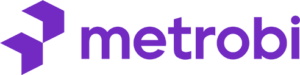
Learning center series
Travelling salesman problem explained
- Published on April 25, 2024
- by Oguzhan Uyar
- Last updated: 1 min ago
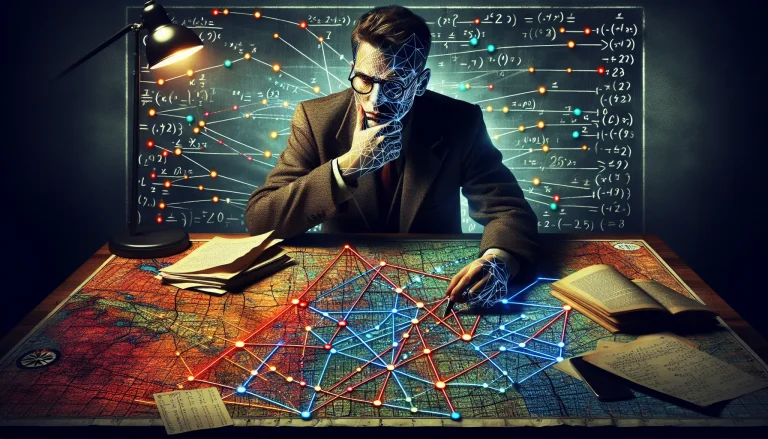
Revolutionize your understanding of the Travelling Salesman Problem (TSP); a mind-boggling conundrum that has compelled academia and industry alike. In the upcoming lines, we decode the key concepts, algorithms, and anticipated solutions for 2024 to this age-old dilemma.
Now, picture the TSP as a globetrotting traveling salesman who’s whirlwind journey. He must stop at every city once, only the origin city once, and find the quickest shortest possible route back home. If daunting to visualize, consider this: the possible number of routes in the problem concerning just 20 cities exceeds the number of atoms in the observable universe.
Fathom the sheer magnitude?
So, what is the Travelling Salesman Problem, and why has it remained unsolved for years? Let’s snap together the puzzle of this notorious problem that spans mathematics, computer science, and beyond. Welcome to an insightful voyage into the astonishing world of the TSP.
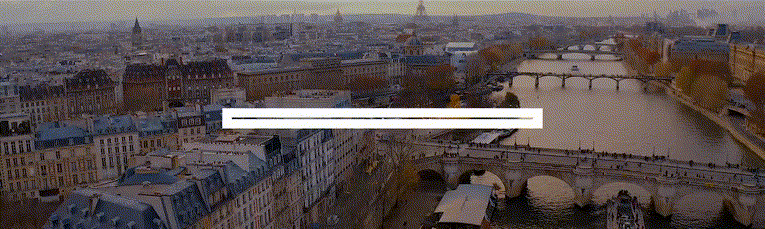
Understanding the Travelling Salesman Problem (TSP): Key Concepts and Algorithms
Defining the travelling salesman problem: a comprehensive overview.
The Travelling Salesman Problem, often abbreviated as TSP, has a strong footing in the realm of computer science. To the untrained eye, it presents itself as a puzzle: a salesperson or traveling salesman must traverse through a number of specific cities before an ending point and return to their ending point as of origin, managing to do so in the shortest possible distance. But this problem is not simply a conundrum for those fond of riddles; it holds immense significance in the broad field of computer science and optimization.
The sheer computational complexity of TSP is what sets it apart, and incidentally, why it is considered a challenging problem to solve. Its complexity derives from the factorial nature of the problem: whenever a new city is added, the total number of possibilities increases exponentially. Thus, as the problem scope enlarges, it swiftly becomes computationally prohibitive to simply calculate all possible solutions to identify an optimal shortest route through. Consequently, developing effective and efficient algorithms to solve the TSP has become a priority in the world of computational complexity.
Polynomial Time Solution: The TSP can be solved by a deterministic Turing machine in polynomial time, which means that the number of steps to solve the problem can be at most 1.5 times the optimal global solution.
One such algorithm is the dynamic programming approach that can solve TSP problems in polynomial time. The approach uses a recursive formula to compute the shortest possible route that visits all other nodes in the cities exactly once and ends at all nodes in the starting point or city. Moreover, linear programming and approximation algorithms have also been used to find near-optimal solutions.
Unraveling TSP Algorithms: From Brute Force to Heuristics
A multitude of algorithms have been developed to contend with the TSP. The brute force method, for example, entails considering the shortest distance for all possible permutations of cities, calculating the total distance for six cities along each route, and selecting the shortest distance for one. While brute force promises an optimal solution, it suffers from exponential computational complexity, rendering it unfeasible for large datasets.
TSP Complexity: A TSP with just 10 cities has 362,880 possible routes , making brute force infeasible for larger instances.
On the other hand, we have heuristic algorithms that generate good, albeit non-optimal, solutions in reasonable timeframes. The greedy algorithm, for instance, initiates from a starting city and looks for the shortest distance from other nodes to the next node minimizes the distance, and guarantees speed but is not necessarily an optimal solution.
Algorithmic Potential: Local Solutions 4/3 Times Optimal Global: The theoretical conjecture suggests an algorithm that can provide a local solution within 4/3 times the optimal global solution.
Record Local TSP Solution: The record for a local solution to the Traveling Salesman Problem (TSP) is 1.4 times the optimal global solution, achieved in September 2012 by Andr´as Seb˝o and Jens Vygen.
Exploring further, we encounter more refined heuristic solutions such as the genetic algorithm and simulated annealing algorithm. These algorithms deploy probabilistic rules, with the former incorporating principles of natural evolution and the latter being inspired by the cooling process in metallurgy. They differ significantly from each other in terms of how they search for solutions, but when compared to brute force, they often offer a promising trade-off between quality and computational effort.
Certainly, the TSP is far more than a problem to puzzle over during a Sunday afternoon tea. It’s a complex computational task with real-world implications, and unraveling it requires more than brute force; it demands the application of sophisticated algorithms designed to balance efficiency and quality of results. Nevertheless, with a better understanding of the problem statement and its dynamics, you can unlock the potential to not just solve problems faster, but to do so more intelligently.
Practical Solutions to the Travelling Salesman Problem
Implementing tsp solutions: a step-by-step guide, a comprehensive process for implementing tsp solutions.
Developing complete, practical solutions for the Travelling Salesman Problem (TSP) requires a good grasp of specific algorithms. Visual aids, for example, such as finding all the edges leading out of a given city, can help to simplify the process.
TSP's Computer Science Quest for Shortest Routes: The TSP involves finding the shortest route to visit a set of cities exactly once before returning to the starting city, aiming to minimize travel distance.
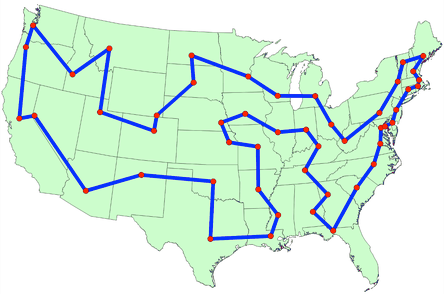
One popular approach to TSP problem solving is the dynamic programming approach, which involves breaking down the problem into smaller sub-problems and recursively solving them. Other approaches include approximation algorithms, which generate near-optimal solutions to small problems in polynomial time, and bound algorithms, which aim to find an upper bound on the optimal solution given graph top.
TSP Variants: ASTP and STSP The TSP can be divided into two types: the asymmetric traveling salesman problem (ASTP) and the symmetric traveling salesman problem (STSP)
Practical code implementation
Before diving into code manipulations, it’s worth noting that practical implementation varies based on the specifics of the problem and the chosen algorithm. Let’s further deconstruct these notions by examining the steps for code implementation for a pre-determined shortest path first. It’s crucial to efficiently concede each point of the shortest path, call other nodes, connect each node, and conclude each route. Hereby, I shall delve into the practicalities of coding from an output standpoint, concentrating on readability, scalability, and execution efficiency.
TSP Instance Size: The size of the TSP instances used in the studies is 100 nodes.
Cluster Quantity in TSP Instances: The number of clusters in the TSP instances used in the studies is 10 .
The Quantity of Instances per Cluster in Studies: The number of instances in each cluster used in the studies is 100.
Optimizing TSP Solutions: Tips and Tricks
Tsp solutions optimization techniques.
An optimized solution for the TSP is often accomplished using advanced algorithms and techniques. Optimization techniques allow professionals to solve more intricate problems, rendering them invaluable tools when handling large datasets and attempting to achieve the best possible solution. Several approaches can be taken, such as heuristic and metaheuristic approaches, that can provide near-optimal solutions with less use of resources.
Expert Advice for Maximum Efficiency Minimum Cost
Even the most proficient problem solvers can gain from learning expert tips and tricks. These nuggets of wisdom often provide the breakthrough needed to turn a good solution into a great one. The TSP is no exception. Peering into the realm of experts can provide novel and creative ways to save time, increase computation speed, manage datasets, and achieve the most efficient shortest route around.
By comprehending and implementing these solutions to the Travelling Salesman Problem, one can unravel the complex web of nodes and paths. This equips any problem-solver with invaluable insights that make navigating through real-world TSP applications much more manageable.
Real-World Applications of the Travelling Salesman Problem
Tsp in logistics and supply chain management.
The Travelling Salesman Problem (TSP) is not confined to theoretical mathematics or theoretical computer science either, it shines in real-world applications too. One of its most potent applications resides in the field of logistics and supply chain management.
With globalization, the importance of more efficient routes for logistics and supply chain operations has risen dramatically. Optimizing routes and decreasing costs hold the key to success in this arena. Here’s where TSP comes into play.
In the vast complexity of supply chain networks, routing can be a colossal task. TSP algorithms bring clarity to the chaos, eliminating redundant paths and pointing to the optimal route covering the most distance, shortest path, and least distance between all the necessary points—leading to a drastic dip in transportation costs and delivery times.
Real-World Examples
Consider the case of UPS – the multinational package delivery company. They’ve reportedly saved hundreds of millions of dollars yearly by implementing route optimization algorithms originating from the Travelling Salesman Problem. The solution, named ORION, helped UPS reduce the distance driven by their drivers roughly by 100 million miles annually.
TSP in GIS and Urban Planning: Optimal Route
TSP’s contributions to cities aren’t confined to logistics; it is invaluable in Geographic Information Systems (GIS) and urban planning as well. A city’s efficiency revolves around transportation. The better the transport networks and systems, the higher the city’s productivity. And as city authorities continuously strive to upgrade transportation systems, they find an ally in TSP.
Advanced GIS systems apply TSP to design more efficient routes and routing systems for public transportation. The aim of the route is to reach maximum locations with the least travel time and least distance, ensuring essential amenities are accessible to everyone in the city.
The city of Singapore, known for its efficient public transportation system, owes part of its success to similar routing algorithms. The Land Transport Authority uses TSP-related solutions to plan bus routes, reducing travel durations and enhancing accessibility across the city.
Delving Deeper: The Complexity and History of the Travelling Salesman Problem
Understanding the complexity of tsp.
TSP is fascinating not just for its inherent practicality but also for the complexity it brings along. TSP belongs to a class of problems known as NP-hard, a category that houses some of the most complex problems in computer science. But what makes TSP so gnarled?
Unravel the Intricacies: Why is TSP complex?
TSP is a combinatorial optimization problem, which simply means that it’s all about figuring out the most often optimal solution among a vast number of possible solutions or combinations of approximate solutions. The real challenge comes from an innocent-sounding feature of TSP: As the number of cities (we call them nodes) increases, the complexity heightens exponentially, not linearly. The number of possible routes takes a dramatic upward turn as you add more nodes, clearly exemplifying why TSP is no walk-in-the-park problem.
NP-hard and TSP: A Connection Explored
In computer science, we use the terms P and NP for classifying problems. P stands for problems where a solution can be found in ‘polynomial time’. NP stands for ‘nondeterministic polynomial time’, which includes problems where a solution can be verified in polynomial time. The concept of ‘NP-hardness’ applies to TSP. Any problem that can be ‘reduced’ to an NP problem in polynomial time is described as NP-hard. In simpler terms, it’s harder than the hardest problems of NP. TSP holds a famed position in this class, making it a noteworthy example of an NP-hard problem.
A Look Back: The History of the Travelling Salesman Problem
TSP is not a new kid on the block. Its roots can be traced back to the 1800s, and the journey since then has been nothing short of a compelling tale of mathematical and computational advancements.
The Origins and Evolution of TSP
Believe it or not, the Travelling Salesman Problem was first formulated as a mathematical problem in the 1800s. This was way before the advent of modern computers. Mathematicians and logisticians were intrigued by the problem and its implications. However, it wasn’t until the mid-20th century that the problem started to gain more widespread attention. Over the years, the TSP has transformed from a theoretical model to a practical problem that helps solve real-world issues.
A Classic Shortest Route Problem Since 1930s: The TSP is a classic optimization problem within the field of operations research, first studied during the 1930s.
Milestones in TSP Research
Looking at all the cities and milestones in TSP research, the story is truly impressive. From some of the initial heuristic algorithms to solve smaller instances of TSP, to geometric methods and then approximation algorithms, TSP research has seen a lot. More recently, we have also seen practical solutions to TSP using quantum computing — a testament to how far we’ve come. Each of these milestones signifies an innovative shift in how we understand and solve TSP.
Wrapping Up The Journey With Algorithms and Solutions
The Travelling Salesman Problem (TSP) remains a complex enigma of business logistics, yet, the advent of sophisticated algorithms and innovative solutions are paving avenues to optimize routing, reduce travel costs further, and enhance customer interactions.
Navigating the TSP intricacies is no longer a daunting challenge but a worthwhile investment in refining operational efficiency and delivering unparalleled customer experiences. With advanced toolsets and intelligent systems, businesses are closer to making more informed, strategic decisions.
Now, it’s your turn. Reflect on your current logistics complexities. How can your business benefit from implementing these key concepts and algorithms? Consider where you could incorporate these practices to streamline and revolutionize your daily operations.
Remember, in the world of dynamic business operations, mastering the TSP is not just an option, but a strategic imperative. As you move forward into 2024, embrace the art of solving the TSP. Unravel the potential, decipher the complexities, and unlock new horizons for your business.
Ready for the challenge?
What is route optimization?
7 reasons for delivery route optimization
What is multi-stop route planning and why is it important?
How to optimize routes with Google Maps
7 benefits of using route scheduling software
Truck route planning vs common route planning
Best delivery route planning software for 2024
‟Great service and support from Metrobi”
Anna’s Taqueria
‟I am able to do twice as many orders because I don't spend time delivering”
‟Flexibility and Accountability”
Benz’s Food Products Inc.
‟My products are handled professionally and I have access to a reliable delivery solution”
Quinlan-Wasserman
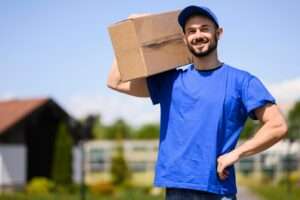
Success Stories
Rebel Bread
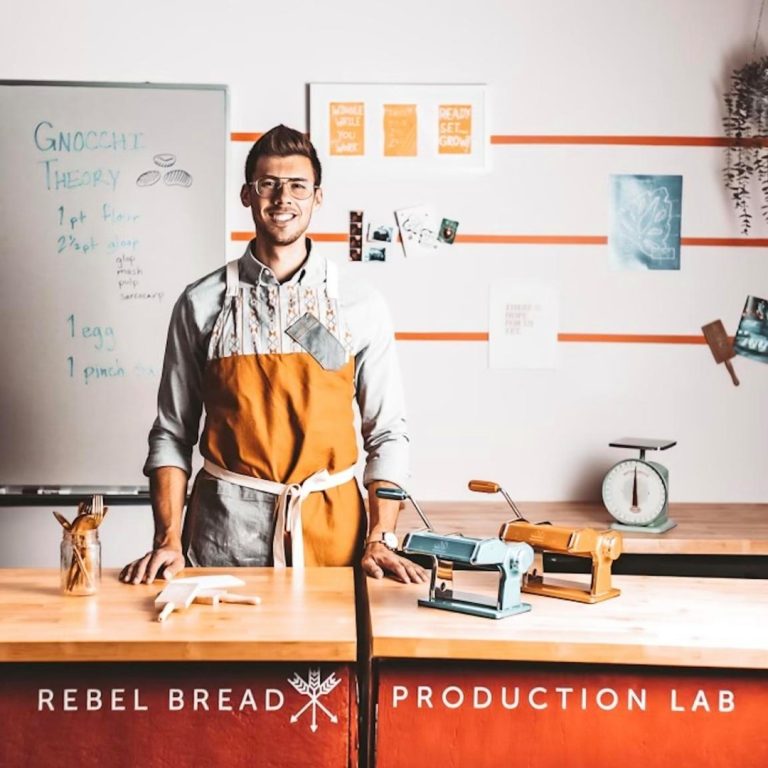
Flamingo Estate
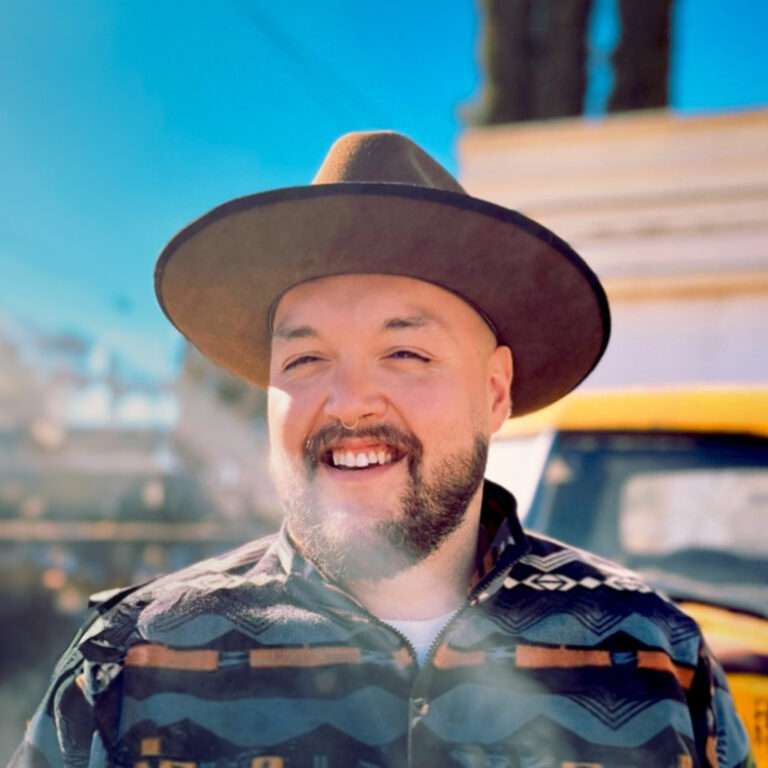
LuNo Culinary
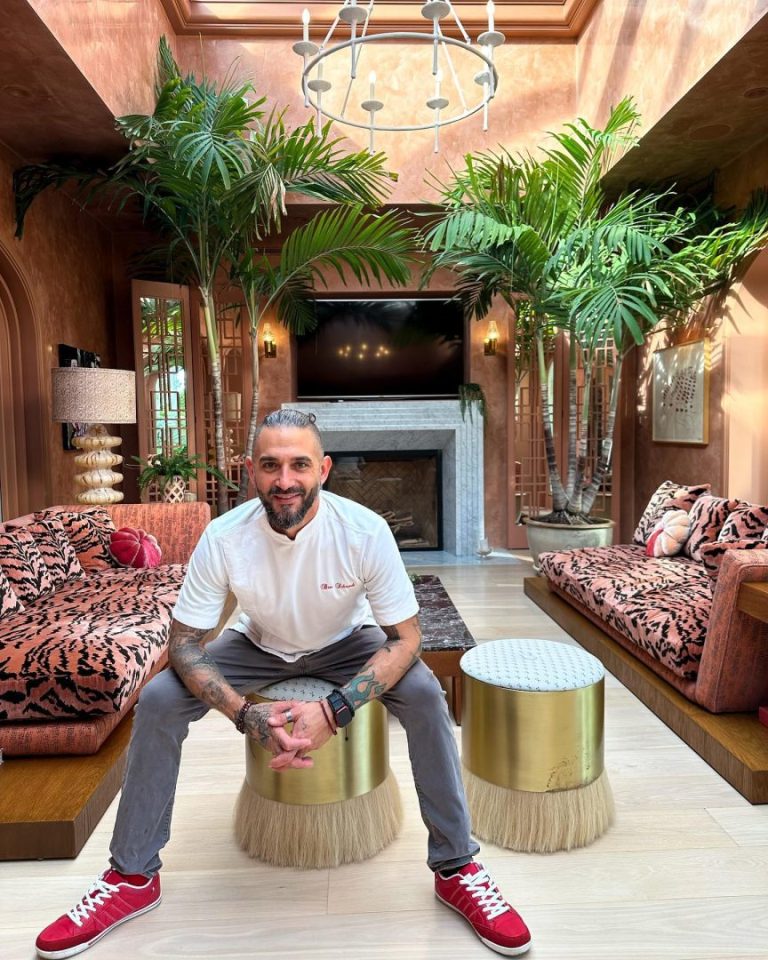
DELIVER WITH METROBI
Grow with confidence
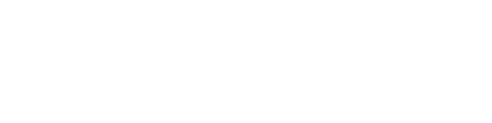
- 55 Court St floor 2, Boston, MA 02108
- [email protected]
- Team Metrobi
- Privacy policy
- Terms of service
- Write for us
Refer us to a company, you earn $250 and they earn $250. Learn more
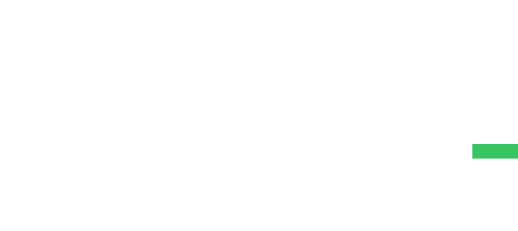
- Shopify Delivery Planner App
- Delivery Management Software
- Atlanta courier service
- Boston courier service
- Chicago courier service
- Denver courier service
- Miami courier service
- New York City courier service
- Los Angeles courier service
- Philadelphia courier service
- San Francisco courier service
- Washington DC courier service
- See all locations
- Bulk Order Delivery Service
- Express Urgent Delivery Service
- Fixed Route Delivery Service
- On Demand Delivery Service
- Overnight Delivery Service
- Same Day Delivery Service
- Scheduled Delivery Service
- Wholesale Delivery Service
- See all delivery services
- Metrobi vs. Onfleet
- Metrobi vs. Roadie
- Artisan Food
- Food Producers

Want to access our large pool of drivers?
We started Metrobi to take operations off your plate. We provide drivers (rated 4.97/5), dedicated operation managers (70% cheaper), and routing software with a receiver notification system.
DSA Tutorial
Linked lists, stacks & queues, hash tables, shortest path, minimum spanning tree, maximum flow, time complexity, dsa reference, dsa examples, dsa the traveling salesman problem.
The Traveling Salesman Problem
The Traveling Salesman Problem states that you are a salesperson and you must visit a number of cities or towns.
Rules : Visit every city only once, then return back to the city you started in.
Goal : Find the shortest possible route.
Except for the Held-Karp algorithm (which is quite advanced and time consuming, (\(O(2^n n^2)\)), and will not be described here), there is no other way to find the shortest route than to check all possible routes.
This means that the time complexity for solving this problem is \(O(n!)\), which means 720 routes needs to be checked for 6 cities, 40,320 routes must be checked for 8 cities, and if you have 10 cities to visit, more than 3.6 million routes must be checked!
Note: "!", or "factorial", is a mathematical operation used in combinatorics to find out how many possible ways something can be done. If there are 4 cities, each city is connected to every other city, and we must visit every city exactly once, there are \(4!= 4 \cdot 3 \cdot 2 \cdot 1 = 24\) different routes we can take to visit those cities.
The Traveling Salesman Problem (TSP) is a problem that is interesting to study because it is very practical, but so time consuming to solve, that it becomes nearly impossible to find the shortest route, even in a graph with just 20-30 vertices.
If we had an effective algorithm for solving The Traveling Salesman Problem, the consequences would be very big in many sectors, like for example chip design, vehicle routing, telecommunications, and urban planning.
Checking All Routes to Solve The Traveling Salesman Problem
To find the optimal solution to The Traveling Salesman Problem, we will check all possible routes, and every time we find a shorter route, we will store it, so that in the end we will have the shortest route.
Good: Finds the overall shortest route.
Bad: Requires an awful lot of calculation, especially for a large amount of cities, which means it is very time consuming.
How it works:
- Check the length of every possible route, one route at a time.
- Is the current route shorter than the shortest route found so far? If so, store the new shortest route.
- After checking all routes, the stored route is the shortest one.
Such a way of finding the solution to a problem is called brute force .
Brute force is not really an algorithm, it just means finding the solution by checking all possibilities, usually because of a lack of a better way to do it.
Speed: {{ inpVal }}
Finding the shortest route in The Traveling Salesman Problem by checking all routes (brute force).
Progress: {{progress}}%
n = {{vertices}} cities
{{vertices}}!={{posRoutes}} possible routes
Show every route: {{showCompares}}
The reason why the brute force approach of finding the shortest route (as shown above) is so time consuming is that we are checking all routes, and the number of possible routes increases really fast when the number of cities increases.
Finding the optimal solution to the Traveling Salesman Problem by checking all possible routes (brute force):
Using A Greedy Algorithm to Solve The Traveling Salesman Problem
Since checking every possible route to solve the Traveling Salesman Problem (like we did above) is so incredibly time consuming, we can instead find a short route by just going to the nearest unvisited city in each step, which is much faster.
Good: Finds a solution to the Traveling Salesman Problem much faster than by checking all routes.
Bad: Does not find the overall shortest route, it just finds a route that is much shorter than an average random route.
- Visit every city.
- The next city to visit is always the nearest of the unvisited cities from the city you are currently in.
- After visiting all cities, go back to the city you started in.
This way of finding an approximation to the shortest route in the Traveling Salesman Problem, by just going to the nearest unvisited city in each step, is called a greedy algorithm .
Finding an approximation to the shortest route in The Traveling Salesman Problem by always going to the nearest unvisited neighbor (greedy algorithm).
As you can see by running this simulation a few times, the routes that are found are not completely unreasonable. Except for a few times when the lines cross perhaps, especially towards the end of the algorithm, the resulting route is a lot shorter than we would get by choosing the next city at random.
Finding a near-optimal solution to the Traveling Salesman Problem using the nearest-neighbor algorithm (greedy):
Other Algorithms That Find Near-Optimal Solutions to The Traveling Salesman Problem
In addition to using a greedy algorithm to solve the Traveling Salesman Problem, there are also other algorithms that can find approximations to the shortest route.
These algorithms are popular because they are much more effective than to actually check all possible solutions, but as with the greedy algorithm above, they do not find the overall shortest route.
Algorithms used to find a near-optimal solution to the Traveling Salesman Problem include:
- 2-opt Heuristic: An algorithm that improves the solution step-by-step, in each step removing two edges and reconnecting the two paths in a different way to reduce the total path length.
- Genetic Algorithm: This is a type of algorithm inspired by the process of natural selection and use techniques such as selection, mutation, and crossover to evolve solutions to problems, including the TSP.
- Simulated Annealing: This method is inspired by the process of annealing in metallurgy. It involves heating and then slowly cooling a material to decrease defects. In the context of TSP, it's used to find a near-optimal solution by exploring the solution space in a way that allows for occasional moves to worse solutions, which helps to avoid getting stuck in local minima.
- Ant Colony Optimization: This algorithm is inspired by the behavior of ants in finding paths from the colony to food sources. It's a more complex probabilistic technique for solving computational problems which can be mapped to finding good paths through graphs.
Time Complexity for Solving The Traveling Salesman Problem
To get a near-optimal solution fast, we can use a greedy algorithm that just goes to the nearest unvisited city in each step, like in the second simulation on this page.
Solving The Traveling Salesman Problem in a greedy way like that, means that at each step, the distances from the current city to all other unvisited cities are compared, and that gives us a time complexity of \(O(n^2) \).
But finding the shortest route of them all requires a lot more operations, and the time complexity for that is \(O(n!)\), like mentioned earlier, which means that for 4 cities, there are 4! possible routes, which is the same as \(4 \cdot 3 \cdot 2 \cdot 1 = 24\). And for just 12 cities for example, there are \(12! = 12 \cdot 11 \cdot 10 \cdot \; ... \; \cdot 2 \cdot 1 = 479,001,600\) possible routes!
See the time complexity for the greedy algorithm \(O(n^2)\), versus the time complexity for finding the shortest route by comparing all routes \(O(n!)\), in the image below.

But there are two things we can do to reduce the number of routes we need to check.
In the Traveling Salesman Problem, the route starts and ends in the same place, which makes a cycle. This means that the length of the shortest route will be the same no matter which city we start in. That is why we have chosen a fixed city to start in for the simulation above, and that reduces the number of possible routes from \(n!\) to \((n-1)!\).
Also, because these routes go in cycles, a route has the same distance if we go in one direction or the other, so we actually just need to check the distance of half of the routes, because the other half will just be the same routes in the opposite direction, so the number of routes we need to check is actually \( \frac{(n-1)!}{2}\).
But even if we can reduce the number of routes we need to check to \( \frac{(n-1)!}{2}\), the time complexity is still \( O(n!)\), because for very big \(n\), reducing \(n\) by one and dividing by 2 does not make a significant change in how the time complexity grows when \(n\) is increased.
To better understand how time complexity works, go to this page .
Actual Traveling Salesman Problems Are More Complex
The edge weight in a graph in this context of The Traveling Salesman Problem tells us how hard it is to go from one point to another, and it is the total edge weight of a route we want to minimize.
So far on this page, the edge weight has been the distance in a straight line between two points. And that makes it much easier to explain the Traveling Salesman Problem, and to display it.
But in the real world there are many other things that affects the edge weight:
- Obstacles: When moving from one place to another, we normally try to avoid obstacles like trees, rivers, houses for example. This means it is longer and takes more time to go from A to B, and the edge weight value needs to be increased to factor that in, because it is not a straight line anymore.
- Transportation Networks: We usually follow a road or use public transport systems when traveling, and that also affects how hard it is to go (or send a package) from one place to another.
- Traffic Conditions: Travel congestion also affects the travel time, so that should also be reflected in the edge weight value.
- Legal and Political Boundaries: Crossing border for example, might make one route harder to choose than another, which means the shortest straight line route might be slower, or more costly.
- Economic Factors: Using fuel, using the time of employees, maintaining vehicles, all these things cost money and should also be factored into the edge weights.
As you can see, just using the straight line distances as the edge weights, might be too simple compared to the real problem. And solving the Traveling Salesman Problem for such a simplified problem model would probably give us a solution that is not optimal in a practical sense.
It is not easy to visualize a Traveling Salesman Problem when the edge length is not just the straight line distance between two points anymore, but the computer handles that very well.

COLOR PICKER

Contact Sales
If you want to use W3Schools services as an educational institution, team or enterprise, send us an e-mail: [email protected]
Report Error
If you want to report an error, or if you want to make a suggestion, send us an e-mail: [email protected]
Top Tutorials
Top references, top examples, get certified.
Traveling salesman problem
This web page is a duplicate of https://optimization.mccormick.northwestern.edu/index.php/Traveling_salesman_problems
Author: Jessica Yu (ChE 345 Spring 2014)
Steward: Dajun Yue, Fengqi You
The traveling salesman problem (TSP) is a widely studied combinatorial optimization problem, which, given a set of cities and a cost to travel from one city to another, seeks to identify the tour that will allow a salesman to visit each city only once, starting and ending in the same city, at the minimum cost. 1
- 2.1 Graph Theory
- 2.2 Classifications of the TSP
- 2.3 Variations of the TSP
- 3.1 aTSP ILP Formulation
- 3.2 sTSP ILP Formulation
- 4.1 Exact algorithms
- 4.2.1 Tour construction procedures
- 4.2.2 Tour improvement procedures
- 5 Applications
- 7 References
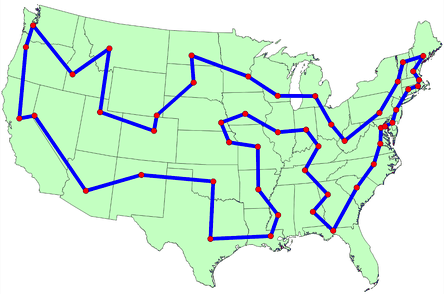
The origins of the traveling salesman problem are obscure; it is mentioned in an 1832 manual for traveling salesman, which included example tours of 45 German cities but gave no mathematical consideration. 2 W. R. Hamilton and Thomas Kirkman devised mathematical formulations of the problem in the 1800s. 2
It is believed that the general form was first studied by Karl Menger in Vienna and Harvard in the 1930s. 2,3
Hassler Whitney, who was working on his Ph.D. research at Harvard when Menger was a visiting lecturer, is believed to have posed the problem of finding the shortest route between the 48 states of the United States during either his 1931-1932 or 1934 seminar talks. 2 There is also uncertainty surrounding the individual who coined the name “traveling salesman problem” for Whitney’s problem. 2
The problem became increasingly popular in the 1950s and 1960s. Notably, George Dantzig, Delber R. Fulkerson, and Selmer M. Johnson at the RAND Corporation in Santa Monica, California solved the 48 state problem by formulating it as a linear programming problem. 2 The methods described in the paper set the foundation for future work in combinatorial optimization, especially highlighting the importance of cutting planes. 2,4
In the early 1970s, the concept of P vs. NP problems created buzz in the theoretical computer science community. In 1972, Richard Karp demonstrated that the Hamiltonian cycle problem was NP-complete, implying that the traveling salesman problem was NP-hard. 4
Increasingly sophisticated codes led to rapid increases in the sizes of the traveling salesman problems solved. Dantzig, Fulkerson, and Johnson had solved a 48 city instance of the problem in 1954. 5 Martin Grötechel more than doubled this 23 years later, solving a 120 city instance in 1977. 5 Enoch Crowder and Manfred W. Padberg again more than doubled this in just 3 years, with a 318 city solution. 5
In 1987, rapid improvements were made, culminating in a 2,392 city solution by Padberg and Giovanni Rinaldi. In the following two decades, David L. Appelgate, Robert E. Bixby, Vasek Chvátal, & William J. Cook led the cutting edge, solving a 7,397 city instance in 1994 up to the current largest solved problem of 24,978 cities in 2004. 5
Description
Graph theory.
In the context of the traveling salesman problem, the verticies correspond to cities and the edges correspond to the path between those cities. When modeled as a complete graph, paths that do not exist between cities can be modeled as edges of very large cost without loss of generality. 6 Minimizing the sum of the costs for Hamiltonian cycle is equivalent to identifying the shortest path in which each city is visiting only once.
Classifications of the TSP
The TRP can be divided into two classes depending on the nature of the cost matrix. 3,6
- Applies when the distance between cities is the same in both directions
- Applies when there are differences in distances (e.g. one-way streets)
An ATSP can be formulated as an STSP by doubling the number of nodes. 6
Variations of the TSP
Formulation
The objective function is then given by
To ensure that the result is a valid tour, several contraints must be added. 1,3
There are several other formulations for the subtour elimnation contraint, including circuit packing contraints, MTZ constraints, and network flow constraints.
aTSP ILP Formulation
The integer linear programming formulation for an aTSP is given by
sTSP ILP Formulation
The symmetric case is a special case of the asymmetric case and the above formulation is valid. 3, 6 The integer linear programming formulation for an sTSP is given by
Exact algorithms
Branch-and-bound algorithms are commonly used to find solutions for TSPs. 7 The ILP is first relaxed and solved as an LP using the Simplex method, then feasibility is regained by enumeration of the integer variables. 7
Other exact solution methods include the cutting plane method and branch-and-cut. 8
Heuristic algorithms
Given that the TSP is an NP-hard problem, heuristic algorithms are commonly used to give a approximate solutions that are good, though not necessarily optimal. The algorithms do not guarantee an optimal solution, but gives near-optimal solutions in reasonable computational time. 3 The Held-Karp lower bound can be calculated and used to judge the performance of a heuristic algorithm. 3
There are two general heuristic classifications 7 :
- Tour construction procedures where a solution is gradually built by adding a new vertex at each step
- Tour improvement procedures where a feasbile solution is improved upon by performing various exchanges
The best methods tend to be composite algorithms that combine these features. 7
Tour construction procedures
Tour improvement procedures
Applications
The importance of the traveling salesman problem is two fold. First its ubiquity as a platform for the study of general methods than can then be applied to a variety of other discrete optimization problems. 5 Second is its diverse range of applications, in fields including mathematics, computer science, genetics, and engineering. 5,6
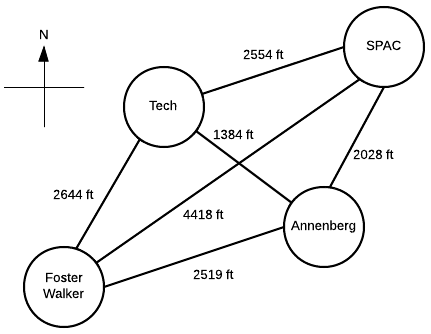
Suppose a Northwestern student, who lives in Foster-Walker , has to accomplish the following tasks:
- Drop off a homework set at Tech
- Work out a SPAC
- Complete a group project at Annenberg
Distances between buildings can be found using Google Maps. Note that there is particularly strong western wind and walking east takes 1.5 times as long.
It is the middle of winter and the student wants to spend the least possible time walking. Determine the path the student should take in order to minimize walking time, starting and ending at Foster-Walker.
Start with the cost matrix (with altered distances taken into account):
Method 1: Complete Enumeration
All possible paths are considered and the path of least cost is the optimal solution. Note that this method is only feasible given the small size of the problem.
From inspection, we see that Path 4 is the shortest. So, the student should walk 2.28 miles in the following order: Foster-Walker → Annenberg → SPAC → Tech → Foster-Walker
Method 2: Nearest neighbor
Starting from Foster-Walker, the next building is simply the closest building that has not yet been visited. With only four nodes, this can be done by inspection:
- Smallest distance is from Foster-Walker is to Annenberg
- Smallest distance from Annenberg is to Tech
- Smallest distance from Tech is to Annenberg ( creates a subtour, therefore skip )
- Next smallest distance from Tech is to Foster-Walker ( creates a subtour, therefore skip )
- Next smallest distance from Tech is to SPAC
- Smallest distance from SPAC is to Annenberg ( creates a subtour, therefore skip )
- Next smallest distance from SPAC is to Tech ( creates a subtour, therefore skip )
- Next smallest distance from SPAC is to Foster-Walker
So, the student would walk 2.54 miles in the following order: Foster-Walker → Annenberg → Tech → SPAC → Foster-Walker
Method 3: Greedy
With this method, the shortest paths that do not create a subtour are selected until a complete tour is created.
- Smallest distance is Annenberg → Tech
- Next smallest is SPAC → Annenberg
- Next smallest is Tech → Annenberg ( creates a subtour, therefore skip )
- Next smallest is Anneberg → Foster-Walker ( creates a subtour, therefore skip )
- Next smallest is SPAC → Tech ( creates a subtour, therefore skip )
- Next smallest is Tech → Foster-Walker
- Next smallest is Annenberg → SPAC ( creates a subtour, therefore skip )
- Next smallest is Foster-Walker → Annenberg ( creates a subtour, therefore skip )
- Next smallest is Tech → SPAC ( creates a subtour, therefore skip )
- Next smallest is Foster-Walker → Tech ( creates a subtour, therefore skip )
- Next smallest is SPAC → Foster-Walker ( creates a subtour, therefore skip )
- Next smallest is Foster-Walker → SPAC
So, the student would walk 2.40 miles in the following order: Foster-Walker → SPAC → Annenberg → Tech → Foster-Walker
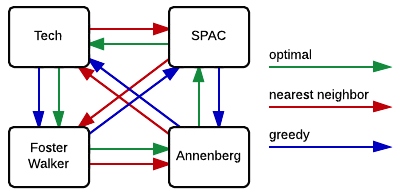
As we can see in the figure to the right, the heuristic methods did not give the optimal solution. That is not to say that heuristics can never give the optimal solution, just that it is not guaranteed.
Both the optimal and the nearest neighbor algorithms suggest that Annenberg is the optimal first building to visit. However, the optimal solution then goes to SPAC, while both heuristic methods suggest Tech. This is in part due to the large cost of SPAC → Foster-Walker. The heuristic algorithms cannot take this future cost into account, and therefore fall into that local optimum.
We note that the nearest neighbor and greedy algorithms give solutions that are 11.4% and 5.3%, respectively, above the optimal solution. In the scale of this problem, this corresponds to fractions of a mile. We also note that neither heuristic gave the worst case result, Foster-Walker → SPAC → Tech → Annenberg → Foster-Walker.
Only tour building heuristics were used. Combined with a tour improvement algorithm (such as 2-opt or simulated annealing), we imagine that we may be able to locate solutions that are closer to the optimum.
The exact algorithm used was complete enumeration, but we note that this is impractical even for 7 nodes (6! or 720 different possibilities). Commonly, the problem would be formulated and solved as an ILP to obtain exact solutions.
- Vanderbei, R. J. (2001). Linear programming: Foundations and extensions (2nd ed.). Boston: Kluwer Academic.
- Schrijver, A. (n.d.). On the history of combinatorial optimization (till 1960).
- Matai, R., Singh, S., & Lal, M. (2010). Traveling salesman problem: An overview of applications, formulations, and solution approaches. In D. Davendra (Ed.), Traveling Salesman Problem, Theory and Applications . InTech.
- Junger, M., Liebling, T., Naddef, D., Nemhauser, G., Pulleyblank, W., Reinelt, G., Rinaldi, G., & Wolsey, L. (Eds.). (2009). 50 years of integer programming, 1958-2008: The early years and state-of-the-art surveys . Heidelberg: Springer.
- Cook, W. (2007). History of the TSP. The Traveling Salesman Problem . Retrieved from http://www.math.uwaterloo.ca/tsp/history/index.htm
- Punnen, A. P. (2002). The traveling salesman problem: Applications, formulations and variations. In G. Gutin & A. P. Punnen (Eds.), The Traveling Salesman Problem and its Variations . Netherlands: Kluwer Academic Publishers.
- Laporte, G. (1992). The traveling salesman problem: An overview of exact and approximate algorithms. European Journal of Operational Research, 59 (2), 231–247.
- Goyal, S. (n.d.). A suvey on travlling salesman problem.
Navigation menu
Forgot password? New user? Sign up
Existing user? Log in
Traveling Salesperson Problem
Already have an account? Log in here.
A salesperson needs to visit a set of cities to sell their goods. They know how many cities they need to go to and the distances between each city. In what order should the salesperson visit each city exactly once so that they minimize their travel time and so that they end their journey in their city of origin?
The traveling salesperson problem is an extremely old problem in computer science that is an extension of the Hamiltonian Circuit Problem . It has important implications in complexity theory and the P versus NP problem because it is an NP-Complete problem . This means that a solution to this problem cannot be found in polynomial time (it takes superpolynomial time to compute an answer). In other words, as the number of vertices increases linearly, the computation time to solve the problem increases exponentially.
The following image is a simple example of a network of cities connected by edges of a specific distance. The origin city is also marked.
Network of cities
Here is the solution for that network, it has a distance traveled of only 14. Any other path that the salesman can takes will result in a path length that is more than 14.
Relationship to Graphs
Special kinds of tsp, importance for p vs np, applications.
The traveling salesperson problem can be modeled as a graph . Specifically, it is typical a directed, weighted graph. Each city acts as a vertex and each path between cities is an edge. Instead of distances, each edge has a weight associated with it. In this model, the goal of the traveling salesperson problem can be defined as finding a path that visits every vertex, returns to the original vertex, and minimizes total weight.
To that end, many graph algorithms can be used on this model. Search algorithms like breadth-first search (BFS) , depth-first search (DFS) , and Dijkstra's shortest path algorithm can certainly be used, however, they do not take into consideration that fact that every vertex must be visited.
The Traveling Salesperson Problem (TSP), an NP-Complete problem, is notoriously complicated to solve. That is because the greedy approach is so computational intensive. The greedy approach to solving this problem would be to try every single possible path and see which one is the fastest. Try this conceptual question to see if you have a grasp for how hard it is to solve.
For a fully connected map with \(n\) cities, how many total paths are possible for the traveling salesperson? Show Answer There are (n-1)! total paths the salesperson can take. The computation needed to solve this problem in this way grows far too quickly to be a reasonable solution. If this map has only 5 cities, there are \(4!\), or 24, paths. However, if the size of this map is increased to 20 cities, there will be \(1.22 \cdot 10^{17}\) paths!
The greedy approach to TSP would go like this:
- Find all possible paths.
- Find the cost of every paths.
- Choose the path with the lowest cost.
Another version of a greedy approach might be: At every step in the algorithm, choose the best possible path. This version might go a little quicker, but it's not guaranteed to find the best answer, or an answer at all since it might hit a dead end.
For NP-Hard problems (a subset of NP-Complete problems) like TSP, exact solutions can only be implemented in a reasonable amount of time for small input sizes (maps with few cities). Otherwise, the best approach we can do is provide a heuristic to help the problem move forward in an optimal way. However, these approaches cannot be proven to be optimal because they always have some sort of downside.
Small input sizes
As described, in a previous section , the greedy approach to this problem has a complexity of \(O(n!)\). However, there are some approaches that decrease this computation time.
The Held-Karp Algorithm is one of the earliest applications of dynamic programming . Its complexity is much lower than the greedy approach at \(O(n^2 2^n)\). Basically what this algorithm says is that every sub path along an optimal path is itself an optimal path. So, computing an optimal path is the same as computing many smaller subpaths and adding them together.
Heuristics are a way of ranking possible next steps in an algorithm in the hopes of cutting down computation time for the entire algorithm. They are often a tradeoff of some attribute - such as completeness, accuracy, or precision - in favor of speed. Heuristics exist for the traveling salesperson problem as well.
The most simple heuristic for this problem is the greedy heuristic. This heuristic simply says, at each step of the network traversal, choose the best next step. In other words, always choose the closest city that you have not yet visited. This heuristic seems like a good one because it is simple and intuitive, and it is even used in practice sometimes, however there are heuristics that are proven to be more effective.
Christofides algorithm is another heuristic. It produces at most 1.5 times the optimal weight for TSP. This algorithm involves finding a minimum spanning tree for the network. Next, it creates matchings for the cities of an odd degree (meaning they have an odd number of edges coming out of them), calculates an eulerian path , and converts back to a TSP path.
Even though it is typically impossible to optimally solve TSP problems, there are cases of TSP problems that can be solved if certain conditions hold.
The metric-TSP is an instance of TSP that satisfies this condition: The distance from city A to city B is less than or equal to the distance from city A to city C plus the distance from city C to city B. Or,
\[distance_{AB} \leq distance_{AC} + distance_{CB}\]
This is a condition that holds in the real world, but it can't always be expected to hold for every TSP problem. But, with this inequality in place, the approximated path will be no more than twice the optimal path. Even better, we can bound the solution to a \(3/2\) approximation by using Christofide's Algorithm .
The euclidean-TSP has an even stricter constraint on the TSP input. It states that all cities' edges in the network must obey euclidean distances . Recent advances have shown that approximation algorithms using euclidean minimum spanning trees have reduced the runtime of euclidean-TSP, even though they are also NP-hard. In practice, though, simpler heuristics are still used.
The P versus NP problem is one of the leading questions in modern computer science. It asks whether or not every problem whose solution can be verified in polynomial time by a computer can also be solved in polynomial time by a computer. TSP, for example, cannot be solved in polynomial time (at least that's what is currently theorized). However, TSP can be solved in polynomial time when it is phrased like this: Given a graph and an integer, x, decide if there is a path of length x or less than x . It's easy to see that given a proposed answer to this question, it is simple to check if it is less than or equal to x.
The traveling salesperson problem, like other problems that are NP-Complete, are very important to this debate. That is because if a polynomial time solution can be found to this problems, then \(P = NP\). As it stands, most scientists believe that \(P \ne NP\).
The traveling salesperson problem has many applications. The obvious ones are in the transportation space. Planning delivery routes or flight patterns, for example, would benefit immensly from breakthroughs is this problem or in the P versus NP problem .
However, this same logic can be applied to many facets of planning as well. In robotics, for instance, planning the order in which to drill holes in a circuit board is a complex task due to the sheer number of holes that must be drawn.
The best and most important application of TSP, however, comes from the fact that it is an NP-Complete problem. That means that its practical applications amount to the applications of any problem that is NP-Complete. So, if there are significant breakthroughs for TSP, that means that those exact same breakthrough can be applied to any problem in the NP-Complete class.
Problem Loading...
Note Loading...
Set Loading...
The traveling salesman game: An application ofcost allocation in a gas and oil company
- Published: August 1998
- Volume 82 , pages 203–218, ( 1998 )
Cite this article
- Stefan Engevall ,
- Maud Göthe-Lundgren &
- Peter Värbrand
672 Accesses
33 Citations
1 Altmetric
Explore all metrics
In this article, a cost allocation problem that arises in a distribution planning situation atthe Logistics Department at Norsk Hydro Olje AB is studied. A specific tour is considered,for which the total distribution cost is to be divided among the customers that are visited.This problem is formulated as a traveling salesman game, and cost allocation methods basedon different concepts from cooperative game theory, such as the nucleolus, the Shapleyvalue and the t-value, are discussed. Additionally, a new concept is introduced: the demandnucleolus. Computational results for the Norsk Hydro case are presented and discussed.
This is a preview of subscription content, log in via an institution to check access.
Access this article
Price includes VAT (Russian Federation)
Instant access to the full article PDF.
Rent this article via DeepDyve
Institutional subscriptions
Similar content being viewed by others
Shapley value-based cost allocation in the cooperative traveling salesman problem under rolling horizon planning.
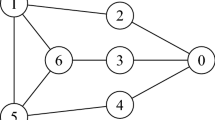
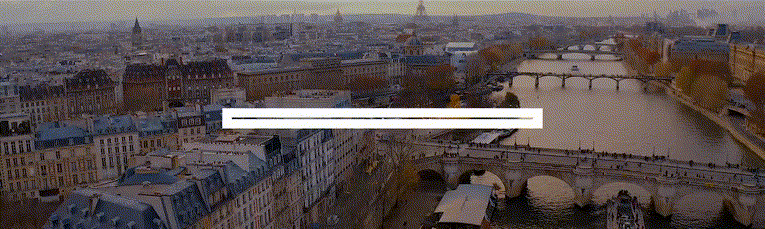
Cooperation of customers in traveling salesman problems with profits
A unified matheuristic for solving multi-constrained traveling salesman problems with profits.
L.J. Billera, D.C. Heath and J. Raanan, Internal telephone billing rates – a novel application of non-atomic game theory, Operations Research 26(1978)956– 965.
Google Scholar
C.G. Bird, On cost allocation for a spanning tree: A game theoretic approach, Networks 6(1976)335 – 350.
E. Bjørndal, Computation of the nucleolus of tree and 1-tree games, Ph.D. Thesis, 1995.
A Claus and D.J. Kleitman, Cost allocation for a spanning tree, Networks 3(1973)289 – 304.
I. Dragan, A procedure for finding the nucleolus of a cooperative n -person game, Z. Oper. Res. Ser. A-B 25(1981)119 – 131.
T. Driessen, Cooperative Games, Solutions and Applications , Kluwer Academic, Dordrecht, The Netherlands, 1988.
M. Dror, The traveling salesman, binpacking and the knapsack, Appl. Math. Comput. 35(1990)195 – 207.
Article Google Scholar
M. Dror and M.O. Ball, Inventory/routing: Reduction from an annual to a short-period problem, Naval Res. Logist. 34(1987)891 – 905.
P. Dubey and L.S. Shapley, Totally balanced games arising from controlled programming problems, Math. Programming 29(1984)245–267.
S. Engevall, Cost allocation in distribution planning, Licentiate Thesis, Department of Mathematics, Linköping Institute of Technology, Linköping, Sweden, 1996.
S. Engevall, M. Göthe-Lundgren, P.O. Lindberg and P. Värbrand, A note on the core of the Euclidean traveling salesman game with a home city, LiTH-MAT-R-1996-24, Department of Mathematics, Linköping Institute of Technology, Linköping, Sweden, 1996.
D. Gately, Sharing the gains from regional cooperation: A game theoretic application to planning investment in electric power, International Economic Review 15(1974)195 – 208.
D.B. Gillies, Solutions to general non-zero-sum games, in: Contributions to the Theory of Games , IV , Annals of Mathematical Studies 40, Princeton University Press, Princeton, NJ, 1959, pp. 47 – 85.
M. Göthe-Lundgren, K. Jörnsten and P. Värbrand, Cost allocation in minimum 1-trees, LiTH-MATR-1992-33, Department of Mathematics, Linköping Institute of Technology, Linköping, Sweden, 1992.
M. Göthe-Lundgren, K. Jörnsten and P. Värbrand, On the nucleolus of the basic vehicle routing game, Math. Programming 72(1996)83 –100.
D. Granot and G. Huberman, Minimum cost spanning tree games, Math. Programming 21(1981)1 – 18.
D. Granot and G. Huberman, On the core and nucleolus of minimum cost spanning tree games, Math. Programming 29(1984)323 – 347.
D. Granot, M. Maschler, G. Owen and W.R. Zhu, The kernel/nucleolus of a standard tree game, Int.. J. Game Theory 25(1996)219 – 244.
J.H. Grotte, Computations of and observations on the nucleolus, the normalized nucleolus and the central games, M.Sc. Thesis, Cornell University, Ithaca, New York, 1970.
Å. Hallefjord, R. Helming and K. Jörnsten, Computing the nucleolus when the characteristic function is given implicitly: A constraint generation approach, Internat. J. Game Theory 24(1995) 357 – 372 (originally: Internal Report, Chr. Michelsen Institute, Bergen, Norway, 1988).
P. Jaillet and A.R. Odoni, The probabilistic vehicle routing problem, in: Vehicle Routing: Methods and Studies , eds. B.L. Golden and A.A. Assad, Studies in Management Science and Systems 16, North-Holland, Amsterdam, 1988, pp. 222–248.
A. Kopelowitz, Computation of the kernels of simple games and the nucleolus of n -person games, Research Memorandum No. 31, Department of Mathematics, The Hebrew University of Jerusalem, 1967.
J. Kuipers, A note on the 5-person traveling salesman game, Z. Oper. Res. 38(1993)131–139.
S.C. Littlechild, G.F. Thompson, Aircraft landing fees: A game theory approach, Bell Journal of Economics 8(1977)186 – 204.
N. Megiddo, Cost allocation for Steiner trees, Networks 8(1978)1 – 6.
T. Parker, Allocation of the Tennessee Valley Authority projects, Transactions of the American Society of Civil Engineering 108(1943)174 – 187.
J.A.M. Potters, A class of traveling salesman games, in: 12th Symposium on Operations Research , Passau, 1987, Methods Oper. Res. 59, Athenäum/Hain/Hanstein, Königstein, 1989, pp. 263 – 276.
J.A.M. Potters, An axiomatization of the nucleolus, Internat. J. Game Theory 19(1990)365 – 373.
J.A.M. Potters, I.J. Curiel and S.H. Tijs, Traveling salesman games, Math. programming 53(1992)113 – 129.
D. Schmeidler, The nucleolus of a characteristic function game, SIAM J. Appl. Math. 17(1969)1163 –1170.
L.S. Shapley, A value for n -person games, in: Contributions to the Theory of Games, II , Annals of Mathematical Studies 28, Princeton University Press, Princeton, NJ, 1953, pp. 307—317.
L.S. Shapley, On balanced sets and cores, Naval Res. Logist. Quart. 14(1967)453 – 460.
L.S. Shapley, Cores of convex games, Int. J. Game Theory 1(1971)11–26; errata: 1(1971) 199.
L. Shapley, Discussant's comments: “Equity considerations in traditional full cost allocation practices: An axiomatic perspective”, in: Joint Cost Allocations , ed. S. Moriarity, Center for Economic and Management Research, Norman, OK, 1981, pp. 131 – 136.
M. Shubik, Game Theory in the Social Sciences. Concepts and Solutions , MIT Press, Cambridge, MA/London, 1982.
C. Snijders, Axiomatization of the nucleolus, Math. Oper. Res. 20(1995)189 – 196.
P.D. Straffin and J.P. Heaney, Game theory and the Tennessee Valley Authority, Int. J. Game Theory, 10(1981)35–43.
A. Tamir, On the core of a traveling salesman cost allocation game, Oper. Res. Lett. 8(1989)31 – 34.
S.H. Tijs, Bounds for the core and the τ-value, in: Game Theory and Mathematical Economics , eds. O. Moeschlin and D. Pallaschke, North-Holland, Amsterdam, 1981, pp. 123 –132.
S.H. Tijs, An axiomatization of the τ-value, Math. Social Sci. 13(1987)177 – 181.
S.H. Tijs and T.S.H. Driessen, Game theory and cost allocation problems, Management Sci. 32 (1986)1015 – 1028.
H.P. Young, Methods and principles of cost allocation, in: Cost Allocation: Methods, Principles, Applications , ed. H.P. Young, North-Holland, Amsterdam, 1985, pp. 3 – 30.
H.P. Young, N. Okada and T. Hashimoto, Cost allocation in water resources development, Water Resources Research 18(1982)463 – 475.
Download references
You can also search for this author in PubMed Google Scholar
Rights and permissions
Reprints and permissions
About this article
Engevall, S., Göthe-Lundgren, M. & Värbrand, P. The traveling salesman game: An application ofcost allocation in a gas and oil company. Annals of Operations Research 82 , 203–218 (1998). https://doi.org/10.1023/A:1018935324969
Download citation
Issue Date : August 1998
DOI : https://doi.org/10.1023/A:1018935324969
Share this article
Anyone you share the following link with will be able to read this content:
Sorry, a shareable link is not currently available for this article.
Provided by the Springer Nature SharedIt content-sharing initiative
- Travel Salesman Problem
- Vehicle Route Problem
- Grand Coalition
- Cost Allocation
- Cooperative Game Theory
- Find a journal
- Publish with us
- Track your research
12.9 Traveling Salesperson Problem
Learning objectives.
After completing this section, you should be able to:
- Distinguish between brute force algorithms and greedy algorithms.
- List all distinct Hamilton cycles of a complete graph.
- Apply brute force method to solve traveling salesperson applications.
- Apply nearest neighbor method to solve traveling salesperson applications.
We looked at Hamilton cycles and paths in the previous sections Hamilton Cycles and Hamilton Paths . In this section, we will analyze Hamilton cycles in complete weighted graphs to find the shortest route to visit a number of locations and return to the starting point. Besides the many routing applications in which we need the shortest distance, there are also applications in which we search for the route that is least expensive or takes the least time. Here are a few less common applications that you can read about on a website set up by the mathematics department at the University of Waterloo in Ontario, Canada:
- Design of fiber optic networks
- Minimizing fuel expenses for repositioning satellites
- Development of semi-conductors for microchips
- A technique for mapping mammalian chromosomes in genome sequencing
Before we look at approaches to solving applications like these, let's discuss the two types of algorithms we will use.
Brute Force and Greedy Algorithms
An algorithm is a sequence of steps that can be used to solve a particular problem. We have solved many problems in this chapter, and the procedures that we used were different types of algorithms. In this section, we will use two common types of algorithms, a brute force algorithm and a greedy algorithm . A brute force algorithm begins by listing every possible solution and applying each one until the best solution is found. A greedy algorithm approaches a problem in stages, making the apparent best choice at each stage, then linking the choices together into an overall solution which may or may not be the best solution.
To understand the difference between these two algorithms, consider the tree diagram in Figure 12.187 . Suppose we want to find the path from left to right with the largest total sum. For example, branch A in the tree diagram has a sum of 10 + 2 + 11 + 13 = 36 10 + 2 + 11 + 13 = 36 .
To be certain that you pick the branch with greatest sum, you could list each sum from each of the different branches:
A : 10 + 2 + 11 + 13 = 36 10 + 2 + 11 + 13 = 36
B : 10 + 2 + 11 + 8 = 31 10 + 2 + 11 + 8 = 31
C : 10 + 2 + 15 + 1 = 28 10 + 2 + 15 + 1 = 28
D : 10 + 2 + 15 + 6 = 33 10 + 2 + 15 + 6 = 33
E : 10 + 7 + 3 + 20 = 40 10 + 7 + 3 + 20 = 40
F : 10 + 7 + 3 + 14 = 34 10 + 7 + 3 + 14 = 34
G : 10 + 7 + 4 + 11 = 32 10 + 7 + 4 + 11 = 32
H : 10 + 7 + 4 + 5 = 26 10 + 7 + 4 + 5 = 26
Then we know with certainty that branch E has the greatest sum.
Now suppose that you wanted to find the branch with the highest value, but you only were shown the tree diagram in phases, one step at a time.
After phase 1, you would have chosen the branch with 10 and 7. So far, you are following the same branch. Let’s look at the next phase.
After phase 2, based on the information you have, you will choose the branch with 10, 7 and 4. Now, you are following a different branch than before, but it is the best choice based on the information you have. Let’s look at the last phase.
After phase 3, you will choose branch G which has a sum of 32.
The process of adding the values on each branch and selecting the highest sum is an example of a brute force algorithm because all options were explored in detail. The process of choosing the branch in phases, based on the best choice at each phase is a greedy algorithm. Although a brute force algorithm gives us the ideal solution, it can take a very long time to implement. Imagine a tree diagram with thousands or even millions of branches. It might not be possible to check all the sums. A greedy algorithm, on the other hand, can be completed in a relatively short time, and generally leads to good solutions, but not necessarily the ideal solution.
Example 12.42
Distinguishing between brute force and greedy algorithms.
A cashier rings up a sale for $4.63 cents in U.S. currency. The customer pays with a $5 bill. The cashier would like to give the customer $0.37 in change using the fewest coins possible. The coins that can be used are quarters ($0.25), dimes ($0.10), nickels ($0.05), and pennies ($0.01). The cashier starts by selecting the coin of highest value less than or equal to $0.37, which is a quarter. This leaves $ 0.37 − $ 0.25 = $ 0.12 $ 0.37 − $ 0.25 = $ 0.12 . The cashier selects the coin of highest value less than or equal to $0.12, which is a dime. This leaves $ 0.12 − $ 0.10 = $ 0.02 $ 0.12 − $ 0.10 = $ 0.02 . The cashier selects the coin of highest value less than or equal to $0.02, which is a penny. This leaves $ 0.02 − $ 0.01 = $ 0.01 $ 0.02 − $ 0.01 = $ 0.01 . The cashier selects the coin of highest value less than or equal to $0.01, which is a penny. This leaves no remainder. The cashier used one quarter, one dime, and two pennies, which is four coins. Use this information to answer the following questions.
- Is the cashier’s approach an example of a greedy algorithm or a brute force algorithm? Explain how you know.
- The cashier’s solution is the best solution. In other words, four is the fewest number of coins possible. Is this consistent with the results of an algorithm of this kind? Explain your reasoning.
- The approach the cashier used is an example of a greedy algorithm, because the problem was approached in phases and the best choice was made at each phase. Also, it is not a brute force algorithm, because the cashier did not attempt to list out all possible combinations of coins to reach this conclusion.
- Yes, it is consistent. A greedy algorithm does not always yield the best result, but sometimes it does.
Your Turn 12.42
The traveling salesperson problem.
Now let’s focus our attention on the graph theory application known as the traveling salesperson problem (TSP) in which we must find the shortest route to visit a number of locations and return to the starting point.
Recall from Hamilton Cycles , the officer in the U.S. Air Force who is stationed at Vandenberg Air Force base and must drive to visit three other California Air Force bases before returning to Vandenberg. The officer needed to visit each base once. We looked at the weighted graph in Figure 12.192 representing the four U.S. Air Force bases: Vandenberg, Edwards, Los Angeles, and Beal and the distances between them.
Any route that visits each base and returns to the start would be a Hamilton cycle on the graph. If the officer wants to travel the shortest distance, this will correspond to a Hamilton cycle of lowest weight. We saw in Table 12.11 that there are six distinct Hamilton cycles (directed cycles) in a complete graph with four vertices, but some lie on the same cycle (undirected cycle) in the graph.
Since the distance between bases is the same in either direction, it does not matter if the officer travels clockwise or counterclockwise. So, there are really only three possible distances as shown in Figure 12.193 .
The possible distances are:
So, a Hamilton cycle of least weight is V → B → E → L → V (or the reverse direction). The officer should travel from Vandenberg to Beal to Edwards, to Los Angeles, and back to Vandenberg.
Finding Weights of All Hamilton Cycles in Complete Graphs
Notice that we listed all of the Hamilton cycles and found their weights when we solved the TSP about the officer from Vandenberg. This is a skill you will need to practice. To make sure you don't miss any, you can calculate the number of possible Hamilton cycles in a complete graph. It is also helpful to know that half of the directed cycles in a complete graph are the same cycle in reverse direction, so, you only have to calculate half the number of possible weights, and the rest are duplicates.
In a complete graph with n n vertices,
- The number of distinct Hamilton cycles is ( n − 1 ) ! ( n − 1 ) ! .
- There are at most ( n − 1 ) ! 2 ( n − 1 ) ! 2 different weights of Hamilton cycles.
TIP! When listing all the distinct Hamilton cycles in a complete graph, you can start them all at any vertex you choose. Remember, the cycle a → b → c → a is the same cycle as b → c → a → b so there is no need to list both.
Example 12.43
Calculating possible weights of hamilton cycles.
Suppose you have a complete weighted graph with vertices N, M, O , and P .
- Use the formula ( n − 1 ) ! ( n − 1 ) ! to calculate the number of distinct Hamilton cycles in the graph.
- Use the formula ( n − 1 ) ! 2 ( n − 1 ) ! 2 to calculate the greatest number of different weights possible for the Hamilton cycles.
- Are all of the distinct Hamilton cycles listed here? How do you know? Cycle 1: N → M → O → P → N Cycle 2: N → M → P → O → N Cycle 3: N → O → M → P → N Cycle 4: N → O → P → M → N Cycle 5: N → P → M → O → N Cycle 6: N → P → O → M → N
- Which pairs of cycles must have the same weights? How do you know?
- There are 4 vertices; so, n = 4 n = 4 . This means there are ( n − 1 ) ! = ( 4 − 1 ) ! = 3 ⋅ 2 ⋅ 1 = 6 ( n − 1 ) ! = ( 4 − 1 ) ! = 3 ⋅ 2 ⋅ 1 = 6 distinct Hamilton cycles beginning at any given vertex.
- Since n = 4 n = 4 , there are ( n − 1 ) ! 2 = ( 4 − 1 ) ! 2 = 6 2 = 3 ( n − 1 ) ! 2 = ( 4 − 1 ) ! 2 = 6 2 = 3 possible weights.
- Yes, they are all distinct cycles and there are 6 of them.
- Cycles 1 and 6 have the same weight, Cycles 2 and 4 have the same weight, and Cycles 3 and 5 have the same weight, because these pairs follow the same route through the graph but in reverse.
TIP! When listing the possible cycles, ignore the vertex where the cycle begins and ends and focus on the ways to arrange the letters that represent the vertices in the middle. Using a systematic approach is best; for example, if you must arrange the letters M, O, and P, first list all those arrangements beginning with M, then beginning with O, and then beginning with P, as we did in Example 12.42.
Your Turn 12.43
The brute force method.
The method we have been using to find a Hamilton cycle of least weight in a complete graph is a brute force algorithm, so it is called the brute force method . The steps in the brute force method are:
Step 1: Calculate the number of distinct Hamilton cycles and the number of possible weights.
Step 2: List all possible Hamilton cycles.
Step 3: Find the weight of each cycle.
Step 4: Identify the Hamilton cycle of lowest weight.
Example 12.44
Applying the brute force method.
On the next assignment, the air force officer must leave from Travis Air Force base, visit Beal, Edwards, and Vandenberg Air Force bases each exactly once and return to Travis Air Force base. There is no need to visit Los Angeles Air Force base. Use Figure 12.194 to find the shortest route.
Step 1: Since there are 4 vertices, there will be ( 4 − 1 ) ! = 3 ! = 6 ( 4 − 1 ) ! = 3 ! = 6 cycles, but half of them will be the reverse of the others; so, there will be ( 4 − 1 ) ! 2 = 6 2 = 3 ( 4 − 1 ) ! 2 = 6 2 = 3 possible distances.
Step 2: List all the Hamilton cycles in the subgraph of the graph in Figure 12.195 .
To find the 6 cycles, focus on the three vertices in the middle, B, E, and V . The arrangements of these vertices are BEV, BVE, EBV, EVB, VBE , and VEB . These would correspond to the 6 cycles:
1: T → B → E → V → T
2: T → B → V → E → T
3: T → E → B → V → T
4: T → E → V → B → T
5: T → V → B → E → T
6: T → V → E → B → T
Step 3: Find the weight of each path. You can reduce your work by observing the cycles that are reverses of each other.
1: 84 + 410 + 207 + 396 = 1097 84 + 410 + 207 + 396 = 1097
2: 84 + 396 + 207 + 370 = 1071 84 + 396 + 207 + 370 = 1071
3: 370 + 410 + 396 + 396 = 1572 370 + 410 + 396 + 396 = 1572
4: Reverse of cycle 2, 1071
5: Reverse of cycle 3, 1572
6: Reverse of cycle 1, 1097
Step 4: Identify a Hamilton cycle of least weight.
The second path, T → B → V → E → T , and its reverse, T → E → V → B → T , have the least weight. The solution is that the officer should travel from Travis Air Force base to Beal Air Force Base, to Vandenberg Air Force base, to Edwards Air Force base, and return to Travis Air Force base, or the same route in reverse.
Your Turn 12.44
Now suppose that the officer needed a cycle that visited all 5 of the Air Force bases in Figure 12.194 . There would be ( 5 − 1 ) ! = 4 ! = 4 × 3 × 2 × 1 = 24 ( 5 − 1 ) ! = 4 ! = 4 × 3 × 2 × 1 = 24 different arrangements of vertices and ( 5 − 1 ) ! 2 = 4 ! 2 = 24 2 = 12 ( 5 − 1 ) ! 2 = 4 ! 2 = 24 2 = 12 distances to compare using the brute force method. If you consider 10 Air Force bases, there would be ( 10 − 1 ) ! = 9 ! = 9 ⋅ 8 ⋅ 7 ⋅ 6 ⋅ 5 ⋅ 4 ⋅ 3 ⋅ 2 ⋅ 1 = 362 , 880 ( 10 − 1 ) ! = 9 ! = 9 ⋅ 8 ⋅ 7 ⋅ 6 ⋅ 5 ⋅ 4 ⋅ 3 ⋅ 2 ⋅ 1 = 362 , 880 different arrangements and ( 10 − 1 ) ! 2 = 9 ! 2 = 9 ⋅ 8 ⋅ 7 ⋅ 6 ⋅ 5 ⋅ 4 ⋅ 3 ⋅ 2 ⋅ 1 2 = 181 , 440 ( 10 − 1 ) ! 2 = 9 ! 2 = 9 ⋅ 8 ⋅ 7 ⋅ 6 ⋅ 5 ⋅ 4 ⋅ 3 ⋅ 2 ⋅ 1 2 = 181 , 440 distances to consider. There must be another way!
The Nearest Neighbor Method
When the brute force method is impractical for solving a traveling salesperson problem, an alternative is a greedy algorithm known as the nearest neighbor method , which always visit the closest or least costly place first. This method finds a Hamilton cycle of relatively low weight in a complete graph in which, at each phase, the next vertex is chosen by comparing the edges between the current vertex and the remaining vertices to find the lowest weight. Since the nearest neighbor method is a greedy algorithm, it usually doesn’t give the best solution, but it usually gives a solution that is "good enough." Most importantly, the number of steps will be the number of vertices. That’s right! A problem with 10 vertices requires 10 steps, not 362,880. Let’s look at an example to see how it works.
Suppose that a candidate for governor wants to hold rallies around the state. They plan to leave their home in city A , visit cities B, C, D, E , and F each once, and return home. The airfare between cities is indicated in the graph in Figure 12.196 .
Let’s help the candidate keep costs of travel down by applying the nearest neighbor method to find a Hamilton cycle that has a reasonably low weight. Begin by marking starting vertex as V 1 V 1 for "visited 1st." Then to compare the weights of the edges between A and vertices adjacent to A : $250, $210, $300, $200, and $100 as shown in Figure 12.197 . The lowest of these is $100, which is the edge between A and F .
Mark F as V 2 V 2 for "visited 2nd" then compare the weights of the edges between F and the remaining vertices adjacent to F : $170, $330, $150 and $350 as shown in Figure 12.198 . The lowest of these is $150, which is the edge between F and D .
Mark D as V 3 V 3 for "visited 3rd." Next, compare the weights of the edges between D and the remaining vertices adjacent to D : $120, $310, and $270 as shown in Figure 12.199 . The lowest of these is $120, which is the edge between D and B .
So, mark B as V 4 V 4 for "visited 4th." Finally, compare the weights of the edges between B and the remaining vertices adjacent to B : $160 and $220 as shown in Figure 12.200 . The lower amount is $160, which is the edge between B and E .
Now you can mark E as V 5 V 5 and mark the only remaining vertex, which is C , as V 6 V 6 . This is shown in Figure 12.201 . Make a note of the weight of the edge from E to C , which is $180, and from C back to A , which is $210.
The Hamilton cycle we found is A → F → D → B → E → C → A . The weight of the circuit is $ 100 + $ 150 + $ 120 + $ 160 + $ 180 + $ 210 = $ 920 $ 100 + $ 150 + $ 120 + $ 160 + $ 180 + $ 210 = $ 920 . This may or may not be the route with the lowest cost, but there is a good chance it is very close since the weights are most of the lowest weights on the graph and we found it in six steps instead of finding 120 different Hamilton cycles and calculating 60 weights. Let’s summarize the procedure that we used.
Step 1: Select the starting vertex and label V 1 V 1 for "visited 1st." Identify the edge of lowest weight between V 1 V 1 and the remaining vertices.
Step 2: Label the vertex at the end of the edge of lowest weight that you found in previous step as V n V n where the subscript n indicates the order the vertex is visited. Identify the edge of lowest weight between V n V n and the vertices that remain to be visited.
Step 3: If vertices remain that have not been visited, repeat Step 2. Otherwise, a Hamilton cycle of low weight is V 1 → V 2 → ⋯ → V n → V 1 V 1 → V 2 → ⋯ → V n → V 1 .
Example 12.45
Using the nearest neighbor method.
Suppose that the candidate for governor wants to hold rallies around the state but time before the election is very limited. They would like to leave their home in city A , visit cities B , C , D , E , and F each once, and return home. The airfare between cities is not as important as the time of travel, which is indicated in Figure 12.202 . Use the nearest neighbor method to find a route with relatively low travel time. What is the total travel time of the route that you found?
Step 1: Label vertex A as V 1 V 1 . The edge of lowest weight between A and the remaining vertices is 85 min between A and D .
Step 2: Label vertex D as V 2 V 2 . The edge of lowest weight between D and the vertices that remain to be visited, B, C, E , and F , is 70 min between D and F .
Repeat Step 2: Label vertex F as V 3 V 3 . The edge of lowest weight between F and the vertices that remain to be visited, B, C, and E , is 75 min between F and C .
Repeat Step 2: Label vertex C as V 4 V 4 . The edge of lowest weight between C and the vertices that remain to be visited, B and E , is 100 min between C and B .
Repeat Step 2: Label vertex B as V 5 V 5 . The only vertex that remains to be visited is E . The weight of the edge between B and E is 95 min.
Step 3: A Hamilton cycle of low weight is A → D → F → C → B → E → A . So, a route of relatively low travel time is A to D to F to C to B to E and back to A . The total travel time of this route is: 85 min + 70 min + 75 min + 100 min + 95 min + 90 min = 515 min or 8 hrs 35 min 85 min + 70 min + 75 min + 100 min + 95 min + 90 min = 515 min or 8 hrs 35 min
Your Turn 12.45
Check your understanding, section 12.9 exercises.
As an Amazon Associate we earn from qualifying purchases.
This book may not be used in the training of large language models or otherwise be ingested into large language models or generative AI offerings without OpenStax's permission.
Want to cite, share, or modify this book? This book uses the Creative Commons Attribution License and you must attribute OpenStax.
Access for free at https://openstax.org/books/contemporary-mathematics/pages/1-introduction
- Authors: Donna Kirk
- Publisher/website: OpenStax
- Book title: Contemporary Mathematics
- Publication date: Mar 22, 2023
- Location: Houston, Texas
- Book URL: https://openstax.org/books/contemporary-mathematics/pages/1-introduction
- Section URL: https://openstax.org/books/contemporary-mathematics/pages/12-9-traveling-salesperson-problem
© Dec 21, 2023 OpenStax. Textbook content produced by OpenStax is licensed under a Creative Commons Attribution License . The OpenStax name, OpenStax logo, OpenStax book covers, OpenStax CNX name, and OpenStax CNX logo are not subject to the Creative Commons license and may not be reproduced without the prior and express written consent of Rice University.
- Data Structures
- Linked List
- Binary Tree
- Binary Search Tree
- Segment Tree
- Disjoint Set Union
- Fenwick Tree
- Red-Black Tree
- Advanced Data Structures
- Graph Data Structure And Algorithms
- Introduction to Graphs - Data Structure and Algorithm Tutorials
- Graph and its representations
- Types of Graphs with Examples
- Basic Properties of a Graph
- Applications, Advantages and Disadvantages of Graph
- Transpose graph
- Difference Between Graph and Tree
BFS and DFS on Graph
- Breadth First Search or BFS for a Graph
- Depth First Search or DFS for a Graph
- Applications, Advantages and Disadvantages of Depth First Search (DFS)
- Applications, Advantages and Disadvantages of Breadth First Search (BFS)
- Iterative Depth First Traversal of Graph
- BFS for Disconnected Graph
- Transitive Closure of a Graph using DFS
- Difference between BFS and DFS
Cycle in a Graph
- Detect Cycle in a Directed Graph
- Detect cycle in an undirected graph
- Detect Cycle in a directed graph using colors
- Detect a negative cycle in a Graph | (Bellman Ford)
- Cycles of length n in an undirected and connected graph
- Detecting negative cycle using Floyd Warshall
- Clone a Directed Acyclic Graph
Shortest Paths in Graph
- How to find Shortest Paths from Source to all Vertices using Dijkstra's Algorithm
- Bellman–Ford Algorithm
- Floyd Warshall Algorithm
- Johnson's algorithm for All-pairs shortest paths
- Shortest Path in Directed Acyclic Graph
- Multistage Graph (Shortest Path)
- Shortest path in an unweighted graph
- Karp's minimum mean (or average) weight cycle algorithm
- 0-1 BFS (Shortest Path in a Binary Weight Graph)
- Find minimum weight cycle in an undirected graph
Minimum Spanning Tree in Graph
- Kruskal’s Minimum Spanning Tree (MST) Algorithm
- Difference between Prim's and Kruskal's algorithm for MST
- Applications of Minimum Spanning Tree
- Total number of Spanning Trees in a Graph
- Minimum Product Spanning Tree
- Reverse Delete Algorithm for Minimum Spanning Tree
Topological Sorting in Graph
- Topological Sorting
- All Topological Sorts of a Directed Acyclic Graph
- Kahn's algorithm for Topological Sorting
- Maximum edges that can be added to DAG so that it remains DAG
- Longest Path in a Directed Acyclic Graph
- Topological Sort of a graph using departure time of vertex
Connectivity of Graph
- Articulation Points (or Cut Vertices) in a Graph
- Biconnected Components
- Bridges in a graph
- Eulerian path and circuit for undirected graph
- Fleury's Algorithm for printing Eulerian Path or Circuit
- Strongly Connected Components
- Count all possible walks from a source to a destination with exactly k edges
- Euler Circuit in a Directed Graph
- Word Ladder (Length of shortest chain to reach a target word)
- Find if an array of strings can be chained to form a circle | Set 1
- Tarjan's Algorithm to find Strongly Connected Components
- Paths to travel each nodes using each edge (Seven Bridges of Königsberg)
- Dynamic Connectivity | Set 1 (Incremental)
Maximum flow in a Graph
- Max Flow Problem Introduction
- Ford-Fulkerson Algorithm for Maximum Flow Problem
- Find maximum number of edge disjoint paths between two vertices
- Find minimum s-t cut in a flow network
- Maximum Bipartite Matching
- Channel Assignment Problem
- Introduction to Push Relabel Algorithm
- Introduction and implementation of Karger's algorithm for Minimum Cut
- Dinic's algorithm for Maximum Flow
Some must do problems on Graph
- Find size of the largest region in Boolean Matrix
- Count number of trees in a forest
- A Peterson Graph Problem
- Clone an Undirected Graph
- Introduction to Graph Coloring
Traveling Salesman Problem (TSP) Implementation
- Introduction and Approximate Solution for Vertex Cover Problem
- Erdos Renyl Model (for generating Random Graphs)
- Chinese Postman or Route Inspection | Set 1 (introduction)
- Hierholzer's Algorithm for directed graph
- Boggle (Find all possible words in a board of characters) | Set 1
- Hopcroft–Karp Algorithm for Maximum Matching | Set 1 (Introduction)
- Construct a graph from given degrees of all vertices
- Determine whether a universal sink exists in a directed graph
- Number of sink nodes in a graph
- Two Clique Problem (Check if Graph can be divided in two Cliques)
Travelling Salesman Problem (TSP) : Given a set of cities and distances between every pair of cities, the problem is to find the shortest possible route that visits every city exactly once and returns to the starting point. Note the difference between Hamiltonian Cycle and TSP. The Hamiltonian cycle problem is to find if there exists a tour that visits every city exactly once. Here we know that Hamiltonian Tour exists (because the graph is complete) and in fact, many such tours exist, the problem is to find a minimum weight Hamiltonian Cycle. For example, consider the graph shown in the figure on the right side. A TSP tour in the graph is 1-2-4-3-1. The cost of the tour is 10+25+30+15 which is 80. The problem is a famous NP-hard problem. There is no polynomial-time known solution for this problem.
Examples:
In this post, the implementation of a simple solution is discussed.
- Consider city 1 as the starting and ending point. Since the route is cyclic, we can consider any point as a starting point.
- Generate all (n-1)! permutations of cities.
- Calculate the cost of every permutation and keep track of the minimum cost permutation.
- Return the permutation with minimum cost.
Below is the implementation of the above idea
Time complexity: O(n!) where n is the number of vertices in the graph. This is because the algorithm uses the next_permutation function which generates all the possible permutations of the vertex set. Auxiliary Space: O(n) as we are using a vector to store all the vertices.
Please Login to comment...
Similar reads.
- NP Complete
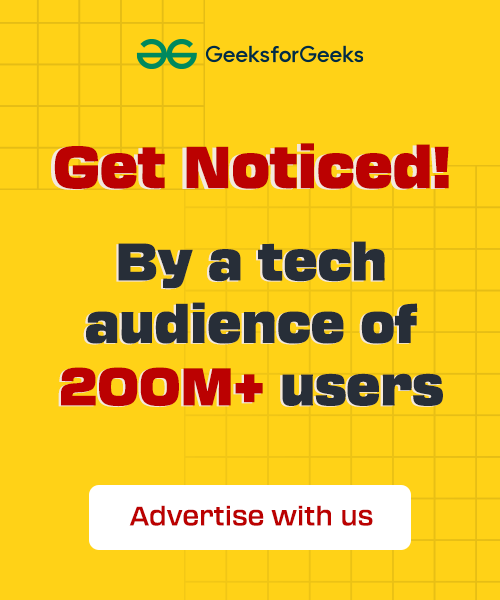
Improve your Coding Skills with Practice
What kind of Experience do you want to share?
To revisit this article, visit My Profile, then View saved stories .
- Backchannel
- Newsletters
- WIRED Insider
- WIRED Consulting
Duncan Geere
Film explores moral and political repercussions of proving P equals NP
What would happen if one government learnt how to bypass the world's encryption systems? That's the all-too-timely question asked by Travelling Salesman , a movie that hands the starring role to a mathematical puzzle.
Its title refers to the "travelling salesman problem" -- given a list of cities and the distances between them all, what's the most efficient way that a salesman can visit them all and return home?
It's easy to check whether a given answer satisfies the criteria, but finding the best solution without trying every single possibility may be impossible. This puzzle, and others like it, are referred to as P vs NP.
In Travelling Salesman , a quartet of mathematicians solve this problem -- they find a way to shortcut the brute-force process of trying every approach, instantaneously negating the way the world's encryption systems operates. They've been hired to do their work by the United States, but they're confronted with the moral quandary of whether to hand over their research to the government or publish it publicly for the whole world's benefit. "We wanted to make a film that felt authentic to a niche group, but also ultimately would be accessible to a wide audience," said Tim Lanzone, one of the movie's creators. "The film is science fiction, but we wanted the science part to be as accurate as possible," his brother and co-writer, Andy Lanzone, added.
It's set almost entirely around a conference table in a sleek, modern, government facility. Initially the four argue over the implications of their research, before a representative of the government arrives and their argument escalates significantly. "I wanted to see if I could contain drama in one room," says Tim Lanzone. "Film school professors always warn that the hardest and most difficult scenes to shoot occur around kitchen tables."
Of course, that makes shooting it cheap, too. The film has a definite DIY aesthetic, but it's impressive what Lanzone has managed to achieve within those constraints. He opted deliberately for a cool colour temperature to make the atmosphere intense and unsettling. "Most of the drama takes place in one room, so we looked to use any sort of additional drama we could harness to our advantage."
That atmosphere is paired with fast-paced and complex dialogue -- it's not a movie that you can put on in the background. Attempts are made to explain the mathematical ideas behind the plot, but you will get more out of it if you've done a little research beforehand. The Lanzones admit this. "Understanding P vs NP directly isn't essential to enjoying the film -- especially if you have some grasp of the implications," says Tim. "We try to simplify it as well as we can during certain scenes but the pace of the dialogue is so fast that a lot of people are just along for the ride."
The movie isn't about the maths, though. Travelling Salesman is a film about power, and how it should be distributed. The government representative's job is to convince the four to sign off their parts of the solution, but it's an uphill struggle as they grapple with the repercussions of what the knowledge would mean for the world.
The bogeyman of China is brought up repeatedly, but it's fascinating to watch the film in light of recent revelations about the extent to which the US and UK's security agencies are already violating global encryption systems through back doors created in many of the world's communication networks.
Matt Jancer
Andy Greenberg
Kathy Gilsinan
Amanda Hoover
The script was written long before Edward Snowden's revelations came to light, but Tim Lanzone says the topic had been on his mind for some time. "I wrote the first draft of the script back in early 2009, which in cyber-time is like an eternity. Back then, articles were really starting to come out more and more highlighting issues related to cyber-security and cyber-espionage -- particularly on important defence targets. It just seemed to be the next cold war-style battleground."
Andy Lanzone adds: "I don't know anyone that foresaw the scope of what the NSA is doing, so that was entirely coincidental. But the more general topic of cyber warfare was an interesting topic that we felt would only become more relevant over time."
The film firmly fails the Bechdel Test in that every one of the characters is white and male, limiting their perspectives considerably. The Lanzones blame this lack of diversity on budget: "We shot the film for under $25,000 and had a whirlwind casting session over two days in LA -- which was all the time we could afford," says Tim. "Knowing we only had about ten days to shoot Travelling Salesman , we were just trying to narrow it down to the five people who could most ably handle the complex language."
Ultimately, you'll get the same amount out of Travelling Salesman that you put into it. It's not a casual watch, but rewards concentration and occasionally pausing the movie and opening Wikipedia to check whether you've understood something right. If you were being cruel, you'd call it an argument for an hour and a half. But the themes and issues it addresses have never been more relevant -- the power of access to private communications, and whether it's right for that power to be concentrated under the auspices of one or a few governments. If you have strong feelings on the matter, on either side, Travelling Salesman is an essential watch.
This article was originally published by WIRED UK
Angela Watercutter
Jennifer M. Wood
Megan Farokhmanesh
What a lovely hat
Is it made out of tin foil , paper 2024/626, exponential quantum speedup for the traveling salesman problem.
The traveling salesman problem is the problem of finding out the shortest route in a network of cities, that a salesman needs to travel to cover all the cities, without visiting the same city more than once. This problem is known to be $NP$-hard with a brute-force complexity of $O(N^N)$ or $O(N^{2N})$ for $N$ number of cities. This problem is equivalent to finding out the shortest Hamiltonian cycle in a given graph, if at least one Hamiltonian cycle exists in it. Quantum algorithms for this problem typically provide with a quadratic speedup only, using Grover's search, thereby having a complexity of $O(N^{N/2})$ or $O(N^N)$. We present a bounded-error quantum polynomial-time (BQP) algorithm for solving the problem, providing with an exponential speedup. The overall complexity of our algorithm is $O(N^3\log(N)\kappa/\epsilon + 1/\epsilon^3)$, where the errors $\epsilon$ are $O(1/{\rm poly}(N))$, and $\kappa$ is the not-too-large condition number of the matrix encoding all Hamiltonian cycles.
Note: 6 pages, 2 figures, comments welcome.
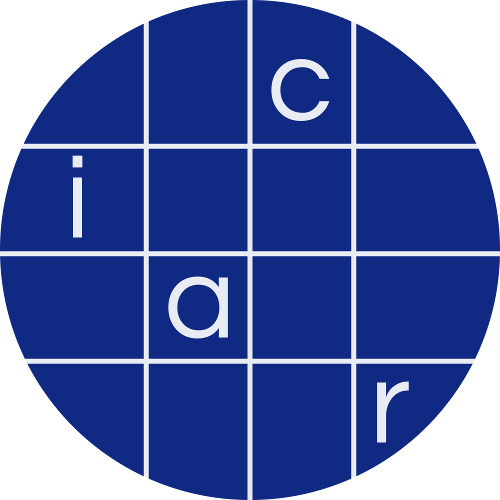

Now Available

"The themes and issues it addresses have never been more relevant ... Travelling Salesman is an essential watch."

" Travelling Salesman ’s mathematicians are all too aware of what their work will do to the world, and watching them argue how to handle the consequences offers a thriller far more cerebral than most."

"Simply unbelievably excellent filmmaking. This is a film to seek out."

"A trip to see this movie might become an obligatory part of all math degrees."

Worldwide Screening Events
New York. Philadelphia. London. Cambridge. Phoenix. Washington D.C. Glasgow. Tel Aviv. Seoul. Hamburg. Hertfordshire. San Francisco. Athens. College Station. Milwaukee. Nanyang. Edinburgh. Ann Arbor.

Winner Best Feature Film
Silicon valley film festival 2012, picture summary.
Travelling Salesman is an intellectual thriller about four mathematicians hired by the U.S. government to solve the most elusive problem in computer science history — P vs. NP. The four have jointly created a "system" which could be the next major advancement for our civilization or destroy the fabric of humanity.
The solution's immediate application would be for theoretical computer science. However, its application would soon extend to countless other disciplines. For example, by utilizing the solution to P vs. NP, a hacker could crack advanced encryption codes within seconds—a task that now takes weeks, months, or even years. He could break into La Guardia's air traffic control or China's communication grid. But the mathematical algorithm would also serve as the basis for accelerated biological research, curing diseases such as AIDS and cancer.
We begin the film with the four at a secret location waiting to meet with a high-ranking official of the United States Department of Defense. The group discusses the global implications of their solution, and they agree that they must be extremely careful with who they allow to control their discovery.
The silver-tongued DoD agent soon arrives and presents them each with an offer of 10 million dollars in exchange for their portion of the algorithmic solution. He attempts to deftly address their concerns and sway the opinions of the four.
In the end, only one mathematician speaks out against selling the solution. In pleading his case, he is forced to reveal the dark truth about his portion of the algorithm. As the mathematicians are about to sign documents that will give the U.S. government sole and private ownership of their solution, they wrestle with the moral dilemma of how this volatile discovery will be used. The math is real. The implications are real.
The Math Behind The Film
The P vs. NP problem is the most notorious unsolved problem in computer science. First introduced in 1971, it asks whether one class of problems (NP) is more difficult than another class (P).
Mathematicians group problems into classes based on how long they take to be solved and verified. " NP " is the class of problems whose answer can be verified in a reasonable amount of time. Some NP problems can also be solved quickly. Those problems are said to be in " P ", which stands for polynomial time. However, there are other problems in NP which have never been solved in polynomial time.
The question is, is it possible to solve all NP problems as quickly as P problems? To date, no one knows for sure. Some NP questions seem harder than P questions, but they may not be.
Currently, many NP problems take a long time to solve. As such, certain problems like logistics scheduling and protein structure prediction are very difficult. Likewise, many cryptosystems, which are used to secure the world's data, rely on the assumption that they cannot be solved in polynomial time.
If someone were to show that NP problems were not difficult—that P and NP problems were the same—it would would have significant practical consequences. Advances in bioinformatics and theoretical chemistry could be made. Much of modern cryptography would be rendered inert. Financial systems would be exposed, leaving the entire Western economy vulnerable.
Proving that P = NP would have enormous ramifications that would be equally enlightening, devastating, and valuable...
"Mathematical puzzles don't often get to star in feature films, but P vs NP is the subject of an upcoming thriller"
"A movie that features science and technology is always welcome, but is it not often we have one that focuses on computer science. Travelling Salesman is just such a rare movie."
"We all know that the P=NP question is truly fascinating, but now it is about to be released as a movie."
"I speak with Timothy about where he got the idea for the movie, how he made sure that the mathematics was correct, and why science movies just may be the new comic book movies."
"At last someone is taking the position that P = NP is a possibility seriously. If nothing else, the film's brain trust realize that being equal is the cool direction, the direction with the most excitement, the most worthy of a major motion picture."
"Travelling Salesman is an unusual movie: despite almost every character being a mathematician there's not a mad person in sight."
Production Stills

Travelling Salesman
About this movie
Ratings and reviews.
- Flag inappropriate
Rate this movie

- Cambridge Dictionary +Plus
Meaning of travelling salesman in English
Your browser doesn't support HTML5 audio
Examples of travelling salesman
Word of the Day
of or relating to birds

Dead ringers and peas in pods (Talking about similarities, Part 2)

Learn more with +Plus
- Recent and Recommended {{#preferredDictionaries}} {{name}} {{/preferredDictionaries}}
- Definitions Clear explanations of natural written and spoken English English Learner’s Dictionary Essential British English Essential American English
- Grammar and thesaurus Usage explanations of natural written and spoken English Grammar Thesaurus
- Pronunciation British and American pronunciations with audio English Pronunciation
- English–Chinese (Simplified) Chinese (Simplified)–English
- English–Chinese (Traditional) Chinese (Traditional)–English
- English–Dutch Dutch–English
- English–French French–English
- English–German German–English
- English–Indonesian Indonesian–English
- English–Italian Italian–English
- English–Japanese Japanese–English
- English–Norwegian Norwegian–English
- English–Polish Polish–English
- English–Portuguese Portuguese–English
- English–Spanish Spanish–English
- English–Swedish Swedish–English
- Dictionary +Plus Word Lists
- Business Noun
- All translations
Add travelling salesman to one of your lists below, or create a new one.
{{message}}
Something went wrong.
There was a problem sending your report.
Log in or sign up for Rotten Tomatoes
Trouble logging in?
By continuing, you agree to the Privacy Policy and the Terms and Policies , and to receive email from the Fandango Media Brands .
By creating an account, you agree to the Privacy Policy and the Terms and Policies , and to receive email from Rotten Tomatoes and to receive email from the Fandango Media Brands .
By creating an account, you agree to the Privacy Policy and the Terms and Policies , and to receive email from Rotten Tomatoes.
Email not verified
Let's keep in touch.
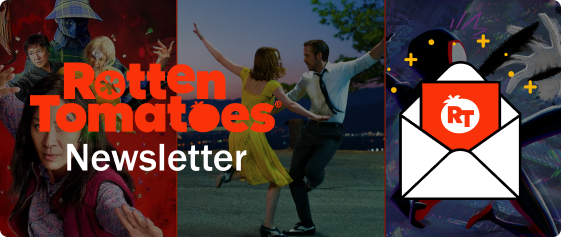
Sign up for the Rotten Tomatoes newsletter to get weekly updates on:
- Upcoming Movies and TV shows
- Trivia & Rotten Tomatoes Podcast
- Media News + More
By clicking "Sign Me Up," you are agreeing to receive occasional emails and communications from Fandango Media (Fandango, Vudu, and Rotten Tomatoes) and consenting to Fandango's Privacy Policy and Terms and Policies . Please allow 10 business days for your account to reflect your preferences.
OK, got it!
Movies / TV
No results found.
- What's the Tomatometer®?
- Login/signup
Movies in theaters
- Opening this week
- Top box office
- Coming soon to theaters
- Certified fresh movies
Movies at home
- Fandango at Home
- Netflix streaming
- Prime Video
- Most popular streaming movies
- What to Watch New
Certified fresh picks
- Challengers Link to Challengers
- Abigail Link to Abigail
- Arcadian Link to Arcadian
New TV Tonight
- Dead Boy Detectives: Season 1
- The Jinx: Season 2
- The Big Door Prize: Season 2
- Knuckles: Season 1
- Them: Season 2
- Velma: Season 2
- Secrets of the Octopus: Season 1
- Thank You, Goodnight: The Bon Jovi Story: Season 1
- We're Here: Season 4
Most Popular TV on RT
- Baby Reindeer: Season 1
- Fallout: Season 1
- Shōgun: Season 1
- Under the Bridge: Season 1
- The Sympathizer: Season 1
- Ripley: Season 1
- 3 Body Problem: Season 1
- X-Men '97: Season 1
- Best TV Shows
- Most Popular TV
- TV & Streaming News
Certified fresh pick
- Baby Reindeer Link to Baby Reindeer
- All-Time Lists
- Binge Guide
- Comics on TV
- Five Favorite Films
- Video Interviews
- Weekend Box Office
- Weekly Ketchup
- What to Watch
DC Animated Movies In Order: How to Watch 54 Original and Universe Films
The Best TV Seasons Certified Fresh at 100%
What to Watch: In Theaters and On Streaming
Awards Tour
Poll: Most Anticipated Movies of May 2024
Poll: Most Anticipated TV and Streaming Shows of May 2024
- Trending on RT
- Challengers
- Play Movie Trivia
Travelling Salesman
Where to watch.
Rent Travelling Salesman on Prime Video, Apple TV, or buy it on Prime Video, Apple TV.
Audience Reviews
Cast & crew.
Timothy Lanzone
Number Four
Marc Raymond
Tyler Seiple
Danny Barclay
No. 1 - Tim Horton
More Like This
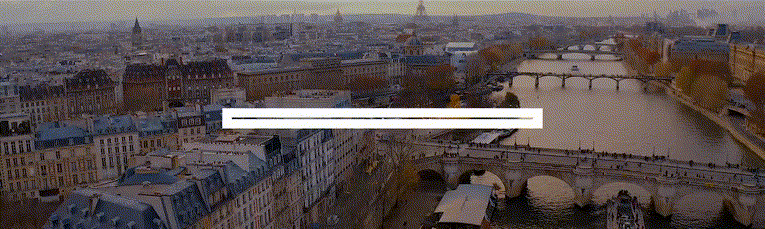
IMAGES
VIDEO
COMMENTS
Norsk bokmål; Polski; ... The travelling salesman problem, also known as the travelling salesperson problem (TSP), asks the following question: "Given a list of cities and the distances between each pair of cities, what is the shortest possible route that visits each city exactly once and returns to the origin city?"
The Traveling Salesman Problem (often called TSP) is a classic algorithmic problem in the field of computer science and operations research. [1] It is focused on optimization. In this context, better solution often means a solution that is cheaper, shorter, or faster. TSP is a mathematical problem. It is most easily expressed as a graph ...
The Travelling Salesman Problem (TSP) is not confined to theoretical mathematics or theoretical computer science either, it shines in real-world applications too. One of its most potent applications resides in the field of logistics and supply chain management.
The Traveling Salesman Problem states that you are a salesperson and you must visit a number of cities or towns. The Traveling Salesman Problem. Rules: Visit every city only once, then return back to the city you started in. Goal: Find the shortest possible route. Except for the Held-Karp algorithm (which is quite advanced and time consuming ...
History Solution to 48 States Traveling Salesman Problem. The origins of the traveling salesman problem are obscure; it is mentioned in an 1832 manual for traveling salesman, which included example tours of 45 German cities but gave no mathematical consideration. 2 W. R. Hamilton and Thomas Kirkman devised mathematical formulations of the problem in the 1800s. 2
The traveling salesperson problem can be modeled as a graph. Specifically, it is typical a directed, weighted graph. Each city acts as a vertex and each path between cities is an edge. Instead of distances, each edge has a weight associated with it. In this model, the goal of the traveling salesperson problem can be defined as finding a path ...
The traveling salesperson problem is one of a handful of foundational problems that theoretical computer scientists turn to again and again to test the limits of efficient computation. The new result "is the first step towards showing that the frontiers of efficient computation are in fact better than what we thought," Williamson said.
In this article, a cost allocation problem that arises in a distribution planning situation atthe Logistics Department at Norsk Hydro Olje AB is studied. A specific tour is considered,for which the total distribution cost is to be divided among the customers that are visited.This problem is formulated as a traveling salesman game, and cost allocation methods basedon different concepts from ...
Figure 12.186 Each door on the route of a traveling salesperson can be represented as a vertex on a graph. (credit: "Three in a row, Heriot Row" by Jason Mason/Flickr, CC BY 2.1) ... exercises to compare the results of the brute force method to the results of the nearest neighbor method for each traveling salesman problem of finding a ...
The traveling salesman was known for his effective and sometimes dubious sales pitches used to draw in a customer. Before brand names and huge company logos, the travelling salesman was the face of small local companies. The travelling salesman usually travelled outside of large cities and into more suburban areas. These salesmen valued the importance...
Travelling Salesman Problem (TSP) : Given a set of cities and distances between every pair of cities, the problem is to find the shortest possible route that visits every city exactly once and returns to the starting point. Note the difference between Hamiltonian Cycle and TSP. The Hamiltonian cycle problem is to find if there exists a tour that visits every city exactly once.
The Traveling Salesman is a 1921 American comedy film starring Fatty Arbuckle. It is based on a 1908 play, The Traveling Salesman, by James Grant Forbes. A 1916 film adaptation of the play starred Frank McIntyre, who had also starred in the play. [1] [2] A print of The Traveling Salesman with German intertitles survives at the George Eastman House.
Travelling Salesman is a film about power, and how it should be distributed. The government representative's job is to convince the four to sign off their parts of the solution, but it's an uphill ...
"Traveling Salesman" was written by Michael Schur, Lee Eisenberg, and Gene Stupnitsky, while co-creator and executive producer Greg Daniels directed. Daniels shot "Traveling Salesmen" and "The Return" in mid-November 2006 before the cast and crew began an eight-week break. John Krasinski enjoyed the episode because he believed it was the first real glimpse of Angela and Dwight's relationship ...
Traveling Salesperson Problem Hamiltonian Cycle Problem Quantum Algorithm Exponential Speedup Contact author(s) anantsharma2410 @ gmail com rajeshdeshpande @ students iisertirupati ac in sanchita ghosh14 @ gmail com sreetama das @ ino cnr it roy shibdas @ gmail com History 2024-04-26: approved 2024-04-23: received See all versions Short URL
Travelling Salesman is an intellectual thriller about four mathematicians hired by the U.S. government to solve the most elusive problem in computer science history — P vs. NP. The four have jointly created a "system" which could be the next major advancement for our civilization or destroy the fabric of humanity.
Four of the world's smartest mathematicians are hired by the U.S. government to solve the most elusive problem in computer science history—P vs. NP. The four have jointly created a "system" which could be the next major advancement for humanity or the downfall of society. As the mathematicians are about to sign documents that will give the government sole and private ownership of their ...
TRAVELLING SALESMAN definition: someone whose job is to travel to different places, trying to persuade people to buy their…. Learn more.
Rent Travelling Salesman on Prime Video, Apple TV, or buy it on Prime Video, Apple TV. The United States government hires four mathematicians to solve the hardest problem in computer science history.
March 10, 1995. Harold Hill, the charming con-artist hero of The Music Man, personifies one of America's enduring icons -- the fast- talking, glad-handing traveling salesman. Not every turn-of ...
Travel from. To. See all options. Search accommodation with Expedia. Duration: 49 min: Distance: 42 mi: Average price: $14: Nearby airports: 5 found: Closest airports to Elektrostal. The nearest airport to Elektrostal is Zhukovsky (ZIA). However, there are better options for getting to Elektrostal. You can take a train from Moscow Domodedovo ...
Norsk nynorsk; Олык марий ... Elektrostal is linked by Elektrichka suburban electric trains to Moscow's Kursky Rail Terminal with a travel time of 1 hour and 20 minutes. Long distance buses link Elektrostal to Noginsk, Moscow and other nearby towns. Local public transport includes buses.
Rome2Rio makes travelling from Elektrostal to Moscow easy. Rome2Rio is a door-to-door travel information and booking engine, helping you get to and from any location in the world. Find all the transport options for your trip from Elektrostal to Moscow right here. Rome2Rio displays up to date schedules, route maps, journey times and estimated ...
Rome2Rio makes travelling from Moscow to Elektrostal easy. Rome2Rio is a door-to-door travel information and booking engine, helping you get to and from any location in the world. Find all the transport options for your trip from Moscow to Elektrostal right here. Rome2Rio displays up to date schedules, route maps, journey times and estimated ...