenomenon was not found ( Fig 1F ).
Since the cell cycle is an ordered sequence of events [ 16 ], the enrichment of genes belonging to and downstream of the S phase in cell nuclei from only subcluster 13 suggested that these cells might have at least partially duplicated their DNA materials [ 31 ]. To predict whether such genome-level changes exist by studying the single-cell transcriptomic data, we used the inferring copy number variations (InferCNV) algorithm [ 32 ]. Among all the excitatory neuronal cell clusters, subcluster 13 was the only subcluster in which the InferCNV heatmaps predicted segmented CNVs ( Fig 1G , t test, P < 2.2e-16). In particular, significant gains in CNV scores were readily apparent across chromosomes 19 and 22 ( Fig 1G , middle panel). Consistent with the cell cycle analysis, similar CNV signals were not detected among the other postmitotic cell types ( S1 Fig ). Similarly, among cells that retained proliferative capacities in the adult brain (e.g., OPCs, microglia and endothelial cells) ( S2A–S2C Fig ), CNV-related events were not detected ( Fig 2D–2G ), as any duplicated genomic materials in these mitotic cells is believed to be distributed evenly to their daughter cells by completing successful rounds of cell division. Together, these negative data further validated that cell cycle re-engagement events are unique to a certain subpopulation of excitatory neurons in the adult brain.
(A) t-SNE plots of all nuclei from a total of 4 datasets containing cohorts of ND and AD brain samples. The coloring code denotes different cell types. (B) t-SNE plot of 123,212 excitatory neuronal nuclei extracted from both ND and AD brain samples from the 4 independent datasets. (C) A proportional stacked bar graph illustrates a random distribution of cells from the 4 datasets in all the subclusters defined. (D) Violin plot indicating the total number of expressed genes detected among all 15 subclusters of excitatory neuronal nuclei. (E) Estimation of copy number variants among all excitatory neuronal nuclei extracted from the 4 datasets by the InferCNV algorithm. The heatmap on the top is an outcome of the Bayesian latent mixture model implemented to identify the posterior probabilities of alteration status in each cell and whole CNA region. True positives of CNV events were identified in subcluster 5 and, to a much lesser extent, in subcluster 3 (red: gain of copy number; blue: loss of copy number). At the bottom are the line plots that illustrate in detail how gene expression levels at different regions of each chromosome are altered in subclusters 5 and 3 in comparison to a negative control (subcluster 0). (F) The absolute CNV scores within each cytogenetic band were aggregated and are presented. A CNV state value of 1.00 was regarded as zero gain or loss. A change in value of 0.5 indicates a significant gain (e.g., 1.50) or loss (e.g., 0.50) of copy number in each chromosome arm. Plots belonging to subclusters 5, 3, and 0 are shown. (G–H) The genes located at the predicted CNV gain or loss regions of subcluster 5 were extracted ( S3 Table ) and were functionally clustered. The top scatter plot (G) illustrates pathways enriched from genes located in the predicted CNV gain regions, whereas the plot at the bottom (H) illustrates those enriched from the predicted CNV loss regions. The metadata underlying this figure can be found at https://zenodo.org/doi/10.5281/zenodo.10604562 . AD, Alzheimer’s disease; CNA, copy number alteration; CNV, copy number variation; ND, nondemented; t-SNE, t-distributed stochastic neighbor embedding.
https://doi.org/10.1371/journal.pbio.3002559.g002
To validate the robustness of these observations, we integrated 3 validation cohorts of cerebral cortex samples (Brodmann areas 8, 9–12) from both individuals without dementia and those diagnosed with AD [ 28 , 33 – 35 ] into our existing analyses ( Fig 2A and S1 Table ). After correction for all batch effects, a total of 123,212 excitatory neuronal nuclei were extracted from 94 samples and were subsequently assigned to 15 subclusters (Figs 2B , S3A and S3B ). The integrity of each subcluster was subsequently confirmed by the clustree function ( S3A and S3B Fig ), which confirmed that each subcluster was affected by neither dimension reduction ( S3C–S3E Fig ) nor integration methods ( S3F–S3H Fig ). In all subclusters, the relative number of nuclei in each individual dataset was calculated to confirm the unbiasedness of the batch distributions (Figs 2C , S4A and S4B ), as well as the sex distribution of the samples ( S4C Fig ). In the subsequent analyses, subclusters 3 and 5 were characterized by a general loss in gene transcription activity, both in terms of the total number of genes expressed ( Fig 2D ) and the average transcript level abundance of each expressed gene (i.e., gene counts; S4D Fig ). In contrast, these 2 clusters, however, exhibited the most robust expression of cell cycle phase genes, as reflected by their average cell cycle scores ( S4E and S4F Fig ). Among the 2 subclusters, subcluster 5 identified in this multi-dataset analysis was the only excitatory neuronal cluster reexpressing S-phase genes and genes downstream of this cell cycle phase ( S4E and S4F Fig ). Consistent with our earlier predictions from the discovery cohort ( Fig 1G ), InferCNV analysis predicted significant signs of CNV (e.g., CNV status >1.50 or <0.50) in this cluster ( Fig 2E and 2F ) compared to the negative control subcluster 0 located adjacent to subclusters 3 and 5 (which shared the highest similarities) on the tSNE plot ( Fig 2B ). Overall, these observations were alternatively validated when the validation cohorts were separately analyzed ( S5 Fig ). To predict how such changes may manifest and affect the physiological properties of excitatory neurons (e.g., subcluster 5), specific gene loci located within the predicted CNV gain (1,798 genes) and loss (911 genes) regions were identified ( S3 Table ). Pathway enrichment analysis indicated that genes located within the predicted CNV gain regions were primarily related to mitogenic signaling (e.g., PI3K/AKT/mTOR signaling, Wnt/β-catenin signaling, and mTORC1 signaling); cell cycle events (e.g., Myc targets V1 and mitotic spindle); the DNA damage response (e.g., DNA repair, UV response up, and p53 pathway); and metabolic reprogramming (e.g., oxidative phosphorylation, adipogenesis, and cholesterol homeostasis) ( Fig 2G ), while those identified from CNV loss regions were related to the sex hormone response (e.g., androgen response and early estrogen response) and reactive oxygen species signaling ( Fig 2H ). These data suggested that the molecular properties of cell cycle re-engaging excitatory neurons were substantially different from those of other cells.
Extensive loss of neuronal function is associated with a gain of senescence related to erroneous cell cycle gene reexpression
The above observations repeatedly suggested that excitatory neurons, as the only postmitotic brain cell type, are detectable and viable after re-engagement of any erroneous cell cycle-related events. To determine the origins of these cells, we analyzed the expression levels of layer-specific markers corresponding to cerebral cortex cytoarchitecture [ 36 ]. A spatial transcriptomic sequencing matching analysis ( S6 Fig ) confirmed the layer-specific properties of all excitatory neuronal subclusters ( Fig 3A–3C ). Notably, these analyses suggested that subcluster 3, which re-expressed the early G1/S phase marker ( S4E and S4F Fig ), indeed best matched the upper II-III layer and II-IV layer neurons [e.g., II-III — GLRA3 and TLE1; II-IV — CUX1 , CUX2 and KITLG ] ( Fig 3A ). In contrast, the same set of analyses indicated that subcluster 5, which robustly re-expressed late cell cycle phase markers ( S4E and S4F Fig ), was more similar to a distinct group of neurons, as they no longer exhibited any obvious layer-specific identities (Figs 3A–3C and S6 ). Given that cell cycle progression and differentiation are inversely related processes [ 37 ], these observations also suggested that these 2 groups of neurons exhibit a gradual decrease in neuronal differentiation. To validate this hypothesis, we performed single-cell trajectory and branching analyses [ 38 ], and the branching locations of both subclusters of interest were shown to be molecularly distinct from the rest of the excitatory neuronal populations, as also validated separately in all the datasets (Figs 3D and S7 ). Notably, the neighboring localization of 2 subclusters on the same pseudotime scale branch (Figs 3D and S7 ) also suggested that they were evolutionarily related. For further analysis, pathways enriched from the overrepresented cell cycle genes found in these 2 clusters were compared, revealing that pathways involving DNA damage checkpoints [e.g., E2F targets [ 39 ], G2/M checkpoint [ 40 ], mitotic spindle [ 41 ], UV response, and DNA repair] were commonly activated ( Fig 3E and 3F and S4 Table ). Intriguingly, detailed evaluation of the status of the DNA repair response pathway revealed that a substantial number of genes encoding core elements of the mismatch repair, homologous recombination, and Fanconi anemia repair pathways were uniquely down-regulated in subcluster 5 compared with subcluster 3 and the remaining non-cell cycling excitatory neuronal nuclei ( S8A–S8C Fig ). These differences suggested a reduction in the number of pathways associated with DNA repair in these cells. Similarly, bipartite gene expression patterns were also observed among the key elements of other repair pathways, such as nonhomologous end joining, nucleotide excision repair, base excision repair, ubiquitin-related pathways, and poly(ADP-ribose) polymerase (PARP) enzyme regulation ( S8D–S8I Fig ). These observations also suggested that DNA lesions addressed by these partially inhibited pathways are at risk of repair infidelity.
(A ) Dot plot showing the expression levels of cerebral cortex layer-specific markers among all excitatory neuron clusters. (B) Violin plots illustrating the expression levels of selected cerebral cortex layer-specific marker genes (highlighted in red in Fig 3A) in different subclusters. (C) t-SNE plot of excitatory neurons colored according to their cortical layer identity. NS = nonspecific. (D) Single-cell trajectory analysis with the Monocle 2.0 algorithm was used to determine the evolutionary relationship between the 2 subclusters of cell cycle gene-expressing neurons. The locations of subclusters 3 and 5 on this trajectory are labeled, with subcluster 5 located at the terminal. Similar findings were observed when each dataset was separately analyzed, as presented in S7 Fig . (E) Heatmap showing the relative expression levels of 357 cell cycle-related genes among all the excitatory neuron clusters. Hierarchical clustering of these genes based on their expression levels resulted in 5 colored groups. Red- and yellow-colored gene clusters represent up-regulated cell cycle gene groups in clusters 5 and 3, respectively. (F) Functional pathway enrichment analyses of cell cycle gene clusters (red and yellow) with reference to the Molecular Signatures Database (MSigDB) on the Enrich R platform [ 42 , 43 ]. (G) Gene set enrichment analysis of multiple gene sets related to senescence signatures and phenotypes in subcluster 5. (H) Metascape enrichment analysis of all the DEGs identified in subcluster 5 compared to the other excitatory neuronal populations. The functional pathways enriched in the up- and down-regulated DEGs are illustrated in blue and red, respectively. (I) TFs that regulate DEGs that do not match the inferred CNV regions in subcluster 5 were predicted based on the SCENIC analysis, as presented in S11 Fig ( S7 Table ). Manhattan plots illustrating the enriched signaling networks of coinhibited (top panel) or coactivated (bottom panel) TFs. Significantly ( p < 0.05) enriched networks are labeled. The metadata underlying this figure can be found at https://zenodo.org/doi/10.5281/zenodo.10604562 . TF, transcription factor; t-SNE, t-distributed stochastic neighbor embedding.
https://doi.org/10.1371/journal.pbio.3002559.g003
Indeed, DNA repair infidelity, which manifests as persistent activation of the DNA damage response (DDR) due to unresolvable DNA lesions (e.g., DNA repair infidelity), is a strong trigger of cellular senescence—a permanent state of cell cycle arrest [ 20 , 44 ] and possibly the cell fate of subcluster 5. In addition, the expression of 7 independently curated gene sets [ 45 – 49 ] implicated in cellular senescence was found to be significantly enriched in this subcluster (Figs 3G and S9A and S5 Table ), and this phenomenon was also likely associated with the activation of the E2F1-RB axis, which could subsequently induce the reexpression of multiple cyclins and cyclin-dependent kinases (CDKs) (Figs 3F , S9B and S9C ). Other possible senescence response mechanisms, including the compromised ATM/ATR signaling axis that coordinates the fidelity of the downstream DDR ( S9D Fig ) and the induction of senescence mediator CDK inhibitors [e.g., CDKN1A (p21), CDKN1B (p27 Kip1 ), and CDKN2C (p18)], were also observed in these cells ( S9E Fig ). Phenotypically, these cells were also likely to acquire a collagenolytic phenotype [ 50 ] characterized by down-regulated expression of collagen components (e.g., COL19A1 , COL25A1 , COL28A1 , COL11A2 , COL14A1 , COL4A3 , COL6A6 , COL4A6 , COL4A4 , and COL6A5 ), an activated secretory profile of mitogens (growth factors) (e.g., VEGFA , FGF7 ), and regulatory factors (e.g., IGFBP2 , IGFBP6 , and IGFBP3 ) that may promote hypermitogenic arrest [ 51 ] and the acquisition of prosurvival/antiapoptotic phenotypes [ 52 ] (e.g., HSP90AB1 , BCL2L1 , and FOXO4 ) ( S9F Fig ). Taken together, these findings suggested that the excitatory neuronal nuclei in subcluster 5 likely committed to a full senescence response; therefore, we defined these cells as “late senescent (LS)” neurons in the following analyses. Similarly, subcluster 3, located adjacent to these LS neurons on the tSNE plot ( Fig 3C ) and along the same trajectory branch (Figs 3D and S7 ), was considered their precursor and was therefore renamed “early senescent (ES)” neurons.
Phenotypically, in an attempt to further determine the salient and unique features of fully senescent neurons compared to nonsenescent neurons, differential gene expression analysis was performed ( S6 Table ), and post hoc system-level pathway network analysis via the Metascape algorithm [ 53 ] was performed. As predicted, the majority of the down-regulated DEGs in LS neurons were involved in axon development, the regulation of membrane potential and synaptic functions that together participate in cerebral cortex development and function (e.g., learning and memory) ( Fig 3H , red labeling). The up-regulated DEGs were involved in pathways that lead to neurodegeneration (multiple diseases), stress responses (e.g., the bacterial infection pathway, monoatomic ion homeostasis, and transcriptional regulation by TP53), and autophagic events (e.g., autophagy and vesicle organization) ( Fig 3H , blue labeling). Moreover, 33.6% of the up-regulated DEGs were indeed common to the list of genes located within the predicted CNV gain regions, with key functions in cell cycle events, such as mitotic centrosome and tubulin modeling, occurring during the G2/M phase ( S10A and S10B Fig and S6 Table ). Similarly, 28.8% of the down-regulated DEGs detected also matched the list of genes located within the predicted CNV loss regions, with roles in neuronal differentiation and function, axonal guidance and synaptic signaling ( S10C and S10D Fig and S6 Table ). These observations therefore suggested that the phenotypic changes associated with LS neurons were at least in part associated with selective chromatin remodeling and DNA replication events occurring at specific chromosomal regions. For the remaining DEGs that were not located in the predicted CNV gain or loss regions, changes could emerge from altered activities of various transcription factors in these cells. As revealed by the SCENIC algorithm [ 54 ], a total of 162 activated transcription regulons were identified to regulate these genes ( S7 Table ). A total of 115 coactivated and 47 coinhibited transcriptional regulons were found ( S11A Fig and S7 Table ). However, although meaningful functions could not be identified from the coinhibitory set of transcriptional regulons, except for PPARG and NR3C1 , which are related to the “down-regulated response to ultraviolet radiation” ( S7 Table ), transcription factors among the coactivated regulons play important roles in TNF-alpha signaling via NF-ĸB [ 55 ], the hypoxic response [ 56 ], the unfolded protein response [ 57 ], the G2-M checkpoint [ 58 ], Wnt/β-catenin signaling [ 21 ], and the DNA repair network [ 59 ], which has been previously reported to be associated with the cellular senescence response ( Fig 3I ). These findings were faithfully repeated when each individual dataset included was separately analyzed ( S11 Fig ), confirming the robustness of these observations.
Identification of disease-associated signatures among senescent neurons that emerged from AD brains
Aging is considered one of the most prominent risk factors for late-onset AD, suggesting that aged and senescent neurons resulting from aberrant cell cycle-related events may also contribute to disease-related pathogenesis. To dissect how these cells are related to AD risk, we performed mapping analysis of their CNV profiles against an array of known disease risk gene loci [ 60 ] and an investigation of their relative expression levels. Since CNV is linearly associated with gene expression levels for the majority of genes [ 61 ], their gain or loss of change can be indirectly predicted from gene expression analysis with reference to controls. With both the integrated ( Fig 4A ) and separated ( S12 Fig ) analyses, elevated expression levels of multiple AD risk genes were identified within the predicted CNV gain regions in LS neurons. This phenomenon was in stark contrast to what was observed in neurons that did not re-express any cell cycle genes [e.g., subcluster 0 (sharing the highest similarities)] located adjacent to LS and ES neuron on the t-distributed stochastic neighbor embedding (t-SNE) plot ( Fig 2B ). With reference to the matching trend of changes observed in previously reported AD-related CNV loci [ 12 ], including those found to be gained (e.g., SDF4 , BIN1 , ERMP1 , and VDLR ) ( S13A Fig ) and lost in AD (e.g., SLC30A3 , DNAJC5G , TRIM54 , KLK6 , HAS , and FPR1 ) ( S13B and S13C Fig ), which validates our approach, an additional set of differentially expressed AD risk genes was identified. Examples of these genes include multiple major histocompatibility complex molecules, such as HLA-A , HLA-B , HLA-C , HLA-DQB , and HLA-DRB1 (6p21.3), which may introduce selective vulnerability to neurons [ 62 ]; NYAP1 (7q22.1), which modulates tau pathology [ 63 ]; and CLU (8q21.1), which promotes amyloidogenesis [ 64 ] ( Fig 4B ); and those that may directly contribute to the production of amyloid and related peptide fragments [e.g., APP (21q21.3), APLP1 (19q13.12), APLP2 (11q24.3), and BACE1 (11q23.3)], which are all induced among LS neurons ( Fig 4B ). Additionally, the expression of both MAPT (17q21.31) and APOE (19q13.32) was induced ( Fig 4B ), which may lead to mitochondrial dysfunction [ 65 ] and the accumulation of amyloid-β at cortical synapses, respectively [ 66 ]. One exception was PSEN1 (14q24.2), which encodes one of the 4 core proteins of γ-secretase; however, this gene was suppressed ( Fig 4B ). Together, these phenomena suggest that the emergence of cell cycle re-engaging neurons in the brain may contribute to AD-related pathogenesis. Quantitatively, the relative number of these cells in the brain was under better control during the normal aging process; however, in disease-affected subjects with AD, these cells tended to accumulate instead ( Fig 4C ). Given that tissue accumulation in senescent cells is generally prevented by the actions of local immune cells triggered by the detection and interactions of cell surface signals [ 67 , 68 ], further analysis of the expression levels of neuronal cell surface ligands and receptors involved in bidirectional communication with glia revealed that significant reductions in the expression of these transcripts were observed in cell cycle re-engaging neurons (e.g., ES and LS neurons) (Figs 4D, 4E and S14 ). This finding supported the notion of compromised recognition and subsequent elimination of these cells by brain-resident immune cells.
(A) AD risk gene loci mapped to CNV locations identified in subclusters 3 (ES) and 5 (LS) compared to the negative control subcluster 0. The yellow highlights indicate chromosomal locations of the classic AD risk gene loci where significant changes in the absolute CNV scores were observed compared with those of the control cluster. The red and blue labels indicate the up- and down-regulated AD risk genes, respectively, located within the highlighted locations. (B) Normalized expression levels of highlighted AD risk loci indicated in (A) among ES, LS, and the remaining non-cell cycle re-engaging neurons stratified in different cortical layers. (C) Scatter plot illustrating the correlation between the cell cycle re-engaging neuron ratio and the total number of neurons in each sample with age. (D) UMAP plot of excitatory neuronal and glial nuclei extracted from the Mathys and colleagues dataset [ 28 ]. The color code represents different cell types and neurons located at different cortical layers. (E) Heatmaps illustrating the differences in intercell communication strength among different cell types, quantified by corresponding ligand (left) or receptor (right) gene expression levels. The details of this analysis are shown in S1 Data files . (F, G) Dot and box plots illustrating the relationships between the cell number ratios of subclusters of concern and total neuronal nuclei in each sample with (F) a definitive diagnostic status and (G) Cogdx scores. (H) Bar plot representing the numbers of DEGs between groups of nuclei from AD versus ND samples in subclusters ES and LS and those belonging to different cortical layers. (I) Upset plot displaying the intersections among DEGs identified in the LS (AD vs ND) , ES (AD vs ND) , and LS (general versus non-cell cycling neurons) populations. (J) Functional enrichment analysis of up-regulated DEGs in ES (AD vs ND) was performed on the Metascape platform. (K) Bar plot showing the disease enrichment analysis of up-regulated DEGs in ES (AD vs ND) with reference to the DisGenNet database. The metadata underlying this figure can be found at https://zenodo.org/doi/10.5281/zenodo.10604562 . AD, Alzheimer’s disease; CNV, copy number variation; ES, early senescent; LS, late senescence.
https://doi.org/10.1371/journal.pbio.3002559.g004
Our data suggest that cell cycle re-engaging and senescent neurons originating from healthy and affected brains share many commonalities. However, the accumulation of these cells in AD ( Fig 4C ) suggested that certain levels of heterogeneity may exist due to the extracellular influence of different brain microenvironments. However, while no significant associations were found between the overall cell number ratio and patient APOE status ( S15 Fig ) or neuropathological changes (e.g., Braak or CERAD) ( S16 and S17 Figs), the specific cell number ratio of ES neurons was consistently correlated with definitive diagnostic status (e.g., AD, Figs 4F and S18 ) or severity of cognitive decline (e.g., higher Cogdx scores, Figs 4G and S19 ). These observations thus suggested that the phenotypic heterogeneities that could also exist were senescence stage specific. Further comparative analysis of the number of DEGs found in different subgroups of excitatory neuronal nuclei revealed that ES neurons from the AD brains (i.e., ES AD ) exhibited the most dramatic changes compared to those from ND brains (i.e., ES ND ) ( Fig 4H ). In AD samples, ES AD neurons exhibited general features of LS neurons (e.g., significant DEG overlap between ES AD and LS General ; Fig 4I ), while LS AD neurons from the affected brains were also atypical (e.g., no significant overlap between LS AD and LS General ; Fig 4I ). In ES AD cells, although the 75 down-regulated DEGs identified from the comparison with ES ND neurons failed to cluster into any meaningful signaling network, pathways enriched from the 213 up-regulated DEGs suggested that signaling networks related to oncogene-induced senescence (e.g., RAS and MAPK signaling, negative regulation of apoptotic process, and neuroinflammation), anabolic fuel metabolism (e.g., aerobic glycolysis, phospholipid metabolism, and insulin signaling), and hyperexcitability-related pathways (e.g., induction of synaptic transmission, postsynaptic organization, and calcium ion transport) were activated ( Fig 4J ). Further analysis of how this set of up-regulated DEGs may correlate with the entire landscape of human diseases [ 69 ] was conducted with reference to the DisGeNET database covered by the Metascape platform [ 53 ]. The results consistently highlighted the roles of these genes in multiple aspects of AD pathogenesis [e.g., the top 2 pathways: senile plaque formation ( ACTB , ACTG1 , APBB1 , CHGA , CLU , ENO2 , HSPB1 , MT3 , NRGN , PRNP , SORL1 , SYN1 , UBB , RTN3 ) and memory impairment ( ACTB , CLU , IDS , KCNQ2 , SMAD2 , NRGN , PDE2A , PKM , PRKAR1B , PRNP , PSAP , SORL1 , SYN1 , UBB , CDK5R1 , CPLX2 , TPPP , GIT1 , CALY , REEP2 , SLC17A7 , JPH3 , SLC12A5 )] ( Fig 4K and S8 Table ). Together, the activated pathological network in these cells (e.g., ES AD ) in part explained how their increased cell number ratio was correlated with more advanced functional impairment and disease pathogenesis ( Fig 4K ).
Profiling of cell cycle re-engaging neurons in (1) human Parkinson’s disease (PD)-Lewy-body dementia (LBD) and (2) mouse brain aging models
To validate the applicability of this analytical approach in other brain regions or disease settings, we also extended our analysis to the Parkinson’s disease (PD)-Lewy-body dementia (LBD) model, as PD is the second most common age-related neurodegenerative disorder after AD [ 70 ]. In PD-LBD, cell cycle-related events were previously reported in the dopaminergic (DA) neurons of the midbrain and dorsal striatum regions [ 71 ]. Based on this preexisting information, these neurons were first identified and selected [ 72 ] ( S21A and S21B Table ), followed by substratification into 9 subclusters (from 0–8) according to their transcriptomic signatures ( S20A Fig ). Subsequently, cell cycle scoring ( S20B Fig ), cell fate trajectory ( S20C Fig ), InferCNV profiling ( S20D Fig ), and targeted analyses of the expression levels of G2/M phase genes ( S21C Fig ) and evaluation of the expression status of 55 differentially regulated senescence genes defined by Hernandez-Segura and colleagues [ 47 ] ( S20E Fig ) were performed to identify subgroups of early and late senescent (LS) neurons. Consistently, these different methods suggested that subclusters 5 and 2 among all the dopaminergic nuclei exhibited features of ES and LS neurons, as defined earlier in Figs 1 – 4 . To further address whether these ES and LS dopaminergic neurons that emerged from the affected (PD-LBD) mid-brains were molecularly distinct from those that emerged under unaffected conditions, DEG analysis was performed. Like in AD ( Fig 4 ), the number of DEGs identified suggested that while the transcriptomic profiles of LS neurons (subcluster 2) that emerged from the PD-LBD and nonaffected groups were similar, a substantial number of differences indeed existed among the ES neurons (subcluster 5) that emerged from these different brain microenvironments ( S20F Fig ). Functional pathway enrichment analysis of this set of DEGs suggested that ES neurons in the PD-LBD brain microenvironment not only exhibited more extensive deregulation of dopaminergic synaptic functions but also revealed more obvious signs of mitochondrial hyperactivity [ 73 , 74 ] (e.g., oxidative phosphorylation and proton transmembrane transport), stress-induced senescence responses (e.g., oncogene-induced senescence and transcriptional regulation by TP53), and iron-related toxicity (e.g., iron update and transport) ( S20G Fig ). Alternatively, pathway enrichment analysis with reference to the DisGeNET also highlighted how this set of DEGs may contribute to protein aggregation [ 75 ] (e.g., amyloidosis), muscle and motor discoordination (e.g., Creutzfeldt–Jakob disease, sporadic; amyotrophic lateral sclerosis, muscle weakness, myopathy) [ 76 ] and dementia-related symptoms (e.g., familial AD; Parkinsonian disorders) that are commonly reported among PD-LBD patients ( S20H Fig ).
To further explore the application potential of this analytical approach in laboratory models, we performed additional analyses in a mouse model of brain aging [ 77 ] ( S22A Fig ). With a similar set of procedures, excitatory neuronal nuclei were again extracted and divided into smaller subclusters ( S22B and S23A Figs). The cortical layer distributions were identified, except that of subcluster 2, as it barely expressed any layer-specific markers ( S22C and S22D Fig ). Subsequent cell cycle scoring ( S22E Fig ) and InferCNV analyses ( S22F Fig ) concordantly indicated that nuclei in subcluster 2 were cell cycle re-engaging with predicted signs of CNVs; moreover, this subcluster was uniquely characterized by a general loss in global gene transcription activities ( S22G and S22H Fig ). These multiple lines of evidence support that subcluster 2 identified from the aged mouse prefrontal cortex dataset closely resembles LS neurons, as defined previously in human dataset analyses (Figs 1 – 4 ). Subsequently, comparisons of both the up- and down-regulated gene signatures of these cells from mice and humans were conducted. To avoid double counting of any gene paralogs due to possible gene duplication [ 78 ], we first identified human and mouse gene orthologs prior to matching analysis ( S22I Fig ). A total of 372 up-regulated gene orthologs were identified from the original list of 392 human genes ( S23B Fig and S9 Table ), 162 of which (162/392 = 41.3%) were indeed common to the same marker gene list curated from the mouse dataset ( S22I Fig ) and implicated in enhanced mitochondrial oxidative phosphorylation and pyruvate metabolism ( S22J Fig ). These findings suggested that aberrant mitochondrial hyperactivity [ 73 ] and altered fates of pyruvate are conserved metabolic signatures of late senescent neurons. Similarly, of a total of 345 down-regulated gene orthologs identified from the original list of 375 human genes ( S9 Table ), 242 (242/345 = 70.01%) were also common to those identified in mice ( S22I Fig ), with roles in regulating synaptic functions and axonogenesis ( S22K Fig ). The interrelationships between neuronal cell cycle gene reexpression, activation of the cellular senescence response, loss of mature neuronal identity, and an altered fuel metabolic (e.g., pyruvate metabolism) landscape were further validated by histology and immunohistology-based analyses. First, colocalization of the senescent cell marker SA-β-gal and the nuclear signal cyclin B [ 79 ] with the weakened signal of the postmitotic neuronal differentiation marker NeuN (e.g., RBFOX1 ) [ 80 ] first confirmed that the cells of interest were truly neurons ( S22L and S24B Figs). Further evaluation of the key pyruvate metabolic pathway regulator pyruvate kinase (PKM) indicated that SA-β-gal+ cells were deficient in the constitutively active variant PKM1 in the cytosol but revealed positive signals of the PKM2 variant, which becomes metabolically defective when localized inside the nucleus ( S22M , S24A and S24B Figs), suggesting that metabolic reprogramming might have occurred to facilitate the loss of mature neuronal identity among these cells [ 3 , 81 ]. Finally, SA-β-gal+ PKM1- cells were confirmed to be positive for the classic S-phase marker nuclear proliferating cell nuclear antigen (PCNA) ( S22N and S24B Figs). Together with the global accumulation of the G2/M-phase marker Cyclin B [ 7 ] ( S22L and S24B Figs), these findings also confirmed that the cellular senescence response is a concurrent event of cell cycle re-engagement in neurons.
Our study presents a readily applicable approach that allows the utilization of human or mouse brain single-nucleus transcriptomic data to decipher the molecular signature and cellular fate of cell cycle gene reexpressing neurons. The success in obtaining consistent findings in multiple independent datasets suggested that this analytical pipeline is a robust method that is applicable for systematically investigating the “cell cycling status” of all brain cell types by surveying their relative cell cycle gene expression levels and predicting possible CNVs. Our data indicated that the predicted signs of CNVs are consistent with a subset of excitatory neurons reexpressing S phase genes and those beyond this phase. Regardless of the disease status and brain regions of the samples, the predicted chromosomal locations of CNV events were not entirely random but were instead consistently concentrated in certain chromosomal regions ( S5 Fig ). We speculated that this difference is potentially related to the spatial organization of chromosomes inside human nuclei, which determines the ease of accessibility of DNA polymerases. For example, chromosome 19, which is frequently detected at the central position of the nucleus [ 82 , 83 ], was strongly associated with CNV gain events in cell cycle re-engaging neurons. In contrast, chromosome 18, which was detected more frequently at the periphery of the nucleus, was strongly associated with CNV loss events. Nevertheless, the lack of complete genome-wide duplication could at least in part be due to the concurrent initiation of S and G2/M DNA replication checkpoints in these cell cycle re-engaging neurons, which is a surveillance mechanism that prevents unchartered cell cycle activities in untransformed cells [ 84 ].
In addition to normal cell cycle arrest, cellular senescence is likely the terminal cell fate of cells reexpressing genes in the S phase and downstream genes, as this phenomenon was consistently observed in multiple dataset analyses. Regardless of the status of the samples (e.g., either unaffected or diseased-affected brains), these cell cycle re-engaging and senescent neuronal populations were molecularly distinct from their neighbors and characterized by a general loss of mature neuronal signatures and synaptic functions. Moreover, the expression of core genes involved in multiple DNA repair pathways was suppressed in fully senescent cells, which may lead to the repair infidelity that underlies the onset of the senescence response [ 19 , 20 ]. In the cerebral cortex, these cell cycle re-engaging and senescent neurons likely evolved from pyramidal neurons located in layers II-III and III-IV. The latter is an important subtype of excitatory neurons because they interconnect brain association areas to shape numerous cortical functions; however, these cells are also selectively vulnerable to neurofibrillary tangle formation [ 85 ]. In support of these findings, a previous study indeed reported that tau protein aggregation is associated with neuronal senescence [ 86 , 87 ], and whether these events are linked to an unresolved DNA damage response and aberrant cell cycle events in humans warrants future investigation. In the context of late-onset AD, our data suggested that the relative quantities of early senescent neurons (e.g., those reexpressing only early G2/S cell cycle phase genes) were strongly associated with the definitive diagnosis of the disease and the severity of cognitive decline, which was in part due to the more extensive molecular heterogeneities that have existed in these cells that have emerged from the disease-affected brain microenvironment. In contrast to the cells found in nonaffected brains, those identified from diseased brains revealed many activated networks of proinflammatory and antiapoptotic pathways, metabolic reprogramming activities, and excitotoxicity-related responses. In addition, up-regulated genes found in early senescent neurons of affected brains were implicated in the formation of senile plaques, memory impairment, and other pathological features. These differences indeed suggested that the initial cell cycle re-engagement and subsequent deviations in molecular reprogramming may have led to heterogeneities in the properties that contributed to disease onset and progression.
In addition to its application in late-onset AD, our work also assessed whether this analytical pipeline is applicable for analyzing cell cycle re-engaging and senescent neurons in other human diseases (e.g., PD-LBD) and in cross-species settings (e.g., mouse models of brain aging). In the context of PD-LBD, cell cycle re-engaging and senescent dopaminergic neurons were identified in mid-brain tissues [ 88 ]. Likewise, the early but not the late senescent neurons that manifested in the PD-LBD brain microenvironment were substantially heterogeneous. These cells not only exhibited activated cellular senescence pathways but also hyperactivated mitochondrial respiratory activities [ 73 , 89 ], dysregulated iron homeostasis [ 90 ], and compromised dopaminergic synaptic activities that are uniquely implicated in parkinsonism [ 89 ]. Alternative disease pathway enrichment analysis also highlighted the potential involvement of these cells in protein aggregation [ 75 ] (e.g., amyloidosis), muscle and motor discoordination (e.g., Creutzfeldt–Jakob disease, sporadic; amyotrophic lateral sclerosis, muscle weakness, myopathy) [ 76 ], and dementia-related symptoms (e.g., familial AD; Parkinsonian disorders) that are commonly reported in PD-LBD. Similarly, in a mouse model of brain aging, this approach also identified these cells, which indeed exhibited features that were similar to those identified in the human brain, such as aberrant activation of mitochondrial respiratory activities and altered pyruvate metabolism, as well as decreased neuronal properties and synaptic functions. These similarities may be useful in explaining how advanced aging may serve as the greatest risk factor for AD and dementia [ 91 ].
Taken together, the results of this study illustrated a robust bioinformatics strategy that allows thorough investigation of the molecular profiles of cell cycle re-engaging neurons. The findings here demonstrated that cellular senescence is likely the immediate cell fate of these neurons, which also offered valuable insights into the molecular heterogeneities that could exist among these cells manifested in healthy and disease-affected brain microenvironments. While experimental validations of these findings in relevant human samples will be conducted in the future, the applicability of this analytical approach in different diseases and cross-species settings offers new opportunities and insights to supplement mainstay histological-based approaches in studying the roles of these cells in brain aging and disease pathogenesis. The data that illustrate disease-specific molecular signatures and the new marker genes detected in senescent neurons may also lead to new directions for future diagnosis and development of senotherapeutic strategies.
Materials and methods
Data sources and availability of data and codes.
The following publicly available datasets were used: the Mathys and colleagues (syn3157322) [ 28 ] dataset downloaded from Synapse.org . The Nagy and colleagues (GSE144136) [ 34 ], Lau and colleagues (GSE157827) [ 33 ], Yang and colleagues [ 35 ] (GSE159812), Kamath and colleagues (GSE178265) [ 72 ], and Allen and colleagues (GSE207848) [ 77 , 92 ] datasets were downloaded from the GEO Omnibus. All analyses were carried out using freely available software packages. All the original codes for each individual figure are available at https://zenodo.org/doi/10.5281/zenodo.10604562 .
Cohort assembly from multiple single-nucleus transcriptome datasets
Both control (nondemented, ND) and affected (Alzheimer’s disease, AD) samples were extracted from publicly available datasets from 4 independent studies: Mathys and colleagues [ 28 ], Nagy and colleagues [ 34 ], Lau and colleagues [ 33 ], and Yang and colleagues [ 35 ]. Only 2 datasets contained AD-related samples (Mathys and colleagues [ 28 ] and Lau and colleagues [ 33 ]).
According to the sample details extracted from the Mathys and colleagues study [ 28 ], all the samples belonged to participants in the Religious Order Study (ROS) or the Rush Memory and Aging Project (ROSMAP). The parameters they considered for categorizing samples as disease-affected (AD) and ND were primarily based on the degree of classic disease pathologies manifested in the samples. These included the “global AD pathology burden (gpath)” (average score: ND = 0.08 ± 0.08; AD = 1.51 ± 0.74), “overall amyloid level (amyloid)” (average score: ND = 0.03 ± 0.08; AD = 6.46 ± 2.31), and “neuritic plaque burden (plaq_n)” (average score: ND = 0.01 ± 0.04; AD = 1.79 ± 1.35). The definitions of these parameters are listed clearly on the Rush Alzheimer’s Disease Centre (RADC) resource hub. The distinct numerical differences in the mean ± SD values in each of these domains indicated that the pathology-based classification of samples as AD versus ND was clear-cut without ambiguity.
On the other hand, according to the sample details extracted from the Lau and colleagues study [ 33 ], their ND and AD samples were also similarly defined; based on the distinct differences in disease-related pathologies, these included the “Age-related plaque scores” (e.g., ND = 0/A; AD = B/C; plaque load severity Low to High = A ➔ B ➔ C; 0 = nil) and “Braak tangle stage” (e.g., ND ≤ 2; AD ≥ 4).
As suggested by the values in both studies, the control ND patients were defined based on the absence of pathologies, whereas the AD patients were defined based on the obvious manifestations of heavy amyloid load and neuritic plaque burden. Details of the sample identities and demographics are listed in S1 Table . Since batch effects were detected among the datasets, batch effect removal was performed. These studies were also selected because all of them contained high proportions of excitatory neurons among all the nuclei sampled. These selection criteria increase the likelihood of identifying small subpopulations of cell cycle re-engaging neurons and increase the robustness of subsequent analyses. A PD/LBD dataset was later incorporated for cross-brain regional validation of the findings [ 72 ]. A mouse model of aging dataset was also included for cross-species application [ 77 ].
General data processing, target neuron extraction and dataset integration
1. general data processing..
In each of the selected snRNA-seq studies, samples of different cell types were collected for clustering [ 28 , 33 – 35 , 72 ]. For identification of each of these genes, Seurat, an R package designed for the exploration of single-cell RNA-seq data for clustering different cell types, was used [ 93 , 94 ]. The filtered matrix of UMI counts and associated row and column metadata for each dataset were downloaded from the corresponding links in the Synapse and GEO Omnibus databases. In the first step, a Seurat object was created with the count matrix, after which the genes were filtered based on their expression in at least 3 cells, and at least 200 genes had to be expressed in each cell for inclusion. All reads encoded from the mitochondrial genome were eliminated to avoid affecting the cell clustering results. After removing unwanted cells from the dataset, we log-normalized the entire dataset. Highly variable genes were identified, and a principal component analysis of the most variable genes was performed. An elbow plot was generated to select the principal components (PCs) capturing the most variance in the dataset. These PCs were used as edge weights in unsupervised, graph-based clustering to identify the top 30 cell clusters at a resolution of 0.5. t-SNE was used for visualization of all the cell clusters. The expression levels of cell type-specific markers were used to determine the putative identities of each cell cluster.
2. Extraction of excitatory/dopaminergic neurons.
In both the Mathys and colleagues and Nagy and colleagues datasets [ 28 , 34 ], preexisting cell type assignments from their corresponding metadata were used for the extraction of excitatory neuronal nuclei in the samples, which was further confirmed by assessing the relative NGRN expression. For the Lau and colleagues and Yang and colleagues datasets [ 33 , 35 ], since the downloaded data did not include cell type assignments, annotations were assigned to the cells based on the expression levels of cell type-specific markers: neurogranin ( NRGN ) for excitatory neurons, glutamate decarboxylase-1 ( GAD1 ) for GABAergic neurons, myelin basic protein ( MBP ) for myelinating oligodendrocytes, aquaporin 4 ( AQP4 ) for astrocytes, versican ( VCAN ) for OPCs, colony stimulating factor 1 receptor ( CSF1R ) for microglia, and Fms-related receptor tyrosine kinase-1 ( FLT1 ) for endothelial cells. Upon successful cell type labeling, excitatory neurons were extracted from the unaffected and affected samples and are presented as t-SNE plots. For the Kamath and colleagues dataset [ 72 ], dopaminergic neurons were extracted based on the cell type-specific markers vesicular monoamine transporter ( SLC18A2 ) and tyrosine hydroxylase ( TH ).
3. Dataset integration.
With all the excitatory neuron transcriptomic data extracted, these data were integrated into one by the Seurat integration method [ 94 ]. Reciprocal principal component analysis (rPCA) was used to identify anchors between any 2 datasets by using data extracted from the Mathys and colleagues study (with the largest number of samples) as the reference. The top 50 significant PCs derived from the elbow plot were used to locate anchors for integrating the datasets, while the top 30 PCs were selected to identify the neighbors. The resolution was selected for precise recognition of cell cycling clusters; therefore, a wide range of resolution values was tested. Clustering trees obtained by the clustree package [ 95 ] were used to facilitate the validation of cell clusters formed based on a range of resolutions. Starting from the low end, we first used a resolution of 0.5 for clustering potential cell cycle gene reexpressing neurons. As the value increased, further refinement of the clusters was allowed. Eventually, the highest resolution at 1.0 was used to validate the properties in the form of small clusters. t-SNE and UMAP plots used for visualizing all the integrated excitatory neuronal clusters. The dataset-wide distribution of sex, disease status, and cluster cell number distribution are shown.
On the other hand, the Harmony algorithm [ 96 ] was used to examine the influence of the integration method on excitatory neuron subcluster identification. The batch effects between datasets were corrected by the RunHarmony function in the Seurat package, with the dataset used as the group variable. The same parameters used in the rPCA integrated pipeline were adopted, including the top 30 PCs, and a resolution of 0.5 was selected to cluster the excitatory neurons. The consistency of subcluster constitutions between Harmony and the rPCA was evaluated by their shared nuclei and visualized using an alluvium plot.
Cell cycle score analysis
Previously reported cell cycle-associated genes and their corresponding phase information were extracted from both the Whitfield and colleagues [ 29 ] and Tirosh and colleagues [ 30 ] studies. Within this combined list, only genes detected by single-nuclei sequencing were retained, while those annotating hypothetical proteins, ESTs, and gene aliases to official names were screened out. The core scoring function AddModuleScore in CellCycleScoring from the Seurat package was used to calculate a cell cycle phase-specific score for each cell. The permutation value set in the AddModuleScore was 100. The cell cycle phase score of each cell was collated and visualized as violin plots for identifying neuronal subclusters with higher expression of most cell cycle-associated genes, i.e., cell cycle re-engaging neurons. In each cell cycle phase, the cell cycle score of a subcluster was compared to that of the other subclusters to determine the significance of the differences. Cell cycle gene enrichment in different clusters, wherever applicable, was visualized via a heatmap. Clustered genes were then functionally annotated by the EnrichR platform, and the details are provided in the corresponding methods section. A schematic workflow of this analysis is illustrated in Fig 1E .
InferCNV copy number detection and AD loci analysis
The InferCNV algorithm was used to predict copy number alterations (CNAs) from the snRNA-seq data (InferCNV of the Trinity CTAT Project: https://github.com/broadinstitute/InferCNV ) [ 97 ]. To date, InferCNV is the only available method for exploring gene expression intensities across various chromosomal positions in the genome. A comparison of the gene expression intensities between cells of interest and a set of reference “normal” cells was performed to confirm the somatic changes observed. This method is therefore considered an ideal tool for identifying large-scale chromosomal CNAs in cell cycle re-engaging neurons via single-cell transcriptomic datasets. In brief, raw counts of 10,000 excitatory neurons were randomly sampled and selected from the corresponding Seurat object. The InferCNV algorithm was subsequently used to predict CNAs using the following settings: cutoff = 0.1 (this value was found to generally work well with 10X Genomics and 3′-end sequencing and droplet assays), HMM_type = ‘i6′ (hidden Markov model (HMM)-based CNA prediction), and HMM_report_by = c(“cell”) (which instructs InferCNV to report per-cell level CNAs).
Given the CNA regions identified by the HMM, a Bayesian latent mixture model was implemented to identify the posterior probabilities of alteration status in each cell and whole CNA region. This method was used to combat possible missed identification by HHM of CNAs or cells that might not be true CNAs (false-positives). CNV regions identified by the HMM were filtered out if the posterior probability of the CNV region being normal exceeded a specified threshold. This step combines the possibility of misidentified CNVs by removing CNVs that were most likely to be normal and not true CNVs. By default, this threshold was set to 0.5, given that any CNV region that had a posterior probability of being in a normal state greater than 0.5 was relabeled “normal” and was no longer considered an identified CNV region. Changes to various chromosome arms were calculated by concatenating the CNV events detected on each arm according to the InferCNV pipeline and visualized by the point function in the ggplot2 package. The locations of the CNVs detected on the chromosome arms were obtained by using centromere and gene coordinate information from the GENCODE hg38, wherein the “end” was the first and last genes on each arm.
The cytogenetic band for each gene was downloaded from the HGNC database, and cytogenetic band-dependent CNV scores were defined as the sum of the CNV scores of the total genes located in the corresponding cytogenetic band. The results are presented as bar plots generated using the geom_bar function of the ggplot2 package. AD gene loci were manually collected from a recent genome-wide association study [ 60 ], and the corresponding cytogenetic bands are highlighted in yellow.
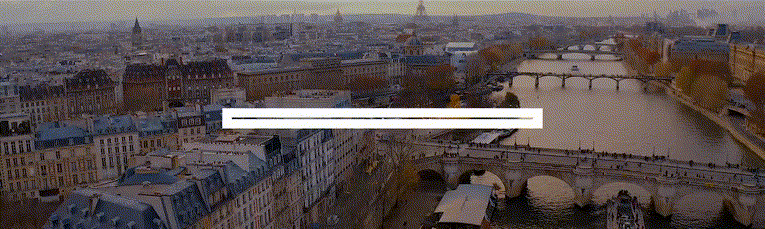
Cortical layer identity evaluation
A list of cortical layer-specific markers was manually collated from the published literature [ 34 , 98 ]. The relationships among excitatory neuronal subclusters were first quantified by unsupervised clustering, followed by integrating the cortical layer markers across the subclusters. The comparative data are presented as layer-specific clusters, with 2 additional clusters indicating early- and late-senescent neurons. For validation of the true layer location of neurons annotated by different layer-specific markers curated from the literature, the expression levels of layer-specific markers across the cerebral cortical layers were quantified and visualized in spatial transcriptomics data [ 98 ] using the spatialLIBD package (v.1.13.4) [ 99 ]. Sample #151507 of the jhpce#HumanPilot10x dataset available at http://research.libd.org/globus [ 98 ] was used as the reference in our analysis. The vis_gene and layer_boxplot functions were used to visualize the layer-specific expression of different marker genes.
Identification of subcluster-specific markers and DEGs between AD patients and ND patients
After the cortical layer identities were revealed, subcluster-specific markers were identified using the FindMarkers function of the Seurat package with the MAST method, which would have considered the dataset source, sex, and age as confounding factors. The markers of fully senescent neurons [i.e., late senescence (LS)] were obtained by comparing this cluster to the remaining excitatory neurons, considered only when the p value <0.05 and the fold change > |2|. For early senescent (ES) neurons, since their layer identity could be distinguished (early layer), marker genes were considered only when the p value was <0.01 via comparison to the excitatory neuronal nuclei that also belong to the upper layers. On the other hand, DEGs between ADs and NDs in neurons in different cortical layers or senescent groups were identified using FindMarkers with the MAST method, which also considered the dataset source, sex, and age as confounding factors. Candidate gene markers with a p value <0.01 were considered.
Comprehensive gene set enrichment analysis with the EnrichR and Metascape platforms
EnrichR is an open-source intuitive enrichment analysis web-based tool that provides various types of visualization summaries of collective functions of genes [ 100 ] ( http://amp.pharm.mssm.edu/Enrichr ). This tool was used to identify gene-related biological processes, and signaling pathways with a threshold value of p < 0.05 and top combined scores were visualized and displayed.
Metascape is another comprehensive, biologist-oriented, publicly available resource ( https://metascape.org/gp/index.html#/main/step1 ) for systems-level dataset analysis [ 53 ]. This tool was used to detect network-level changes and relationships among various significantly ( p < 0.05) enriched pathways from more than 40 independent knowledge bases within the Metascape portal. Related analyses were visualized and displayed.
Cell fate trajectory analysis with the Monocle 2.0 algorithm
Among the cerebral cortex samples, transcriptome profiles of excitatory neuronal nuclei extracted from all datasets were selected for analysis. Among the mid-brain samples, transcriptome profiles of dopaminergic neuronal nuclei were extracted for analysis instead. For determination of the cell evolutionary trajectory among cell cycle gene reexpressing neurons, Monocle 2.0 [ 38 ] was used to order cells in a pseudotime manner based on the differentially expressed gene profiles. In brief, the genes differentially expressed between subclusters of excitatory neurons in each study were identified by the “differentialGeneTest,” followed by DDRTree deconvolution, which returned a principal tree of centroids of cell clusters with reduced complexity in low dimensions.
Cell–cell communication
CellChat, a tool that enables quantitative inference and analysis of intercellular communication networks from single-cell RNA-seq data, was applied to predict the major signaling inputs and outputs for cells based on the curated list of protein interactions, such as paracrine/autocrine ligands/receptors, extracellular matrix receptor interactions, and cell–cell contact interactions [ 101 ]. Relevant ligand (L) and receptor (R) genes were extracted from excitatory neurons, astrocytes, oligodendrocytes, and microglia. For every pair of cell types, the L-R interactions were identified and measured. The degree of intercellular communication was then predicted based on the projected L and R profiles, where the expression levels were approximated by their geometrical mean across individual cells of a type. These interactions represented the interaction strengths (also referred to as “probability” in CellChat) between all the ligands and their receptors expressed in 2 given cell types. Note that CellChat considers important signaling factors such as heteromeric complexes involved in each interaction in addition to an L-R pair; therefore, the absence of any of those components leads to a null interaction. Genes expressed in less than 20% of the cells in one cell type were excluded, and only statistically significant ( p < 0.05, permutation test) communications were considered in our analysis. From individual L-R interactions to signaling, the interaction score of a signaling pathway was calculated by summing the interaction strengths for all the L-R interactions in the pathway. Dysregulated pathways among different neuronal layers were identified (Wilcoxon test, p < 0.05) and visualized in chord diagrams and dot plots.
The regulon activity of transcription factors determined with the SCENIC algorithm
The single-cell regulatory network inference and clustering (SCENIC) algorithm was developed to conduct regulatory network analysis of transcription factors and discovery regulons (that is, transcription factors and their target genes) in individual cells [ 54 ]. In brief, a gene expression matrix was created with the gene names in rows and cells in columns as input for the python implementation of the SCENIC algorithm (pyscenic) [ 54 ]. A single pool of excitatory neurons merged from the 4 datasets included a total of 30,000 cells from random sampling of all the subclusters. The coregulation of transcription factors and their potential target genes was inferred using the grn function of the grnboost2 method. The ctx function was used to define the transcription factor-dependent regulons and to identify high-confidence target genes based on their cis -regulatory cues with the mask_dropouts parameter turned on. The AUCell command was subsequently used to calculate the regulon activity for each cell. Any transcription factors with AUC scores smaller than the 0.01 threshold among any cortical layers were omitted. The final AUC matrix was scaled across the cortical layers and was clustered using the ward. D2 method. The cisTarget database used for this analysis included the “hg38__refseq-r80__10kb_up_and_down_tss.feather” and “hg38__refseq-r80__500bp_up_and_100bp_down_tss.mc9nr.feather.”
Selected gene list analyses
Multiple lists of senescent genes were obtained from the Reactome database (R-HSA-2559583), KEGG database (HSA-04218), Xu and colleagues [ 49 ], Hernandez-Segura and colleagues [ 47 ], and Coppe and colleagues [ 102 ]. The human DNA damage response gene set was extracted from https://www.mdanderson.org/documents/Labs/Wood-Laboratory/human-dna-repair-genes.html [ 103 ] for DNA repair fidelity assessment. Layer-specific markers of the prefrontal cortex layers, as indicated by a list of cell surface markers implicated in immune surveillance [ 104 ], were ordered by a combination of their relative expression levels and percentage of gene-expressing cells in each subcluster. Dot plots were generated by the DotPlot function in the Seurat package, with normalization by the ggplot R package. A heatmap was generated with the pheatmap R package.
Clinical trait association analyses
Clinical traits and disease status of the samples, whenever available, were extracted from the details provided along with the transcriptome datasets. The associations with the total cell number in different neuronal clusters were visualized as boxplots generated by the ggplot R package. Associations between age and the respective number of senescent neurons in the same sample were displayed by the point function in ggplot. Correlation coefficients were estimated by the Pearson method.
Animal maintenance and brain tissue harvesting
C57BL/6J mice of both sexes were obtained from the Jackson Laboratory. Mouse colonies were maintained and bred at the Laboratory Animal Services Centre of CUHK. All animal experimental protocols were approved by the Animal Ethics Committees at CUHK (Approval number: 19/243/GRF), and their care was in accordance with both the institutional and Hong Kong guidelines. Brain tissue harvesting was performed by first anesthetizing the mice via the intraperitoneal administration of 1.25% (vol/vol) Avertin at 30 ml/kg body weight. The heart of each mouse was then surgically exposed, the left chamber was catheterized, and the right atrium was opened. Chilled physiological saline was perfused transcardially for 3 min to remove blood from the body. After perfusion, the cranial bones were opened; cortex and cerebellum tissues were harvested, snap-frozen in liquid nitrogen, and stored at −80°C until use.
Reagents for immunostaining
Unless otherwise specified, all chemicals and reagents were purchased from Sigma-Aldrich. All primary antibodies used were purchased from Thermo Fisher, and the sequences of the antibodies used were as follows: anti-PKM1 antibody (15821-1-AP), anti-PKM2 antibody (PA5-28700), anti-NeuN antibody (Cat #702022), anti-PCNA (13–3900), and anti-cyclin B1 antibody (MA5-13128). Alexa Fluor secondary antibodies were also purchased from Thermo Fisher.
Senescence-associated β-galactosidase assay with immunostaining
Senescence β-galactosidase staining was performed as we previously reported (Chow and colleagues) with a colorimetric kit according to the manufacturer’s protocol. In brief, frozen specimens 10 μm thick were briefly fixed in 1% formaldehyde for 1 min, and staining was subsequently performed using the same procedures as those used for the cultured cells. Caution was taken in determining the incubation time in brain sections, as subpopulations of neurons in the brain will sometimes develop false-positive signals if the incubation time extends beyond 12 h or overnight. Immunostaining was performed after the SA-β-gal staining procedures. In brief, sections were washed twice in PBS after the blue color developed and then permeabilized with 0.5% Triton X-100 in PBS for 5 min at room temperature. After that, the samples were blocked with 0.5% BSA in PBS for 1 h and incubated with the primary antibody in 0.5% BSA for another 3 h at room temperature. Once this incubation step was completed, the samples were washed 3 times with PBS (10 min each) and incubated with secondary antibodies in 0.5% BSA for 1 h at room temperature. The washing step was then repeated, followed by nuclear staining with 1 μg ml–1 4,6-diamidino-2-phenylindole (DAPI) solution for 5 min. Samples were washed twice in PBS, mounted, and observed under a microscope.
Histology-based data quantification procedures and statistical analysis
For each experiment, no statistical methods were used to predetermine sample sizes, but our sample sizes were similar to those reported in our laboratory’s recent publications [ 20 , 21 ]. The data were tested and confirmed to be normally distributed. All samples were analyzed, and the data collected were blinded to the experimental conditions. All the experiments were performed on at least 3 independent occasions. Quantification of cellular morphology parameters was performed in a blinded manner. Analyses of the immunohistochemistry data were performed on Prism 8.0. Differences between groups were analyzed using two-tailed unpaired Student’s t test (for 2 groups) for all normally distributed data.
Supporting information
S1 fig. postmitotic cells other than excitatory neurons revealed limited signs of predicted cnv events..
Estimation of copy number variants by the InferCNV algorithm in (A) inhibitory neurons, (B) oligodendrocytes, and (C) astrocytes. The heatmap located at the top of each panel indicates the copy number alteration regions identified by the hidden Markov model, i.e., regions of gain (red) and loss (blue) in expression along each chromosome at various regions from the p-arm (left side of each box) to the q-arm (right side of each box), in all subclusters. The heatmap located at the bottom of each panel is an outcome of the Bayesian latent mixture model implemented to identify the posterior probabilities of alteration status in each cell and whole CNA region. Red: gain of copy number. Blue: loss of copy number.
https://doi.org/10.1371/journal.pbio.3002559.s001
S2 Fig. Brain cells with reserved mitotic capacities revealed limited signs of predicted CNV events.
( A, B ) t-SNE plot of 1,455 mitotic cell nuclei. (A) Cell types are colored differently: microglia (Mic), endothelial cells (En), oligodendrocyte progenitor cells (Opc), pericytes (Per), and T cells (T). (B) Cells are colored based on subclustering numbers. (C) Violin plots of the cell cycle phase scores of all subclusters of mitotic nuclei. Bolded violins in different phases indicate the subcluster with the most significant above-average gene expression levels among all the clusters. Corresponding significance values against other clusters are shown below. (D–G) Estimation of copy number variants by the InferCNV algorithm in (D) Opc, (E) En/Per, (F) Mic, and (G) T cells. The heatmap located at the top of each panel indicates the copy number alteration regions identified by the hidden Markov model, e.g., regions of gain (red) and loss (blue) in expression along each chromosome at various regions from the p-arm (left side of each box) to the q-arm (right side of each box), in all subclusters. The heatmap located at the bottom of each panel is an outcome of the Bayesian latent mixture model implemented to identify the posterior probabilities of alteration status in each cell and whole CNA region. Red: gain of copy number. Blue: loss of copy number. The metadata underlying this figure can be found at https://zenodo.org/doi/10.5281/zenodo.10604562 .
https://doi.org/10.1371/journal.pbio.3002559.s002
S3 Fig. The definition of excitatory neuronal subclusters was not affected by resolutions, reduction dimensions, or dataset integration methods.
(A) Clustering tree diagram illustrating how excitatory neuronal subclusters were defined at different resolution settings. Each row represents the number of subclusters identified at one particular resolution, and each column represents the number of subclusters and consistencies across different resolutions. As expected, higher numbers of subclusters were defined under higher resolution settings. The target subclusters (e.g., #3 and #5) had already emerged since the resolution was set at 0.4, and their distinct identities held when the settings were increased to 0.7. As the resolution increased to greater than 0.7, these 2 major subclusters started to split into smaller subclusters; nevertheless, they never cross-mixed with others derived from non-cell cycle re-engaging neurons and vice versa. (B) t-SNE plots of excitatory neuronal nuclei subclustering in the 4-dataset integrated analysis settings at different resolutions corresponding to those illustrated in (A). (C) t-SNE and (D) UMAP plots of excitatory neuronal subclusters generated by the rPCA integration method at a resolution of 0.5. (E) UMAP plot of the excitatory neuronal subclusters generated by the t-SNE dimension reduction method. (F) t-SNE plot of the excitatory neuronal subclustering generated by the Harmony integration method at a resolution of 0.5. (G) Excitatory neuronal nuclei were colored and labeled according to the findings from the rPCA integration method, as illustrated in (C) . (H) Alluvial diagram showing the intersecting subclusters defined by the Harmony (left) versus the rPCA (right) integration methods. The resulting high consistency in subcluster identity indicated that excitatory neuronal cluster identification was not affected by the integration methods or other analytical settings. The metadata underlying this figure can be found at https://zenodo.org/doi/10.5281/zenodo.10604562 .
https://doi.org/10.1371/journal.pbio.3002559.s003
S4 Fig. Distribution of neuronal cells based on dataset source, sex, and disease status, as determined by cell cycle analysis in the integrated analysis setting.
(A) t-SNE plots of excitatory neuronal nuclei extracted from nondemented (ND) samples from different studies. (B) t-SNE plots of excitatory neuronal nuclei extracted from disease-affected (AD) samples from Mathys and colleagues and Lau and colleagues. (C) t-SNE plots of the excitatory neuronal nuclei distribution based on sex and disease status. (D) Violin plot illustrating the average feature counts of global transcriptomic profiles among excitatory neurons in different subclusters. (E) Violin plots presenting the cell cycle phase scores of all subclusters of excitatory neurons. Bolded violins highlighted in different phases indicate the subclusters that exhibit the most significant above-average cell cycle gene reexpression among all the subclusters. The corresponding significance values obtained for each subcluster compared to the rest of the others are shown in (F) . The metadata underlying this figure can be found at https://zenodo.org/doi/10.5281/zenodo.10604562 .
https://doi.org/10.1371/journal.pbio.3002559.s004
S5 Fig. Prediction and profiling of copy number variation separately in multiple datasets.
(A, B) Estimation of copy number variants in excitatory neuronal clusters extracted from either (A) nondemented (ND) or (B) disease-affected (AD) brain samples by the InferCNV algorithm. Red: gain of copy number. Blue: loss of copy number. (C, D) Line plots illustrating in detail how gene expression levels at different chromosome locations were altered in subclusters 3 and 5 compared to those in the negative control subcluster 0 extracted from either (C) nondemented (ND) or (D) disease-affected (AD) brain samples. The metadata underlying this figure can be found at https://zenodo.org/doi/10.5281/zenodo.10604562 .
https://doi.org/10.1371/journal.pbio.3002559.s005
S6 Fig. Validation of cortical layer markers by spatial transcriptomic analysis.
(A) Visualization of cortical layers in sample #151707 from the jhpce#HumanPilot10x dataset using spatialLIBD. (B) Visualization of the distribution and counts of cortical layer-specific markers per spot. (C) Boxplots showing the expression levels of various layer-specific markers across different spatial locations defined in (A) to validate their layer specificities. (D) t-SNE and (E) UMAP plots illustrating the differential enrichment of cortical layer-specific markers in different neuronal subclusters in the integrated cohort analyses.
https://doi.org/10.1371/journal.pbio.3002559.s006
S7 Fig. Single-cell trajectory analysis was performed using Monocle 2.0.
Cells on the trees are colored based on cell states, subcluster identities, cortical layer distributions, senescent neuronal cluster assignments, and pseudotime scales. Subclusters 3 and 5 were found along the same branch and were deemed to be the most similar to one another based on their pseudotime values. Subcluster 3, located at the terminal location of a branch, indicated terminal cell fate. The data represent findings from (A) nondemented (ND) or (B) disease-affected (AD) samples.
https://doi.org/10.1371/journal.pbio.3002559.s007
S8 Fig. Compromised DNA damage response network in late senescent neurons.
(A–I) Normalized expression levels of core DNA damage response genes implicated in (A) mismatch repair, (B) homologous recombination, (C) Fanconi anemia, (D) nonhomologous end joining, (E) nucleotide excision repair, (F) base excision repair, (G) ubiquitination and modification, (H) poly(ADP-ribose) polymerase (PARP) enzymes that bind to DNA, and (I) other conserved DNA damage response genes. The metadata underlying this figure can be found at https://zenodo.org/doi/10.5281/zenodo.10604562 .
https://doi.org/10.1371/journal.pbio.3002559.s008
S9 Fig. Additional marker signatures of late senescent neurons.
(A) Expression levels of 55 differentially regulated senescence genes defined by the Hernandez-Segura and colleagues study [ 47 ]. (B–F) Expression levels of selected and classic genes involved in the (B) E2F-RB axis, (C) Cyclin-CDK axis, (D) ATM-Chk2/ATM-Chk1 axis, (E) CDK inhibitor, and (F) senescence-associated secretory phenotype profile obtained from the study by Coppe and colleagues [ 102 ]. The metadata underlying this figure can be found at https://zenodo.org/doi/10.5281/zenodo.10604562 .
https://doi.org/10.1371/journal.pbio.3002559.s009
S10 Fig. Impact of predicted CNVs on the transcriptomic signature in subcluster 5 (late senescent neurons).
(A, C) Venn diagram illustrating the degree of similarity of the DEGs identified in subcluster 5 compared to the remaining non-cell cycle gene reexpressing excitatory neurons in the list of genes located in the predicted (A) CNV gain and (C) loss regions. (B, D) Functional overrepresentation analysis of common genes identified in (A) and (C), respectively, with reference to pathways in the Reactome database. The metadata underlying this figure can be found at https://zenodo.org/doi/10.5281/zenodo.10604562 .
https://doi.org/10.1371/journal.pbio.3002559.s010
S11 Fig. Characterization of upstream events underlying the transcriptome profile changes in terminally senescent neurons.
(A) In an integrated cohort setting, an SCENIC binary regulon activity matrix showing that all 162 corrected regulons were activated in more than one subcluster. Each column represents neurons in a single cortical layer (or ES or LS neuronal clusters), and each row represents one regulon. The term “regulon” refers to the regulatory network of transcription factors and their target genes. Key regulons (rows) are magnified and colored according to their activities: active (orange) or inactive (blue) in the ES and LS neuronal clusters. (B) The same set of analyses illustrated in (A) was conducted separately for each individual dataset. Manhattan plots illustrating the enriched signaling networks of coinhibited (top panel) or coactivated (bottom panel) TFs. Significantly ( p < 0.05) enriched networks are labeled. The metadata underlying this figure can be found at https://zenodo.org/doi/10.5281/zenodo.10604562 .
https://doi.org/10.1371/journal.pbio.3002559.s011
S12 Fig. AD risk gene loci mapping to predicted CNV locations in subclusters 5, 3, and 0.
The yellow highlights indicate chromosomal locations of classic AD risk gene loci. The metadata underlying this figure can be found at https://zenodo.org/doi/10.5281/zenodo.10604562 .
https://doi.org/10.1371/journal.pbio.3002559.s012
S13 Fig. Normalized expression levels of canonical AD-related CNV loci [ 12 ].
Comparisons were made among ES, LS, and the remaining non-cell cycle re-engaging neurons stratified based on their cortical layer identity. Reference list of CNV genes that were found to be (A) lost or (B) gained in AD. (C) Dot plot presenting the scale average expression levels of these canonical AD-related CNV genes among all the clusters compared. The metadata underlying this figure can be found at https://zenodo.org/doi/10.5281/zenodo.10604562 .
https://doi.org/10.1371/journal.pbio.3002559.s013
S14 Fig. Cell cycle gene reexpressing and senescent neurons exhibit compromised two-way communication with glia.
Dot plots illustrating the detailed results of the connectome analysis, which indicate the probabilities of communication between the indicated pairs of ligands and receptors according to the color label. The strength of the interaction, as indicated by the presence of a dot and the color between ligands expressed on glia and cell surface receptors expressed on neurons in various clusters, are shown on the left. Similarly, cell surface receptors expressed on glia and ligands expressed on neurons in various clusters are shown on the right. The metadata underlying this figure can be found at https://zenodo.org/doi/10.5281/zenodo.10604562 .
https://doi.org/10.1371/journal.pbio.3002559.s014
S15 Fig. Correlation plots between the cell number ratios of different neuronal subclusters and the sample APOE status.
The metadata underlying this figure can be found at https://zenodo.org/doi/10.5281/zenodo.10604562 .
https://doi.org/10.1371/journal.pbio.3002559.s015
S16 Fig. Correlation plots between the cell number ratios of different neuronal clusters and the sample Braak stage.
https://doi.org/10.1371/journal.pbio.3002559.s016
S17 Fig. Correlation plots between the cell number ratios of different neuronal clusters and the sample CERAD scores.
https://doi.org/10.1371/journal.pbio.3002559.s017
S18 Fig. Correlation plots between the cell number ratios of different neuronal clusters and the sample definitive disease diagnosis status.
https://doi.org/10.1371/journal.pbio.3002559.s018
S19 Fig. Correlation plots between the cell number ratios of different neuronal clusters and the sample Cogdx scores.
https://doi.org/10.1371/journal.pbio.3002559.s019
S20 Fig. Application of the bioinformatics analytical pipeline in the Parkinson’s disease (PD)/Lewy-body dementia (LBD) model.
(A) t-SNE plot of dopaminergic neurons extracted from healthy and diseased mid-brain samples; these nuclei were divided into 9 subclusters (0–8). (B) Violin plots showing the distribution of cell cycle phase scores in all dopaminergic neuronal nuclei subclusters. The bold highlights indicate the subcluster with the most significant above-average gene expression levels in any particular cell cycle phase; p values against other subclusters are shown. (C) Single-cell trajectory analysis with the Monocle 2.0 algorithm revealing the evolutionary relationship between 2 subclusters of cell cycle gene-expressing neurons. Locations of subclusters 2 and 5 on this trajectory are labeled, indicating that they are on the same trajectory of fate, with subcluster 2 located at the terminal. (D) Estimation of copy number variants among all the dopaminergic neuronal nuclei extracted via the InferCNV algorithm. True positives of copy number variation events were identified in subcluster 2 (red: gain of copy number. Blue: loss of copy number). (E) Dot plot showing the expression levels of 55 differentially expressed senescence genes defined by Hernandez-Segura and colleagues [ 47 ] among all dopaminergic neuronal subclusters. (F) Bar plot representing the numbers of DEGs between groups of nuclei from PD-LBD patients and nondemented samples in all subclusters of dopaminergic neuronal nuclei. (G) Functional enrichment analysis of both up- and down-regulated DEGs in subcluster 5/ES (PD-LBD vs ND) was performed on the Metascape platform. (H) Bar plot showing the disease enrichment analysis of both up- and down-regulated DEGs in the ES cohort (PD-LBD vs ND) with the DisGenNet database. The metadata underlying this figure can be found at https://zenodo.org/doi/10.5281/zenodo.10604562 .
https://doi.org/10.1371/journal.pbio.3002559.s020
S21 Fig. Supporting data for the analysis performed with the PD/LBD dataset ( S20 Fig ).
(A) t-SNE plots of all nuclei derived from both the unaffected and affected samples in the Kamath dataset [ 105 ]. Clusters are colored according to cell type identity. (B) Dopaminergic neurons were identified and selected based on 2 classic markers: SLC18A2 and TH . (C) Dot plot showing the scaled average expression levels of the classic G2/M genes among all the neuronal clusters. Elevated expression of most of these genes was identified in subclusters 5 (ES) and 2 (LS). The metadata underlying this figure can be found at https://zenodo.org/doi/10.5281/zenodo.10604562 .
https://doi.org/10.1371/journal.pbio.3002559.s021
S22 Fig. Application of the bioinformatics analytical pipeline in a mouse model of brain aging.
(A) UMAP plot of a total of 48,052 nuclei derived from frontal cortex samples harvested from four 21-month-old female C57BL/6J mice from the Allen and colleagues (GSE207848) dataset [ 77 , 92 ]. The different cell types used are abbreviated as follows: excitatory neurons (Ex), oligodendrocytes (Oli), inhibitory neurons (In), astrocytes (Ast), microglia (Mic), vascular cells (Vas), oligodendrocyte progenitor cells (Opc), microglia (Mic), and macrophages (Mac). (B) t-SNE plot of extracted excitatory neuronal nuclei divided into 16 subclusters (0–15). (C) Dot plot showing the expression levels of cerebral cortex layer-specific markers among all excitatory neuron clusters. (D) t-SNE plot of excitatory neurons colored according to their cortical layer identity. NS = nonspecific. (E) Violin plots presenting the cell cycle phase scores of all subclusters of excitatory neurons. Bolded violins highlighted in different phases indicate the subclusters that exhibit the most significant above-average cell cycle gene reexpression among all the subclusters. * p < 0.05. (F) Estimation of copy number variants among all excitatory neurons via the InferCNV algorithm. The heatmap illustrates the copy number alteration regions identified by the hidden Markov model, e.g., regions of gain (red) and loss (blue) in expression along each chromosome, at various regions from the p-arm (left side of each box) to the q-arm (right side of each box) in all subclusters. (G, H) t-SNE plots indicating the (G) total number of expressed genes and (H) expressed gene counts detected among all 16 subclusters of excitatory neuronal nuclei. (I) UpSet plot displaying the intersections among marker genes identified in human and mouse LS neurons. (J, K) Pathway enrichment analyses of the (J) 242 common up- and (K) 162 down-regulated LS neuronal marker genes were conducted on the EnrichR platform. (L) Representative SA-β-gal and immunofluorescence staining images validating the interrelationship between cellular senescence, neuronal cell cycle reentry, and altered expression of PKM isozymes in the prefrontal cortex regions ( n = 10; quantification is shown in S24B Fig ; scale bar: 200 μm). The metadata underlying this figure can be found at https://zenodo.org/doi/10.5281/zenodo.10604562 .
https://doi.org/10.1371/journal.pbio.3002559.s022
S23 Fig. Supporting data for the analysis were generated with the mouse brain aging dataset ( S22 Fig ).
(A) Normalized expression levels of cell type-specific markers in all cell types mentioned in S22A Fig . (B) Dot plot showing the scaled average expression levels of common markers identified between human and mouse LS neurons. The metadata underlying this figure can be found at https://zenodo.org/doi/10.5281/zenodo.10604562 .
https://doi.org/10.1371/journal.pbio.3002559.s023
S24 Fig. Supporting data for the mouse brain histology analysis ( S22L Fig ).
(A) Representative SA-β-gal and immunofluorescence staining images validating the inverse relationship between PKM1 and PKM2 expression in neurons [ n = 10; quantification is shown in (B) ; scale bar = 200 μm]. (B) Quantification analyses of the brain histology data presented in S22L and S24A Figs ( n = 10, *** p < 0.0001, unpaired t test). The metadata underlying this figure can be found at https://zenodo.org/doi/10.5281/zenodo.10604562 .
https://doi.org/10.1371/journal.pbio.3002559.s024
S1 Table. Patient demographic details and related clinical information.
https://doi.org/10.1371/journal.pbio.3002559.s025
S2 Table. Original cell cycle gene lists extracted from Whitfield and colleagues and Tirosh and colleagues studies and the final refined gene list.
https://doi.org/10.1371/journal.pbio.3002559.s026
S3 Table. Lists of genes located at the predicted copy number alteration regions in subcluster 5 and pathway enrichment analysis.
https://doi.org/10.1371/journal.pbio.3002559.s027
S4 Table. Overrepresented cell cycle genes in subclusters 3 and 5 and corresponding pathway enrichment analyses.
https://doi.org/10.1371/journal.pbio.3002559.s028
S5 Table. Cellular senescence-related gene lists extracted from multiple databases and independent studies.
https://doi.org/10.1371/journal.pbio.3002559.s029
S6 Table. Differentially expressed genes identified in subcluster 5 (late senescence) compared with the remaining excitatory neurons.
https://doi.org/10.1371/journal.pbio.3002559.s030
S7 Table. Details of the transcription factor lists identified from the SCENIC algorithm via pathway enrichment analysis.
https://doi.org/10.1371/journal.pbio.3002559.s031
S8 Table. Pathway enrichment analysis of up-regulated DEGs in ESAD with reference to DisGeNET.
https://doi.org/10.1371/journal.pbio.3002559.s032
S9 Table. Common marker genes shared between human and mouse late senescent (LS) neurons and pathway enrichment analysis.
https://doi.org/10.1371/journal.pbio.3002559.s033
S1 Data files. Supporting data for Figs 1F, 1G ; 2C–2H ; 3A, 3E–3I ; 4A–4C, 4F–4J ; S2C ; S3H ; S4D and S4F ; S5 ; S8 ; S9A–S9F ; S10A–S10D ; S11A, S11B ; S12 ; S13 ; S14 ; S15 ; S16 ; S17 ; S18 ; S19 ; S20B, S20E–S20G ; S21C ; S22C and S22I ; S23B ; S24B .
https://doi.org/10.1371/journal.pbio.3002559.s034
Acknowledgments
The authors gratefully acknowledge the following parties. The results published here are in whole or in part based on data obtained from the AD Knowledge Portal related to the ROSMAP metadata accessed at https://www.synapse.org/#!Synapse:syn3157322 and deposited by Mathys and colleagues. We would also like to acknowledge the GEO Omnibus for the datasets deposited by Nagy and colleagues (GSE144136), Lau and colleagues (GSE157827), Yang and colleagues (GSE159812), Karmath and colleagues (GSE178265), and Allen and colleagues (GSE207848). We would also like to thank Karl Herrup of the University of Pittsburgh and Ronald P. Hart of Rutgers University for helpful advice, support, and comments.
Consent statement
The data available in the AD Knowledge Portal would not be possible without the participation of research volunteers and the contribution of the data by collaborating researchers. Informed consent was obtained from all individual subjects by the depositors of the data. An agreement on the data and sharing policies was signed to ensure proper protection of the privacy, application and publication of the deidentified data. No further consent or approval from the human data was needed.
- View Article
- PubMed/NCBI
- Google Scholar
Covering a story? Visit our page for journalists or call (773) 702-8360.
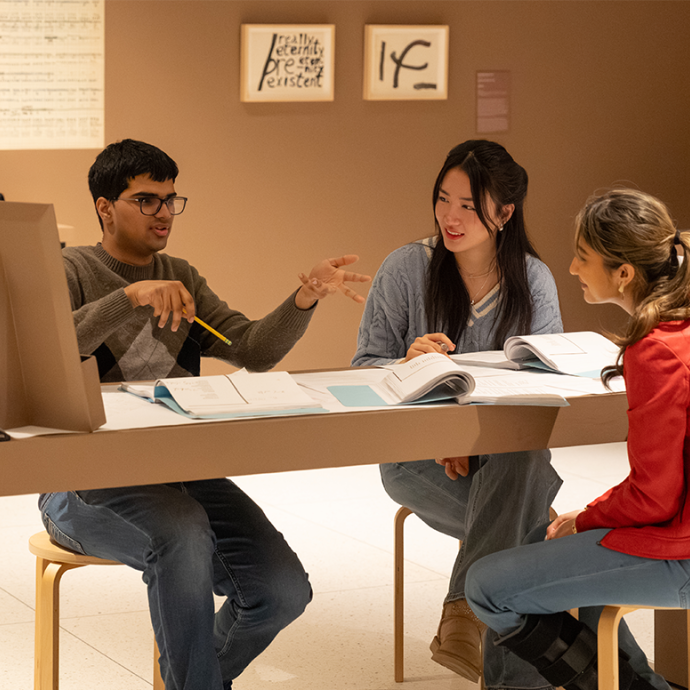
UChicago Class Visits
Top stories.
- ‘Are we doomed?’ Class debates end of the world—and finds reason for hope
- Museum talks give UChicago graduate students’ research a new audience
- UChicago students help support young migrants at South Side schools
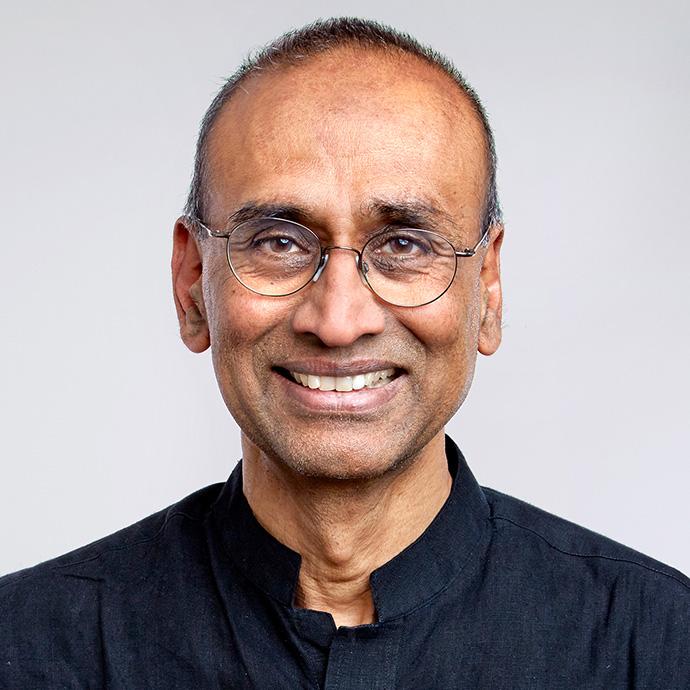
- Big Brains: All Episodes
Why we die—and how we can live longer, with Nobel laureate Venki Ramakrishnan (Ep. 134)
Nobel Prize-winning scientist explains how our quest to slow aging is becoming a reality
They’re perhaps the oldest questions in the science: Why do we die? And could we find a way to live forever? But for decades, anti-aging research was a “backwater” of the scientific community, consider too fanciful and unrealistic. That is until the last few years. Modern advances in biology have taught us a lot about how we age and why we die—could that knowledge help us turn back the clock?
In his new book, “ Why We Die: The New Science of Ageing and the Quest for Immortality ”, Nobel Prize-winning scientist Venki Ramakrishnan delves into the latest science of aging and investigates the nearly $30 billion dollar longevity industry to separate fact from fiction in our modern quest for immortality.
Subscribe to Big Brains on Apple Podcasts and Spotify .
(Episode published April 18, 2024)
Subscribe to the Big Brains newsletter.
Please rate and review the Big Brains podcast.
- Award-winning molecular biologist on the consequences of aging — ABC News
- We are driven by the fear of death: A Wknd interview with Nobel laureate Venki Ramakrishnan — Hindustan Times
- Why do we die? The latest on aging and immortality from a Nobel Prize-winning scientist — Yahoo News Canada
- Why Do We Age? Scientists Are Figuring It Out. — The New York Times
- Why we die: Lessons on genes from a lowly worm — Popular Science
- Why We Die by Venki Ramakrishnan review — a cure for death? — The Times
Transcript:
Venki Ramakrishnan: It’s a question that has bothered humanity ever since we were aware of mortality.
Paul Rand: That’s Venki Ramakrishnan, a Nobel Prize-winning structural biologist.
Venki Ramakrishnan: We are the only people who know that we have a certain lifespan. Ever since we discovered that we have worried about it.
Paul Rand: He’s the head of the MRC Laboratory of Molecular biology in Cambridge, England, and the author of a fascinating new book, Why We Die.
Venki Ramakrishnan: Most of our history, there was nothing we could do about it, death and taxes that were just taken for granted. It’s only in the last 40 or 50 years that because of all the advances in biology, we’re understanding really what is the basis for why we age and die. And that’s a foundation for being able to do something about it.
Tape: If you would like to live longer, maybe to 100 and in good health, there is real science now that is claiming this might be possible. A breakthrough in reversing the signs of aging. Researchers say that they found a way to make mice look and even feel younger.
Tape: We want to talk to these mice.
Venki Ramakrishnan: Aging used to be a kind of backwater field. It was not considered serious biology, but now because of modern tools, we’re actually able to make it into harder science. And so more and more mainstream biologists are going into aging research. All of those things have come together to create this, what you might call a moment.
Paul Rand: Today, there are more than 700 biotech companies focused on aging and longevity with the combined market cap of at least $30 billion.
Venki Ramakrishnan: I think these billionaires are used to success, especially these tech billionaires, achieve success very early in life. They think that life is just some sort of software to be hacked, but biology is complicated. And there is this old saying that when they were young, they wanted to be rich, but now they’re rich, they want to be young. And of course, you can’t buy youth, but you can buy aging research. And so they’re investing heavily in aging research.
Paul Rand: With all this investment in hype in the anti-aging industry, it can be difficult to figure out what’s really going on. Many of the top distinguished scientists in the field now have financial stakes in these companies, but Ramakrishnan does not. And with his expertise in structural biology, he may be one of the best experts to tell us what’s real and what the future of anti-aging may look like.
Venki Ramakrishnan: There’s so much interest in aging and there’s also so much hype. So I thought, well, let me look at all the molecular biology because I’m so close to it that I can read the papers and understand what’s absolutely solid and what’s iffy and what’s really unlikely. And I think what my book tries to do really is give people an understanding of the fundamental principles so that when they come across the next big discovery or read about something in some magazine or newspaper or listen to it on a podcast, they can connect it with what they know about what’s going on in ourselves. So it’s really giving them the tools almost for how to live and what to do and how to understand aging.
Paul Rand: Welcome to Big Brains, where we translate the biggest ideas and complex discoveries into digestible brain food. Big Brains, Little Bites from the University of Chicago Podcast Network. I’m your host, Paul Rand. On today’s episode, Why We Die, How We Age, and What We Can Do About It. Big Brains is supported by UChicago’s Online Master of Liberal Arts program, which empowers working professionals to think deeply, communicate clearly, and act purposely to advance their careers, choose from optional concentrations and ethics and leadership, literary studies and tech and society. More at Mla.uchicago.edu. Everything alive ages, everything alive dies. At first glance, this seems to be an immutable fact of nature, but it turns out things aren’t that straightforward.
Venki Ramakrishnan: We’ve wondered about, for example, what makes life spans so different? Sharks and whales, some of them can live several hundred years. A giant tortoise lives 170 plus years, and then at the other end, you have a mayfly that lives for a day and then you have a mouse that lives for two years, but a bat the same size can live for 40 years. So I think the idea that we’re all made of the same material but we age differently, suggests that we could do something about it, and that requires understanding the basis of aging.
Paul Rand: The first step in figuring out what we can do about aging is understanding what it really is. The problem of aging isn’t just one cause and it’s not just a single negative process in our bodies. In fact, many of the causes of aging are the very same things that keep us alive when we’re young.
Venki Ramakrishnan: Biology has a bias. Its main selection is for making sure that the genes we have are passed on. So it’s about the survival of our genes. It doesn’t much care what happens later on in life. So you can see throughout biology, throughout all the causes of aging, there’s always this delicate balance between what’s good for you early in life and what causes aging later. And if you start tinkering with one, you might affect the other.
Paul Rand: Untangling this complicated web of multiple causes starts by figuring out how each strand works, and one of the most fundamental strands is DNA damage.
Venki Ramakrishnan: Absolutely. So if you look at the program of life and of all our cells, it’s encoded in our genes, and that program is there to make all of the proteins that are required for life. We require proteins for almost every function we carry out, including thinking and remembering and fighting infections, not just our muscles. So think of DNA as a long string of letters. They’re chemical letters. DNA uses four kinds of units. And so you can think of it as a long code written in a four-letter alphabet. If you have environmental attack on the DNA, it doesn’t even have to be from carcinogens, it could be just in the natural process of living. It’ll corrupt some of those letters in the DNA.
Paul Rand: Over the course of one single day, 100,000 changes happen to the DNA in each of our cells. In fact, just exposure to water can cause 10,000 transformations.
Venki Ramakrishnan: In other words, some of those elements of DNA will be changed, others will be removed, and sometimes the DNA will be broken, so it’ll be like you have a long sentence and then the sentence is suddenly broken and you don’t know how it ends and you don’t know where the rest of the sentence is. And as we age, those errors build up and this leads to proteins that are the wrong protein. The proteins have mistakes or they’re made at the wrong time, or they’re made in the wrong amounts, or they’re not degraded when they should be. So the program, which is this orchestra of proteins that all have to work together is slowly breaking down. So you get a discordant dysfunctional orchestra if you like.
Paul Rand: But if our DNA is being constantly damaged like this, how do we even make it through a single day, let alone years? The answer is that our body has developed ways to repair DNA. The problem is, this repair response is itself a cause of aging.
Venki Ramakrishnan: One of the big risks if you have DNA damage is cancer. You have over a trillion cells in your body. If a few million of them die, you’re not even going to notice it. In fact, they die all the time. But if one of those cells becomes cancerous, it could kill you. It could kill the whole organism, right? So preventing cancer early on is quite important. So when the cell senses DNA damage, the first thing it does is try to repair the DNA. But if it senses that the damage is too extensive, then it triggers a series of responses which have two possibilities. One is, it just sends a cell into death. It’s called apoptosis. It’s a kind of cellular suicide. So the cell basically dies. The other is that it sends a cell into a state called senescence. These cells are not dead, they’re not even dormant.
They just can’t divide and they can’t carry out their normal function. But one thing they do is they secrete inflammatory compounds because what they’re trying to do is attract the immune system to the site of it. And so it’s a signal to the body to say, “Hey, there’s a problem here. This cell is sending an alarm signal. Go there, deal with it and then you can clear it away.” Now, as we get older, that mechanism itself deteriorates. And so we start accumulating these senescent cells and so we have more and more inflammation as we get older, and that’s one of the major problems with aging.
Paul Rand: Studies have shown that transplanting even small numbers of senescent cells into young mice cause persistent dysfunction.
Venki Ramakrishnan: So the DNA damage response is a curious thing.
Paul Rand: This balance between aging and cancer is one that keeps coming up again and again for researchers. Many of the possible ways to turn back the clock lead to an increased risk of cancer, and many of the ways our body fights cancer lead to aging. In fact, some cancer therapies actually work by inhibiting DNA repair mechanisms.
Venki Ramakrishnan: If you inhibit DNA repair pathways, that’s detrimental to the cancer cell and preferentially it kills off cancer cells. So it’s a paradox. But of course, you can’t inhibit DNA repair for the sake of aging because you really want DNA repair to work well.
Paul Rand: But there are other mechanisms around DNA that may be more promising. One has to do with something called DNA methylation.
Venki Ramakrishnan: With time, our DNA gets additional chemical marks. Some of them are called methyl groups, so they’re called methylation. These additional marks are signals to either inhibit the expression of some genes or sometimes to enhance it. And it turns out, DNA methylation seems to be a better indication of your age. It correlates better, for example, with mortality with late life diseases, than just chronological age. Because we all know there are 70-year-olds who are very spry climb mountains and stuff, and others who are really not in good shape at all. So the places where their DNA is methylated on their genome, that pattern will be different for the two people. There’ll be probably more methylation in the person who has aged more compared to the person who’s aged less. They are inhibiting more of their genes by methylation. And of course, you need many of these genes in order to function.
And people are asking, if we were to reverse the pattern of methylation, could we in fact reverse aging? That’s the theory anyway. But in order to test it, what you would have to do is be able to reverse that methylation and see if somehow the symptoms of aging improved. Okay, that hasn’t quite been done, but people are working on that. And if that works, it would be a way to turn the clock back a little bit. Now, one reason to think that there’s something there, is one place where all our methylation gets erased completely, is when we give birth to a new generation. So when you have a fertilized egg, it goes through a program where all the methylation marks on the original donor cells are erased, and it starts all over again. And that’s why a child born to a forty-year-old woman is not 20 years older than a child born to a twenty-year-old woman. They’re both starting at zero, and it’s because the aging clock has been reset in both of them.
Paul Rand: There’s another piece of our DNA that scientists think play a crucial role in the process of aging, and it may teach us more about how to reverse it. And that piece is the very, very ends of our DNA strands, also known as telomeres.
Venki Ramakrishnan: So our DNA are like a long linear molecule, so they have two ends and in the cell, they’re wrapped up with proteins and form these structures called chromosomes. Now, the thing is that when cells divide, the chromosomes have to be replicated. So you need two where they used to be one, because you’re making two cells out of one. The mechanism for replicating the DNA or copying the DNA has a peculiarity. It can’t copy the very ends of the DNA.
Paul Rand: That’s the telomeres.
Venki Ramakrishnan: So our ends keep getting shorter and shorter. As they get shorter, that structure unravels, and then the cells starts saying, “Oh, there’s a problem here.” And then it sends that cell into senescence, just like as if it were DNA damage.
Paul Rand: And just like with DNA repair, our body has a system to try and fix the shortening telomere problem.
Venki Ramakrishnan: There’s some cells which have to keep dividing like our stem cells. So some cells can’t let the telomeres get shorter, and that special telomere sequence is added by this enzyme called telomerase. It turns out that in most of our end point cells, by end point, I mean let’s say we start with a fertilized egg. The end point would be all the specialized cells that we have, like skin and blood cells and hair, our eye cells and so on, and neurons. Those end point cells, the telomerase is turned off, so they can’t keep on dividing because keeping on dividing is a cancer risk.
Paul Rand: Again, a connection between a possible anti-aging remedy and increased cancer risk.
Venki Ramakrishnan: So we’ve evolved to turn off telomerase, unless we absolutely need them. But cancer cells have figured out how to turn it back on again, and that’s why cancer cells can keep on dividing.
Paul Rand: Now, many researchers are excited about turning telomerase back on as anti-aging mechanisms try to stop our telomeres from shortening. In fact, people with less than normal amount of telomerase have been shown to prematurely develop diseases associated with old age. But of course, if we were just to turn on telomerase, it would increase our risk of cancer.
Venki Ramakrishnan: Other people are trying to tackle this by turning on telomerase just a little bit, so that it can extend your telomeres, so that then as they shorten, you delay senescence. So that’s the idea behind some of these anti-aging companies.
Paul Rand: Now, stress also can affect the telomeres too. Is that right?
Venki Ramakrishnan: Yes. I thought that was a very bizarre connection, but apparently the stress hormone, cortisol, has multiple effects. A lot of times chronic stress is very bad for you, and this stress, oddly enough, actually shortens your telomeres. So it probably is accelerating telomere loss in some way.
Paul Rand: So when you hear people talking about the negative life span effects of stress, there’s a real biological reason for it.
Venki Ramakrishnan: Oh, yeah.
Paul Rand: In the last few years, tens of billions of dollars have been poured into the anti-aging industry to try to use these advances in our biological understanding, to turn back the clock. We’ll get into the specific anti-aging mechanisms being developed after the break.
If you’re getting a lot out of the important research that’s shared on Big Brains, there’s another University of Chicago Podcast Network show that you should check out. It’s called Capital Isn’t. Capital Isn’t uses the latest economic thinking to zero in on the ways that capitalism is and more often isn’t working today. From the debate over how to distribute a vaccine, to the morality of a wealth tax, Capital Isn’t clearly explains how capitalism can go wrong and what we can do about it. Listen to Capital Isn’t, part of the University of Chicago Podcast Network.
Before the break, we talked about the unique abilities of stem cells as they relate to telomeres, but many researchers and companies think that these cells may just be the ultimate key to slowing or even reversing aging.
Venki Ramakrishnan: Many of the ways to attack aging are what I would call slowing down or delaying aging, but stem cells are slightly different. The idea behind that is, it’s a field called cellular reprogramming. This has to do with the fact that if you take a cell from an early embryo, it can produce any kind of cell. It could produce a skin cell or an eye cell or a blood cell or a neuron, but as they keep developing, they specialize into certain kinds of stem cells. So there are stem cells which can only result in new types of blood cells. Other cells can only generate skin or hair, other cells can only generate neurons. What happens with aging is that we need these stem cells even when we’re grown up, because our tissues are constantly being regenerated.
For example, our skin is being replaced quite frequently, and so is our hair as we know, and our blood. You can donate blood and two weeks later, you’ll have remade the blood cells you donated. Other cells, not so much. So neurons turn over very slowly, only a small fraction. A lot of them, we’re stuck with the neurons we have. Heart muscle does not regenerate very well, and that’s why if you damage heart muscle in a heart attack, it’s a serious problem. You can’t just replace it. But if you were able to generate the stem cells that are responsible for regenerating those tissues, you might be able to regenerate as necessary. Now, one problem as we age is our stem cells also age and they go into senescence and they die off. So as we get older, we’re depleting our stem cells and the stem cells we’re left with are what are call clones. They’re descended from just a few strains so they don’t have the same diversity that we were born with.
Now, what cellular reprogramming guys want to do is they want to use a method for which a guy named Yamanaka won the Nobel Prize, which is by introducing four factors that turn on various genes, they’re able to make cells go backwards in this development process, so they could take a fully adult cell and make it go backwards into a stem cell. So this is actually like reversing the clock, but of course, it’s a very complicated thing. Today, if you use Yamanaka factors and turn cells all the way back to what are called pluripotent cells, that is cells that could make any kind of cell, then you get these growths called teratomas, which are kind of cancer.
So the reason that we’ve shut a lot of this ability is again, to prevent cancer early in life. So it does come with all kinds of risks, and also how much do you turn it back and how would you apply it to the body? Because not all our organs age at the same rate. In the same individual, if you look at various markers, their different organs have aged differently in different people. And so how to apply this in a safe and effective way in humans, it’s a big problem. It’s a challenge that these people have to work out. Yes, there’s a lot of promise, and of course, there’s a lot of hype as well. But on the other hand, there is real biology there, and I think it’s going to be an exciting time in the field.
Paul Rand: Another avenue that has many scientists excited revolves around the concept of caloric restriction and the drugs that may be able to induce this state while still allowing us to eat whatever we want. But what does caloric restriction have to do with aging?
Venki Ramakrishnan: In a wide variety of species, it has been found that reducing caloric intake has extended life, at least as compared to an all you can eat. So then the question is, what does this do to our underlying pathways, the biochemical pathways? What does caloric restriction actually do? How does it work? So people found at least two major pathways that are influenced by caloric restriction. One of them, for example, controls protein production, so we don’t make too much protein. During the normal course of life, we’re making proteins and then getting rid of them when we don’t need them anymore, and having them hang around when they’re not needed is also detrimental. So it’s not just producing proteins at the right time. You also have to be able to destroy them at the right time. If the degrading part doesn’t work, then you get a pileup of stuff that you don’t need. So excess protein production is turned down and recycling of garbage is turned up, and both of those things are beneficial for aging. So that’s a pathway called the TOR Pathway.
Paul Rand: When calories are restricted, there are fewer nutrients for cells to use. And when TOR sees this, it can switch off protein synthesis, growth pathways, and turn out cell’s ability to recycle junk, all of which as we’ve seen, are important for aging.
Venki Ramakrishnan: And then people want to know, can we have a drug that does the same thing as caloric restriction?
Paul Rand: Is that rapamycin?
Venki Ramakrishnan: And that’s rapamycin, is one of the more popular drugs that lots of people are studying, because it directly affects one of the major pathways.
Paul Rand: The inhibition of the TOR Pathway by caloric restriction or the drug rapamycin, has also been shown to increase mitochondria. Another reason why it may be helpful for aging.
Venki Ramakrishnan: Mitochondria are a place where the energy units that the cell needs are made. Okay, so you can think of it as they’re the place where the energy currency of the cells make. They’re also involved in a lot of metabolic processes, controlled with the cell.
Paul Rand: Additionally, exercise turns on the same pathways that stimulate mitochondrial production.
Venki Ramakrishnan: Well, of course, rapamycin, originally it was discovered as an antifungal compound. Then it was shown to have antitumor activity. Then it was shown to be an immune suppressor. I mean, it does a lot of things because it is a major pathway, but it’s an immune suppressor, which means you’re more prone to infection, you will heal wounds less effectively. It has a bunch of other side effects as well.
Paul Rand: Rapamycin has been shown to significantly improve health and lifespan in mice. Excitement around the drug has hit such a fever pitch, that Ramakrishnan says, some scientists are quietly self-medicating with it. But he warns the hype is really going past the data at this point.
Venki Ramakrishnan: If you’re perfectly healthy, the bar is higher for you to take it. You’re saying, “I’m going to take rapamycin for 10 years so that at the end of my life, I’ll live an extra 10 years.” Well, that’s a trickier proposition. And so the safety requirements are different. So people have to demonstrate that. And the hope of the rapamycin community is, that they’ll find some sweet spot where it doesn’t have the negative effects like immune suppression, et cetera, but still has the benefits for aging.
Paul Rand: All of these drugs come with trade offs and are incredibly complicated. Could there be a simpler way? Of course, one of the oldest and most straightforward anti-aging stories in human history, revolves around a famous fictional character, Dracula. But recent research has shown he may have actually been onto something with this whole blood thing. When we look back and we look at the science fiction stories of yore, and there was some wealthy industrialist that brings people in, and he replaces his old blood with new blood as a way of extending his life, that actually is not so far-fetched in people’s minds these days, is it?
Venki Ramakrishnan: Oddly enough, there’s some science behind it. And the science behind it is when they connected two animals like an old rat with a young rat, and this experiment’s called parabiosis. It’s basically, they’re sharing their blood supply. It turns out that the old animal benefited by the young blood that was being pumped into it by the young animal. It turned out the young animal also suffered as a result of old blood. And that finding has been reproduced a number of times. And so it seems perfectly good science. So people are then trying to ask, “Well, what is it about young blood that’s different from old blood?” And blood has lots and lots of protein factors, which can do all kinds of things. So they’re trying to figure out what the differences are, and not all the differences might be relevant for this effect.
So they have to figure out which factors that are different, are actually relevant for this beneficial effect. And then they have to find out how they work. Are they activating stem cells? Are they helping with repair? There could be lots of possibilities. That’s a big ongoing area of research. Lots of scientists working on it. There’s some very distinguished ones as well.
Of course, when people saw this in the news media, young blood prevents aging. Then you had a bunch of companies, at least one infamous company that started offering young plasma from that is plasma from the blood of young donors to rich people. And then the FDA tried to shut it down, and they opened under a different name, and it’s still going, I think. There’s this enormous amount of hype. And what this does is they try to jump the gun because there are a group of people who are so worried about aging. They love their lives, they love their active lives, and they’ll do anything, or they’ll do it just to push the envelope. These people are taking advantage of that. Whereas what really needs to happen is people need to understand how this works and then figure out ways of testing it first in animals safely, and then doing proper trials in humans.
Paul Rand: There’s not a person that’s going to be listening to this saying, “You idiot, ask him what we should do today. Make sure he tells you what you should do to extend your life today.” Which is, I’m sure the question you get asked 100 times a day, give me the magical answer, please.
Venki Ramakrishnan: Yeah. Well, yeah. So none of the drugs so far have gone through clinical trials and shown to be effective. So today, the trio of things that you can do yourself is better than any anti-aging medicine on the market. And that is a moderate and healthy diet, including lots of fruits and vegetables. And now exercise has all kinds of things. It induces reaction that helps us repair damage. It even helps with regenerating our mitochondria. So all those things that we discuss about aging, you later learn that exercise has those benefits. Now, a third leg to this trio is sleep, which is very underappreciated, and especially in many Western countries, and I should say particularly in the US, people skimp on sleep. It turns out that sleep is when a lot of the repair and maintenance of our body goes on. And so that trio is very good.
And then there are social things which affect these. For example, reducing stress, socializing, having friendships, et cetera. They’re all free. They don’t have side effects, and as I say, better than anything on the market now. But I want to say, that those three things are also useful for reducing your cholesterol and your blood pressure. And yet, I’m on statins and I’m on anti-blood pressure medication. Why? Because the things I did on my own weren’t quite enough. Things kept creeping up with age. And so I suppose you could say one of the goals of the aging community is to supplement that, to go beyond what you can do yourself. But of course, what you do yourself is what you should start with. And these things hopefully in the future can help you go beyond that.
Paul Rand: The advancements in anti-aging while in their early stages, do hold a lot of promise, and we’re only going to keep taking more steps toward the future when people may live significantly longer. Ramakrishnan says this raises a whole host of ethical questions that we need to figure out before that day comes.
Venki Ramakrishnan: Just like with AI, this is going to have unforeseen social consequences, which is, what would happen if everybody lived to be 100 or everybody lived beyond 120 if they somehow cracked that barrier. And it would result in a very different sort of society. And we need to think about what kind of society we want and how that would end up. And the other is that we already have a huge social disparity. The richest 10% live over a decade, sometimes two decades longer than the poorest 10%.
The things I talked about, exercise, diet, and sleep, those are all things that poor people find it hardest too, because they can’t find a good diet, they have to eat on the run, whatever they can get. They don’t have time to sleep, they’re working two, three jobs. And they don’t have time for exercise often, so everything’s stacked against them. Now, if we now have very sophisticated life extension measures or anti-aging measures, who will get them? If only rich people get them, you’re going to increase the disparity even more. So I think we need to think about socially, how to make these advances available to everyone.
Matt Hodapp: Big Brains is a production of the University of Chicago Podcast Network. We are sponsored by the Graham School. Are you a lifelong learner with an insatiable curiosity? Access more than 50 open enrollment courses every quarter. Learn more at graham.uchicago.edu/bigbrains. If you like what you heard on our podcast, please leave us a rating and review. The show is hosted by Paul M. Rand, and produced by Lea Ceasrine and me, Matt Hodapp. Thanks for listening.
Episode List
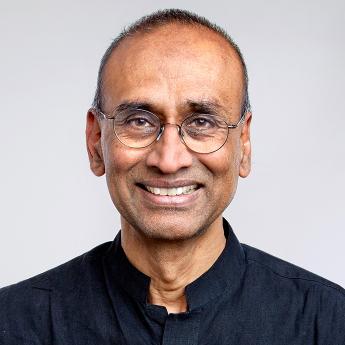
What dogs are teaching us about aging, with Daniel Promislow (Ep. 133)
World’s largest study of dogs finds clues in exercise, diet and loneliness
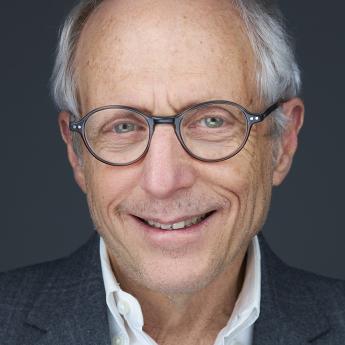
Where has Alzheimer’s research gone wrong? with Karl Herrup (Ep. 132)
Neurobiologist claims the leading definition of the disease may be flawed—and why we need to fix it to find a cure
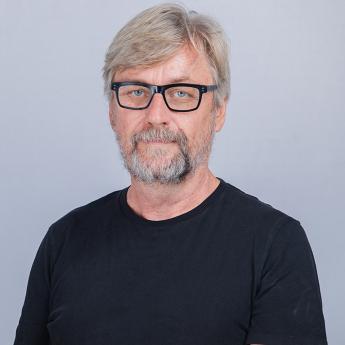
Why breeding millions of mosquitoes could help save lives, with Scott O’Neill (Ep. 131)
Nonprofit's innovative approach uses the bacteria Wolbachia to combat mosquito-borne diseases
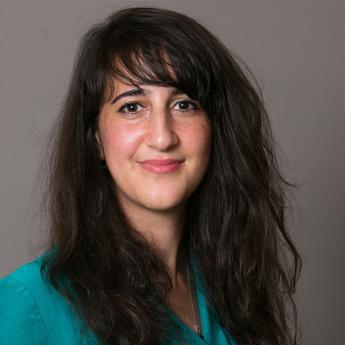
Why shaming other countries often backfires, with Rochelle Terman (Ep. 130)
Scholar examines the geopolitical impacts of confronting human rights violations
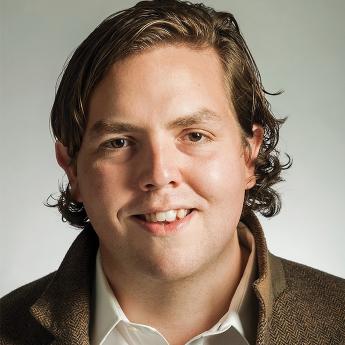
Can Trump legally be president?, with William Baude (Ep. 129)
Scholar who ignited debate over 14th Amendment argument for disqualification examines upcoming Supreme Court case
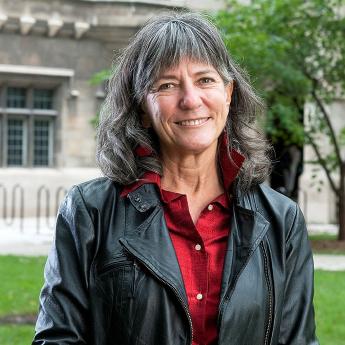
What our hands reveal about our thoughts, with Susan Goldin-Meadow (Ep. 128)
Psychologist examines the secret conversations we have through gestures
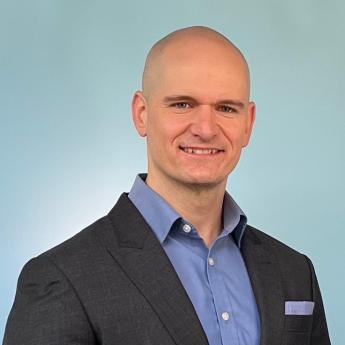
Psychedelics without the hallucinations: A new mental health treatment? with David E. Olson (Ep. 127)
Scientist examines how non-hallucinogenic drugs could be used to treat depression, addiction and anxiety
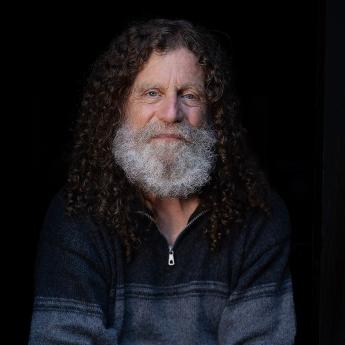
Do we really have free will? with Robert Sapolsky (Ep. 126)
Renowned scholar argues that biology doesn’t shape our actions; it completely controls them
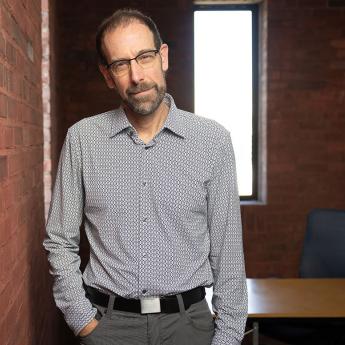
A radical solution to address climate change, with David Keith (Ep. 125)
Solar geoengineering technology holds possibilities and pitfalls, renowned scientist argues
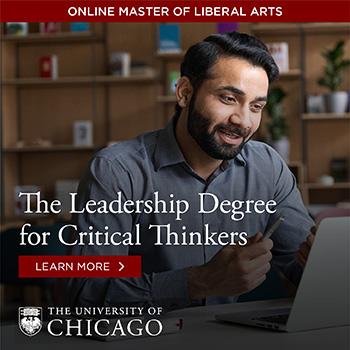
Subscribe to the Big Brains Insider newsletter

- News Releases
Wandering minds associated with aging cells
UCSF study finds association between attentional state and length of telomeres
University of California - San Francisco
image: This is Elissa Epel, Ph.D. view more
Credit: Susan Merrell/UCSF
Scientific studies have suggested that a wandering mind indicates unhappiness, whereas a mind that is present in the moment indicates well-being. Now, a preliminary UCSF study suggests a possible link between mind wandering and aging, by looking at a biological measure of longevity.
In the study, telomere length, an emerging biomarker for cellular and general bodily aging, was assessed in association with the tendency to be present in the moment versus the tendency to mind wander, in research on 239 healthy, midlife women ranging in age from 50 to 65 years.
Being present in the moment was defined as an inclination to be focused on current tasks, while mind wandering was defined as the inclination to have thoughts about things other than the present or being elsewhere.
According to the findings, published online on Nov. 15 in the new Association for Psychological Science journal Clinical Psychological Science , those who reported more mind wandering had shorter telomeres, while those who reported more presence in the moment, or having a greater focus and engagement with their current activities, had longer telomeres, even after adjusting for current stress.
Telomeres are the DNA-caps that protect the ends of chromosomes, preventing them from deteriorating or fusing with neighboring chromosomes. Telomeres typically shorten with age and in response to psychological and physiological stressors. In research pioneered at UCSF, scientists have discovered that telomere shortness predicts early disease and mortality.
As the study assessed mind wandering and telomeres at the same time, the researchers don't yet know whether mind wandering leads to shorter telomeres, whether the reverse occurs, or some common third factor is contributing to both.
Mindful meditation interventions, which promote attention on the present with a compassionate attitude of acceptance, lead to increases in some aspects of health. Previous studies have found that they are associated with increased activity of an enzyme known as telomerase, which is responsible for protecting and in some cases, replenishing telomeres.
Along with the new UCSF study, these findings support the possibility that a focus on the present may be part of what promotes health measurable at the cellular level, the researchers said.
"Our attentional state—where our thoughts rest at any moment – turns out to be a fascinating window into our well-being. It may be affected by our emotional state as well as shape our emotional state," said Elissa Epel, PhD, associate professor of psychiatry and lead author on the study. "In our healthy sample, people who report being more engaged in their current activities tend to have longer telomeres. We don't yet know how generalizable or important this relationship is."
Moving forward, Epel, along with Eli Puterman, PhD, a psychologist in the UCSF Department of Psychiatry, and colleagues are developing a series of classes to promote more mindful presence, to see if this intervention protects telomere maintenance or even lengthens telomeres.
In the current study, participants self-reported a tendency to mind wander, and were measured for aspects of psychological distress and well-being. The sample was highly educated and had a narrow range of both chronological age and psychological stress (most were low stress), all of which might have contributed to the ability to detect this relationship, Epel said.
The study is the first to link attentional state to telomere length and to control for stress and depression, Epel said. Previous studies have shown links between telomere length and particular types of stress and depression. Since this study relied on self-reported attentional state, she said, further studies directly measuring presence and mind wandering will be needed.
"This study was a first step and suggests it's worth delving into understanding the link between mind wandering and cell health to get a better understanding of whether there is causality and reversibility," said Epel. "For example, does reducing mind wandering promote better cell health? Or are these relationships just reflective of some underlying long-standing characteristics of a person?"
"Results suggest the possibility that the attitude of acceptance of negative experiences might be one of the factors that promotes greater ability to be more present - to be okay with one's current experience and not avoid the unpleasant aspects of everyday experiences," she said.
"A number of emotion theories suggest that greater attentional control leads to less suppression of negative emotions, and thus less of the rebound effect of unsuccessful suppression," said Wendy Berry Mendes, PhD, associate professor and Sarlo/Ekman Endowed Chair of Emotion at UCSF and co-author on this study. "Alternatively, attentional control may help us interpret emotions in a more constructive way, what we call 'positive reappraisals.' Such styles of thinking have been associated with healthy physiological states."
In addition to Epel, Mendes and Puterman, co-authors on this study include Jue Lin, UCSF research biochemist in the Department of Biochemistry and Biophysics; Elizabeth Blackburn, PhD, UCSF molecular biologist and Alanie Lazaro, UCSF laboratory manager in the Department of Psychiatry.
Research on telomeres, and the enzyme that makes them, was pioneered by three Americans, including Blackburn, who co-discovered the telomerase enzyme in 1985. The scientists received the Nobel Prize in Physiology or Medicine in 2009 for this work.
Epel, Blackburn and Lin are co-founders of Telome Health Inc, a telomere measurement company.
This study was funded by the Baumann Foundation and the Barney & Barbro Foundation.
UCSF is a leading university dedicated to promoting health worldwide through advanced biomedical research, graduate-level education in the life sciences and health professions, and excellence in patient care.
Follow UCSF UCSF.edu | Facebook.com/ucsf | Twitter.com/ucsf | YouTube.com/ucsf
Clinical Psychological Science
Disclaimer: AAAS and EurekAlert! are not responsible for the accuracy of news releases posted to EurekAlert! by contributing institutions or for the use of any information through the EurekAlert system.
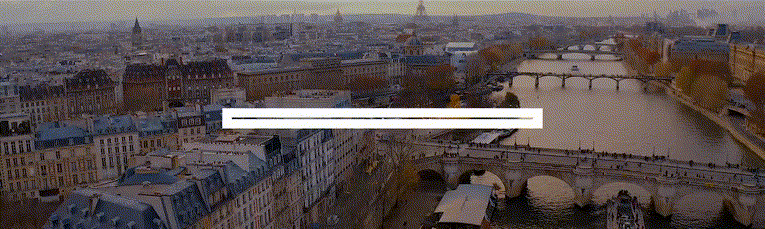
IMAGES
COMMENTS
There is a vast divide between mind wandering and cell aging, and understanding any causal mechanistic links between the two could provide a better understanding of the mind-body relationship. At the least, this finding opens the door to new questions about the experiential aspect of the flow of thought and why this appears to be tied to ...
Wandering Minds and Aging Cells. January 2013. Clinical Psychological Science 1 (1):75-83. DOI: 10.1177/2167702612460234. Authors: Elissa S. Epel. Eli Puterman. University of British Columbia ...
Those who reported high mind wandering had shorter telomeres, consistently across immune cell types (granulocytes, lymphocytes), than did those who reported low mind wandering, even after adjusting for stress. Telomere length varies widely between adults, and these findings suggest that presence of mind may explain some of these differences.
Many ancient contemplative traditions believe presence of mind promotes greater longevity, a belief that is hard to test. Scientific evidence suggests that mind wandering predicts unhappiness, whereas presence in the moment predicts well-being. It is important to test whether a tendency toward mind wandering is associated with biological measures of longevity beyond self-reported measures of ...
Those who reported high mind wandering had shorter telomeres, consistently across immune cell types (granulocytes, lymphocytes), than did those who reported low mind wandering, even after adjusting for stress. Many ancient contemplative traditions believe presence of mind promotes greater longevity, a belief that is hard to test. Scientific evidence suggests that mind wandering predicts ...
Now, a preliminary study suggests a possible connection between mind wandering and aging, by looking at a biological measure of longevity. In the new study, psychological scientist Elissa Epel and colleagues at the University of California, San Francisco examined the relationship between telomere length, an emerging biomarker for cellular and ...
Wandering minds associated with aging cells November 16 2012 Scientific studies have suggested that a wandering mind indicates unhappiness, whereas a mind that is present in the moment indicates well-
Introduction. In recent years, many studies have consistently reported that healthy older adults exhibit a reduction in task-unrelated thoughts (commonly referred to as mind-wandering) compared to young adults [for reviews, see 1, 2].These age-related differences have been found in numerous tasks including those assessing episodic memory encoding [], sustained attention [4, 5], reading ...
Those who reported high mind wandering had shorter telomeres, consistently across immune cell types (granulocytes, lymphocytes), than did those who reported low mind wandering, even after adjusting for stress. Telomere length varies widely between adults, and these findings suggest that presence of mind may explain some of these differences.
Now a preliminary UCSF study suggests a possible link between mind wandering and aging, by looking at a biological measure of longevity. In the study, telomere length, an emerging biomarker for cellular and general bodily aging, was assessed in association with the tendency to be present in the moment versus the tendency to mind wander, in ...
Scientific studies have suggested that a wandering mind indicates unhappiness, whereas a mind that is present in the moment indicates well-being. Now, a preliminary UCSF study suggests a possible ...
Wandering Minds and Aging Cells. Clinical Psychological Science, 2012; DOI: 10.1177/2167702612460234; Cite This Page: MLA; APA; Chicago; University of California, San Francisco (UCSF). "Wandering ...
Questionnaire-based investigations of mind-wandering and aging have similarly found a reduction in off-task thought with age (Singer and McCraven, 1961; ... a SD in parentheses for this and all subsequent cells. b One younger adult did not provide these ratings. c One older adult did not provide these ratings. d NEO = NEO-FFI personality ...
Wandering minds associated with aging cells. by University of California at San Francisco. November 17, 2012. ... Now, a preliminary UCSF study suggests a possible link between mind wandering and aging, by looking at a biological measure of longevity. In the study, telomere length, an emerging biomarker for cellular and general bodily aging ...
With regard to perseverative cognitions, there is evidence that meditation training reduces rumination and mind wandering ... Epel ES, Puterman E, Lin J, Blackburn E, Lazaro A, Mendes WB: Wandering minds and aging cells. Clin Psychol Sci 2013, 1:75-83. [Google Scholar]
This type Wandering Minds and Aging Cells of disposition may lead one away from unwanted thoughts or feelings in the present moment and feed subsequent mind wandering. It could also create fertile ground for more frequent or exaggerated threat appraisals of everyday hassles. Threat appraisals and associated physiological reactivity appear to ...
Wandering Minds Are Associated With Aging Cells November 19, 2012 Scientific studies have suggested that a wandering mind is linked with unhappiness, whereas a mind that ... is present in the moment is linked with well-being. Now, a preliminary study suggests a possible connection between mind wandering and aging, by looking at a biological ...
Wandering Minds and Aging Cells 3 Reliability. One-year follow-up data reveal a significant year-to-year correlation coefficient of .55 (p < .001), suggesting some stability in mind-wandering tendency for this two-item variable that focuses on past-week behavior. These stability results are similar to the test-retest correlation for the 10-item
byAbigail Mortimore for Health Canal: Scientific studies have suggested that a wandering mind indicates unhappiness, whereas a mind that is present in the moment indicates well-being. Now a preliminary UCSF study suggests a possible link between mind wandering and aging, by looking at a biological m
Now, a preliminary UCSF study suggests a possible link between mind wandering and aging, by looking at a biological measure of longevity. In the study, telomere length, an emerging biomarker for cellular and general bodily aging, was assessed in association with the tendency to be present in the moment versus the tendency to mind wander, in ...
The first decade of the twenty-first century was characterized by renewed scientific interest in self-generated mental activity (activity largely generated by the individual, rather than in direct response to experimenters' instructions or specific external sensory inputs). To understand this renewal of interest, we interrogated the peer-reviewed literature from 2003 to 2012 (i) to explore ...
The frequency of mind-wandering decreases as a function of age in healthy individuals. One possible explanation is that mind-wandering is a resource-dependent process, and cognitive resources decline with age. The present study provides the first investigation of mind-wandering in the earliest stages of Alzheimer's disease (AD) to further ...
Recent evidence suggests that cell cycle genes become re-activated in some post-mitotic neurons in the aged brain. In this study, the authors develop a bioinformatic approach to robustly characterize the prevalence, nature, and fate of these cell cycle re-entry neurons, within the healthy aging and diseased human brain.
Venki Ramakrishnan: Many of the ways to attack aging are what I would call slowing down or delaying aging, but stem cells are slightly different. The idea behind that is, it's a field called cellular reprogramming. This has to do with the fact that if you take a cell from an early embryo, it can produce any kind of cell.
In the study, telomere length, an emerging biomarker for cellular and general bodily aging, was assessed in association with the tendency to be present in the moment versus the tendency to mind ...
But starting in the early 2010s, genetic studies revealed that many autism-associated genes actually play a very different role: They encode proteins that reside far from the synapses — in the cell's nucleus, where the DNA is located, and regulate the activity of hundreds of other genes. "This was a huge surprise," Nord said.
In patients with myeloma, cancerous plasma cells grow out of control and crowd out healthy cells, causing fractured bones, bruising, fatigue, and weakness. While myeloma isn't curable, Esteghamat noted that it's highly treatable, with treatment goals including inducing remissions, increasing progression-free intervals, and overall survival ...
The California Office of Statewide Health Planning and Development (OSHPD) has awarded $875,000 to UC Davis Health residency training programs to help ensure a robust primary health care workforce in California. "We are thrilled to have received a grant from the Song-Brown Primary Care Residencies Award fund," said Véronique Taché ...