An international meta-analysis of values of travel time savings
Affiliation.
- 1 Institute for Transport Studies, University of Leeds, Leeds LS2 9JT, UK. [email protected]
- PMID: 19679355
- DOI: 10.1016/j.evalprogplan.2009.06.010
Values of travel time savings are often used in cost-benefit analysis of transport projects and policies, and also to compute generalised travel costs. There has been considerable debate as to whether different research methods (e.g. stated versus revealed preference) will lead to different values of travel time savings, and which segmentations (e.g. by income or mode) are most important to capture the heterogeneity in these values. In addition there are many countries where no specific valuation studies have been done. In this paper new equations are estimated on the outcomes of value of travel time savings studies from various countries. In the data set, several countries appear more than once, which is taken into account by estimating random effects panel models. The meta-analysis sheds some new light on the variation of the value of travel time savings by income, country, travel purpose, mode, distance and by survey method. Furthermore, the resulting meta-models are applied to produce new values of travel time savings for business travel, commuting and for other purposes in passenger transport, for 25 European Union Member states. Similar methods could be used to statistically analyse studies carried out on other non-monetary effects, both for transport and non-transport projects, and for inclusion in cost-benefit analysis.
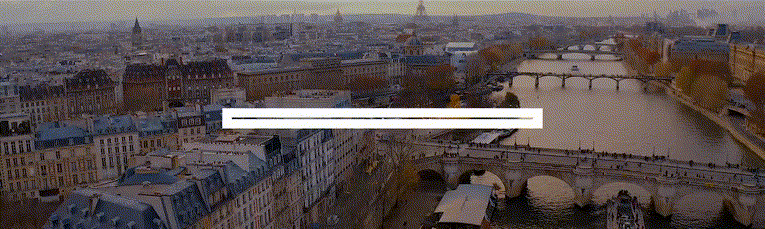
Publication types
- Meta-Analysis
- Cost-Benefit Analysis
- Models, Econometric
- Time Factors
- Transportation / economics*
Is generalised cost justified in travel demand analysis?
- Open access
- Published: 14 February 2018
- Volume 47 , pages 75–108, ( 2020 )
Cite this article
You have full access to this open access article
- Mark Wardman 1 , 2 &
- Jeremy Toner 2
7029 Accesses
8 Citations
1 Altmetric
Explore all metrics
Generalised cost (GC) has long been widely used as a measure of the attractiveness of travel alternatives. We argue that its limitations have been forgotten, overlooked or not appreciated, and it is used because ‘it has always been used’. We explore the relationships between GC and its components (price, time, value of time) and their respective demand elasticities and show that the variation of component elasticities over time and space is not consistent with the variation implied by a GC formulation. We therefore conduct detailed tests of whether the GC approach is justified. The datasets used are recorded ticket sales between pairs of railway stations for a number of years and covering a variety of types of flow. When restricted to a GC formulation, we find that allowing VoT to be estimated as part of the modelling process is greatly superior to using either the UK official VoTs or using VoTs from meta-analyses. We conclude that the importance of using the correct VoT in calculating GC cannot be overstated. Failure to do so will lead to incorrect derivation of component elasticities from the GC elasticity. A more flexible approach estimating elasticities to components of GC is better than even the best GC formulation. We conclude that robust models, with large sample sizes and very precise coefficient estimates in their standard form, are not able to support the variation in GJT and fare elasticities that would be implied by the GC approach. It might be time to stop using GC.
Similar content being viewed by others
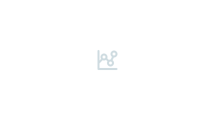
Investigating demand models with more flexible elasticity functions: empirical insights from rail demand analysis
Mark Wardman
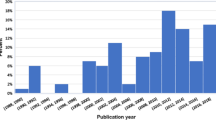
The value of travel time savings in freight transport: a meta-analysis
Jawaher Binsuwadan, Gerard De Jong, … Phill Wheat
Cost and time damping: evidence from aggregate rail direct demand models
Andrew Daly, Nobuhiro Sanko & Mark Wardman
Avoid common mistakes on your manuscript.
Introduction
Transport planners the world over make much use of generalised cost (GC) and/or generalised time (GT) which represent the overall attractiveness of a travel alternative in equivalent units of money or travel time. This is because such composite terms have a number of attractions at both the estimation and application stages. These include overcoming estimation problems due to co-linearity between or lack of variation in the constituent variables, Footnote 1 introducing the potentially desirable feature that the elasticities to the constituent variables depend upon the proportion they form of the composite term, supporting a consistent approach to forecasting and economic appraisal, reducing concerns about the use of constituent elasticities for large proportionate changes, and avoiding the problems in consumer surplus measurement where a constituent variable, such as fare under a concessionary scheme, is zero. Searle ( 1978 ) justified its use on the grounds that “it results in better practice than its more simple alternatives” and that “we [should not] overlook the commonsense virtues of generalised cost”.
Nonetheless, one possible reason why GC is used is because ‘it has always been used’, and we here contend that its potential limitations have been forgotten, overlooked or indeed not appreciated. This paper not only raises the question of whether the use of GC in demand models is justified but provides some empirically based answers. Bruzelius ( 1981 ) contends that the “articles (Grey 1978a , b ; Goodwin 1978 ; Searle 1978 ) demonstrate that there is a considerable lack of understanding in the profession”. We contend that this is still the case with regard to GC.
Although the GC formulation is widely adopted, it imposes properties without any explicit testing of whether they are empirically justified. This has long been recognised (Jones 1977 ; Grey 1978a ). Indeed, Grey ( 1978a ) stated that, “The principal restriction is that the ratio of the rates of change of demand Q with respect to any two independent variables C i , C j is not fixed directly by empirical evidence, but by the predetermined generalised cost specification”. The GC approach forces the implied constituent elasticities to exhibit large cross-sectional variations according to the make-up of GC on different journeys and an increase (reduction) in time (cost) related elasticities, all other things equal, as monetary values increase over time with income growth. Footnote 2 Moreover, it is implicitly assumed that the value of time used in appraisal can be taken to be appropriate for demand forecasting.
A different approach, and one used by the rail industry in Great Britain, is to merge the time related aspects (journey time, frequency and interchange) Footnote 3 into a single variable, termed generalised journey time (GJT), but to maintain fare as a separate term. We term this the GJT-Fare approach. This approach also forces elasticity variation, given that the elasticities of the component variables of GJT will vary with the proportion that they form of GJT. Whilst this might not be justified, and indeed was addressed in Wardman ( 1994 ), the formulation of the GJT function is not the focus of this paper.
Finally, what might be regarded as the conventional economics approach would be to enter into the demand model separately each of the key attributes regarded to influence demand. We term this the separate components (SC) approach. We note that it invariably characterises the very extensive discrete choice modelling literature. The SC approach is here covered to demonstrate the potential for further disaggregation of the components of GJT, and for reference and interest given that we can estimate it, but its most appropriate formulation is not the focus of this paper.
The opportunity and aims
The railway industry in Great Britain has available very large amounts of ticket sales data which provide a reasonably accurate account of rail travel between very many stations over numerous years. Whilst rail fares exhibit variation over time, just as the costs of other modes do, a key attraction is that rail routes can also exhibit variations in the timetable related service quality attributes of journey time, frequency and interchange. This then supports modelling opportunities not otherwise possible which we here exploit to test a number of important hypotheses. As Bruzelius ( 1981 ), summarising Searle ( 1978 ), concluded: “the use of generalised cost has to be based on [the claim that] travel demand functions in terms of generalised costs seem to be working better than other types of travel demand models”. Specifically, the aims of the research reported here are to test:
whether the GC approach of standard transport planning provides a better explanation of rail travel demand than the standard rail industry GJT and fare approach or indeed an approach that specifies separate elasticities to each component of GC;
whether the elasticities to GJT and fare implied by the GC approach can be empirically justified;
whether variations over time and across routes in the GJT and fare elasticities implied by the GC formulation are empirically justified;
the appropriate values of time to adopt conditional upon the use of the GC approach.
In short, is generalised cost justified in travel demand analysis?
Scope and significance
The research findings here discussed are both original and significant because, as far as we aware, there has been no explicit and detailed testing of the ‘hypotheses’ set out in “ The opportunity and aims ” section along with GC being a dominant formulation worldwide where transport planning and appraisal is practised. It is important to know whether GC is empirically valid because of the fact that GC is consistent with conventional microeconomic demand theory if and only if the willingness to pay to save travel time is not a function of real income (Bruzelius 1981 ). In addition to insights of a methodological nature, we also provide a range of results that are of broader interest for the purposes of forecasting, benchmarking and interpretation in the passenger rail market.
In terms of the scope of the research reported here, which is primarily concerned with the validity of the GC approach, we make a number of important points largely stemming from its originality. We aim to understand these fundamentals of GC as currently used in practice but with a view to developing this investigation to address more complex issues.
Firstly, we have not considered ‘flexible’ functional forms where the GC, GJT and SC formulations might through appropriate parameterisation approximate each other. The analysis reported here is couched very much in the tradition of these approaches, that is, characterised by constant elasticities to GC, GJT and Fare, and the SC variables. The analysis of more flexible functions is a separate study in its own right.
Secondly, the analysis could have been extended to the direct estimation of a wider range of weights used in the composite GJT terms. We are though of the view that this would not materially add to or alter the findings presented here. Again, this is a separate study beyond the scope of the significant issues this paper aims to address.
Thirdly, and related to the previous point, our focus here is on comparing the GC and GJT-Fare approaches on the grounds that the latter is dominant in the rail industry in Britain and serves as a sound empirical basis to test the former whereas the robust estimation of each of the SC elements to provide a firm basis of comparison is a much more challenging task.
Finally, we have not addressed dynamic behavioural responses. In part, this is because two of the three data sets available to us cover only a few years. But more importantly, the analysis of dynamic effects would cloud our investigations and add an element of complexity in terms of the relationships explored that would not contribute to answering the important hypotheses set out in “ The opportunity and aims ” section.
The structure of the paper is as follows. “ The candidate model formulations ” section provides background discussion covering the competing demand model formulations. “ What insights on expected elasticity variation are provided by existing evidence? ” section considers the insights that are provided by review study evidence into the elasticity variation here being examined whilst the rail ticket sales data used in our analysis are described in “ Data used in analysis ” section. “ Model specification ” section sets out the candidate models to be evaluated and examines whether fixed or random effects models are more appropriate to our investigations. The main empirical findings and tests of hypotheses are contained in “ Empirical findings ” section and concluding remarks are provided in “ Conclusions ” section.
The candidate model formulations
The gc approach footnote 4.
The concept of GC has been widely used in transport planning since the late 1960s (Goodwin 1978 ). Indeed, Searle ( 1978 ) claimed that, “…… what could be more sensible as a simple working hypothesis than to assume that major determinants of travel behaviour are a linear combination of time and money cost parameters?” McIntosh and Quarmby ( 1970 ) first set out the procedures recommended for use in transport planning studies in Britain but the term seems to originate with Wilson ( 1969 ).
Despite the widespread use of GC, review studies (TRRL 1980 ; Goodwin 1992 ; Balcombe et al. 2004 ; ATOC 2013 ; Wardman 2014 ) all point to there being extensive evidence relating to fare elasticities but surprisingly little for GC elasticities. Footnote 5 Indeed the GC elasticity is sometimes deduced from the much more common evidence on price elasticities.
We can compose GC in this rail context simply as the summation of fare (F) and generalised journey time (GJT):
GJT represents the timetable related service quality in time units, as discussed in “ The GJT and fare approach ” section, and μ is the money value of time that converts GJT into an equivalent monetary amount. The value of μ is invariably pre-specified, but as we shall see it can here be directly estimated.
If we specify the relationship between the volume of rail demand (V) and GC in typical constant elasticity form:
then the implied elasticities (η) to GJT and fare are:
The elasticity to a constituent variable is the product of the proportion that it forms of GC and the GC elasticity (λ). Since GT is simply a change from money to time units, the GT and GC elasticity are the same and the implied GJT and fare elasticities are unaffected. The sum of the constituent elasticities equals the component elasticity. Note also that functional relationships between demand and GC other than Eq. 2 still impose the property that the component elasticities depend upon the proportion that they form of GC.
The implied GJT and fare elasticities will exhibit strong variation across routes according to the proportion that each variable forms of GC and, since μ will increase over time with income, the implied GJT (fare) elasticity will be forced to increase (fall) over time, all other things equal. Whilst these are not a priori unreasonable properties, as far as we are aware they have not been subjected to explicit empirical testing. Footnote 6 In addition, GC is invariably calculated using the best available evidence on the value of time rather than upon a directly estimated value of time that provides the best explanation of demand conditional upon the use of GC.
The GC approach implies that the value of time is:
We subsequently make use of this relationship although, more usefully where there is limited evidence on one of the elasticities, its re-arrangement allows η GJT (η F ) to be deduced if we have evidence on η F (η GJT ) and μ. Footnote 7 So, for example:
The GJT and fare approach
With a pedigree almost as long as the GC approach is the UK rail industry’s practice of using separate fare and GJT terms. This is set out in the Passenger Demand Forecasting Handbook (PDFH) which is unique worldwide amongst railway organisations and indeed transport authorities in general. It has set out a recommended forecasting procedure and associated parameters since 1986 (ATOC 2013 ). Footnote 8 GJT stemmed from the need for an index to represent the timetable related attractiveness of train services which have somewhat different journey times, departure time profiles and interchange requirements across the day (Tyler and Hassard 1973 ). The different British Rail computer systems dealing with timetables and revenue led to a separation of GJT and fare which has persisted to this day without any empirical testing of whether movement to the conventional GC approach or indeed the SC approach would provide a better forecasting approach.
There is a considerable evidence base covering GJT and fare elasticities in the rail industry in Great Britain, as is clear from PDFH and large-scale reviews of the literature (Wardman 2012 , 2014 ).
PDFH specifies the demand function in conventional constant elasticity form as:
GJT is constituted as:
ST is the station-to-station journey time including any interchange connection time. Footnote 9 The frequency penalty (τ) and interchange penalty (ω) convert service headway (H) and the number of interchanges (I) into equivalent journey time. Whilst GJT could, in principle, be enhanced with other terms, such as travel time reliability, rolling stock and crowding levels, their inclusion in the time-series models that provide the evidence base is fraught with serious problems such as the lack of historical evidence, the lack of variation over time and difficulties of measurement.
The estimation of the GJT elasticity (δ) has almost always been based on the ‘best’ prior evidence relating to τ and ω to construct GJT. The few exceptions are Wardman and Whelan ( 2004 ) and Wheat and Wardman ( 2017 ) who simultaneously estimated τ, ω and δ.
Whilst this GJT-Fare approach is more flexible than the GC approach, it still forces the elasticities to time, headway and interchange to depend upon the proportion that each forms of GJT. Footnote 10
The SC approach
The conventional (Marshallian) demand models of economic theory and practice typically relate the volume of demand to distinct terms such as time, cost, frequency and interchange. But in addition an extensive discrete choice literature has developed over the same period as GC’s existence and central to the latter is the composite utility term that reflects the attractiveness of an alternative. Whilst this could itself be made a function of a created GC, this is never done and instead the key travel attributes enter separately. Footnote 11
For the variables of interest here, the conventional constant elasticity SC demand model specification would be:
Interchange takes this form because it is discrete and often zero, and the parameter ψ denotes the constant proportionate change in demand after a change in the number of interchanges. If connection time is separated from ST, as it is in some of our reported SC models, then it also enters in the same form as interchange, albeit in minutes rather than as the number of interchanges, given that it can be zero.
What insights on expected elasticity variation are provided by existing evidence?
Whilst we contend that there have been no studies that have tested the GC approach to the degree that is reported below, available evidence might still be able to shed light on the validity of the GC model’s elasticity properties.
The GC approach implies that there will be considerable variation in GJT and fare elasticities over time and across routes. Our experience from analysing rail demand and conducting review studies over the past 30 years is that it is often difficult to discern statistically significant variations in GJT and fare elasticities that overturn constant elasticity models, let alone ones that approach those implied by the GC approach. Notwithstanding our impressions and experiences, a much firmer empirical basis is provided by drawing upon the insights provided by three major reviews and meta-analyses of UK evidence.
Wardman ( 2014 ) covers 1633 price elasticities for car, rail and bus drawn from 167 studies published between 1968 and 2010. Wardman ( 2012 ) covers the period 1977–2010 and 209 (rail) GJT elasticities and 168 IVT elasticities. Abrantes and Wardman ( 2011 ) cover 1749 valuations between 1960 and 2008 including 933 IVT values, 29 car congested time values and 39 car free flow values.
The evidence from these major meta-analyses can be used in conjunction with Eq. 6 to provide insights as to whether the empirical relationships between the time elasticity, price elasticity and value of time support the GC approach.
The relevant insights from these meta-analyses are summarised in Table 1 for the key variables of income growth over time, journey distance and journey purpose. We distinguish rail from other modes given that this paper analyses rail data and that rail provides substantial proportions of the meta-analysis evidence. The elasticity terms in Table 1 indicate how the value of time varies with income or how an elasticity varies with distance. So the distance elasticity for IVT for rail indicates that a 10% increase in distance would increase the IVT elasticity by 2.5%. The other terms denote proportionate effects on a parameter.
Income is a very important variable given that the value of time increases with it over time and hence the balance between the GJT and fare elasticities implied by the GC approach would be expected to vary with income growth, all else equal. However, all else is not equal, and the ratio GJT/F might vary over time. We subsequently analyse a data set covering the period 1995–2005 and over that period the GJT/F ratio fell by around 10% or 1% per annum, being broadly similar across the different types of flow covered. Given an elasticity of the value of time with respect to income of 0.9, the value of time would have increased by around 2% per annum over that period for rail. Hence we might expect the elasticity of the GJT elasticity with respect to income to be larger than the corresponding fare elasticity variation. This is not the case.
Turning to car, real money costs have increased over time but so have journey times due to greater congestion. Over the period 1995–2014, inspection of National Travel Survey data (Leigh Fisher et al. 2016 ) implied that the ratio of time to cost was falling by around 1½ % per annum. This contrasts with 2% value of time growth per annum and hence is not entirely consistent with the GC approach given the absence of income effects on the time and price elasticities.
As for bus travel, fares have increased over time but then speeds have also deteriorated. The net effect would be expected to dampen but not remove the effect of the value of time growth within Eq. 6 whereby time elasticities should become more important than price elasticities over time but this is not the case.
With regard to journey distance, it is not clear how the GJT/F ratio for rail might be expected to vary. Whilst fares taper with distance, longer distance journeys are often faster and service frequency does not deteriorate in line with distance. Inspecting our 1995–2005 data sets, we regressed the logarithm of GJT/F on the logarithm of miles which provides elasticities which can conveniently be compared with the value of time distance elasticity of 0.16 reported in Table 1 . The estimated elasticity of GJT/F to miles was − 0.16 for Non London flows and − 0.10 for London flows. Thus the variations in GJT/F and the value of time in Eq. 6 essentially cancel out, implying that the time and fare elasticities should exhibit similar variation with respect to distance. However, we find that the rail time elasticities increase with distance quite strongly whereas there is a slight reduction on some flows for the price elasticity.
With respect to bus travel, speeds are higher for longer distance journeys and fares tend to taper. The balance between time and fare might though be fairly constant with distance. The value of time increases with distance for bus, the fare elasticity is larger for inter-urban trips and the IVT elasticity is somewhat larger for inter-urban trips. We might then conclude that the distance effects for bus are in line with what would be implied by the GC approach.
For car travel, speeds are higher for longer distances but fuel efficiency is also better. The balance between time and cost might not vary much with distance. The evidence points to time and cost elasticities being similarly larger for inter-urban trips, but given the quite strong distance elasticity on the value of time for car then the GC approach would imply a somewhat greater increase in the time elasticity than the cost elasticity with distance.
As for the other main variable of journey purpose, the key variations relate to business travel. The value of time for business travellers is essentially twice that of other travellers and the price elasticity is just over half that of commuters and around 60% less than leisure travellers. The variations in the value of time and fare elasticity essentially cancel out in Eq. 6 and so, given that there were no variations in time elasticities by purpose, consistency with the GC approach requires that the balance between time and cost is similar between business and other trips. This can be expected to be the case for car but we would expect GJT/F to be somewhat lower for rail business travellers given that they purchase more expensive tickets to travel first class or at peak times.
Whilst there are inevitable approximations and assumptions involved in using Eq. 6 and review evidence to test the relationships implied by the GC approach, we conclude that this consideration of existing empirical evidence at the very least indicates that the new primary research reported here into the validity of the GC approach is clearly warranted. It is to this that we now turn.
Data used in analysis
The data used here is recorded tickets sales between pairs of railway stations. In Great Britain, this has since the 1970s supported empirical analysis of how a wide range of factors impact on the demand for rail travel.
Elasticity estimation requirements
The ability to estimate credible and precise elasticities is fundamental to the hypotheses here being tested. Drawing firm conclusions from comparisons of elasticities implied by the GC approach with those directly estimated using the GJT and Fare approach would clearly be hampered if the elasticity estimates were not plausible or possessed very large confidence intervals. Although not a primary requirement of our investigation, a desirable feature is that we can obtain reliable elasticity estimates for the component variables of GJT.
The ticket sales data used
Given that the issues we are here investigating are fundamental aspects of transport planning and forecasting, we have made use of the three datasets at our disposal which support our investigation in terms of being able to provide reliable GJT and fare elasticities. Within these data sets, we distinguish flow types which the rail industry in Great Britain customarily regard to be different and for which PDFH provides separate recommendations. Whilst this coverage of a range of datasets and flow types inevitably leads to an element of repetition, we feel that it is important to bring as much evidence to bear given the significance of the hypotheses we are testing and to determine whether our conclusions apply across a range of contexts.
Although rail has a significant presence in commuting markets in Great Britain, season ticket data is often unsuitable because the widespread use of area-wide ‘travelcard’ tickets in major urban areas means that we do not have an accurate account of travel between specific pairs of stations. In addition, key commuter flows tend to exhibit relatively little by way of GJT variation and fares are regulated.
Annual data for non-London non season tickets 2006–2010
This data was assembled for a project with the primary objective of examining the impact of large changes in timetable related service quality on rail demand (Wheat and Wardman 2017 ). Flows were selected where timetable changes had brought about an increase or reduction in GJT of at least 20% at some time in the period 2006–2010. This yielded 274 flows on Non-London long distance Footnote 12 routes. The monetary variables are all expressed in 2006 prices. The timetable related service quality data distinguishes train in-vehicle time, service frequency, the number of interchanges and any connection time.
Annual data for long distance non-season tickets 1996–2001
This data set was assembled for the specific purpose of examining the influence on rail demand of changes in timetable related service quality (Wardman and Whelan 2004 ). It covers 138 inter-urban London flows and 594 Non London inter-urban flows. All monetary terms are in 2001 prices. The timetable related service quality data distinguishes train in-vehicle time, service frequency, the number of interchanges and any connection time.
Annual data for non-season tickets 1995–2005
This data was provided in order to examine the effects of external factors on rail demand (Wardman and Dargay 2007 ). Nonetheless, it turned out to be ‘fit for purpose’ in terms of being able to recover precise and reasonable elasticities to fare and GJT. It covers 621 long distance London flows, 2442 long distance Non London flows, 738 Non London short distance flows not entirely within travelcard areas and 686 flows within the South East of England around London but not entirely within the London Travelcard Area. The monetary variables are expressed in 2005 prices. The timetable related service quality data covers station-to-station journey time, without any distinction between in-vehicle time and connection time, along with service frequency and the number of interchanges.
Summary of data analysed
Table 2 summarises the data we have used in testing the hypotheses set out in “ The opportunity and aims ” section. We have removed flows to and from airports as atypical. The largest removal of observations was for long distance London flows covering the years 1995–2005. Models estimated to the full data set failed to recover sensible GJT and fare elasticities and this was attributed to volatility in demand on some minor flows. We removed 149 flows where in any year demand was less than 5 return trips per day which then enabled sensible results to be obtained. Note that whilst this reduces the number of flows by 24%, the number of recorded journeys is reduced by less than 1%. Excepting this flow type, it is clear from Table 2 that the modelled data is the vast majority of the available data.
Model specification
The candidate model forms.
In conducting our tests of the central hypothesis of whether GC is justified, we estimate six different model forms for each of our data sets. These are:
GJT-Fare: The standard GJT and fare model of the rail industry in Britain
GC-Direct1: The GC formulation with a single directly estimated value of time.
GC-Direct2: The GC formulation with a directly estimated value of time that is proportional to income. Footnote 13
GC-Meta: The GC formulation with the value of time derived from the meta-model of Eq. 10 below weighted by journey purpose.
GC-Official: The GC formulation with the value of time based on official recommendations and weighted by journey purpose.
SC: Splitting GJT into its separate components of in-vehicle-time, headway, interchange and, where possible, connection time
All models can be estimated by ordinary least squares regression except for GC-Direct1 and GC-Direct2 where non-linear least squares is required.
The mix of journey purposes used in calculating overall values of time in the GC-Meta and GC-Official models is set out in Table 3 . Note that the results are not very sensitive to even quite large variations upon these journey purpose shares.
GC and GJT weights
The weights attached to headway and interchange in constructing GJT are set out in PDFH and we take these as given for the purposes of the analysis conducted here.
GC-Meta and GC-Official follow the conventional approach of adopting values of time (VoT) from prior evidence to construct GC. The former uses the values of time implied by the extensive meta-analysis of UK evidence reported in Abrantes and Wardman ( 2011 ) which is:
VoT is in pence per minute in 2008 quarter four prices, D denotes distance in miles, G is GDP per capita with an index of 5927, EB and Comm are dummy variables denoting the purposes of employer’s business and commuting, IU denotes whether the trip is inter-urban, which is deemed to be over 20 miles, and SE represents a journey made in the South East of England which is more affluent as well as having one of the densest rail networks in the world.
Another source of values of time is, quite naturally, official UK recommendations. We used the values recommended when we commenced this research, which were 44.76 pence per minute for business, 11.35 for commuting, and 10.07 for leisure trips, in 2010 prices and incomes. The income elasticity is unity for business travel but 0.8 for all other trips in line with the Department for Transport recommendations at the time. Footnote 14
The key external drivers of non-season rail demand are economic activity and income, and the models reported here contain regional GVA per capita specified for the origin station. Of course, other variables impact on rail demand, such as car ownership levels, population, and the costs, journey times and service frequencies of other modes. We have not included these terms for a number of reasons. Firstly, obtaining reliable historic evidence on some of these terms, such as route specific car journey times and bus fares and service frequencies, is notoriously difficult. Secondly, some of the external factors tend to be highly correlated, such as income, car ownership, population and in some years car fuel costs. Thirdly, the cross-elasticity effects tend to be of second-order (ATOC 2013 ; TRL et al. 2004 ; TRRL 1980 ). Footnote 15 Finally, some of these influences, especially those that vary little over time, will have been discerned by the fixed effects in our estimated models. Taken overall, we feel it is highly unlikely that the conclusions we draw would have been materially altered with the inclusion of a wide range of other factors even if it were possible to reliably do so.
The reported models take the following general form:
\(V_{ijt}\) is the demand for rail travel between stations i and j in time period t. It is a function of n continuous variables ( \(X_{ijkt}\) ), entered so that their coefficients ( \(\alpha_{k} )\) are interpreted as elasticities, and m continuous variables ( \(X_{ijlt}\) ), entered so that their coefficients \((\beta_{l} )\) denote the proportionate change in demand after a unit change in the variable whereupon the elasticity is then proportional to the level of the variable \((\beta_{l} X_{ijlt} )\) . In addition, there are \(s\) discrete variables denoted by the dummy variables \((D_{ijrt} )\) and their coefficients \((\gamma_{r} )\) denote the proportionate effect on demand of a particularly category of a variable relative to an arbitrarily selected base category.
We explored both fixed-effects (FE) and the more parsimonious random-effects (RE) specifications. The FE specification is equivalent to dummy variables \((D_{ijrt} )\) for p − 1 of the p station-to-station movements without any variation by time period t, effectively estimating route specific intercepts, and is potentially useful for controlling for unobserved heterogeneity over the cross-sections when that heterogeneity is constant over time. By contrast, a RE specification assumes that any differences between cross-sections are essentially random and that all cross-sections have been drawn from the same underlying population.
For each flow type, we computed two statistics to test whether a FE or RE specification of the GJT-Fare model was preferred. The first test is of an F statistic that tests the null hypothesis that the fixed-effect parameters are all zero. Rejection of the null hypothesis suggests an FE specification may be appropriate. The second test is of a Hausman m statistic that provides information about the appropriateness of the RE specification. The m statistic is based on the idea that, under the null hypothesis of no correlation between the effects variables and the regressors, OLS and GLS are consistent, but OLS is inefficient. Hence, the test is based on the result that the covariance of an efficient estimator with its difference from an inefficient estimator is zero (SAS Institute 2011 ). Rejection of the null hypothesis suggests that an FE specification may be more appropriate.
In general, the greater the number of cross-sections (and the greater their within-cross-section homogeneity and between-cross-section heterogeneity), the more likely it is that FE will be supported and RE not supported. This is especially so as sample size approaches population size.
The results in Table 4 indicate that the FE specification performed better than the RE specification for all flow types. In respect of the former, the F test statistics indicated that the models need to recognise the panel structure of the data; the fixed-effects were in all cases found to be jointly significant. With respect to the latter, the random-effects specifications were rejected convincingly based on the Hausman test. This means that cross-sectional differences between routes are picked up by route-specific constants. Only in the Non-London Long Distance 1995–2005 segment is the case for FE not totally overwhelming. In the latter case, it is the shorter-distance trips (under 100 miles) which exhibit less unattributable route-specific variation. However, since we have not segmented by distance, we prefer its FE specification.
We subsequently proceed with the FE specification, although the (numerous) fixed-effect parameters are not reported. It turned out that the GJT, Fare and GVA elasticities from the FE and RE models were generally very similar as is reported in “ Appendix ”.
Empirical findings
Model outputs.
The model outputs for the seven sets of flows described in Table 2 are reported in Tables 5 , 6 , 7 , 8 , 9 , 10 and 11 , with long distance London flows first, followed by long distance Non London flows and then the South East flows and finally the short distance Non London flows. The estimated models are logarithmic transformations of Eq. 11 and the SAS package was used. These models are estimated by ordinary least squares, except GC-Direct1 and GC-Direct2 where non-linear least squares is used to enable estimation of the VoT within GC.
Common elements of each table and each model are the GVA per capita elasticity estimate, the residual sum of squares (RSS), the adjusted R 2 goodness of fit measure (ADJ R 2 ) and, in the table heading, the number of observations. The GC, GJT, fare, headway, IVT and station-to-station time (TIME) coefficient estimates are all elasticities (X ijkt in Eq. 11 ). INT denotes the number of interchanges and CONN is any connection time (X ijlt in Eq. 11 ) which are entered in absolute form since they can be zero. The t ratio for each coefficient estimate is reported in brackets.
VoT-Est denotes an estimated value of time, in pence per minute. For the GC-Direct2 model, where the value of time is forced to increase in line with income, it denotes the estimated value of time for the first year before the subsequent income effect.
The row denoted VoT takes several forms. For the GJT-Fare model and the SC model it is the mean value of time across flows for the first and last years in the data set deduced using Eq. 5 . For GC-Direct1 it is the constant directly estimated VoT. For the other cases, we report the mean value for the first and last year in the data set. GC-Direct2 and GC-Official have common VoTs across flows and hence the standard errors, given in brackets, are zero.
The rows denoted η GJT and η FARE report the mean GJT and fare elasticities respectively implied by the four GC approaches for the first and last years in the respective data sets, along with their standard errors in brackets. For the SC model in the final column, η GJT is the sum of α, β and ψI of Eq. 9 , where I is taken as its mean level in the data for the years in question. Where connection time is separately identified, then its contribution to η GJT is its coefficient estimate multiplied by the mean amount of connection time.
For the models reported in Tables 6 , 8 and 9 , the Durbin–Watson statistic was in the range 1.6–2.0. This indicates autocorrelation is not an issue. In the other tables, which all cover the 1995–2005 data, the Durbin–Watson statistic was very much centred around 1. Nonetheless, the t ratios are so large that allowing for autocorrelation, or indeed inspecting and correcting for heteroscedasticity, would not make a material difference to the confidence that could be placed in the parameter estimates.
In all models, the GJT, fare and GC elasticities are precisely estimated, almost always extremely so, and the GJT and fare elasticity estimates are on the whole very credible and broadly in line with PDFH recommendations and major reviews and meta-analyses of UK evidence on fare elasticities (Wardman 2014 ) and GJT elasticities (Wardman 2012 ). Footnote 16 We conclude that the data sets provide a very firm basis for our tests.
The SC model is not central to the hypotheses here being tested but the results are of interest not only because its performance relative to other model forms indicates that it warrants further and more detailed attention but also because we here provide new evidence where little exists. The IVT/TIME elasticity is generally estimated very precisely and in all but one case, where it exceeds the estimated GJT elasticity, it is plausible. The importance of interchange comes across very clearly and the headway elasticity estimates are plausible and highly significant in four of the seven cases. The results for CONN are disappointing, but correlation with INT could be an explanatory factor. Nonetheless, the general credibility of the separate estimates for variables that underpin GJT gives us further confidence that our GJT data provides a robust basis for the tests we are undertaking.
The other key elasticity estimate relates to GVA per capita which is specified relative to the origin station. A noticeable feature of the result in Table 9 is that the GVA elasticity is negative. This is because over the period there was a sharp contraction in GVA yet for complex reasons beyond the scope of this investigation rail demand continued to increase. We do not feel though that this invalidates the investigation of our key hypotheses for this dataset.
But more importantly, it can be seen that the GVA elasticity is very similar across the GJT-Fare, GC-Direct1 and SC models, which contain no other income term. For the GC-Direct2, GC-Meta, and GC-Official models, which contain income within the value of time element of GC, the coefficient on GVA is more positive. In these cases the true GVA elasticity is the coefficient on GVA plus a fraction of the GC elasticity which, being negative, means the true GVA elasticity is less than the GVA coefficient. For example in Table 6 , approximately 60% of the GC elasticity needs to be taken off the ‘direct’ GVA elasticity in order to generate the ‘true’ GVA elasticity. Once this adjustment is undertaken, the GVA elasticities for the cases where VoT depends on income are broadly comparable to those where VoT does not depend on income. Thus the central story as regards GVA is that all six model forms give similar results on any given data set.
The remaining parameter that we estimate is VoT. We are not aware of direct demand models outside this stream of work that have directly estimated VoT. The VoTs are estimated extremely precisely. These estimated values along with the prior official and meta-analysis values used are central to the hypotheses here being tested and are summarised and discussed in “ Insights into values of time ” section.
A key feature of this research investigation is the extent to which the GJT and fare elasticities implied by the GC models are consistent with the freely estimated elasticities. We provide summary statistics and discussion surrounding them in “ Implied and directly estimated GJT and fare elasticities ” section but note some patterns here. The models which directly estimate the VoT can be seen to provide average implied GJT and fare elasticities that are broadly in line with the directly estimated GJT and fare elasticities. This is not the case with the models using pre-specified values, where there is a tendency to place too much weight on the impact of GJT changes and too little on fare changes. This is hardly surprising given that the prior VoTs are generally larger than the freely estimated VoTs.
Our view is that we have here an impressive set of plausible, precise and in some regards original estimates, and that these provide a firm basis for the pioneering tests that we are here conducting. We now discuss these tests and investigations in more detail. These cover:
Goodness of fit comparisons of different model forms
Comparisons of directly estimated GJT and Fare elasticities with those implied by the GC approach and of directly estimated and implied GC elasticities
Insights into values of time
Explicit testing of the elasticity variation implied by the GC approach
Relative goodness of fit
Although not the single nor indeed most important criterion against which we assess the model outputs, it is informative to compare how the different models compare in terms of how well they explain the data.
For models with the same number of parameters, we can simply compare the adjusted R 2 or RSS. This can be done for the GJT-Fare, GC-Direct1 and GC-Direct2 models and also for GC-Meta and GC-Official models. However, the latter two have one less estimated parameter and hence we use an F test to compare the GC-Meta and GC-Official models as restricted cases of the GJT-Fare, GC-Direct1 and GC-Direct2 models and to compare the SC model with any other restricted model. The test F statistic is based on the residual sum of squares of the restricted (RSS R ) and unrestricted (RSS U ) models as:
with degrees of freedom r, denoting the number of restrictions imposed, and n − k, where n is the number of observations and k is the number of estimated parameters.
Table 12 reports the relative explanatory performance across all six models for each of the seven datasets. Noticeably, the SC model provides the best fit in all cases. This is an interesting finding although one that impacts more on future research priorities and needs than on the tests we are here conducting.
Of more significance here is that the GJT-Fare specification always provides a better explanation of the data than the GC models. This is our first evidence that the GJT-Fare model is preferable to various forms of the standard GC expression as typically formulated.
Another notable pattern in Table 12 is that GC-Direct2, which freely estimates a VoT that increases in line with income, is in all but one case superior to GC-Direct1 where the freely estimated VoT is constant. This is reassuring given that the income effect is to be expected but is by no means guaranteed in such free estimation.
We also take confidence in the finding that the GC models with directly estimated VoTs, which the railway industry is generally in a position to undertake, generally perform better than GC models based on ‘best evidence’ and official values.
It is noticeable, but nonetheless unsurprising, that the GC models based on official values, which primarily serve purposes of economic appraisal, tend to be the worst performing. This is though, in our understanding, the conventional way in which GC would be constructed.
This evidence points to the GC approach being inferior to other formulations, and that if the GC approach is to be used it is preferable to base it on directly estimated VoT with best evidence VoT better than ‘official’ values.
Implied and directly estimated GJT and fare elasticities
Table 13 summarises the previous evidence regarding implied and directly estimated elasticities. The first three rows provide the average directly estimated and implied GC, GJT and Fare elasticities for each model across the seven data sets along with their standard errors.
The GC elasticities on average do not vary greatly, and are not significantly different. This is encouraging since we can then explore how similar GC elasticities are decomposed into GJT and Fare elasticities.
Turning to the GJT and fare elasticities, on average the GC-Direct1 and GC-Direct2 models provide implied GJT and fare elasticities very similar to the directly estimated ones. GC-Meta tends to provide GJT elasticities a little larger than those directly estimated but somewhat lower fare elasticities with the GC-Official model on average performing well on GJT but poorly on fare. What is noticeable is that the official and review VoTs imply GJT elasticities around twice the fare elasticities, somewhat out of line with all other models. The SC model provides GJT elasticities farthest removed from those directly estimated but, as would be expected, its fare elasticities are very much in line with the GJT-Fare model.
Of course, average values of two parameters can be very similar even when the individual values are often somewhat different. We therefore report in the final three rows the means and associated standard errors of the proportionate absolute deviation of the direct and implied elasticities from those of the GJT-Fare model.
Whilst the proportionate deviations in the GC elasticities are significant in all cases, they are nonetheless small. This similarity of elasticities is though not the case with regard to GJT and fare. We can see that when the VoT is directly estimated the implied GJT and fare elasticities are on average less than 10% different to the directly estimated values. Contrast this with the cases where the VoT is based on prior evidence. Here the implied GJT elasticities of the GC-Meta and GC-Official models are on average just over 20% different to the directly estimated ones with corresponding figures of 35 and 27% for the fare elasticity. The SC model performs by far the poorest on implied relative to directly estimated GJT elasticities. We attribute this to the greater challenges of obtaining precise estimates when there are more service quality variables to be covered, including the increased possibilities of multicollinearity and of lack of variation in some of the more detailed attributes.
At the level of central estimates, it seems that the GC approach can approximate the GJT-Fare model well when the VoT is directly estimated but there are concerns when predetermined VoTs are used to construct GC.
Table 14 summarises the implied, directly estimated and prior VoTs for each model type in terms of their mean and associated standard errors. We are unaware of VoTs being directly estimated in this way from direct demand models.
On average, there is little to choose between the mean VoTs except for the larger value of the GC-Meta model. However, when we inspect the proportionate absolute deviation relative to GC-Direct2, given that it directly estimates a VoT that is allowed to increase with income, we see a very close correspondence with the freely estimated but constant VoT of GC-Direct1 and with the implied values of the GJT-Fare model. The VoTs based on prior evidence are very different on average.
This evidence is indicating that the averages of the directly estimated VoTs are similar to each other and to that implied by the GJT-Fare model but are distinctly different to the official and meta-analysis evidence. The VoTs implied by the SC model differ but are on average closer to the former than the latter. In several cases, the standard approach of using ‘best evidence’ would involve VoTs somewhat different to those which best explain rail demand and implied by the balance between time and price elasticities.
Testing the implied elasticity variation
So far, we have compared at an average level actual and implied elasticities and values of time along with explanatory performance. However, a more rigorous test of the GC approach, whichever of our types, is to examine the spread of implied elasticities rather than just the means. Are the variations in the GJT and Fare elasticities implied by the GC approach empirically justified?
Equations 3 and 4 denote the dependence of the implied GJT and fare elasticities of the GC approach on the proportion that each forms of GC. We therefore created dummy variables denoting five different categories of expected elasticity according to the proportion that GJT and Fare form of GC. In order to avoid endogeneity problems, the five categories on each flow are defined for the first year in the time-series Footnote 17 and are created using the constant VoT of the GC-Direct1 model.
We then specify an extended GJT-Fare model that allows the GJT and Fare elasticities to vary with the proportion that each forms of GC. Ignoring terms other than GJT and fare, this model takes the form:
The d 2 , d 3 , d 4 and d 5 are dummy variables denoting sensible categorisations of expected elasticity variation. We use five categories to provide a more rigorous test, given that the previously reported models have very precisely estimated elasticities to GJT and fare and hence can support so many categories. Footnote 18
Interpreting the model parameters with regard to GJT, the base elasticity is δ 1 . For the second expected elasticity category the GJT elasticity is δ 1 + δ 2 and for the fifth it is δ 1 + δ 5 . Given that we specify the dummy variables to denote increasing proportions that GJT forms of GC, we would expect that δ 5 < δ 4 < δ 3 < δ 2 < 0. For fare we would expect that γ 5 > γ 4 > γ 3 > γ 2 > 0.
Table 15 reports the segmented models for each flow type, covering quite large variations in expected elasticity variation but bearing in mind the split of observations across each category. Exp η GJT and Exp η F denote the expected elasticity categories for GJT and fare respectively with Est η GJT and Est η F reporting the estimates of Eq. 13 . The first column of results provides the base GJT or fare elasticity and their t statistics. These are for categories that are the lowest (highest) proportions that GJT (fare) forms of GC. The properties of the GC approach would require that as we move to the right in Table 15 the incremental effects should imply a GJT (fare) elasticity that becomes more (less) negative.
Encouragingly, all the base GJT and Fare elasticities are statistically significant, and almost all are estimated with a high level of precision. However, around 40% of the incremental effects were not significant at the usual 5% level. Moreover, it is only in the case of the GJT elasticity for South East to and from London flows that there is a convincing case in support of the GC implied elasticity variation, although a few more instances could be argued to possibly support the GC approach but then relying on the argument that all else is not equal across the various categories. We should also point out that the pattern of results was not greatly different when the segmentations of the GJT elasticity and the fare elasticity were conducted separately.
We conclude that robust models, with large sample sizes and plausible and very precise coefficient estimates in their standard form, are not able to support the variation in GJT and fare elasticities that would be implied by the GC approach.
Conclusions
The GC formulation has been used in transport models for many years to represent the overall attractiveness of different alternatives and can be regarded as an ‘industry standard’. Given its significant place in transport planning, modelling and forecasting, an observer might reasonably expect that its quite specific implied elasticity properties would have been subject to empirical testing, and indeed this point was made many years ago by Grey ( 1978a , b ). That same informed observer might also ponder the stark contrast between the large variation in time and fare elasticities implied by the GC approach and the common failure of demand models to find statistical support for alternatives to the conventional constant elasticity formulation. Indeed, examination of the elasticity and value of time review literature raises serious questions surrounding the implied elasticity properties of GC models.
We contend that there has been little empirical questioning of the implied elasticity properties of the GC formulation, and also of the VoTs that underpin it, and moreover that the limitations of the GC approach have been forgotten, overlooked or not appreciated, and it is used because ‘it has always been used’.
The research reported here is original and significant in conducting detailed tests of whether the GC approach is justified, directly estimating the VoT that should be used in constructing GC and making a significant contribution to the evidence base regarding a number of rail demand elasticities.
The opportunity to test the properties and parameters of the GC approach is afforded by the unique availability of large amounts of rail ticket sales data in Great Britain alongside the necessary variations in service quality attributes over time that complement more customary price variations. Such data sets and the required variations do not exist for other modes.
We have examined six model forms across seven different data sets. Four models are of the GC formulation, of which two represent conventional practice, with GC constructed using official values of time and best evidence as represented by the findings of meta-analysis, and two which can be regarded to be original in directly estimating the VoT within the GC function that best explains demand responses. The two alternatives to these GC formulations are a model based on GJT and fare (GJT-Fare), which represents the well-established analysis and forecasting approach used by railways in Great Britain, and an approach more akin to disaggregate model, where GJT is split into its separate components (SC) covering journey time, service headway, interchange and connection time.
The elasticities across the various models we report are generally plausible and estimated with a very high degree of precision whilst the directly estimated VoTs are almost always extremely precise. We therefore have a very firm basis for empirical testing of the GC approach.
In terms of our hypotheses set out in “ The opportunity and aims ” section, we have found that:
The industry standard GJT-Fare model always provided a better fit than our four candidate GC approaches. Amongst the GC models, those that directly estimate the VoT almost always provided a better fit than those that use pre-determined values even though the latter contain more route specific variations, such as by distance and purpose, than the former.
The GC models that directly estimate the value of time imply mean GJT and fare elasticities that correspond well with the directly estimated GJT and fare elasticities. This though is not the case when pre-determined VoTs are used in constructing GC.
The temporal and spatial variations in GJT and fare elasticities according to the proportions that they respectively form of GC as forced by the GC approach are not supported when explicitly tested in robust rail demand models based on large data sets.
Examination of the review literature finds that the variations in the fare elasticities, time elasticities and values of time are not consistent with what would be implied by the GC approach.
If the GC approach is to be used, then the directly estimated VoTs that best explain the balance between the demand impacts of time and fare changes are somewhat different to the pre-determined values.
Whilst directly estimated VoTs are clearly preferable within the GC approach, this will not always be possible for rail and, significantly, will hardly ever be the case for other modes. In addition, the evidence might even be indicating that official VoTs, based largely on Stated Preference evidence, are inaccurate.
In summary, the results raise serious concerns about the wisdom of the composite GC term in demand forecasting. Bruzelius ( 1981 ) queried whether we had “really shown that such models work?” We have shown that they do not work. To answer the question that is the title of this paper: GC in the form that it conventionally takes is not justified in a variety of rail markets. Given that the problems with GC apply pari passu to models based on Generalised Time also, Gunn’s ( 1983 ) argument that “neither time nor cost is likely to provide a plausible unit system for conventional forecasting” further supports the view that the railway industry in Great Britain is entirely vindicated in its persistence with the GJT-Fare approach rather than going down the GC path.
Whilst the findings of this study are original, extensive and significant, much further research on this important subject is needed.
We have restricted our investigations to the standard constant elasticity models that dominate the analysis and forecasting practice based around aggregate models of the direct demand form. More flexible functions which allow the GC elasticity to vary can be estimated which also permit closer approximation of the GC, GJT-Fare, and the SC models through appropriate parameterisation of each model. For example, we might reject the strong elasticity variation forced by the conventional GC approach and here tested, but a more limited degree of variation might be supported using a more flexible functional form. Equally, interaction terms within the GJT-Fare and SC approaches can bestow elasticity properties similar to the GC approach.
We have not here tested the weights used to construct GJT, and hence also GC, and whilst the SC approach demonstrates promise it makes more demands of the data and we face a challenge in recovering precise and plausible estimates of all parameters. Nor have we considered lagged behavioural responses, where there are inevitable additional challenges of interpretation if GC, GJT, fare and the SC variables exhibit different lags.
We have here focussed entirely on direct demand models based on secondary data. There is, of course, an ‘alternative world’ of behavioural modelling at a more disaggregate level based upon discrete choice models. These models do not use GC as an independent variable, although they could, and the default here is essentially the SC approach. An important point though is that such models in practical application often force considerable elasticity variation without empirical justification along the lines of the elasticity variation imposed by the GC models considered here. Nor have we considered the justification of using GC in network modelling.
There are therefore important further research issues to investigate, but our conclusions regarding the validity of the GC approach are profound and provide a long overdue start in a very much neglected area of analytical transport research.
For example, the GC approach has attractions where, as is often the case in the car and bus markets, there is sufficient evidence on price elasticities but a dearth of evidence on time elasticities (TRL et al. 2004 ).
There is also what Gunn ( 1983 ) termed “a crucial ambiguity” that models expressed in terms of GC (GT) will imply a reduction (increase) in demand over time as the value of time increases with income. We do not here address this issue but rather focus our attention on the use of GC (GT) in the elasticity domain.
The wait time element common in GC calculations is taken up by the frequency term. Walk time is also a common feature of GC but not in the rail demand models which form the evidence base here since they are estimated to recorded demand between stations.
We take this to be the conventional formulation where GC is a linear-additive combination of its component parts.
It is surprising why there is so little evidence on GC elasticities when GC is so widely used in forecasting.
Indeed, our experience is that when rail demand models are allowed to depart from the standard constant elasticity position the alternative is either rarely empirically preferable or else, with flexible functional forms, the estimated elasticity variation is slight.
For example, the TRL et al. ( 2004 ) review of public transport demand elasticities uses this relationship to provide deduced time-based elasticities in the bus market where there is a dearth of directly estimated evidence. Of course, the elasticities to other terms within GJT can also be deduced.
The initial PDFH was issued in 1986 and the most recent update (v6) was undertaken in 2017. Updates on the original were 1989 (v2), 1997 (v3), 2002 (v4), 2005 (v4.1), 2009 (v5) and 2013 (v5.1).
The standard formulation of GJT in PDFH remains station-to-station journey time even though it has been possible for many years to isolate connection time in the industry model that supplies the GJT data. All GJT models reported in this paper do not isolate the connection time component of station-to-station time.
Nonetheless, this separation of GJT and fare, as advocated by Jones ( 1977 ), would overcome Gunn’s “crucial ambiguity” units problem when using either GC or GT in forecasting over time.
These choice models have their own untested properties, such as the elasticities being very strongly influenced by market share.
In Britain, the railways define long distance to be over 20 miles (32 km).
We did experiment with freely estimating both the value of time and its income elasticity but this proved to be a ‘step too far’!.
The UK Department for Transport has recently revised its official valuations. Nonetheless, the officially recommended values of time over the periods of the data we have analysed are more appropriate since they would have been used in creating GC at the time.
Indeed, the required homogeneity of degree zero of a full demand system implies that all cross-elasticities for substitutes must be smaller than the own-elasticity, even more so if the income elasticity is positive.
We are not aware of GC elasticity evidence relating to rail travel which could be used here for comparison purposes.
The numbers in each category do not vary much depending upon whether the categories are defined for the first year or separately for each year. And the conclusions are not altered when the latter specification was used.
We experimented with four and three categories, but the findings would not alter our conclusions.
Abrantes, P.A.L., Wardman, M.: Meta-analysis of U.K. values of time: an update. Transp. Res. A 45 (1), 1–17 (2011)
Article Google Scholar
Association of Train Operating Companies.: Passenger Demand Forecasting Handbook. Edition 5.1, London (2013)
Balcombe, R., Mackett, R., Paulley, N., Preston, J., Titheridge, H., Wardman, M., White, P.: The demand for public transport: a practical guide. TRL Report 593, Crowthorne, Berks (2004)
Bruzelius, N.A.: Microeconomic theory and generalised cost. Transportation 10 , 233–245 (1981)
Department for Transport.: Values of time and operating costs. Transport Analysis Guidance (TAG) Unit 3.5.6. www.webtag.org.uk (2006)
Goodwin, P.B.: On Grey’s critique of generalised cost. Transportation 7 , 281–295 (1978)
Goodwin, P.B.: A review of new demand elasticities with special reference to short and long run effects of price changes. J. Transp. Econ. Policy 26 (2), 155–163 (1992)
Google Scholar
Grey, A.: The generalised cost dilemma. Transportation 7 , 261–280 (1978a)
Grey, A.: Has generalised cost any benefit? Transportation 7 , 417–422 (1978b)
Gunn, H.F.: Generalised cost or generalised time? A note on the forecasting implications. J. Transp. Econ. Policy 17 (1), 91–94 (1983)
Jones, I.: Urban Transport Appraisal. Macmillan, London (1977)
Book Google Scholar
Leigh Fisher, ITS Leeds, RAND Europe and SYSTRA: Rail demand forecasting extension. Prepared for Department for Transport. available at https://www.gov.uk/government/uploads/system/uploads/attachment_data/file/610059/phase2-rail-demand-forecasting-estimation-study.pdf (2016)
McIntosh, P.T., Quarmby, D.A.: Generalised costs and the estimation of movement costs and benefits in transport planning. MAU Note 179, Mathematical Advisory Note, Department of the Environment (1970)
SAS Institute.: SAS/ETS(R) 9.3 user’s guide: the PANEL procedure. http://support.sas.com/documentation/cdl/en/etsug/63939/HTML/default/viewer.htm#etsug_panel_sect004.htm (2011). Accessed 23 May 2017
Searle, G.: Generalised cost: fool’s gold or useful currency? Transportation 7 , 297–299 (1978)
Transport Research Laboratory (TRL), Centre for Transport Studies University College London, Transport Studies Unit University of Oxford, Institute for Transport Studies University of Leeds, Transport Studies Group University of Westminster.: Demand for public transport: A practical guide. TRL Report TRL593, Crowthorne, Berkshire (2004)
TRRL.: The demand for public transport. Report of the International Collaborative Study of the Factors Affecting Public Transport Patronage. Crowthorne, Berks (1980)
Tyler, J., Hassard, R.: Gravity/elasticity models for the planning of the inter urban rail passenger business. In: Proceedings of Seminar G, PTRC Summer Annual Meeting, Warwick (1973)
Wardman, M.: Forecasting the impact of service quality changes on the demand for inter urban rail travel. J. Transp. Econ. Policy 28 (3), 287–306 (1994)
Wardman, M.: Price elasticities of surface travel demand: a meta-analysis of UK evidence. J. Transp. Econ. Policy 48 (3), 367–384 (2014)
Wardman, M.: Review and meta-analysis of U.K. time elasticities of travel demand. Transportation 39 (3), 465–490 (2012)
Wardman, M., Dargay, J.M.: External factors data extension and modelling. Prepared for Department for Transport, Institute for Transport Studies, University of Leeds (2007)
Wardman, M., Whelan, G.: Estimating the effects of service quality changes on the demand for rail travel. Paper presented at AET European Transport Conference (2004)
Wheat, P., Wardman, M.: Effects of timetable related service quality on rail demand. Transp. Res. A 95 , 96–108 (2017)
Wilson, A.: The use of entropy maximising models. J. Transp. Econ. Policy 3 (1), 108–126 (1969)
Download references
Acknowledgements
An earlier version of this work was presented at European Transport Conference 2008. This paper extends that work considerably. We are grateful for the comments of two anonymous referees.
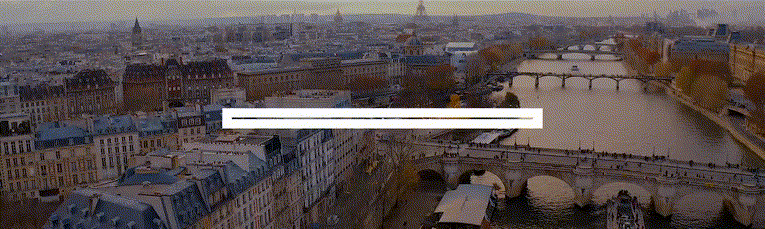
Author information
Authors and affiliations.
SYSTRA, Manchester, UK
Institute for Transport Studies, University of Leeds, Leeds, UK
Mark Wardman & Jeremy Toner
You can also search for this author in PubMed Google Scholar
Corresponding author
Correspondence to Mark Wardman .
Appendix: Key parameters of fixed effects and random effects models
See Table 16 .
Rights and permissions
Open Access This article is distributed under the terms of the Creative Commons Attribution 4.0 International License ( http://creativecommons.org/licenses/by/4.0/ ), which permits unrestricted use, distribution, and reproduction in any medium, provided you give appropriate credit to the original author(s) and the source, provide a link to the Creative Commons license, and indicate if changes were made.
Reprints and permissions
About this article
Wardman, M., Toner, J. Is generalised cost justified in travel demand analysis?. Transportation 47 , 75–108 (2020). https://doi.org/10.1007/s11116-017-9850-7
Download citation
Published : 14 February 2018
Issue Date : February 2020
DOI : https://doi.org/10.1007/s11116-017-9850-7
Share this article
Anyone you share the following link with will be able to read this content:
Sorry, a shareable link is not currently available for this article.
Provided by the Springer Nature SharedIt content-sharing initiative
- Generalised cost
- Travel demand modelling
- Value of time
- Find a journal
- Publish with us
- Track your research
- Systematic Review
- Open access
- Published: 04 April 2024
Effectiveness and cost-effectiveness of community-based mental health services for individuals with severe mental illness in Iran: a systematic review and meta-analysis
- Mozhgan Taban 1 ,
- Sara Nooraeen 2 ,
- Kiarash Tanha 3 ,
- Maziar Moradi-Lakeh 4 &
- Seyed Kazem Malakouti 5
BMC Psychiatry volume 24 , Article number: 256 ( 2024 ) Cite this article
90 Accesses
13 Altmetric
Metrics details
Severe mental illness (SMI) imposes a substantial worldwide burden of disability, highlighting the need for comprehensive and adaptable mental health services. This study aims to assess the efficacy and cost-effectiveness of community-based mental health services (CBMHS) in reducing relapse and rehospitalization rates among individuals with SMI in Iran.
A systematic review and meta-analysis were conducted. Medline, EMBASE, ISI, SCOPUS, and ProQuest were searched until December 2022. We focused on randomized controlled trials, quasi-experimental studies, or economic studies related to individuals with SMI. Out of 127 articles, 17 were selected for a full-text review. The primary outcomes were the severity of psychopathology, rehospitalization rates, and the mental health of caregivers. We also examined community-based interventions and their impact on various outcomes. Data extraction and risk of bias assessment were performed, and critical appraisal was conducted using JBI checklists. Meta-analysis was carried out using STATA software. (PROSPERO registration. CRD42022332660).
Rehospitalization rates among patients who received CBMHS were significantly lower, with an odds ratio of 2.14 (95% CI: 1.44 to 3.19), indicating a 2.14 times lower likelihood than those who received treatment as usual. A reduction in psychopathology accompanied this, SMD: -0.31, 95% CI: -0.49 to -0.13, I2 = 40.23%). Moreover, there was a notable improvement in social skills (SMD: -0.7, 95% CI: -0.98 to -0.44, I2 = 0.00%). The burden on caregivers also decreased (SMD: -0.55, 95% CI: -0.99 to -0.1, I2 = 63.2). The Incremental Cost-Effectiveness Ratio (ICER) for QUALY was acceptable, albeit with a wide range of 613 to 8400 Dollars.
CBMHS has demonstrated effectiveness and efficiency in Iran as a developing country. Additionally, it shows promise in mitigating the shortage of acute psychiatry beds. Using multiple data collection tools poses a limitation regarding data consolidation and conducting a meta-analysis.
Peer Review reports
Introduction
Severe mental illness (SMIs) is a prevalent global cause of disability. In 2019, mental disorders contributed to approximately 418 million disability-adjusted life years (DALYS), accounting for 16% of global DALYS. This represents a significant increase compared to previous estimates. The World Health Organization's (WHO) Action Plan (2013–2030) emphasizes establishing inclusive and adaptable community-based mental health and social care services. The goal is to empower individuals affected by these disorders to exercise their full human rights and gain timely access to culturally appropriate, high-quality healthcare and social support. This approach promotes recovery, enables individuals to achieve optimal well-being, actively participate in society and employment, and eliminates stigmatization and discrimination [ 1 ].
Although medication is essential for symptom control and relapse prevention, it is insufficient to address the social needs of patients with severe mental illness. In recent years, there has been notable progress in pharmacotherapy, particularly in managing the acute phase of the disorder, which has subsequently increased the inclination toward providing community-based mental health services (CBMHS) [ 2 , 3 ]. The various aspects of psychiatric disorders, including their health, familial, social, and economic dimensions, also highlight the different CBMHS, such as home visits, outpatient services, community-based rehabilitation, psychological training, family therapy, and other methods. Additionally, it acknowledges the financial burden that SMIs place on families and governments, with an estimated economic impact of approximately USD 5 trillion in 2019 [ 4 ].
Based on the findings of the Iranian Mental Health Survey (IranMHS) conducted in 2011, nearly a quarter of the population experiences psychiatric disorders, of which 3 to 5 presents suffer severe illness [ 5 ]. Furthermore, it emphasizes that these conditions are the leading cause of disability among individuals aged 10 to 40 in Iran [ 6 ].
Studies demonstrate that the provision of CBMHS effectively reduces relapse and rehospitalization rates in patients and alleviates the burden on families. Additionally, by lowering hospitalization and daycare costs, the economic burden associated with SMIS is reduced. However, offering these services in Low- and Middle-Income Countries (LMICS) encounters obstacles related to social, cultural, and financial factors [ 7 ]. In Iran, notable attention has been given to providing comprehensive, integrated, and responsive mental health services in community settings [ 8 ]. Conducted in Iran, it demonstrated a 67% reduction in hospitalization rates after individuals received CBMHS [ 9 ]. There are numerous studies conducted worldwide that have demonstrated the effectiveness and cost–benefit of community-based services [ 10 , 11 , 12 , 13 ]. However, in parallel with the expansion of community-based services, long-term hospitalization and asylum-like services were expanded nationwide in the last two decades. Our intention in this study was not to evaluate these services at the global level, but we checked whether this system works in Iran's cultural, social, and economic conditions, and maybe this Rio systematic is useful for mental health policymakers and shows that the development of these services requires It takes more effort. For this reason, we focused on Iranian studies.
To address the knowledge gap, we conducted a systematic review and meta-analysis of Randomized Clinical Trials (RCTs), quasi-experimental studies, or economic studies to evaluate the cost-effectiveness and cost benefit effectiveness of CBMHS in reducing relapse and rehospitalization rates among patients with SMIs. The findings of this study may have implications not only for future research in Iran but also for neighboring countries.
Eligibility criteria
RCTs, quasi-experimental studies, or economic studies conducted on specific outcomes such as clinical relapse, rehospitalization, cost, and the severity of psychopathology, were included. The intervention on individuals with a diagnosis of psychotic spectrum disorder (schizophrenia, schizophreniform, and other long-lasting psychotic disorders), Bipolar Mood Disorders (BMD), or severe refractory major depression were considered were considerd.
Amongst them, studies with any community-based intervention, including home-visit services (by professionals or peers and family members), telephone follow-up, family psychoeducation, and skill training, and the studies which aim the caregivers' knowledge, burden, and mental conditions as by proxy groups to have an impact on SMI clinical outcome were included.
Rehospitalization, relapses, clinical condition and severity of the symptoms, treatment adherence, economic outcomes including QALYS, CER (Cost-Effectiveness Ratio), social functioning, quality of life, and family knowledge were outcomes of interest.
Exclusion criteria were any studies performed on individuals with substance use disorders, intellectual disability, brain trauma, or; the intervention model was not transparent and did not have a follow-up interval.
The study protocol had been approved by the Ethics Committee of the Iran University of Medical Sciences (code: IR.IUMS.REC.1400.733) and registered in PROSPERO. (CRD42022332660, available here: https://www.crd.york.ac.uk/prospero/display_record.php?ID=CRD42022332660 .)
Search strategy
We systematically reviewed the published literatures on the intervention models for SMIs in Iran. This review includes all RCTs, quasi-experimental studies, or economic studies reporting on the effectiveness and cost-effectiveness of community-based care and interventions designed to promote social engagement among individuals with SMI.
The search was conducted for articles published in English using databases such as Medline, EMBASE, ISI, SCOPUS, and ProQuest. Additionally, peer-reviewed papers in the Persian language, were accessed via Iranian websites including SID, MAGIRAN, and Iran doc. Our search terms (keywords and Mesh terms) reflected central concepts: severe mental illness, models of intervention, outcome, and relapse. We limited our search to publications in English and Persian available in full text. If the full text was not available, the authors were contacted. publications included in the study were published until December 2022, the complete search strategy can be found in the Supplementary Material 1 : Appendix.
Since this study aimed to estimate the effectiveness of CBMHS, and some of the included articles used alternative versions of the questionnaires, we excluded the data from alternative versions. We extracted the relevant data by carefully studying the tables and text.
Screening and data extraction
Two independent reviewers (S.N., M.T.) assessed article titles and abstracts to exclude unrelated records. The full text of the remaining studies was also reviewed independently by S.N. and M.T., with unrelated articles being excluded. Any disagreements were resolved through discussion and judgment by the principal investigator (SK.M.).
A pre-designed data sheet was completed for each of the included studies. Data extraction from each included paper was performed by two independent authors (S.N., M.T.) based on the author's name, publication year, journal name, study population, city of the study population, sex, sample size, type of intervention, and tools.
Risk of bias assessment
The relevant JBI critical appraisal checklist regarding the study designs (i.e., RCT, quasi-experimental, and economic evaluation) was used to evaluate the articles. JBI critical appraisal Checklist for RCTs has 13 questions evaluating different methodological aspects of an RCT, including randomization, concealed allocation, blinding, follow-up, and analysis (all versions of JBI available: https://jbi.global/critical-appraisal-tools ) .
Statistical analysis
To ensure comparable results, we calculated Standardized Mean Differences (SMD) and 95% confidence interval between the intervention and control groups [ 10 ]. SMDs were calculated, where available, to assess the intervention's effectiveness during the follow-up period (i.e., a pre-post comparison in the experimental group) and to measure the differences between the experimental and control groups at the follow-up time (i.e., calculated as the post–pre-experimental mean minus the post–pre control mean). The odds ratio and 95% confidence interval were calculated to compare the rehospitalization rate between the groups.
The data were analyzed using STATA, version 17.0 (STATA Corporation, College Station, TX, USA). The statistical heterogeneity between the studies was assessed using the I2 statistic, which was able to measure the inconsistency across the results of the studies and describe the proportion of the total variations based on their estimates due to the presence of heterogeneity rather than sampling errors. A random-effects model was used if heterogeneity was observed (the I2 values > 50).
Seventy-one articles were selected through the English-language website, and fifty-six Persian-language articles were selected through the Iranian website. After removing duplicates, 115 articles remained. In the next steps, the titles and abstracts were reviewed, and 84 articles were excluded. The full text of thirty-one articles was reviewed, of which 14 unrelated articles were excluded. Finally, 17 English and Persian articles were included in the study (Fig. 1 ).
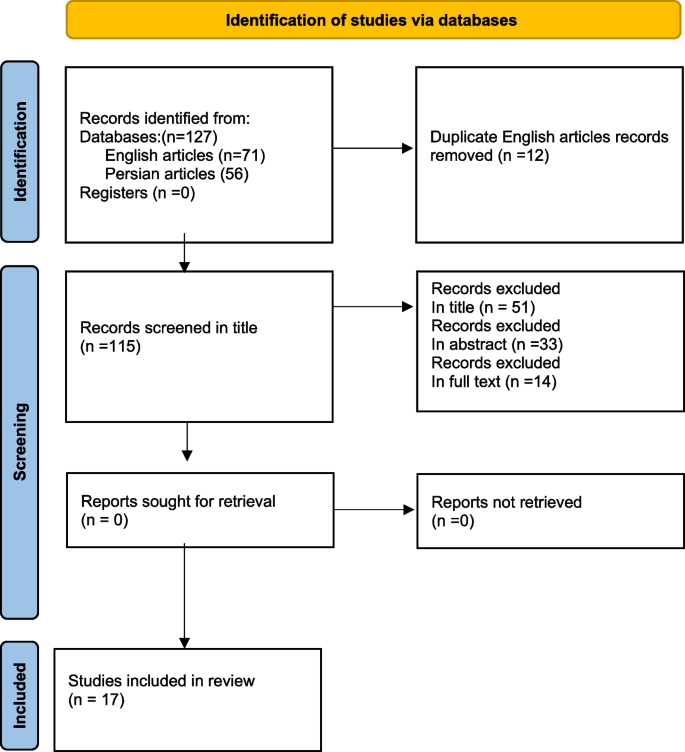
PRISMA flow chart to illustrate the article search and selection process
Critical appraisal and risk of bias assessment
The results of the risk of bias and critical appraisal in the qualitative assessment of the articles are as follows. We have 8 experimental articles that meet the inclusion criteria for our study. These articles were selected based on their relevance to our research question and their adherence to our predetermined criteria for experimental design. We evaluated the articles based on the JBI critical appraisal checklist (Fig. 2 ). Question four (Were participants blind to treatment assignment?) And question five (Were those delivering treatment blind to treatment assignment?) Were not applicable for most of the studies as in the communicate based intervention it is not feasible for the participants and the person who deliver the services to be blind of interventions. The rest of the items were enough qualified to rely on the results (Fig. 2 ). The result of the quasi experimental and economy studies checklists depicted in the Supplementary Material 1 :Appendix.
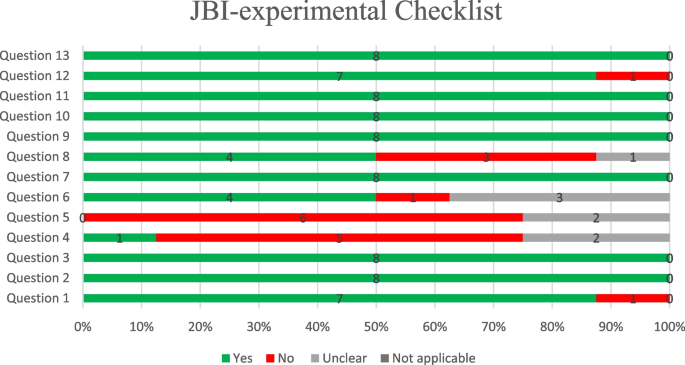
Quality assessment of experimental studies
The demographic characteristics of included articles
Demographic characteristics of included articles revealed Table 1 .
The results of intervention comparing before and after 12-months follow-up presented. The findings related to the tools used in intervention studies are presented in Table 2 . As noted, most tools reported a weak level of heterogeneity(I 2 ), so the meta-analysis has not been performed. The meta-analysis was conducted for rehospitalization, PANSS (Positive and Negative Syndrome Scale) as a psychopathology assessment tool and KELS (Kohlman Evaluation of Living Skills of the patients) and FEIS variables indicating psychological distress in caregivers.
The rehospitalization rate
One of the study's primary goals was to evaluate the effect of any CBMHS on rehospitalization after the index discharge. The CBMHS included home-visit and telephone follow-ups, the rehospitalization rate among patients who received CBMHS (with a total of 595 participants in both intervention and control groups) was 2.14 times lower compared to those who received treatment as usual (OR: 2.14,95%CI: 1.44, 3.19). Mohebi [ 17 ] was the only article that used Medication Adherence Rating Scale (MARS) to evaluate the compliance of the patient with treatment (SMD: 3.15, CI: 95% 2.31, 3.98). The result of the meta-analysis of rehospitalization among four studies showed in Fig. 3 . There was not any publication bias. It shown as Fig. 4 .
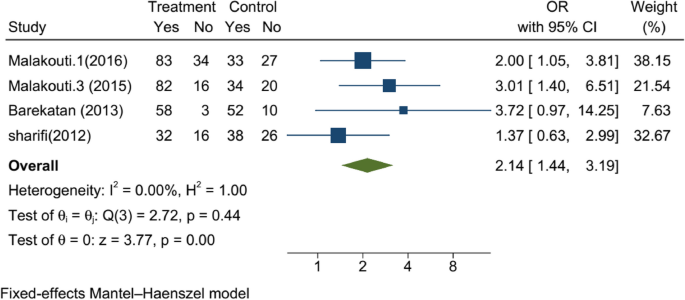
The result of the meta-analysis of rehospitalization among four studies
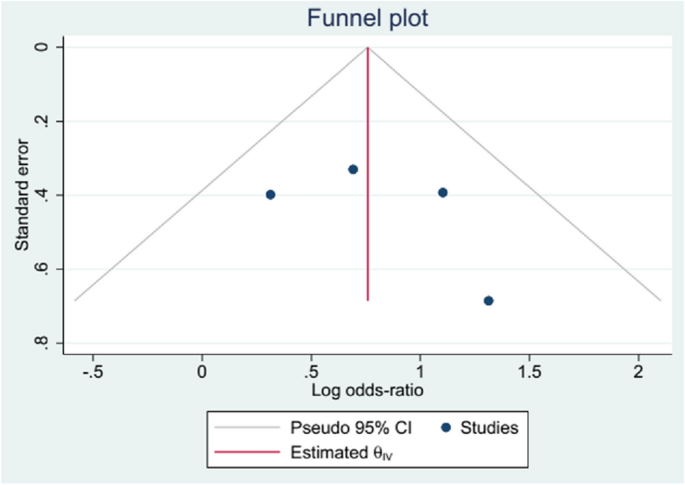
Funnel plot, publication bias for rehospitalization in studies
Severity of psychopathology
Out of 17 studies, 5 used PANSS to evaluate the effect of intervention on psychopathology, whose data were amenable to analysis (with a total of 669 participants in both intervention and control groups). Meta-analysis shows that after 12 months of intervention, CBMHS are successful in reducing significantly of the severity of psychopathology (SMD: -0.31, 95%CI: -0.49 to -0.13, I 2 = 40.23%). Akbari [ 18 ] was the only one that used ANSQ -Anderson Negative Symptoms- (SMD: -0.581, 95%CI: -1.312, 0.149). The meta-analysis of the studies for PANSS showed in Fig. 5 .
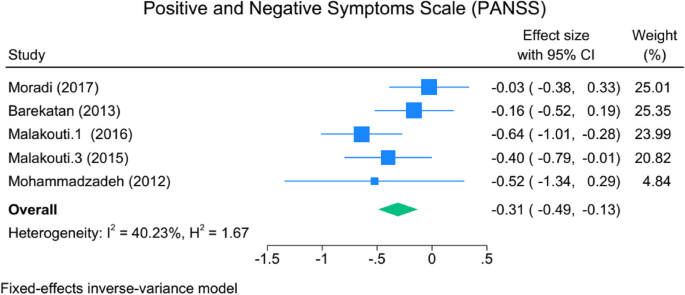
The meta-analysis of the studies for PANSS
The result of meta-analysis on the YOUNG (evaluating the severity of Bipolar mood disorder) shows significant difference by implementing the intervention (SMD: -0.764, 95% CI: -1.274, -0.253, I 2 = 85.22%), however the heterogeneity among four studies were not acceptable.
Upon visual examination of the funnel plot, no significant signs of asymmetry were observed (located in the Supplementary Material 1 : Appendix).
Social skills as secondary outcomes of the study could be considered as one of the outcomes of any intervention that aims to help the patient to be as independent as possible in the family and society. Just two studies used KELS to evaluate the social skills of the patients. The result of KELS shows a high effect size (SMD: -0.7, 95%CI: -0.98 to -0.44, I2 = 0.00%), and the community-based interventions are more promising. The same result was revealed by the study of Shahmiri (2014) by Matson evaluation of social skills (MESS) (SMD: -0.877, 95%CI: -1.749, 0.041) (lower scores indicating better functioning).
The tools of ACIS Assessment of Communication and Interaction Skills (mean difference 1.747 (CI: 1.08, 2.41).) Higher scores indicate better skills, such as DSK Dehbozorgi's social skills (SMD: 0.835, 95%CI: 0.088, 1.581). (Lower scores indicate lower social skills).
The burden of the caregivers was evaluated by FEIS (evaluating the burden of the caregivers) in the meta-analysis (not shown in the article). It shows that again in the two articles, the effect size was -0.55 (SMD: -0.55, 95%CI: -0.99, -0.1, I2 = 63.2) (in favor of community-based services.
For the CSQ, which evaluated the satisfaction of the clients from the services, there were not any significant differences with the control group. In the study of Sharifi [ 14 ], the quality of life of (WHOQOL) patients has been improved marginally (SMD:-0.246, 95%CI: -0.500, 0.007) ( P = 0.057). However, in the study of Hojati- Abad [ 27 ] WQOLCQ (Wisconsin Quality of Life Client Questionnaire (SMD: 0.798, 95%CI: 0.29, 5, 1.301), there was not any significant difference.
Economic evaluation studies
For economic evaluation we considered tow indexes reported incremental cost-effectiveness ratios (ICER) [ 28 ] and Quality-Adjusted Life Year (QUALY) [ 29 , 30 ], which is are more common indexes economic evaluation.
The QALY serves as a metric for assessing the worth of health outcomes. As health is contingent upon both lifespan and well-being, the QALY was formulated as an endeavor to amalgamate the value of these attributes into a solitary index. In the field of mental health, improving the quality of life for patients and reducing the burden of the disease not only for the patients themselves but also for their families and society as a whole. QALYs can be integrated with medical expenses to derive a final universal measure of cost/QALY. This parameter facilitates the comparison of the cost-effectiveness of various treatments without bias.
In numerous healthcare systems, determinations regarding the reimbursement and availability of new medications hinge upon health technology assessments. These assessments, involve the evaluation of an ICER. Decision-makers then weigh the ICER against a predetermined benchmark for cost-effectiveness, referred to as the cost-effectiveness threshold (CET), in order to ascertain whether reimbursement should be granted or withheld [ 28 ].
We identified two reports that met our inclusion criteria concerning economic evaluations of community-based interventions to improve the mental health of individuals with SMI [ 13 , 21 ]. In both studies were included QUALY and ICER.
Malakouti et al. ICER for aftercare home services following the discharge of individuals with SMI. Their analysis was based on a 12-month follow-up of participants in a clinical trial conducted between 2007 and 2008. They found that the ICER was 5.7 million Rials (IRR) per QALY when using general practitioners (GPs) as care providers during home visits and 5.0 million IRR per QALY when replacing GPs with nurses [ 13 ]. In a separate study, Moradi-Lakeh et al. conducted a cost-utility analysis of aftercare services following the discharge of individuals hospitalized for SMI. Their analysis was based on a clinical trial performed from 2012 to 2014, with a 20-month follow-up. They reported an ICER of US$8,399 (95% CI: 8,178–8,620) per QALY for the intervention [ 13 ]. It is worth noting that the services provided by the second study were more comprehensive. In these two studies showed that community-based interventions can be useful in terms of cost–benefit and cost-effectiveness.
While the ICER measures in these two studies differed considerably (partly due to a significant fluctuation in IRR-USD exchange rates $1 = 935 to 10,402 IRR, and other services provided by the second study included the expenses of general psychologists, supervising psychiatrists, as well as the costs of weekly co-ordination meetings of home visit teams, costs of classes for caregivers' education, training of social skills), both were found to be below the World Health Organization's recommended threshold for cost-effectiveness of health interventions [ 13 , 21 ]. The second study aimed to provide the cheapest and most effective intervention and evaluated the feasibility of providing such CBMHS. However, from $ 613 to $8400, it is feasible to provide such services while considering the exchange and inflation rates.
Out of 127 English and Persian language articles which were conducted in IRAN, seventeen met the eligibility criteria for inclusion in the systematic review and meta-analysis. community-based services effectively reduced rehospitalizations by 2times. reduction in psychopathology with moderate effect sizes. economic cost-effectiveness, with ICER values falling below the recommendations set by the WHO s.
Community psychiatry was launched in the USA in 1970 [ 31 ], community psychiatry was launched in the USA in 1970 [ 31 ] Reducing hospitalization, enabling individuals to have an active social life in society [ 32 ], and providing holistic care are among the main goals of community psychiatry community psychiatry was launched in the USA in 1970 [ 31 ].
CBMHS, including home visits, telephone follow-ups, case management systems, intensive care systems, and other CBMHS developed in different societies, have emerged and expanded to address this new challenge [ 32 ]. Even in a crisis, CBMHS mobile crisis intervention can reduce the number of readmissions [ 33 ]. Providing and connecting discharged patients to community services as soon as possible is a critical issue to prevent readmission [ 34 ].
On the other hand, the evidence shows that the direct daily costs for community-based social psychiatric care were about half the costs of inpatient treatment over the entire period [ 34 , 35 ]. However, reducing the number of readmissions depends on the intensity of community services, and it may yield different results in some societies [ 36 ].
Some reasons may be nominated for reduction of rehospitalization [ 37 , 38 ]. However, compared with the developed societies, we need to examine this issue from a dual perspective. First, in addition to having social activities and supporting the patients to have an almost independent life, second, the shortage of psychiatric beds is an essential matter in our country's mental health services. According to the according recommended assesment, we should have forty more than 56 thousand psychiatric beds in Iran [ 39 , 40 ] which falls behindrrrr. With the best estimation, we have thirty thousand psychiatric beds, of which fifty percent belong to the Welfare Organization for long-term hospitalization and rehabilitation [ 40 ]. Therefore, an extension of CBMHS psychiatry could help the patient and their family but also help the national mental health service to compensate for the shortage of facilities. Exacerbating the severity of psychopathology could increase the odd behaviors and tension in the family, leading to the patient's hospitalization [ 41 , 42 ]. Reducing the severity of psychopathology, as revealed in the study results as the severity of PANSS and YMRS in the current study, could be helpful. Increasing adherence to medication due to frequent contact (in person or by telephone) encourages the patient to comply with the treatment, which is the main reason for reducing the severity of psychopathology.
Having a long-duration mental health stability state without exacerbation of the symptoms, in addition to psychoeducation and behavioral rehearsal, which is part of community-based intervention, could provide suitable opportunities for the patient to retrieve his/her behavioral skills or learn new skills, which are necessary for independent living [ 43 , 44 ]. Social skills, evaluated by KELS and MESS, showed promising outcomes for CBMHS with remarkable effect size. However, gaining social skills requires intense and close supervision, which any community-based intervention may not obtain, as shown by the study of Jamshidi [ 25 ]; the results of this study are controversial ACIS.
The burden and mental health of the caregivers for chronic diseases such as schizophrenia, bipolar mood disorder, and dementia are essential in mental health services. Given that in developing countries [ 45 , 46 ], taking care of patients is on the shoulders of the families, providing aftercare services and regular contact with the patient and the caregivers could help them overcome the long-lasting problems in their family. The reduction of burden and improvement of the mental health condition of the caregivers show that such services have a positive impact on them [ 44 ].
Satisfaction with the services and quality of life, which did not exhibit significant differences from the control group in our study, remains a topic of extensive debate. Satisfaction and quality of life depend on physical and mental health and suitable living conditions such as housing, jobs, and income, which are not fulfilled by community-based services and are beyond the scope of these services and require intersectoral collaboration [ 12 , 21 , 38 ].
The challenge of the studies performed in Iran as CBMHS was using multiple tools with similar goals to evaluate corresponding variables. This hinders the ability to compare and analyze the results effectively. For instance, the study employed four different tools to measure life skills. Also, despite the history of CBMHS in Iran, there is a lower publication rate for the results of these interventions and programs. The expectation of having more articles that evaluate this specific area emphasizes the need for attention to be given to this issue.
The findings of community-based studies in Iran, although rare, showed that in line with international studies, this type of service is compatible and effective in Iran's economic, social, and cultural conditions. Even though there are challenges regarding patient satisfaction and organizational interdepartmental cooperation. These findings emphasize that community-based services not only in Iran but also in similar countries should be preferred over long-term inpatient services.
Availability of data and materials
This is an evidence synthesis study, all data is available from the primary research studies, or can be circulated from the corresponding author.
Singh OP. Comprehensive mental health action plan 2013–2030: we must rise to the challenge. Indian J Psychiatry. 2021;63:5415–7.
Article Google Scholar
Ojha SP, Pant SB. Community based rehabilitation for task shifting in mental health. J Psychosoc Rehabil Ment Health. 2018;5:109–10.
Keegan J. Community-based mental health care: bridging the gap between community care and primary care. Aust N Z J Ment Health Nurs. 1998;7(3):95–102.
CAS PubMed Google Scholar
Arias D, Saxena S, Verguet S. Quantifying the global burden of mental disorders and their economic value. EClinicalMedicine. 2022;54.
Sharifi V, Amin-Esmaeili M, Hajebi A, Motevalian A, Radgoodarzi R, Hefazi M, et al. Twelve-month prevalence and correlates of psychiatric disorders in Iran: the Iranian Mental Health Survey, 2011. Arch Iran Med. 2015;18(2):76–84.
PubMed Google Scholar
Forouzanfar MH, Sepanlou SG, Shahraz S, Dicker D, Naghavi P, Pourmalek F, et al. Evaluating causes of death and morbidity in Iran, global burden of diseases, injuries, and risk factors study 2010. Arch Iran Med. 2014;17(5):304–20.
Rathod S, Pinninti N, Irfan M, Gorczynski P, Rathod P, Gega L, et al. Mental health service provision in low-and middle-income countries. Health Serv Insights. 2017;10:1178632917694350.
PubMed PubMed Central Google Scholar
Sharifi V, Abolhasani F, Farhoudian A, Amin-Esmaeili M. Community mental health centers in Iran: planning evidence-based services. Iran J Psychiatry Clin Psychol. 2014;19(3):163–76.
Google Scholar
Malakouti SK, Nojomi M, Panaghi L, Chimeh N, Mottaghipour Y, Joghatai MT, et al. Case-management for patients with schizophrenia in Iran: a comparative study of the clinical outcomes of mental health workers and consumers’ family members as case managers. Community Ment Health J. 2009;45:447–52.
Article PubMed Google Scholar
Andrade C. Mean difference, Standardized Mean Difference (SMD), and their use in meta-analysis: as simple as it gets. J Clin Psychiatry. 2020 2020/09//; 81(5):[20f13681 p.]. Available from: http://europepmc.org/abstract/MED/32965803. https://www.psychiatrist.com/read-pdf/11349 . https://doi.org/10.4088/JCP.20f13681 .
Barekatain M, Maracy MR, Rajabi F, Baratian H. Aftercare services for patients with severe mental disorder: a randomized controlled trial. J Res Med Sci. 2014;19(3):240–5.
Malakouti SK, Nojomi M, Mirabzadeh A, Mottaghipour Y, Zahiroddin A, Kangrani HM. A comparative study of nurses as case manager and telephone follow-up on clinical outcomes of patients with severe mental illness. Iran J Med Sci. 2016;41(1):19–27.
Moradi-Lakeh M, Yaghoubi M, Hajebi A, Malakouti SK, Vasfi MG. Cost-effectiveness of aftercare services for people with severe mental disorders: an analysis parallel to a randomised controlled clinical trial in Iran. Health Soc Care Community. 2017;25(3):1151–9.
Sharifi V, Tehranidoost M, Yunesian M, Amini H, Mohammadi M, Jalali RM. Effectiveness of a low-intensity home-based aftercare for patients with severe mental disorders: a 12-month randomized controlled study. Community Ment Health J. 2012;48(6):766–70.
Babokani ZS, Ghazanfari A, Ahmadhi R, Chorami M. The comparison of the effectiveness of behavioral activation and psychosocial on mental cognitive functions in women with chronic schizophrenia. Q J Psychol Stud. 2020;15(4):91–108.
Malakouti SK, Chimeh N, Panaghi L, AhmadAbadi Z, Nojomi M. The effectiness of two case management methods on mental health, knowledge, and burden of schizophernics. Family Member Fam Res. 2009;5(17):29–42.
Mohebi P, Fallahi-Khoshknab M, Rahgoi A, Tabrizi K, Khodaei-Ardakani M-R. Effect of the community re-entry program on medication adherence in patients with bipolar disorder type I. Iran J Rehabil Res Nurs. 2017;3:58–65.
Akbari M, Saeidi S. The effectiveness of psychosocial rehabilitation in reducing negative symptoms and improving social skills of chronic schizophrenia patients. Shenakht J Psychol Psychiatry. 2017;4(3):21–35.
Fayyazi Bordbar MR, Soltanifar A, Talaei A. Short-term family-focused psycho-educational program for bipolar mood disorder in Mashhad. Iran J Med Sci. 2009;34.
Ahmadi A, Farahbakhsh K, Moatamedy A, Khodaei M, Safi MH. The effectiveness of family psychological training on prevention of recurrence of symptoms in patients with schizophrenia spectrum disorders. Iran J Psychiatr Nurs. 2020;8(3):93–103.
Malakouti SK, Mirabzadeh A, Nojomi M, Ahmadi Tonkaboni A, Nadarkhani F, Mirzaie M, et al. Clinical outcomes and cost effectiveness of two aftercare models provided by general physicians and nurses to patients with severe mental illness. Med J Islam Repub Iran. 2015;29:196.
Shahmir E, Talebizadeh M, Jafarifard S. The effectiveness of the returning to society program in improving the social skills of schizophrenic patients. Thoughts Behav Clin Psychol. 2014;9(31):27–36.
Mohammadzadeh Nanehkaran S, Karbalaie Noori A, Hosseini SA. Effect of Community Re-entry Program (CRM) in patients with schizophrenia. Arch Rehabil. 2013;14(2):22–8.
Fallahi-Khoshknab M. Effectiveness of Psychiatric Nursing Home Care Services for Psychiatric Status in Schizophrenics. Arch Rehabil. 2007;8:77–81.
Jamshidi F, Shayan A, Forouhari S, Taherkhani H, Seyedi M. The effect of community Re-entry program (CRP) on social function of patients with chronic mental disorders. Acta Med Mediterr. 2016;32(Specia):1311–6.
Dashtbozorgi B, Ghadirian F, Khajeddin N, Karatni K. Effect of family psychoeducation on the level of adaptation and improvement of patients with mood disorders. Iran J Psychiatry Clin Psychol. 2009;15(2):193–200.
Hojjati-Abed E, Karbalaaei-nouri A, Rafiei H, Karimlou M. The efficacy of psychosocial occupational therapy services on quality of life of chronic pschiatric patents. Arch Rehabil. 2010;11(1):0.
Berdud M, Ferraro J, Towse A. A theory on ICER pricing and optimal levels of cost-effectiveness thresholds: a bargaining approach. Front Health Serv. 2023;3:1055471.
Article PubMed PubMed Central Google Scholar
Prieto L, Sacristán JA. Problems and solutions in calculating quality-adjusted life years (QALYs). Health Qual Life Outcomes. 2003;1:80.
Rand LZ, Kesselheim AS. Controversy over using quality-adjusted life-years in cost-effectiveness analyses: a systematic literature Review. Health Aff (Millwood). 2021;40(9):1402–10.
Dean C, Phillips J, Gadd E, Joseph M, England S. Comparison of community based service with hospital based service for people with acute, severe psychiatric illness. Br Med J. 1993;307(6902):473–6.
Article CAS Google Scholar
Kisely S, Yu D, Maehashi S, Siskind D. A systematic review and meta-analysis of predictors and outcomes of community treatment orders in Australia and New Zealand. Aust N Z J Psychiatry. 2021;55(7):650–65.
Guo S, Biegel DE, Johnsen JA, Dyches H. Assessing the impact of community-based mobile crisis services on preventing hospitalization. Psychiatr Serv. 2001;52(2):223–8.
Article CAS PubMed Google Scholar
Haywood TW, Kravitz HM, Grossman LS, Cavanaugh JL, Davis JM, Lewis DA. Predicting the" revolving door" phenomenon among patients with schizophrenic, schizoaffective, and affective disorders. Am J Psychiatry. 1995.
Bachrach LL. Deinstitutionalisation: promises, problems and prospects. Ment Health Serv Eval. 1996:3–18.
Sytema S, Burgess P, Tansella M. Does community care decrease length of stay and risk of rehospitalization in new patients with schizophrenia disorders? A comparative case register study in Groningen, The Netherlands; Victoria, Australia; and South-Verona. Italy Schizophr Bull. 2002;28(2):273–81.
Joynt KE, Sarma N, Epstein AM, Jha AK, Weissman JS. Challenges in reducing readmissions: lessons from leadership and frontline personnel at eight minority-serving hospitals. Jt Comm J Qual Patient Saf. 2014;40(10):435–7.
Phillips MS, Steelesmith DL, Campo JV, Pradhan T, Fontanella CA. Factors associated with multiple psychiatric readmissions for youth with mood disorders. J Am Acad Child Adolesc Psychiatry. 2020;59(5):619–31.
Allison S, Bastiampillai T, Licinio J, Fuller DA, Bidargaddi N, Sharfstein SS. When should governments increase the supply of psychiatric beds? Mol Psychiatry. 2018;23(4):796–800.
Malakouti SK, Keshavarz Akhlaghi A, Shirzad F, Rashedi V, Khlafbeigi M, Moradi Lakeh M, et al. Urban mental health initiative: developing interdisciplinary collaboration, the role of the family physician in management of severe mentally ill patients. Iran J Psychiatry Clin Psychol. 2021;27(1):104–19.
Hinojosa-Marqués L, Domínguez-Martínez T, Barrantes-Vidal N. Family environmental factors in at-risk mental states for psychosis. Clini Psychol Psychother. 2022;29(2):424–54.
Kuhney FS, Miklowitz DJ, Schiffman J, Mittal VA. Family-based psychosocial interventions for severe mental illness: social barriers and policy implications. Policy Insights Behav Brain Sci. 2023;10(1):59–67.
de Pablo GS, De Micheli A, Nieman DH, Correll CU, Kessing LV, Pfennig A, et al. Universal and selective interventions to promote good mental health in young people: systematic review and meta-analysis. Eur Neuropsychopharmacol. 2020;41:28–39.
Estrada-Fernández ME, Gil-Lacruz M, Gil-Lacruz AI, Viñas-López A. The impact of community care: burden and quality of life of the informal caregivers of patients with severe mental disorder. J Community Psychol. 2022;50(1):487–501.
Ofovwe CE, Osasona SO. Burden of caregiving among caregivers of patients with severe mental illnesses in Benin City. Nigeria Afr Health Sci. 2022;22(2):657–67.
Ahmadzadeh Asl M, Shojaee A, Shariati B, Rasoolian M, Rashedi V. A comparative study on the burden of disease of schizophrenia, bipolar disorder type I, and autism spectrum disorder on the family caregivers in Iran. Arch Rehabil. 2021;22(3):320–41.
Download references
Acknowledgements
Not applicable.
The current study has been financially supported by the Iran University of Medical Sciences (Registration number: IR.IUMS.REC. 1400–1-99–21128). The funders had no role in the study implementation, data collection and analysis, or interpretation of study results.
Author information
Authors and affiliations.
Mental Health Research Center, Psychosocial Health Research Institute, Iran University of Medical Sciences, Tehran, Iran
Mozhgan Taban
Department of Psychiatry and Psychology, Mayo Clinic, Rochester, MN, USA
Sara Nooraeen
Oxford Vaccine Group, Department of Pediatrics, University of Oxford, Oxford, U.K.
Kiarash Tanha
Gastrointestinal and Liver Disease Research Center (GILDRC), Iran University of Medical Sciences, Tehran, Iran
Maziar Moradi-Lakeh
Geriatric Mental Health Research Center, School of Behavioral Sciences and Mental Health, Iran University of Medical Sciences, Tehran, Iran
Seyed Kazem Malakouti
You can also search for this author in PubMed Google Scholar
Contributions
Conceived the project (SKM); Developed the protocol (SKM, SN, KT, MT); Carried out the searches (SN, MT, KT), carried out the synthesis (MT, SN), Interpreted the findings (SKM, MT, KT), drafted the manuscript (SKM, MT), approved the manuscript (SKM). SKM is the guarantor of this review. All authors have read and approved the manuscript.
Corresponding author
Correspondence to Seyed Kazem Malakouti .
Ethics declarations
Ethics approval and consent to participate.
The study protocol had been approved by the Ethics Committee of the Iran University of Medical Sciences (code: IR.IUMS.REC.1400.733) and about consent and participate: Not applicable.
Consent for publication
Competing interests.
The authors declare no competing interests.
Additional information
Publisher’s note.
Springer Nature remains neutral with regard to jurisdictional claims in published maps and institutional affiliations.
Supplementary Information
Supplementary material 1., rights and permissions.
Open Access This article is licensed under a Creative Commons Attribution 4.0 International License, which permits use, sharing, adaptation, distribution and reproduction in any medium or format, as long as you give appropriate credit to the original author(s) and the source, provide a link to the Creative Commons licence, and indicate if changes were made. The images or other third party material in this article are included in the article's Creative Commons licence, unless indicated otherwise in a credit line to the material. If material is not included in the article's Creative Commons licence and your intended use is not permitted by statutory regulation or exceeds the permitted use, you will need to obtain permission directly from the copyright holder. To view a copy of this licence, visit http://creativecommons.org/licenses/by/4.0/ . The Creative Commons Public Domain Dedication waiver ( http://creativecommons.org/publicdomain/zero/1.0/ ) applies to the data made available in this article, unless otherwise stated in a credit line to the data.
Reprints and permissions
About this article
Cite this article.
Taban, M., Nooraeen, S., Tanha, K. et al. Effectiveness and cost-effectiveness of community-based mental health services for individuals with severe mental illness in Iran: a systematic review and meta-analysis. BMC Psychiatry 24 , 256 (2024). https://doi.org/10.1186/s12888-024-05666-7
Download citation
Received : 10 November 2023
Accepted : 07 March 2024
Published : 04 April 2024
DOI : https://doi.org/10.1186/s12888-024-05666-7
Share this article
Anyone you share the following link with will be able to read this content:
Sorry, a shareable link is not currently available for this article.
Provided by the Springer Nature SharedIt content-sharing initiative
- Effectiveness
- Cost-effectiveness
- Community-based mental health services
- Severe mental illness
BMC Psychiatry
ISSN: 1471-244X
- Submission enquiries: [email protected]
- General enquiries: [email protected]
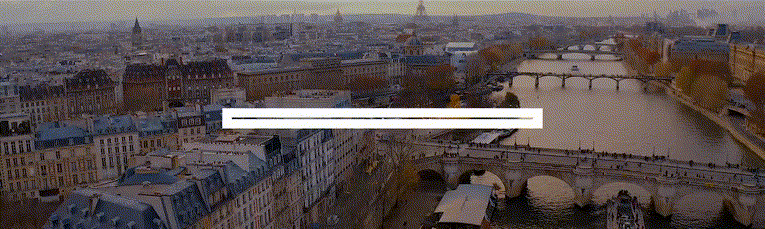
IMAGES
VIDEO
COMMENTS
taxes). 2 If gas mileage is 25 miles to the gallon—at an assumed average speed of 50 miles per. hour —and if the individual lives 50 miles away from the recreational site, the cost of the ...
The value of travel time savings (VTTS) is an important concept used in travel demand modelling and cost-benefit analysis of transport projects ( Mackie et al., 2001; Gunn and Sillaparcharn, 2007 ). It plays a key role in policy and investment decision-making, since time savings can account for up to 80% of the estimated benefits of road ...
Values of travel time savings are often used in cost-benefit analysis of transport projects and policies, and also to compute generalised travel costs. ... Meta-analysis and the value of travel time savings: A transatlantic perspective on passengers' transport. Networks and Spatial Economics, 7 (4) (2007), pp. 377-396.
This paper aims to evaluate the touristic value in the area by applying the travel cost method, a non-market evaluation method used to derive consumers' preferences. ... The economic value of wetland services: A meta-analysis. Ecol. Econ. 2001, 37, 257-270. [Google Scholar] Schuyt, K.; e Brander, L. The Economic Values of the World's ...
The meta-analysis sheds some new light on the variation of the value of travel time savings by income, country, travel purpose, mode, distance and by survey method. Furthermore, the resulting meta-models are applied to produce new values of travel time savings for business travel, commuting and for other purposes in passenger transport, for 25 ...
The primary purpose of the meta-analysis is to enable general conclusions to be drawn from the major published empirical studies regarding the relationships between international tourism demand and income, transportation costs, and tourism prices. ... "A Statistical Analysis of the Demand for Visits to U.S. National Parks: Travel Costs and ...
The meta-analysis sheds some new light on the variation of the value of travel time savings by income, country, travel purpose, mode, distance and by survey method. ... in which one of the explanatory variables is a linear combination of travel time and cost, called 'generalised cost'. Different methods can be used to derive the VTTS (see ...
The value of freight travel time savings (VFTTS) is a monetary value that is considered an important input into cost-benefit analysis and traffic forecasting. The VFTTS is defined as the marginal rate of substitution between travel time and cost and may therefore differ across firms, time and countries. The paper aims to explain variations in the VFTTS by using the meta-analysis method. The ...
willingness-to-pay for related goods (e.g. SSD or hedonics). The travel cost method is another. indirect measure that is useful in certain circumstances, but which has flaws from both an. economist's and an environmentalist's perspective. The central theoretical flaw in the travel cost method, in common with SSD and.
an important input into cost-benet analysis and trac forecasting. The VFTTS is dened as the marginal rate of substitution between travel time and cost and may therefore dier across rms, time and countries. The paper aims to explain variations in the VFTTS by using the meta-analysis method. The analysis covers 106 monetary valuations extracted
The travel cost model determines site use by examining the time and travel expenses that people incur when visiting a recreation site. It is then assumed that these costs represent the ... (meta-analysis), from simulated markets, or as in this paper, from a different type of non-market
Step 5: The fifth step is to estimate, using regression analysis, the equation that relates visits per capita to travel costs and other important variables.From this, the researcher can estimate the demand function for the average visitor. In this simple model, the analysis might include demographic variables, such as age, income, gender, and education levels, using the average values for each ...
Gunn H (2001) Spatial and temporal transferability of relationships between travel demand, trip cost and travel time. Transp Res E 37:163-189. Article Google Scholar ... L., Reggiani, A. Meta-Analysis and the Value of Travel Time Savings: A Transatlantic Perspective in Passenger Transport. Netw Spat Econ 7, 377 -396 (2007 ...
This paper presents a comprehensive synthesis of coastal wetland valuations and identifies the important factors that determine the value of coastal wetlands through a meta-regression analysis of ...
Regarding the different approaches of the travel cost method, the individual approach provides more realistic estimates and has greater statistical support than the zonal approach; since it obtains the data directly from tourists through surveys, which allows estimating the real expenses they made during their trip.
In cost-benefit analysis, the impacts of a project are measured in money units. The travel time benefits are in the first instance measured in time units. Values of travel time are used to convert these into money units so that they can be included in the cost-benefit analysis alongside other monetised benefits and the financial costs.
TCM is used to value recreational uses of the environment. It is commonly applied in benefit cost analyses (BCA) and in natural resource damage assessments. It is based on „observed behaviour‟, thus is used to estimate use values only. TCM is a demand-based model for use of a recreation site or sites. A site: a river for fishing, a trail ...
Business travel demand is determined by factors such as travel costs, relative price of complementary production input factors and a firm's output level. ... Since the objective of our meta-analysis basically consists of a comparative reevaluation of previous research on price elasticites for passenger air transport, we collected a number of 37 ...
The effects of such strategies on travel demand have not been generalized in recent years from the multitude of available studies. Purpose: We conducted a meta-analysis of the built environment-travel literature existing at the end of 2009 in order to draw generalizable conclusions for practice. We aimed to quantify effect sizes, update earlier ...
The travel cost method of economic valuation, travel cost analysis, or Clawson method is a revealed preference method of economic valuation used in cost-benefit analysis to calculate the value of something that cannot be obtained through market prices (i.e. national parks, beaches, ecosystems). The aim of the method is to calculate ...
The value of travel time is an important input to cost-benefit analysis of transport projects. Time gains are often one of the main benefits of transport projects, but they need to be converted from hours and minutes to money units, using a value of travel time. ... Wardman et al. (2016): European meta-analysis of passenger VTT (building on ...
Freight Transport and the Value of Travel Time Savings: A Meta‐analysis of Empirical Studies. September 2007; ... namely the trade-off between the cost and travel time (e.g. Wynter 1995 ...
The issues. Transport planners the world over make much use of generalised cost (GC) and/or generalised time (GT) which represent the overall attractiveness of a travel alternative in equivalent units of money or travel time. This is because such composite terms have a number of attractions at both the estimation and application stages.
Meta-analysis was carried out using STATA software. (PROSPERO registration. CRD42022332660). ... -0.55, 95% CI: -0.99 to -0.1, I2 = 63.2). The Incremental Cost-Effectiveness Ratio (ICER) for QUALY was acceptable, albeit with a wide range of 613 to 8400 Dollars. CBMHS has demonstrated effectiveness and efficiency in Iran as a developing country ...