Forgot password? New user? Sign up
Existing user? Log in
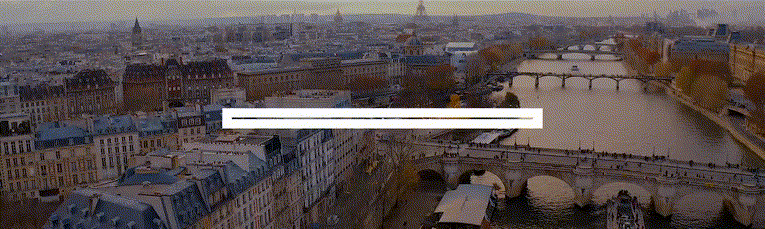
Traveling Salesperson Problem
Already have an account? Log in here.
A salesperson needs to visit a set of cities to sell their goods. They know how many cities they need to go to and the distances between each city. In what order should the salesperson visit each city exactly once so that they minimize their travel time and so that they end their journey in their city of origin?
The traveling salesperson problem is an extremely old problem in computer science that is an extension of the Hamiltonian Circuit Problem . It has important implications in complexity theory and the P versus NP problem because it is an NP-Complete problem . This means that a solution to this problem cannot be found in polynomial time (it takes superpolynomial time to compute an answer). In other words, as the number of vertices increases linearly, the computation time to solve the problem increases exponentially.
The following image is a simple example of a network of cities connected by edges of a specific distance. The origin city is also marked.
Network of cities
Here is the solution for that network, it has a distance traveled of only 14. Any other path that the salesman can takes will result in a path length that is more than 14.
Relationship to Graphs
Special kinds of tsp, importance for p vs np, applications.
The traveling salesperson problem can be modeled as a graph . Specifically, it is typical a directed, weighted graph. Each city acts as a vertex and each path between cities is an edge. Instead of distances, each edge has a weight associated with it. In this model, the goal of the traveling salesperson problem can be defined as finding a path that visits every vertex, returns to the original vertex, and minimizes total weight.
To that end, many graph algorithms can be used on this model. Search algorithms like breadth-first search (BFS) , depth-first search (DFS) , and Dijkstra's shortest path algorithm can certainly be used, however, they do not take into consideration that fact that every vertex must be visited.
The Traveling Salesperson Problem (TSP), an NP-Complete problem, is notoriously complicated to solve. That is because the greedy approach is so computational intensive. The greedy approach to solving this problem would be to try every single possible path and see which one is the fastest. Try this conceptual question to see if you have a grasp for how hard it is to solve.
For a fully connected map with \(n\) cities, how many total paths are possible for the traveling salesperson? Show Answer There are (n-1)! total paths the salesperson can take. The computation needed to solve this problem in this way grows far too quickly to be a reasonable solution. If this map has only 5 cities, there are \(4!\), or 24, paths. However, if the size of this map is increased to 20 cities, there will be \(1.22 \cdot 10^{17}\) paths!
The greedy approach to TSP would go like this:
- Find all possible paths.
- Find the cost of every paths.
- Choose the path with the lowest cost.
Another version of a greedy approach might be: At every step in the algorithm, choose the best possible path. This version might go a little quicker, but it's not guaranteed to find the best answer, or an answer at all since it might hit a dead end.
For NP-Hard problems (a subset of NP-Complete problems) like TSP, exact solutions can only be implemented in a reasonable amount of time for small input sizes (maps with few cities). Otherwise, the best approach we can do is provide a heuristic to help the problem move forward in an optimal way. However, these approaches cannot be proven to be optimal because they always have some sort of downside.
Small input sizes
As described, in a previous section , the greedy approach to this problem has a complexity of \(O(n!)\). However, there are some approaches that decrease this computation time.
The Held-Karp Algorithm is one of the earliest applications of dynamic programming . Its complexity is much lower than the greedy approach at \(O(n^2 2^n)\). Basically what this algorithm says is that every sub path along an optimal path is itself an optimal path. So, computing an optimal path is the same as computing many smaller subpaths and adding them together.
Heuristics are a way of ranking possible next steps in an algorithm in the hopes of cutting down computation time for the entire algorithm. They are often a tradeoff of some attribute - such as completeness, accuracy, or precision - in favor of speed. Heuristics exist for the traveling salesperson problem as well.
The most simple heuristic for this problem is the greedy heuristic. This heuristic simply says, at each step of the network traversal, choose the best next step. In other words, always choose the closest city that you have not yet visited. This heuristic seems like a good one because it is simple and intuitive, and it is even used in practice sometimes, however there are heuristics that are proven to be more effective.
Christofides algorithm is another heuristic. It produces at most 1.5 times the optimal weight for TSP. This algorithm involves finding a minimum spanning tree for the network. Next, it creates matchings for the cities of an odd degree (meaning they have an odd number of edges coming out of them), calculates an eulerian path , and converts back to a TSP path.
Even though it is typically impossible to optimally solve TSP problems, there are cases of TSP problems that can be solved if certain conditions hold.
The metric-TSP is an instance of TSP that satisfies this condition: The distance from city A to city B is less than or equal to the distance from city A to city C plus the distance from city C to city B. Or,
\[distance_{AB} \leq distance_{AC} + distance_{CB}\]
This is a condition that holds in the real world, but it can't always be expected to hold for every TSP problem. But, with this inequality in place, the approximated path will be no more than twice the optimal path. Even better, we can bound the solution to a \(3/2\) approximation by using Christofide's Algorithm .
The euclidean-TSP has an even stricter constraint on the TSP input. It states that all cities' edges in the network must obey euclidean distances . Recent advances have shown that approximation algorithms using euclidean minimum spanning trees have reduced the runtime of euclidean-TSP, even though they are also NP-hard. In practice, though, simpler heuristics are still used.
The P versus NP problem is one of the leading questions in modern computer science. It asks whether or not every problem whose solution can be verified in polynomial time by a computer can also be solved in polynomial time by a computer. TSP, for example, cannot be solved in polynomial time (at least that's what is currently theorized). However, TSP can be solved in polynomial time when it is phrased like this: Given a graph and an integer, x, decide if there is a path of length x or less than x . It's easy to see that given a proposed answer to this question, it is simple to check if it is less than or equal to x.
The traveling salesperson problem, like other problems that are NP-Complete, are very important to this debate. That is because if a polynomial time solution can be found to this problems, then \(P = NP\). As it stands, most scientists believe that \(P \ne NP\).
The traveling salesperson problem has many applications. The obvious ones are in the transportation space. Planning delivery routes or flight patterns, for example, would benefit immensly from breakthroughs is this problem or in the P versus NP problem .
However, this same logic can be applied to many facets of planning as well. In robotics, for instance, planning the order in which to drill holes in a circuit board is a complex task due to the sheer number of holes that must be drawn.
The best and most important application of TSP, however, comes from the fact that it is an NP-Complete problem. That means that its practical applications amount to the applications of any problem that is NP-Complete. So, if there are significant breakthroughs for TSP, that means that those exact same breakthrough can be applied to any problem in the NP-Complete class.
Problem Loading...
Note Loading...
Set Loading...
12.9 Traveling Salesperson Problem
Learning objectives.
After completing this section, you should be able to:
- Distinguish between brute force algorithms and greedy algorithms.
- List all distinct Hamilton cycles of a complete graph.
- Apply brute force method to solve traveling salesperson applications.
- Apply nearest neighbor method to solve traveling salesperson applications.
We looked at Hamilton cycles and paths in the previous sections Hamilton Cycles and Hamilton Paths . In this section, we will analyze Hamilton cycles in complete weighted graphs to find the shortest route to visit a number of locations and return to the starting point. Besides the many routing applications in which we need the shortest distance, there are also applications in which we search for the route that is least expensive or takes the least time. Here are a few less common applications that you can read about on a website set up by the mathematics department at the University of Waterloo in Ontario, Canada:
- Design of fiber optic networks
- Minimizing fuel expenses for repositioning satellites
- Development of semi-conductors for microchips
- A technique for mapping mammalian chromosomes in genome sequencing
Before we look at approaches to solving applications like these, let's discuss the two types of algorithms we will use.
Brute Force and Greedy Algorithms
An algorithm is a sequence of steps that can be used to solve a particular problem. We have solved many problems in this chapter, and the procedures that we used were different types of algorithms. In this section, we will use two common types of algorithms, a brute force algorithm and a greedy algorithm . A brute force algorithm begins by listing every possible solution and applying each one until the best solution is found. A greedy algorithm approaches a problem in stages, making the apparent best choice at each stage, then linking the choices together into an overall solution which may or may not be the best solution.
To understand the difference between these two algorithms, consider the tree diagram in Figure 12.187 . Suppose we want to find the path from left to right with the largest total sum. For example, branch A in the tree diagram has a sum of 10 + 2 + 11 + 13 = 36 10 + 2 + 11 + 13 = 36 .
To be certain that you pick the branch with greatest sum, you could list each sum from each of the different branches:
A : 10 + 2 + 11 + 13 = 36 10 + 2 + 11 + 13 = 36
B : 10 + 2 + 11 + 8 = 31 10 + 2 + 11 + 8 = 31
C : 10 + 2 + 15 + 1 = 28 10 + 2 + 15 + 1 = 28
D : 10 + 2 + 15 + 6 = 33 10 + 2 + 15 + 6 = 33
E : 10 + 7 + 3 + 20 = 40 10 + 7 + 3 + 20 = 40
F : 10 + 7 + 3 + 14 = 34 10 + 7 + 3 + 14 = 34
G : 10 + 7 + 4 + 11 = 32 10 + 7 + 4 + 11 = 32
H : 10 + 7 + 4 + 5 = 26 10 + 7 + 4 + 5 = 26
Then we know with certainty that branch E has the greatest sum.
Now suppose that you wanted to find the branch with the highest value, but you only were shown the tree diagram in phases, one step at a time.
After phase 1, you would have chosen the branch with 10 and 7. So far, you are following the same branch. Let’s look at the next phase.
After phase 2, based on the information you have, you will choose the branch with 10, 7 and 4. Now, you are following a different branch than before, but it is the best choice based on the information you have. Let’s look at the last phase.
After phase 3, you will choose branch G which has a sum of 32.
The process of adding the values on each branch and selecting the highest sum is an example of a brute force algorithm because all options were explored in detail. The process of choosing the branch in phases, based on the best choice at each phase is a greedy algorithm. Although a brute force algorithm gives us the ideal solution, it can take a very long time to implement. Imagine a tree diagram with thousands or even millions of branches. It might not be possible to check all the sums. A greedy algorithm, on the other hand, can be completed in a relatively short time, and generally leads to good solutions, but not necessarily the ideal solution.
Example 12.42
Distinguishing between brute force and greedy algorithms.
A cashier rings up a sale for $4.63 cents in U.S. currency. The customer pays with a $5 bill. The cashier would like to give the customer $0.37 in change using the fewest coins possible. The coins that can be used are quarters ($0.25), dimes ($0.10), nickels ($0.05), and pennies ($0.01). The cashier starts by selecting the coin of highest value less than or equal to $0.37, which is a quarter. This leaves $ 0.37 − $ 0.25 = $ 0.12 $ 0.37 − $ 0.25 = $ 0.12 . The cashier selects the coin of highest value less than or equal to $0.12, which is a dime. This leaves $ 0.12 − $ 0.10 = $ 0.02 $ 0.12 − $ 0.10 = $ 0.02 . The cashier selects the coin of highest value less than or equal to $0.02, which is a penny. This leaves $ 0.02 − $ 0.01 = $ 0.01 $ 0.02 − $ 0.01 = $ 0.01 . The cashier selects the coin of highest value less than or equal to $0.01, which is a penny. This leaves no remainder. The cashier used one quarter, one dime, and two pennies, which is four coins. Use this information to answer the following questions.
- Is the cashier’s approach an example of a greedy algorithm or a brute force algorithm? Explain how you know.
- The cashier’s solution is the best solution. In other words, four is the fewest number of coins possible. Is this consistent with the results of an algorithm of this kind? Explain your reasoning.
- The approach the cashier used is an example of a greedy algorithm, because the problem was approached in phases and the best choice was made at each phase. Also, it is not a brute force algorithm, because the cashier did not attempt to list out all possible combinations of coins to reach this conclusion.
- Yes, it is consistent. A greedy algorithm does not always yield the best result, but sometimes it does.
Your Turn 12.42
The traveling salesperson problem.
Now let’s focus our attention on the graph theory application known as the traveling salesperson problem (TSP) in which we must find the shortest route to visit a number of locations and return to the starting point.
Recall from Hamilton Cycles , the officer in the U.S. Air Force who is stationed at Vandenberg Air Force base and must drive to visit three other California Air Force bases before returning to Vandenberg. The officer needed to visit each base once. We looked at the weighted graph in Figure 12.192 representing the four U.S. Air Force bases: Vandenberg, Edwards, Los Angeles, and Beal and the distances between them.
Any route that visits each base and returns to the start would be a Hamilton cycle on the graph. If the officer wants to travel the shortest distance, this will correspond to a Hamilton cycle of lowest weight. We saw in Table 12.11 that there are six distinct Hamilton cycles (directed cycles) in a complete graph with four vertices, but some lie on the same cycle (undirected cycle) in the graph.
Since the distance between bases is the same in either direction, it does not matter if the officer travels clockwise or counterclockwise. So, there are really only three possible distances as shown in Figure 12.193 .
The possible distances are:
So, a Hamilton cycle of least weight is V → B → E → L → V (or the reverse direction). The officer should travel from Vandenberg to Beal to Edwards, to Los Angeles, and back to Vandenberg.
Finding Weights of All Hamilton Cycles in Complete Graphs
Notice that we listed all of the Hamilton cycles and found their weights when we solved the TSP about the officer from Vandenberg. This is a skill you will need to practice. To make sure you don't miss any, you can calculate the number of possible Hamilton cycles in a complete graph. It is also helpful to know that half of the directed cycles in a complete graph are the same cycle in reverse direction, so, you only have to calculate half the number of possible weights, and the rest are duplicates.
In a complete graph with n n vertices,
- The number of distinct Hamilton cycles is ( n − 1 ) ! ( n − 1 ) ! .
- There are at most ( n − 1 ) ! 2 ( n − 1 ) ! 2 different weights of Hamilton cycles.
TIP! When listing all the distinct Hamilton cycles in a complete graph, you can start them all at any vertex you choose. Remember, the cycle a → b → c → a is the same cycle as b → c → a → b so there is no need to list both.
Example 12.43
Calculating possible weights of hamilton cycles.
Suppose you have a complete weighted graph with vertices N, M, O , and P .
- Use the formula ( n − 1 ) ! ( n − 1 ) ! to calculate the number of distinct Hamilton cycles in the graph.
- Use the formula ( n − 1 ) ! 2 ( n − 1 ) ! 2 to calculate the greatest number of different weights possible for the Hamilton cycles.
- Are all of the distinct Hamilton cycles listed here? How do you know? Cycle 1: N → M → O → P → N Cycle 2: N → M → P → O → N Cycle 3: N → O → M → P → N Cycle 4: N → O → P → M → N Cycle 5: N → P → M → O → N Cycle 6: N → P → O → M → N
- Which pairs of cycles must have the same weights? How do you know?
- There are 4 vertices; so, n = 4 n = 4 . This means there are ( n − 1 ) ! = ( 4 − 1 ) ! = 3 ⋅ 2 ⋅ 1 = 6 ( n − 1 ) ! = ( 4 − 1 ) ! = 3 ⋅ 2 ⋅ 1 = 6 distinct Hamilton cycles beginning at any given vertex.
- Since n = 4 n = 4 , there are ( n − 1 ) ! 2 = ( 4 − 1 ) ! 2 = 6 2 = 3 ( n − 1 ) ! 2 = ( 4 − 1 ) ! 2 = 6 2 = 3 possible weights.
- Yes, they are all distinct cycles and there are 6 of them.
- Cycles 1 and 6 have the same weight, Cycles 2 and 4 have the same weight, and Cycles 3 and 5 have the same weight, because these pairs follow the same route through the graph but in reverse.
TIP! When listing the possible cycles, ignore the vertex where the cycle begins and ends and focus on the ways to arrange the letters that represent the vertices in the middle. Using a systematic approach is best; for example, if you must arrange the letters M, O, and P, first list all those arrangements beginning with M, then beginning with O, and then beginning with P, as we did in Example 12.42.
Your Turn 12.43
The brute force method.
The method we have been using to find a Hamilton cycle of least weight in a complete graph is a brute force algorithm, so it is called the brute force method . The steps in the brute force method are:
Step 1: Calculate the number of distinct Hamilton cycles and the number of possible weights.
Step 2: List all possible Hamilton cycles.
Step 3: Find the weight of each cycle.
Step 4: Identify the Hamilton cycle of lowest weight.
Example 12.44
Applying the brute force method.
On the next assignment, the air force officer must leave from Travis Air Force base, visit Beal, Edwards, and Vandenberg Air Force bases each exactly once and return to Travis Air Force base. There is no need to visit Los Angeles Air Force base. Use Figure 12.194 to find the shortest route.
Step 1: Since there are 4 vertices, there will be ( 4 − 1 ) ! = 3 ! = 6 ( 4 − 1 ) ! = 3 ! = 6 cycles, but half of them will be the reverse of the others; so, there will be ( 4 − 1 ) ! 2 = 6 2 = 3 ( 4 − 1 ) ! 2 = 6 2 = 3 possible distances.
Step 2: List all the Hamilton cycles in the subgraph of the graph in Figure 12.195 .
To find the 6 cycles, focus on the three vertices in the middle, B, E, and V . The arrangements of these vertices are BEV, BVE, EBV, EVB, VBE , and VEB . These would correspond to the 6 cycles:
1: T → B → E → V → T
2: T → B → V → E → T
3: T → E → B → V → T
4: T → E → V → B → T
5: T → V → B → E → T
6: T → V → E → B → T
Step 3: Find the weight of each path. You can reduce your work by observing the cycles that are reverses of each other.
1: 84 + 410 + 207 + 396 = 1097 84 + 410 + 207 + 396 = 1097
2: 84 + 396 + 207 + 370 = 1071 84 + 396 + 207 + 370 = 1071
3: 370 + 410 + 396 + 396 = 1572 370 + 410 + 396 + 396 = 1572
4: Reverse of cycle 2, 1071
5: Reverse of cycle 3, 1572
6: Reverse of cycle 1, 1097
Step 4: Identify a Hamilton cycle of least weight.
The second path, T → B → V → E → T , and its reverse, T → E → V → B → T , have the least weight. The solution is that the officer should travel from Travis Air Force base to Beal Air Force Base, to Vandenberg Air Force base, to Edwards Air Force base, and return to Travis Air Force base, or the same route in reverse.
Your Turn 12.44
Now suppose that the officer needed a cycle that visited all 5 of the Air Force bases in Figure 12.194 . There would be ( 5 − 1 ) ! = 4 ! = 4 × 3 × 2 × 1 = 24 ( 5 − 1 ) ! = 4 ! = 4 × 3 × 2 × 1 = 24 different arrangements of vertices and ( 5 − 1 ) ! 2 = 4 ! 2 = 24 2 = 12 ( 5 − 1 ) ! 2 = 4 ! 2 = 24 2 = 12 distances to compare using the brute force method. If you consider 10 Air Force bases, there would be ( 10 − 1 ) ! = 9 ! = 9 ⋅ 8 ⋅ 7 ⋅ 6 ⋅ 5 ⋅ 4 ⋅ 3 ⋅ 2 ⋅ 1 = 362 , 880 ( 10 − 1 ) ! = 9 ! = 9 ⋅ 8 ⋅ 7 ⋅ 6 ⋅ 5 ⋅ 4 ⋅ 3 ⋅ 2 ⋅ 1 = 362 , 880 different arrangements and ( 10 − 1 ) ! 2 = 9 ! 2 = 9 ⋅ 8 ⋅ 7 ⋅ 6 ⋅ 5 ⋅ 4 ⋅ 3 ⋅ 2 ⋅ 1 2 = 181 , 440 ( 10 − 1 ) ! 2 = 9 ! 2 = 9 ⋅ 8 ⋅ 7 ⋅ 6 ⋅ 5 ⋅ 4 ⋅ 3 ⋅ 2 ⋅ 1 2 = 181 , 440 distances to consider. There must be another way!
The Nearest Neighbor Method
When the brute force method is impractical for solving a traveling salesperson problem, an alternative is a greedy algorithm known as the nearest neighbor method , which always visit the closest or least costly place first. This method finds a Hamilton cycle of relatively low weight in a complete graph in which, at each phase, the next vertex is chosen by comparing the edges between the current vertex and the remaining vertices to find the lowest weight. Since the nearest neighbor method is a greedy algorithm, it usually doesn’t give the best solution, but it usually gives a solution that is "good enough." Most importantly, the number of steps will be the number of vertices. That’s right! A problem with 10 vertices requires 10 steps, not 362,880. Let’s look at an example to see how it works.
Suppose that a candidate for governor wants to hold rallies around the state. They plan to leave their home in city A , visit cities B, C, D, E , and F each once, and return home. The airfare between cities is indicated in the graph in Figure 12.196 .
Let’s help the candidate keep costs of travel down by applying the nearest neighbor method to find a Hamilton cycle that has a reasonably low weight. Begin by marking starting vertex as V 1 V 1 for "visited 1st." Then to compare the weights of the edges between A and vertices adjacent to A : $250, $210, $300, $200, and $100 as shown in Figure 12.197 . The lowest of these is $100, which is the edge between A and F .
Mark F as V 2 V 2 for "visited 2nd" then compare the weights of the edges between F and the remaining vertices adjacent to F : $170, $330, $150 and $350 as shown in Figure 12.198 . The lowest of these is $150, which is the edge between F and D .
Mark D as V 3 V 3 for "visited 3rd." Next, compare the weights of the edges between D and the remaining vertices adjacent to D : $120, $310, and $270 as shown in Figure 12.199 . The lowest of these is $120, which is the edge between D and B .
So, mark B as V 4 V 4 for "visited 4th." Finally, compare the weights of the edges between B and the remaining vertices adjacent to B : $160 and $220 as shown in Figure 12.200 . The lower amount is $160, which is the edge between B and E .
Now you can mark E as V 5 V 5 and mark the only remaining vertex, which is C , as V 6 V 6 . This is shown in Figure 12.201 . Make a note of the weight of the edge from E to C , which is $180, and from C back to A , which is $210.
The Hamilton cycle we found is A → F → D → B → E → C → A . The weight of the circuit is $ 100 + $ 150 + $ 120 + $ 160 + $ 180 + $ 210 = $ 920 $ 100 + $ 150 + $ 120 + $ 160 + $ 180 + $ 210 = $ 920 . This may or may not be the route with the lowest cost, but there is a good chance it is very close since the weights are most of the lowest weights on the graph and we found it in six steps instead of finding 120 different Hamilton cycles and calculating 60 weights. Let’s summarize the procedure that we used.
Step 1: Select the starting vertex and label V 1 V 1 for "visited 1st." Identify the edge of lowest weight between V 1 V 1 and the remaining vertices.
Step 2: Label the vertex at the end of the edge of lowest weight that you found in previous step as V n V n where the subscript n indicates the order the vertex is visited. Identify the edge of lowest weight between V n V n and the vertices that remain to be visited.
Step 3: If vertices remain that have not been visited, repeat Step 2. Otherwise, a Hamilton cycle of low weight is V 1 → V 2 → ⋯ → V n → V 1 V 1 → V 2 → ⋯ → V n → V 1 .
Example 12.45
Using the nearest neighbor method.
Suppose that the candidate for governor wants to hold rallies around the state but time before the election is very limited. They would like to leave their home in city A , visit cities B , C , D , E , and F each once, and return home. The airfare between cities is not as important as the time of travel, which is indicated in Figure 12.202 . Use the nearest neighbor method to find a route with relatively low travel time. What is the total travel time of the route that you found?
Step 1: Label vertex A as V 1 V 1 . The edge of lowest weight between A and the remaining vertices is 85 min between A and D .
Step 2: Label vertex D as V 2 V 2 . The edge of lowest weight between D and the vertices that remain to be visited, B, C, E , and F , is 70 min between D and F .
Repeat Step 2: Label vertex F as V 3 V 3 . The edge of lowest weight between F and the vertices that remain to be visited, B, C, and E , is 75 min between F and C .
Repeat Step 2: Label vertex C as V 4 V 4 . The edge of lowest weight between C and the vertices that remain to be visited, B and E , is 100 min between C and B .
Repeat Step 2: Label vertex B as V 5 V 5 . The only vertex that remains to be visited is E . The weight of the edge between B and E is 95 min.
Step 3: A Hamilton cycle of low weight is A → D → F → C → B → E → A . So, a route of relatively low travel time is A to D to F to C to B to E and back to A . The total travel time of this route is: 85 min + 70 min + 75 min + 100 min + 95 min + 90 min = 515 min or 8 hrs 35 min 85 min + 70 min + 75 min + 100 min + 95 min + 90 min = 515 min or 8 hrs 35 min
Your Turn 12.45
Check your understanding, section 12.9 exercises.
As an Amazon Associate we earn from qualifying purchases.
This book may not be used in the training of large language models or otherwise be ingested into large language models or generative AI offerings without OpenStax's permission.
Want to cite, share, or modify this book? This book uses the Creative Commons Attribution License and you must attribute OpenStax.
Access for free at https://openstax.org/books/contemporary-mathematics/pages/1-introduction
- Authors: Donna Kirk
- Publisher/website: OpenStax
- Book title: Contemporary Mathematics
- Publication date: Mar 22, 2023
- Location: Houston, Texas
- Book URL: https://openstax.org/books/contemporary-mathematics/pages/1-introduction
- Section URL: https://openstax.org/books/contemporary-mathematics/pages/12-9-traveling-salesperson-problem
© Dec 21, 2023 OpenStax. Textbook content produced by OpenStax is licensed under a Creative Commons Attribution License . The OpenStax name, OpenStax logo, OpenStax book covers, OpenStax CNX name, and OpenStax CNX logo are not subject to the Creative Commons license and may not be reproduced without the prior and express written consent of Rice University.
Traveling salesman problem
This web page is a duplicate of https://optimization.mccormick.northwestern.edu/index.php/Traveling_salesman_problems
Author: Jessica Yu (ChE 345 Spring 2014)
Steward: Dajun Yue, Fengqi You
The traveling salesman problem (TSP) is a widely studied combinatorial optimization problem, which, given a set of cities and a cost to travel from one city to another, seeks to identify the tour that will allow a salesman to visit each city only once, starting and ending in the same city, at the minimum cost. 1
- 2.1 Graph Theory
- 2.2 Classifications of the TSP
- 2.3 Variations of the TSP
- 3.1 aTSP ILP Formulation
- 3.2 sTSP ILP Formulation
- 4.1 Exact algorithms
- 4.2.1 Tour construction procedures
- 4.2.2 Tour improvement procedures
- 5 Applications
- 7 References
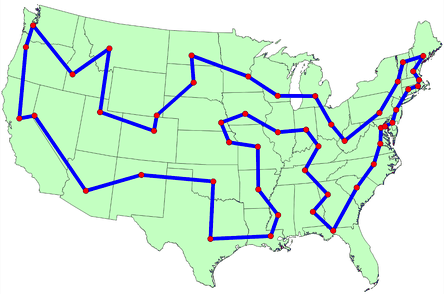
The origins of the traveling salesman problem are obscure; it is mentioned in an 1832 manual for traveling salesman, which included example tours of 45 German cities but gave no mathematical consideration. 2 W. R. Hamilton and Thomas Kirkman devised mathematical formulations of the problem in the 1800s. 2
It is believed that the general form was first studied by Karl Menger in Vienna and Harvard in the 1930s. 2,3
Hassler Whitney, who was working on his Ph.D. research at Harvard when Menger was a visiting lecturer, is believed to have posed the problem of finding the shortest route between the 48 states of the United States during either his 1931-1932 or 1934 seminar talks. 2 There is also uncertainty surrounding the individual who coined the name “traveling salesman problem” for Whitney’s problem. 2
The problem became increasingly popular in the 1950s and 1960s. Notably, George Dantzig, Delber R. Fulkerson, and Selmer M. Johnson at the RAND Corporation in Santa Monica, California solved the 48 state problem by formulating it as a linear programming problem. 2 The methods described in the paper set the foundation for future work in combinatorial optimization, especially highlighting the importance of cutting planes. 2,4
In the early 1970s, the concept of P vs. NP problems created buzz in the theoretical computer science community. In 1972, Richard Karp demonstrated that the Hamiltonian cycle problem was NP-complete, implying that the traveling salesman problem was NP-hard. 4
Increasingly sophisticated codes led to rapid increases in the sizes of the traveling salesman problems solved. Dantzig, Fulkerson, and Johnson had solved a 48 city instance of the problem in 1954. 5 Martin Grötechel more than doubled this 23 years later, solving a 120 city instance in 1977. 5 Enoch Crowder and Manfred W. Padberg again more than doubled this in just 3 years, with a 318 city solution. 5
In 1987, rapid improvements were made, culminating in a 2,392 city solution by Padberg and Giovanni Rinaldi. In the following two decades, David L. Appelgate, Robert E. Bixby, Vasek Chvátal, & William J. Cook led the cutting edge, solving a 7,397 city instance in 1994 up to the current largest solved problem of 24,978 cities in 2004. 5
Description
Graph theory.
In the context of the traveling salesman problem, the verticies correspond to cities and the edges correspond to the path between those cities. When modeled as a complete graph, paths that do not exist between cities can be modeled as edges of very large cost without loss of generality. 6 Minimizing the sum of the costs for Hamiltonian cycle is equivalent to identifying the shortest path in which each city is visiting only once.
Classifications of the TSP
The TRP can be divided into two classes depending on the nature of the cost matrix. 3,6
- Applies when the distance between cities is the same in both directions
- Applies when there are differences in distances (e.g. one-way streets)
An ATSP can be formulated as an STSP by doubling the number of nodes. 6
Variations of the TSP
Formulation
The objective function is then given by
To ensure that the result is a valid tour, several contraints must be added. 1,3
There are several other formulations for the subtour elimnation contraint, including circuit packing contraints, MTZ constraints, and network flow constraints.
aTSP ILP Formulation
The integer linear programming formulation for an aTSP is given by
sTSP ILP Formulation
The symmetric case is a special case of the asymmetric case and the above formulation is valid. 3, 6 The integer linear programming formulation for an sTSP is given by
Exact algorithms
Branch-and-bound algorithms are commonly used to find solutions for TSPs. 7 The ILP is first relaxed and solved as an LP using the Simplex method, then feasibility is regained by enumeration of the integer variables. 7
Other exact solution methods include the cutting plane method and branch-and-cut. 8
Heuristic algorithms
Given that the TSP is an NP-hard problem, heuristic algorithms are commonly used to give a approximate solutions that are good, though not necessarily optimal. The algorithms do not guarantee an optimal solution, but gives near-optimal solutions in reasonable computational time. 3 The Held-Karp lower bound can be calculated and used to judge the performance of a heuristic algorithm. 3
There are two general heuristic classifications 7 :
- Tour construction procedures where a solution is gradually built by adding a new vertex at each step
- Tour improvement procedures where a feasbile solution is improved upon by performing various exchanges
The best methods tend to be composite algorithms that combine these features. 7
Tour construction procedures
Tour improvement procedures
Applications
The importance of the traveling salesman problem is two fold. First its ubiquity as a platform for the study of general methods than can then be applied to a variety of other discrete optimization problems. 5 Second is its diverse range of applications, in fields including mathematics, computer science, genetics, and engineering. 5,6
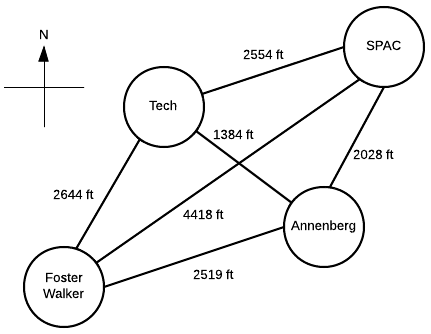
Suppose a Northwestern student, who lives in Foster-Walker , has to accomplish the following tasks:
- Drop off a homework set at Tech
- Work out a SPAC
- Complete a group project at Annenberg
Distances between buildings can be found using Google Maps. Note that there is particularly strong western wind and walking east takes 1.5 times as long.
It is the middle of winter and the student wants to spend the least possible time walking. Determine the path the student should take in order to minimize walking time, starting and ending at Foster-Walker.
Start with the cost matrix (with altered distances taken into account):
Method 1: Complete Enumeration
All possible paths are considered and the path of least cost is the optimal solution. Note that this method is only feasible given the small size of the problem.
From inspection, we see that Path 4 is the shortest. So, the student should walk 2.28 miles in the following order: Foster-Walker → Annenberg → SPAC → Tech → Foster-Walker
Method 2: Nearest neighbor
Starting from Foster-Walker, the next building is simply the closest building that has not yet been visited. With only four nodes, this can be done by inspection:
- Smallest distance is from Foster-Walker is to Annenberg
- Smallest distance from Annenberg is to Tech
- Smallest distance from Tech is to Annenberg ( creates a subtour, therefore skip )
- Next smallest distance from Tech is to Foster-Walker ( creates a subtour, therefore skip )
- Next smallest distance from Tech is to SPAC
- Smallest distance from SPAC is to Annenberg ( creates a subtour, therefore skip )
- Next smallest distance from SPAC is to Tech ( creates a subtour, therefore skip )
- Next smallest distance from SPAC is to Foster-Walker
So, the student would walk 2.54 miles in the following order: Foster-Walker → Annenberg → Tech → SPAC → Foster-Walker
Method 3: Greedy
With this method, the shortest paths that do not create a subtour are selected until a complete tour is created.
- Smallest distance is Annenberg → Tech
- Next smallest is SPAC → Annenberg
- Next smallest is Tech → Annenberg ( creates a subtour, therefore skip )
- Next smallest is Anneberg → Foster-Walker ( creates a subtour, therefore skip )
- Next smallest is SPAC → Tech ( creates a subtour, therefore skip )
- Next smallest is Tech → Foster-Walker
- Next smallest is Annenberg → SPAC ( creates a subtour, therefore skip )
- Next smallest is Foster-Walker → Annenberg ( creates a subtour, therefore skip )
- Next smallest is Tech → SPAC ( creates a subtour, therefore skip )
- Next smallest is Foster-Walker → Tech ( creates a subtour, therefore skip )
- Next smallest is SPAC → Foster-Walker ( creates a subtour, therefore skip )
- Next smallest is Foster-Walker → SPAC
So, the student would walk 2.40 miles in the following order: Foster-Walker → SPAC → Annenberg → Tech → Foster-Walker
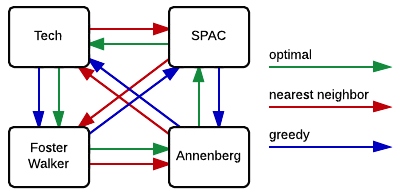
As we can see in the figure to the right, the heuristic methods did not give the optimal solution. That is not to say that heuristics can never give the optimal solution, just that it is not guaranteed.
Both the optimal and the nearest neighbor algorithms suggest that Annenberg is the optimal first building to visit. However, the optimal solution then goes to SPAC, while both heuristic methods suggest Tech. This is in part due to the large cost of SPAC → Foster-Walker. The heuristic algorithms cannot take this future cost into account, and therefore fall into that local optimum.
We note that the nearest neighbor and greedy algorithms give solutions that are 11.4% and 5.3%, respectively, above the optimal solution. In the scale of this problem, this corresponds to fractions of a mile. We also note that neither heuristic gave the worst case result, Foster-Walker → SPAC → Tech → Annenberg → Foster-Walker.
Only tour building heuristics were used. Combined with a tour improvement algorithm (such as 2-opt or simulated annealing), we imagine that we may be able to locate solutions that are closer to the optimum.
The exact algorithm used was complete enumeration, but we note that this is impractical even for 7 nodes (6! or 720 different possibilities). Commonly, the problem would be formulated and solved as an ILP to obtain exact solutions.
- Vanderbei, R. J. (2001). Linear programming: Foundations and extensions (2nd ed.). Boston: Kluwer Academic.
- Schrijver, A. (n.d.). On the history of combinatorial optimization (till 1960).
- Matai, R., Singh, S., & Lal, M. (2010). Traveling salesman problem: An overview of applications, formulations, and solution approaches. In D. Davendra (Ed.), Traveling Salesman Problem, Theory and Applications . InTech.
- Junger, M., Liebling, T., Naddef, D., Nemhauser, G., Pulleyblank, W., Reinelt, G., Rinaldi, G., & Wolsey, L. (Eds.). (2009). 50 years of integer programming, 1958-2008: The early years and state-of-the-art surveys . Heidelberg: Springer.
- Cook, W. (2007). History of the TSP. The Traveling Salesman Problem . Retrieved from http://www.math.uwaterloo.ca/tsp/history/index.htm
- Punnen, A. P. (2002). The traveling salesman problem: Applications, formulations and variations. In G. Gutin & A. P. Punnen (Eds.), The Traveling Salesman Problem and its Variations . Netherlands: Kluwer Academic Publishers.
- Laporte, G. (1992). The traveling salesman problem: An overview of exact and approximate algorithms. European Journal of Operational Research, 59 (2), 231–247.
- Goyal, S. (n.d.). A suvey on travlling salesman problem.
Navigation menu

- Coding Problems
Travelling Salesman Problem (TSP)
Problem statement, example 1: travelling salesman problem, example 2: travelling salesman problem, 1. simple approach, c++ code implementation, java code implementation, python code implementation, 2. travelling salesman problem using dynamic programming, c code implementation, 3. greedy approach, practice questions, frequently asked questions, 1. which algorithm is used for the travelling salesman problem, 2. what is the complexity of the travelling salesman problem, 3. how is this problem modelled as a graph problem, 4: what is the difficulty level of the travelling salesman problem.
Travelling Salesman Problem (TSP) – Given a set of cities and the distance between every pair of cities as an adjacency matrix, the problem is to find the shortest possible route that visits every city exactly once and returns to the starting point. The ultimate goal is to minimize the total distance travelled, forming a closed tour or circuit.
The TSP is referred to as an NP-hard problem, meaning there is no known algorithm to solve it in polynomial time for large instances. As the number of cities increases, the number of potential solutions grows exponentially, making an exhaustive search unfeasible. This complexity is one of the reasons why the TSP remains a popular topic of research. Learn More .
Input –
Confused about your next job?
Output –
Here, the TSP Tour is 0-2-1-3-0 and the cost of the tour is 48.
Minimum weight Hamiltonian Cycle : EACBDE= 32
Wondering how the Hamiltonian Cycle Problem and the Traveling Salesman Problem differ? The Hamiltonian Cycle problem is to find out if there exists a tour that visits each city exactly once. Here, we know that the Hamiltonian Tour exists (due to the graph being complete), and there are indeed many such tours. The problem is to find a minimum weight Hamiltonian Cycle.
There are various approaches to finding the solution to the travelling salesman problem- simple (naïve) approach, dynamic programming approach, and greedy approach. Let’s explore each approach in detail:
- Consider city 1 as the starting and ending point. Since the route is cyclic, we can consider any point as a starting point.
- Now, we will generate all possible permutations of cities which are (n-1)!.
- Find the cost of each permutation and keep track of the minimum cost permutation.
- Return the permutation with minimum cost.
- Time complexity: O(N!), Where N is the number of cities.
- Space complexity: O(1).
In the travelling salesman problem algorithm, we take a subset N of the required cities that need to be visited, the distance among the cities dist, and starting cities s as inputs. Each city is identified by a unique city id which we say like 1,2,3,4,5………n
Here we use a dynamic approach to calculate the cost function Cost(). Using recursive calls, we calculate the cost function for each subset of the original problem.
To calculate the cost(i) using Dynamic Programming , we need to have some recursive relation in terms of sub-problems.
We start with all subsets of size 2 and calculate C(S, i) for all subsets where S is the subset, then we calculate C(S, i) for all subsets S of size 3 and so on.
There are at most O(n2^n) subproblems, and each one takes linear time to solve. The total running time is, therefore, O(n^22^n). The time complexity is much less than O(n!) but still exponential. The space required is also exponential.
- Time Complexity: O(N^2*2^N).
- First of them is a list that can hold the indices of the cities in terms of the input matrix of distances between cities
- And the Second one is the array which is our result
- Perform traversal on the given adjacency matrix tsp[][] for all the cities and if the cost of reaching any city from the current city is less than the current cost update the cost.
- Generate the minimum path cycle using the above step and return their minimum cost.
- Time complexity: O(N^2*logN), Where N is the number of cities.
- Space complexity: O(N).
- City Tour Problem
- Shortest Common Substring
Ans . Travelling Salesman Problem uses Dynamic programming with a masking algorithm.
Ans.: The complexity of TSP using Greedy will be O(N^2 LogN) and using DP will be O(N^2 2^N).
Ans .: The TSP can be modelled as a graph problem by considering a complete graph G = (V, E). A tour is then a circuit in G that meets every node. In this context, tours are sometimes called Hamiltonian circuits.
Ans.: It is an NP-hard problem.
- Travelling Salesman Problem
Previous Post
Longest common subsequence, minimum spanning tree – kruskal algorithm.
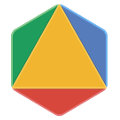
- Google OR-Tools
- Español – América Latina
- Português – Brasil
- Tiếng Việt
Traveling Salesperson Problem
This section presents an example that shows how to solve the Traveling Salesperson Problem (TSP) for the locations shown on the map below.
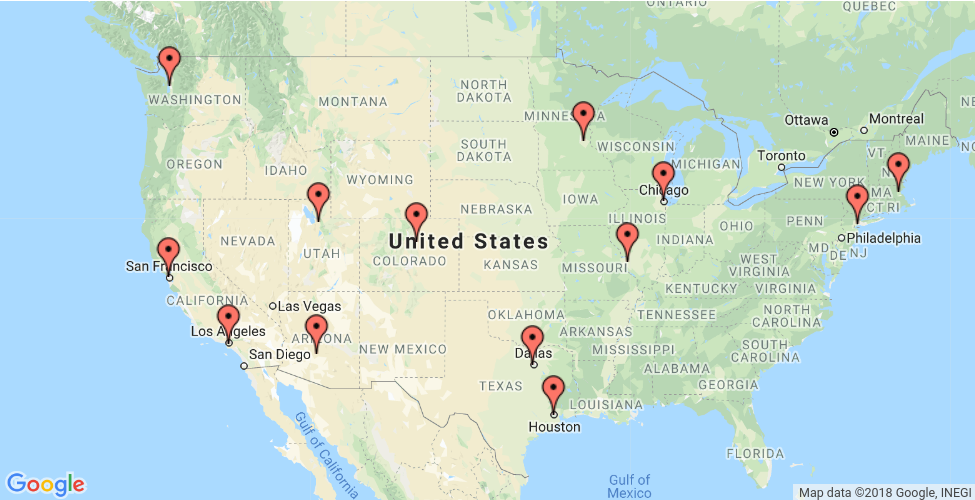
The following sections present programs in Python, C++, Java, and C# that solve the TSP using OR-Tools
Create the data
The code below creates the data for the problem.
The distance matrix is an array whose i , j entry is the distance from location i to location j in miles, where the array indices correspond to the locations in the following order:
The data also includes:
- The number of vehicles in the problem, which is 1 because this is a TSP. (For a vehicle routing problem (VRP), the number of vehicles can be greater than 1.)
- The depot : the start and end location for the route. In this case, the depot is 0, which corresponds to New York.
Other ways to create the distance matrix
In this example, the distance matrix is explicitly defined in the program. It's also possible to use a function to calculate distances between locations: for example, the Euclidean formula for the distance between points in the plane. However, it's still more efficient to pre-compute all the distances between locations and store them in a matrix, rather than compute them at run time. See Example: drilling a circuit board for an example that creates the distance matrix this way.
Another alternative is to use the Google Maps Distance Matrix API to dynamically create a distance (or travel time) matrix for a routing problem.
Create the routing model
The following code in the main section of the programs creates the index manager ( manager ) and the routing model ( routing ). The method manager.IndexToNode converts the solver's internal indices (which you can safely ignore) to the numbers for locations. Location numbers correspond to the indices for the distance matrix.
The inputs to RoutingIndexManager are:
- The number of rows of the distance matrix, which is the number of locations (including the depot).
- The number of vehicles in the problem.
- The node corresponding to the depot.
Create the distance callback
To use the routing solver, you need to create a distance (or transit) callback : a function that takes any pair of locations and returns the distance between them. The easiest way to do this is using the distance matrix.
The following function creates the callback and registers it with the solver as transit_callback_index .
The callback accepts two indices, from_index and to_index , and returns the corresponding entry of the distance matrix.
Set the cost of travel
The arc cost evaluator tells the solver how to calculate the cost of travel between any two locations — in other words, the cost of the edge (or arc) joining them in the graph for the problem. The following code sets the arc cost evaluator.
In this example, the arc cost evaluator is the transit_callback_index , which is the solver's internal reference to the distance callback. This means that the cost of travel between any two locations is just the distance between them. However, in general the costs can involve other factors as well.
You can also define multiple arc cost evaluators that depend on which vehicle is traveling between locations, using the method routing.SetArcCostEvaluatorOfVehicle() . For example, if the vehicles have different speeds, you could define the cost of travel between locations to be the distance divided by the vehicle's speed — in other words, the travel time.
Set search parameters
The following code sets the default search parameters and a heuristic method for finding the first solution:
The code sets the first solution strategy to PATH_CHEAPEST_ARC , which creates an initial route for the solver by repeatedly adding edges with the least weight that don't lead to a previously visited node (other than the depot). For other options, see First solution strategy .
Add the solution printer
The function that displays the solution returned by the solver is shown below. The function extracts the route from the solution and prints it to the console.
The function displays the optimal route and its distance, which is given by ObjectiveValue() .
Solve and print the solution
Finally, you can call the solver and print the solution:
This returns the solution and displays the optimal route.
Run the programs
When you run the programs, they display the following output.
In this example, there's only one route because it's a TSP. But in more general vehicle routing problems, the solution contains multiple routes.
Save routes to a list or array
As an alternative to printing the solution directly, you can save the route (or routes, for a VRP) to a list or array. This has the advantage of making the routes available in case you want to do something with them later. For example, you could run the program several times with different parameters and save the routes in the returned solutions to a file for comparison.
The following functions save the routes in the solution to any VRP (possibly with multiple vehicles) as a list (Python) or an array (C++).
You can use these functions to get the routes in any of the VRP examples in the Routing section.
The following code displays the routes.
For the current example, this code returns the following route:
As an exercise, modify the code above to format the output the same way as the solution printer for the program.
Complete programs
The complete TSP programs are shown below.
Example: drilling a circuit board
The next example involves drilling holes in a circuit board with an automated drill. The problem is to find the shortest route for the drill to take on the board in order to drill all of the required holes. The example is taken from TSPLIB, a library of TSP problems.
Here's scatter chart of the locations for the holes:
The following sections present programs that find a good solution to the circuit board problem, using the solver's default search parameters. After that, we'll show how to find a better solution by changing the search strategy .
The data for the problem consist of 280 points in the plane, shown in the scatter chart above. The program creates the data in an array of ordered pairs corresponding to the points in the plane, as shown below.
Compute the distance matrix
The function below computes the Euclidean distance between any two points in the data and stores it in an array. Because the routing solver works over the integers, the function rounds the computed distances to integers. Rounding doesn't affect the solution in this example, but might in other cases. See Scaling the distance matrix for a way to avoid possible rounding issues.
Add the distance callback
The code that creates the distance callback is almost the same as in the previous example. However, in this case the program calls the function that computes the distance matrix before adding the callback.
Solution printer
The following function prints the solution to the console. To keep the output more compact, the function displays just the indices of the locations in the route.
Main function
The main function is essentially the same as the one in the previous example , but also includes a call to the function that creates the distance matrix.
Running the program
The complete programs are shown in the next section . When you run the program, it displays the following route:
Here's a graph of the corresponding route:
The OR-Tools library finds the above tour very quickly: in less than a second on a typical computer. The total length of the above tour is 2790.
Here are the complete programs for the circuit board example.
Changing the search strategy
The routing solver does not always return the optimal solution to a TSP, because routing problems are computationally intractable. For instance, the solution returned in the previous example is not the optimal route.
To find a better solution, you can use a more advanced search strategy, called guided local search , which enables the solver to escape a local minimum — a solution that is shorter than all nearby routes, but which is not the global minimum. After moving away from the local minimum, the solver continues the search.
The examples below show how to set a guided local search for the circuit board example.
For other local search strategies, see Local search options .
The examples above also enable logging for the search. While logging isn't required, it can be useful for debugging.
When you run the program after making the changes shown above, you get the following solution, which is shorter than the solution shown in the previous section .
For more search options, see Routing Options .
The best algorithms can now routinely solve TSP instances with tens of thousands of nodes. (The record at the time of writing is the pla85900 instance in TSPLIB, a VLSI application with 85,900 nodes. For certain instances with millions of nodes, solutions have been found guaranteed to be within 1% of an optimal tour.)
Scaling the distance matrix
Since the routing solver works over the integers, if your distance matrix has non-integer entries, you have to round the distances to integers. If some distances are small, rounding can affect the solution.
To avoid any issue with rounding, you can scale the distance matrix: multiply all entries of the matrix by a large number — say 100. This multiplies the length of any route by a factor of 100, but it doesn't change the solution. The advantage is that now when you round the matrix entries, the rounding amount (which is at most 0.5), is very small compared to the distances, so it won't affect the solution significantly.
If you scale the distance matrix, you also need to change the solution printer to divide the scaled route lengths by the scaling factor, so that it displays the unscaled distances of the routes.
Except as otherwise noted, the content of this page is licensed under the Creative Commons Attribution 4.0 License , and code samples are licensed under the Apache 2.0 License . For details, see the Google Developers Site Policies . Java is a registered trademark of Oracle and/or its affiliates.
Last updated 2023-01-16 UTC.

- school Campus Bookshelves
- menu_book Bookshelves
- perm_media Learning Objects
- login Login
- how_to_reg Request Instructor Account
- hub Instructor Commons
- Download Page (PDF)
- Download Full Book (PDF)
- Periodic Table
- Physics Constants
- Scientific Calculator
- Reference & Cite
- Tools expand_more
- Readability
selected template will load here
This action is not available.
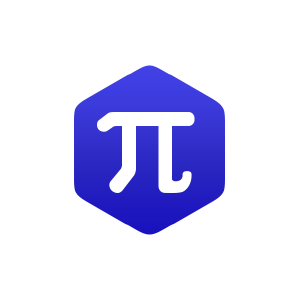
12.10: Traveling Salesperson Problem
- Last updated
- Save as PDF
- Page ID 129677
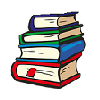
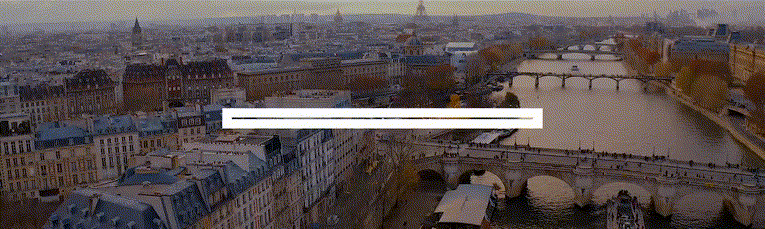
Learning Objectives
After completing this section, you should be able to:
- Distinguish between brute force algorithms and greedy algorithms.
- List all distinct Hamilton cycles of a complete graph.
- Apply brute force method to solve traveling salesperson applications.
- Apply nearest neighbor method to solve traveling salesperson applications.
We looked at Hamilton cycles and paths in the previous sections Hamilton Cycles and Hamilton Paths. In this section, we will analyze Hamilton cycles in complete weighted graphs to find the shortest route to visit a number of locations and return to the starting point. Besides the many routing applications in which we need the shortest distance, there are also applications in which we search for the route that is least expensive or takes the least time. Here are a few less common applications that you can read about on a website set up by the mathematics department at the University of Waterloo in Ontario, Canada:
- Design of fiber optic networks
- Minimizing fuel expenses for repositioning satellites
- Development of semi-conductors for microchips
- A technique for mapping mammalian chromosomes in genome sequencing
Before we look at approaches to solving applications like these, let's discuss the two types of algorithms we will use.
Brute Force and Greedy Algorithms
An algorithm is a sequence of steps that can be used to solve a particular problem. We have solved many problems in this chapter, and the procedures that we used were different types of algorithms. In this section, we will use two common types of algorithms, a brute force algorithm and a greedy algorithm . A brute force algorithm begins by listing every possible solution and applying each one until the best solution is found. A greedy algorithm approaches a problem in stages, making the apparent best choice at each stage, then linking the choices together into an overall solution which may or may not be the best solution.
To understand the difference between these two algorithms, consider the tree diagram in Figure 12.214. Suppose we want to find the path from left to right with the largest total sum. For example, branch A in the tree diagram has a sum of 10 + 2 + 11 + 13 = 36 10 + 2 + 11 + 13 = 36 .
To be certain that you pick the branch with greatest sum, you could list each sum from each of the different branches:
A : 10 + 2 + 11 + 13 = 36 10 + 2 + 11 + 13 = 36
B : 10 + 2 + 11 + 8 = 31 10 + 2 + 11 + 8 = 31
C : 10 + 2 + 15 + 1 = 28 10 + 2 + 15 + 1 = 28
D : 10 + 2 + 15 + 6 = 33 10 + 2 + 15 + 6 = 33
E : 10 + 7 + 3 + 20 = 40 10 + 7 + 3 + 20 = 40
F : 10 + 7 + 3 + 14 = 34 10 + 7 + 3 + 14 = 34
G : 10 + 7 + 4 + 11 = 32 10 + 7 + 4 + 11 = 32
H : 10 + 7 + 4 + 5 = 26 10 + 7 + 4 + 5 = 26
Then we know with certainty that branch E has the greatest sum.
Now suppose that you wanted to find the branch with the highest value, but you only were shown the tree diagram in phases, one step at a time.
After phase 1, you would have chosen the branch with 10 and 7. So far, you are following the same branch. Let’s look at the next phase.
After phase 2, based on the information you have, you will choose the branch with 10, 7 and 4. Now, you are following a different branch than before, but it is the best choice based on the information you have. Let’s look at the last phase.
After phase 3, you will choose branch G which has a sum of 32.
The process of adding the values on each branch and selecting the highest sum is an example of a brute force algorithm because all options were explored in detail. The process of choosing the branch in phases, based on the best choice at each phase is a greedy algorithm. Although a brute force algorithm gives us the ideal solution, it can take a very long time to implement. Imagine a tree diagram with thousands or even millions of branches. It might not be possible to check all the sums. A greedy algorithm, on the other hand, can be completed in a relatively short time, and generally leads to good solutions, but not necessarily the ideal solution.
Example 12.42
Distinguishing between brute force and greedy algorithms.
A cashier rings up a sale for $4.63 cents in U.S. currency. The customer pays with a $5 bill. The cashier would like to give the customer $0.37 in change using the fewest coins possible. The coins that can be used are quarters ($0.25), dimes ($0.10), nickels ($0.05), and pennies ($0.01). The cashier starts by selecting the coin of highest value less than or equal to $0.37, which is a quarter. This leaves $ 0.37 − $ 0.25 = $ 0.12 $ 0.37 − $ 0.25 = $ 0.12 . The cashier selects the coin of highest value less than or equal to $0.12, which is a dime. This leaves $ 0.12 − $ 0.10 = $ 0.02 $ 0.12 − $ 0.10 = $ 0.02 . The cashier selects the coin of highest value less than or equal to $0.02, which is a penny. This leaves $ 0.02 − $ 0.01 = $ 0.01 $ 0.02 − $ 0.01 = $ 0.01 . The cashier selects the coin of highest value less than or equal to $0.01, which is a penny. This leaves no remainder. The cashier used one quarter, one dime, and two pennies, which is four coins. Use this information to answer the following questions.
- Is the cashier’s approach an example of a greedy algorithm or a brute force algorithm? Explain how you know.
- The cashier’s solution is the best solution. In other words, four is the fewest number of coins possible. Is this consistent with the results of an algorithm of this kind? Explain your reasoning.
- The approach the cashier used is an example of a greedy algorithm, because the problem was approached in phases and the best choice was made at each phase. Also, it is not a brute force algorithm, because the cashier did not attempt to list out all possible combinations of coins to reach this conclusion.
- Yes, it is consistent. A greedy algorithm does not always yield the best result, but sometimes it does.
Your Turn 12.42
The traveling salesperson problem.
Now let’s focus our attention on the graph theory application known as the traveling salesperson problem (TSP) in which we must find the shortest route to visit a number of locations and return to the starting point.
Recall from Hamilton Cycles, the officer in the U.S. Air Force who is stationed at Vandenberg Air Force base and must drive to visit three other California Air Force bases before returning to Vandenberg. The officer needed to visit each base once. We looked at the weighted graph in Figure 12.219 representing the four U.S. Air Force bases: Vandenberg, Edwards, Los Angeles, and Beal and the distances between them.
Any route that visits each base and returns to the start would be a Hamilton cycle on the graph. If the officer wants to travel the shortest distance, this will correspond to a Hamilton cycle of lowest weight. We saw in Table 12.11 that there are six distinct Hamilton cycles (directed cycles) in a complete graph with four vertices, but some lie on the same cycle (undirected cycle) in the graph.
a → b → c → d → a
a → b → d → c → a
a → c → b → d → a
a → d → c → b → a
a → c → d → b → a
a → d → b → c → a
Since the distance between bases is the same in either direction, it does not matter if the officer travels clockwise or counterclockwise. So, there are really only three possible distances as shown in Figure 12.220.
The possible distances are:
396 + 410 + 106 + 159 = 1071 207 + 410 + 439 + 159 = 1215 396 + 439 + 106 + 207 = 1148 396 + 410 + 106 + 159 = 1071 207 + 410 + 439 + 159 = 1215 396 + 439 + 106 + 207 = 1148
So, a Hamilton cycle of least weight is V → B → E → L → V (or the reverse direction). The officer should travel from Vandenberg to Beal to Edwards, to Los Angeles, and back to Vandenberg.
Finding Weights of All Hamilton Cycles in Complete Graphs
Notice that we listed all of the Hamilton cycles and found their weights when we solved the TSP about the officer from Vandenberg. This is a skill you will need to practice. To make sure you don't miss any, you can calculate the number of possible Hamilton cycles in a complete graph. It is also helpful to know that half of the directed cycles in a complete graph are the same cycle in reverse direction, so, you only have to calculate half the number of possible weights, and the rest are duplicates.
In a complete graph with n n vertices,
- The number of distinct Hamilton cycles is ( n − 1 ) ! ( n − 1 ) ! .
- There are at most ( n − 1 ) ! 2 ( n − 1 ) ! 2 different weights of Hamilton cycles.
TIP! When listing all the distinct Hamilton cycles in a complete graph, you can start them all at any vertex you choose. Remember, the cycle a → b → c → a is the same cycle as b → c → a → b so there is no need to list both.
Example 12.43
Calculating possible weights of hamilton cycles.
Suppose you have a complete weighted graph with vertices N, M, O , and P .
- Use the formula ( n − 1 ) ! ( n − 1 ) ! to calculate the number of distinct Hamilton cycles in the graph.
- Use the formula ( n − 1 ) ! 2 ( n − 1 ) ! 2 to calculate the greatest number of different weights possible for the Hamilton cycles.
- Are all of the distinct Hamilton cycles listed here? How do you know? Cycle 1: N → M → O → P → N Cycle 2: N → M → P → O → N Cycle 3: N → O → M → P → N Cycle 4: N → O → P → M → N Cycle 5: N → P → M → O → N Cycle 6: N → P → O → M → N
- Which pairs of cycles must have the same weights? How do you know?
- There are 4 vertices; so, n = 4 n = 4 . This means there are ( n − 1 ) ! = ( 4 − 1 ) ! = 3 ⋅ 2 ⋅ 1 = 6 ( n − 1 ) ! = ( 4 − 1 ) ! = 3 ⋅ 2 ⋅ 1 = 6 distinct Hamilton cycles beginning at any given vertex.
- Since n = 4 n = 4 , there are ( n − 1 ) ! 2 = ( 4 − 1 ) ! 2 = 6 2 = 3 ( n − 1 ) ! 2 = ( 4 − 1 ) ! 2 = 6 2 = 3 possible weights.
- Yes, they are all distinct cycles and there are 6 of them.
- Cycles 1 and 6 have the same weight, Cycles 2 and 4 have the same weight, and Cycles 3 and 5 have the same weight, because these pairs follow the same route through the graph but in reverse.
TIP! When listing the possible cycles, ignore the vertex where the cycle begins and ends and focus on the ways to arrange the letters that represent the vertices in the middle. Using a systematic approach is best; for example, if you must arrange the letters M, O, and P, first list all those arrangements beginning with M, then beginning with O, and then beginning with P, as we did in Example 12.42.
Your Turn 12.43
The brute force method.
The method we have been using to find a Hamilton cycle of least weight in a complete graph is a brute force algorithm, so it is called the brute force method . The steps in the brute force method are:
Step 1: Calculate the number of distinct Hamilton cycles and the number of possible weights.
Step 2: List all possible Hamilton cycles.
Step 3: Find the weight of each cycle.
Step 4: Identify the Hamilton cycle of lowest weight.
Example 12.44
Applying the brute force method.
On the next assignment, the air force officer must leave from Travis Air Force base, visit Beal, Edwards, and Vandenberg Air Force bases each exactly once and return to Travis Air Force base. There is no need to visit Los Angeles Air Force base. Use Figure 12.221 to find the shortest route.
Step 1: Since there are 4 vertices, there will be ( 4 − 1 ) ! = 3 ! = 6 ( 4 − 1 ) ! = 3 ! = 6 cycles, but half of them will be the reverse of the others; so, there will be ( 4 − 1 ) ! 2 = 6 2 = 3 ( 4 − 1 ) ! 2 = 6 2 = 3 possible distances.
Step 2: List all the Hamilton cycles in the subgraph of the graph in Figure 12.222.
To find the 6 cycles, focus on the three vertices in the middle, B, E, and V . The arrangements of these vertices are BEV, BVE, EBV, EVB, VBE , and VEB . These would correspond to the 6 cycles:
1: T → B → E → V → T
2: T → B → V → E → T
3: T → E → B → V → T
4: T → E → V → B → T
5: T → V → B → E → T
6: T → V → E → B → T
Step 3: Find the weight of each path. You can reduce your work by observing the cycles that are reverses of each other.
1: 84 + 410 + 207 + 396 = 1097 84 + 410 + 207 + 396 = 1097
2: 84 + 396 + 207 + 370 = 1071 84 + 396 + 207 + 370 = 1071
3: 370 + 410 + 396 + 396 = 1572 370 + 410 + 396 + 396 = 1572
4: Reverse of cycle 2, 1071
5: Reverse of cycle 3, 1572
6: Reverse of cycle 1, 1097
Step 4: Identify a Hamilton cycle of least weight.
The second path, T → B → V → E → T , and its reverse, T → E → V → B → T , have the least weight. The solution is that the officer should travel from Travis Air Force base to Beal Air Force Base, to Vandenberg Air Force base, to Edwards Air Force base, and return to Travis Air Force base, or the same route in reverse.
Your Turn 12.44
Now suppose that the officer needed a cycle that visited all 5 of the Air Force bases in Figure 12.221. There would be ( 5 − 1 ) ! = 4 ! = 4 × 3 × 2 × 1 = 24 ( 5 − 1 ) ! = 4 ! = 4 × 3 × 2 × 1 = 24 different arrangements of vertices and ( 5 − 1 ) ! 2 = 4 ! 2 = 24 2 = 12 ( 5 − 1 ) ! 2 = 4 ! 2 = 24 2 = 12 distances to compare using the brute force method. If you consider 10 Air Force bases, there would be ( 10 − 1 ) ! = 9 ! = 9 ⋅ 8 ⋅ 7 ⋅ 6 ⋅ 5 ⋅ 4 ⋅ 3 ⋅ 2 ⋅ 1 = 362 , 880 ( 10 − 1 ) ! = 9 ! = 9 ⋅ 8 ⋅ 7 ⋅ 6 ⋅ 5 ⋅ 4 ⋅ 3 ⋅ 2 ⋅ 1 = 362 , 880 different arrangements and ( 10 − 1 ) ! 2 = 9 ! 2 = 9 ⋅ 8 ⋅ 7 ⋅ 6 ⋅ 5 ⋅ 4 ⋅ 3 ⋅ 2 ⋅ 1 2 = 181 , 440 ( 10 − 1 ) ! 2 = 9 ! 2 = 9 ⋅ 8 ⋅ 7 ⋅ 6 ⋅ 5 ⋅ 4 ⋅ 3 ⋅ 2 ⋅ 1 2 = 181 , 440 distances to consider. There must be another way!
The Nearest Neighbor Method
When the brute force method is impractical for solving a traveling salesperson problem, an alternative is a greedy algorithm known as the nearest neighbor method , which always visit the closest or least costly place first. This method finds a Hamilton cycle of relatively low weight in a complete graph in which, at each phase, the next vertex is chosen by comparing the edges between the current vertex and the remaining vertices to find the lowest weight. Since the nearest neighbor method is a greedy algorithm, it usually doesn’t give the best solution, but it usually gives a solution that is "good enough." Most importantly, the number of steps will be the number of vertices. That’s right! A problem with 10 vertices requires 10 steps, not 362,880. Let’s look at an example to see how it works.
Suppose that a candidate for governor wants to hold rallies around the state. They plan to leave their home in city A , visit cities B, C, D, E , and F each once, and return home. The airfare between cities is indicated in the graph in Figure 12.223.
Let’s help the candidate keep costs of travel down by applying the nearest neighbor method to find a Hamilton cycle that has a reasonably low weight. Begin by marking starting vertex as V 1 Figure 12.224. The lowest of these is $100, which is the edge between A and F .
Mark F as V 2 Figure 12.225. The lowest of these is $150, which is the edge between F and D .
Mark D as V 3 Figure 12.226. The lowest of these is $120, which is the edge between D and B .
So, mark B as V 4 Figure 12.227. The lower amount is $160, which is the edge between B and E .
Now you can mark E as V 5 Figure 12.228. Make a note of the weight of the edge from E to C , which is $180, and from C back to A , which is $210.
The Hamilton cycle we found is A → F → D → B → E → C → A . The weight of the circuit is $ 100 + $ 150 + $ 120 + $ 160 + $ 180 + $ 210 = $ 920 $ 100 + $ 150 + $ 120 + $ 160 + $ 180 + $ 210 = $ 920 . This may or may not be the route with the lowest cost, but there is a good chance it is very close since the weights are most of the lowest weights on the graph and we found it in six steps instead of finding 120 different Hamilton cycles and calculating 60 weights. Let’s summarize the procedure that we used.
Step 1: Select the starting vertex and label V 1 V 1 for "visited 1st." Identify the edge of lowest weight between V 1 V 1 and the remaining vertices.
Step 2: Label the vertex at the end of the edge of lowest weight that you found in previous step as V n V n where the subscript n indicates the order the vertex is visited. Identify the edge of lowest weight between V n V n and the vertices that remain to be visited.
Step 3: If vertices remain that have not been visited, repeat Step 2. Otherwise, a Hamilton cycle of low weight is V 1 → V 2 → ⋯ → V n → V 1 V 1 → V 2 → ⋯ → V n → V 1 .
Example 12.45
Using the nearest neighbor method.
Suppose that the candidate for governor wants to hold rallies around the state but time before the election is very limited. They would like to leave their home in city A , visit cities B , C , D , E , and F each once, and return home. The airfare between cities is not as important as the time of travel, which is indicated in Figure 12.229. Use the nearest neighbor method to find a route with relatively low travel time. What is the total travel time of the route that you found?
Step 1: Label vertex A as V 1 V 1 . The edge of lowest weight between A and the remaining vertices is 85 min between A and D .
Step 2: Label vertex D as V 2 V 2 . The edge of lowest weight between D and the vertices that remain to be visited, B, C, E , and F , is 70 min between D and F .
Repeat Step 2: Label vertex F as V 3 V 3 . The edge of lowest weight between F and the vertices that remain to be visited, B, C, and E , is 75 min between F and C .
Repeat Step 2: Label vertex C as V 4 V 4 . The edge of lowest weight between C and the vertices that remain to be visited, B and E , is 100 min between C and B .
Repeat Step 2: Label vertex B as V 5 V 5 . The only vertex that remains to be visited is E . The weight of the edge between B and E is 95 min.
Step 3: A Hamilton cycle of low weight is A → D → F → C → B → E → A . So, a route of relatively low travel time is A to D to F to C to B to E and back to A . The total travel time of this route is: 85 min + 70 min + 75 min + 100 min + 95 min + 90 min = 515 min or 8 hrs 35 min 85 min + 70 min + 75 min + 100 min + 95 min + 90 min = 515 min or 8 hrs 35 min
Your Turn 12.45
Check your understanding, section 12.9 exercises.
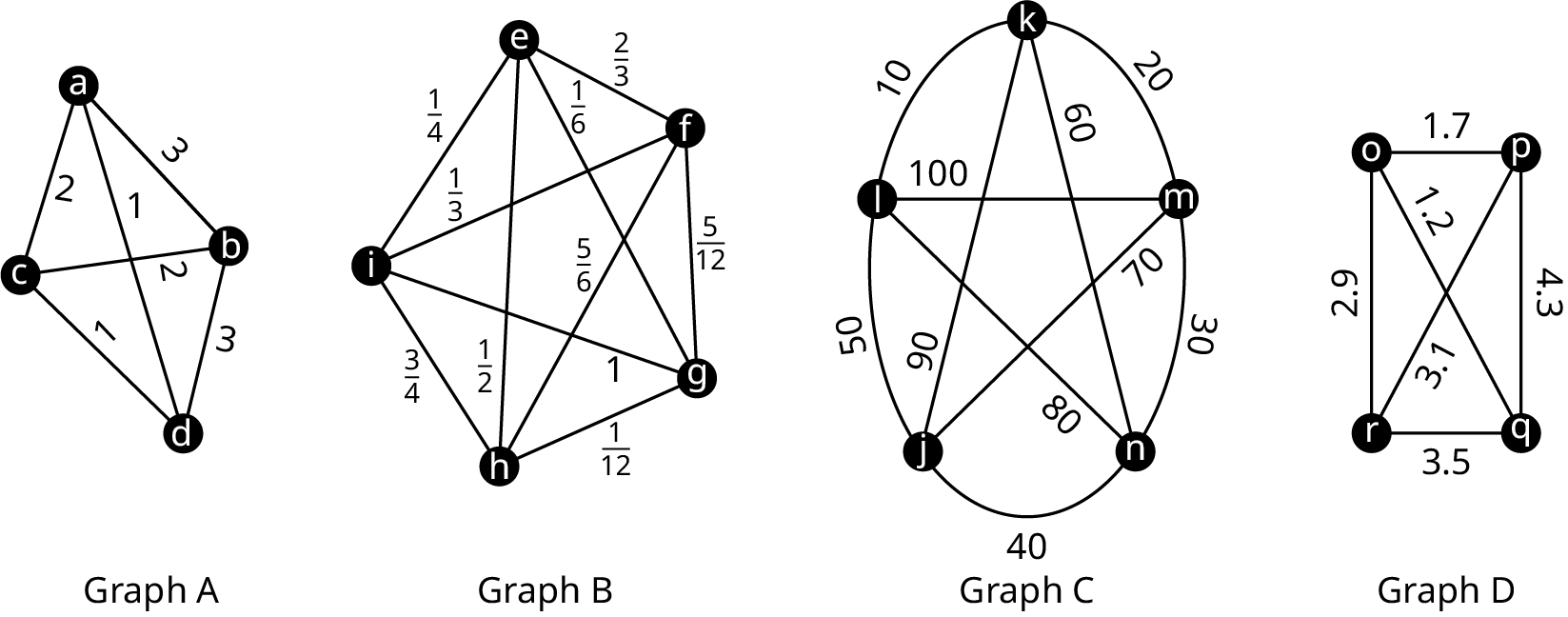

Travelling Salesman Problem: Python, C++ Algorithm
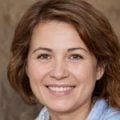
What is the Travelling Salesman Problem (TSP)?
Travelling Salesman Problem (TSP) is a classic combinatorics problem of theoretical computer science. The problem asks to find the shortest path in a graph with the condition of visiting all the nodes only one time and returning to the origin city.
The problem statement gives a list of cities along with the distances between each city.
Objective: To start from the origin city, visit other cities only once, and return to the original city again. Our target is to find the shortest possible path to complete the round-trip route.
Example of TSP
Here a graph is given where 1, 2, 3, and 4 represent the cities, and the weight associated with every edge represents the distance between those cities.
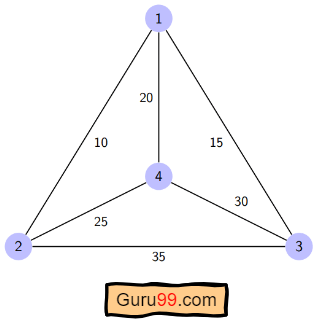
The goal is to find the shortest possible path for the tour that starts from the origin city, traverses the graph while only visiting the other cities or nodes once, and returns to the origin city.
For the above graph, the optimal route is to follow the minimum cost path: 1-2-4-3-1. And this shortest route would cost 10+25+30+15 =80
Different Solutions to Travelling Salesman Problem

Travelling Salesman Problem (TSP) is classified as a NP-hard problem due to having no polynomial time algorithm. The complexity increases exponentially by increasing the number of cities.
There are multiple ways to solve the traveling salesman problem (tsp). Some popular solutions are:
The brute force approach is the naive method for solving traveling salesman problems. In this approach, we first calculate all possible paths and then compare them. The number of paths in a graph consisting of n cities is n! It is computationally very expensive to solve the traveling salesman problem in this brute force approach.
The branch-and-bound method: The problem is broken down into sub-problems in this approach. The solution of those individual sub-problems would provide an optimal solution.
This tutorial will demonstrate a dynamic programming approach, the recursive version of this branch-and-bound method, to solve the traveling salesman problem.
Dynamic programming is such a method for seeking optimal solutions by analyzing all possible routes. It is one of the exact solution methods that solve traveling salesman problems through relatively higher cost than the greedy methods that provide a near-optimal solution.
The computational complexity of this approach is O(N^2 * 2^N) which is discussed later in this article.
The nearest neighbor method is a heuristic-based greedy approach where we choose the nearest neighbor node. This approach is computationally less expensive than the dynamic approach. But it does not provide the guarantee of an optimal solution. This method is used for near-optimal solutions.
Algorithm for Traveling Salesman Problem
We will use the dynamic programming approach to solve the Travelling Salesman Problem (TSP).
Before starting the algorithm, let’s get acquainted with some terminologies:
- A graph G=(V, E), which is a set of vertices and edges.
- V is the set of vertices.
- E is the set of edges.
- Vertices are connected through edges.
- Dist(i,j) denotes the non-negative distance between two vertices, i and j.
Let’s assume S is the subset of cities and belongs to {1, 2, 3, …, n} where 1, 2, 3…n are the cities and i, j are two cities in that subset. Now cost(i, S, j) is defined in such a way as the length of the shortest path visiting node in S, which is exactly once having the starting and ending point as i and j respectively.
For example, cost (1, {2, 3, 4}, 1) denotes the length of the shortest path where:
- Starting city is 1
- Cities 2, 3, and 4 are visited only once
- The ending point is 1
The dynamic programming algorithm would be:
- Set cost(i, , i) = 0, which means we start and end at i, and the cost is 0.
- When |S| > 1, we define cost(i, S, 1) = ∝ where i !=1 . Because initially, we do not know the exact cost to reach city i to city 1 through other cities.
- Now, we need to start at 1 and complete the tour. We need to select the next city in such a way-
cost(i, S, j)=min cost (i, S−{i}, j)+dist(i,j) where i∈S and i≠j
For the given figure, the adjacency matrix would be the following:

Let’s see how our algorithm works:
Step 1) We are considering our journey starting at city 1, visit other cities once and return to city 1.
Step 2) S is the subset of cities. According to our algorithm, for all |S| > 1, we will set the distance cost(i, S, 1) = ∝. Here cost(i, S, j) means we are starting at city i, visiting the cities of S once, and now we are at city j. We set this path cost as infinity because we do not know the distance yet. So the values will be the following:
Cost (2, {3, 4}, 1) = ∝ ; the notation denotes we are starting at city 2, going through cities 3, 4, and reaching 1. And the path cost is infinity. Similarly-
cost(3, {2, 4}, 1) = ∝
cost(4, {2, 3}, 1) = ∝
Step 3) Now, for all subsets of S, we need to find the following:
cost(i, S, j)=min cost (i, S−{i}, j)+dist(i,j), where j∈S and i≠j
That means the minimum cost path for starting at i, going through the subset of cities once, and returning to city j. Considering that the journey starts at city 1, the optimal path cost would be= cost(1, {other cities}, 1).
Let’s find out how we could achieve that:
Now S = {1, 2, 3, 4}. There are four elements. Hence the number of subsets will be 2^4 or 16. Those subsets are-
1) |S| = Null:
2) |S| = 1:
{{1}, {2}, {3}, {4}}
3) |S| = 2:
{{1, 2}, {1, 3}, {1, 4}, {2, 3}, {2, 4}, {3, 4}}
4) |S| = 3:
{{1, 2, 3}, {1, 2, 4}, {2, 3, 4}, {1, 3, 4}}
5) |S| = 4:
{{1, 2, 3, 4}}
As we are starting at 1, we could discard the subsets containing city 1.
The algorithm calculation:
1) |S| = Φ:
cost (2, Φ, 1) = dist(2, 1) = 10
cost (3, Φ, 1) = dist(3, 1) = 15
cost (4, Φ, 1) = dist(4, 1) = 20
cost (2, {3}, 1) = dist(2, 3) + cost (3, Φ, 1) = 35+15 = 50
cost (2, {4}, 1) = dist(2, 4) + cost (4, Φ, 1) = 25+20 = 45
cost (3, {2}, 1) = dist(3, 2) + cost (2, Φ, 1) = 35+10 = 45
cost (3, {4}, 1) = dist(3, 4) + cost (4, Φ, 1) = 30+20 = 50
cost (4, {2}, 1) = dist(4, 2) + cost (2, Φ, 1) = 25+10 = 35
cost (4, {3}, 1) = dist(4, 3) + cost (3, Φ, 1) = 30+15 = 45
cost (2, {3, 4}, 1) = min [ dist[2,3]+Cost(3,{4},1) = 35+50 = 85,
dist[2,4]+Cost(4,{3},1) = 25+45 = 70 ] = 70
cost (3, {2, 4}, 1) = min [ dist[3,2]+Cost(2,{4},1) = 35+45 = 80,
dist[3,4]+Cost(4,{2},1) = 30+35 = 65 ] = 65
cost (4, {2, 3}, 1) = min [ dist[4,2]+Cost(2,{3},1) = 25+50 = 75
dist[4,3]+Cost(3,{2},1) = 30+45 = 75 ] = 75
cost (1, {2, 3, 4}, 1) = min [ dist[1,2]+Cost(2,{3,4},1) = 10+70 = 80
dist[1,3]+Cost(3,{2,4},1) = 15+65 = 80
dist[1,4]+Cost(4,{2,3},1) = 20+75 = 95 ] = 80
So the optimal solution would be 1-2-4-3-1

Pseudo-code
Implementation in c/c++.
Here’s the implementation in C++ :
Implementation in Python
Academic solutions to tsp.
Computer scientists have spent years searching for an improved polynomial time algorithm for the Travelling Salesman Problem. Until now, the problem is still NP-hard.
Though some of the following solutions were published in recent years that have reduced the complexity to a certain degree:
- The classical symmetric TSP is solved by the Zero Suffix Method.
- The Biogeography‐based Optimization Algorithm is based on the migration strategy to solve the optimization problems that can be planned as TSP.
- Multi-Objective Evolutionary Algorithm is designed for solving multiple TSP based on NSGA-II.
- The Multi-Agent System solves the TSP of N cities with fixed resources.
Application of Traveling Salesman Problem
Travelling Salesman Problem (TSP) is applied in the real world in both its purest and modified forms. Some of those are:
- Planning, logistics, and manufacturing microchips : Chip insertion problems naturally arise in the microchip industry. Those problems can be planned as traveling salesman problems.
- DNA sequencing : Slight modification of the traveling salesman problem can be used in DNA sequencing. Here, the cities represent the DNA fragments, and the distance represents the similarity measure between two DNA fragments.
- Astronomy : The Travelling Salesman Problem is applied by astronomers to minimize the time spent observing various sources.
- Optimal control problem : Travelling Salesman Problem formulation can be applied in optimal control problems. There might be several other constraints added.
Complexity Analysis of TSP
So the total time complexity for an optimal solution would be the Number of nodes * Number of subproblems * time to solve each sub-problem. The time complexity can be defined as O(N 2 * 2^N).
- Space Complexity: The dynamic programming approach uses memory to store C(S, i), where S is a subset of the vertices set. There is a total of 2 N subsets for each node. So, the space complexity is O(2^N).
Next, you’ll learn about Sieve of Eratosthenes Algorithm
- Linear Search: Python, C++ Example
- DAA Tutorial PDF: Design and Analysis of Algorithms
- Heap Sort Algorithm (With Code in Python and C++)
- Kadence’s Algorithm: Largest Sum Contiguous Subarray
- Radix Sort Algorithm in Data Structure
- Doubly Linked List: C++, Python (Code Example)
- Singly Linked List in Data Structures
- Adjacency List and Matrix Representation of Graph
DSA Tutorial
Linked lists, stacks & queues, hash tables, shortest path, minimum spanning tree, maximum flow, time complexity, dsa reference, dsa examples, dsa the traveling salesman problem.
The Traveling Salesman Problem
The Traveling Salesman Problem states that you are a salesperson and you must visit a number of cities or towns.
Rules : Visit every city only once, then return back to the city you started in.
Goal : Find the shortest possible route.
Except for the Held-Karp algorithm (which is quite advanced and time consuming, (\(O(2^n n^2)\)), and will not be described here), there is no other way to find the shortest route than to check all possible routes.
This means that the time complexity for solving this problem is \(O(n!)\), which means 720 routes needs to be checked for 6 cities, 40,320 routes must be checked for 8 cities, and if you have 10 cities to visit, more than 3.6 million routes must be checked!
Note: "!", or "factorial", is a mathematical operation used in combinatorics to find out how many possible ways something can be done. If there are 4 cities, each city is connected to every other city, and we must visit every city exactly once, there are \(4!= 4 \cdot 3 \cdot 2 \cdot 1 = 24\) different routes we can take to visit those cities.
The Traveling Salesman Problem (TSP) is a problem that is interesting to study because it is very practical, but so time consuming to solve, that it becomes nearly impossible to find the shortest route, even in a graph with just 20-30 vertices.
If we had an effective algorithm for solving The Traveling Salesman Problem, the consequences would be very big in many sectors, like for example chip design, vehicle routing, telecommunications, and urban planning.
Checking All Routes to Solve The Traveling Salesman Problem
To find the optimal solution to The Traveling Salesman Problem, we will check all possible routes, and every time we find a shorter route, we will store it, so that in the end we will have the shortest route.
Good: Finds the overall shortest route.
Bad: Requires an awful lot of calculation, especially for a large amount of cities, which means it is very time consuming.
How it works:
- Check the length of every possible route, one route at a time.
- Is the current route shorter than the shortest route found so far? If so, store the new shortest route.
- After checking all routes, the stored route is the shortest one.
Such a way of finding the solution to a problem is called brute force .
Brute force is not really an algorithm, it just means finding the solution by checking all possibilities, usually because of a lack of a better way to do it.
Speed: {{ inpVal }}
Finding the shortest route in The Traveling Salesman Problem by checking all routes (brute force).
Progress: {{progress}}%
n = {{vertices}} cities
{{vertices}}!={{posRoutes}} possible routes
Show every route: {{showCompares}}
The reason why the brute force approach of finding the shortest route (as shown above) is so time consuming is that we are checking all routes, and the number of possible routes increases really fast when the number of cities increases.
Finding the optimal solution to the Traveling Salesman Problem by checking all possible routes (brute force):
Using A Greedy Algorithm to Solve The Traveling Salesman Problem
Since checking every possible route to solve the Traveling Salesman Problem (like we did above) is so incredibly time consuming, we can instead find a short route by just going to the nearest unvisited city in each step, which is much faster.
Good: Finds a solution to the Traveling Salesman Problem much faster than by checking all routes.
Bad: Does not find the overall shortest route, it just finds a route that is much shorter than an average random route.
- Visit every city.
- The next city to visit is always the nearest of the unvisited cities from the city you are currently in.
- After visiting all cities, go back to the city you started in.
This way of finding an approximation to the shortest route in the Traveling Salesman Problem, by just going to the nearest unvisited city in each step, is called a greedy algorithm .
Finding an approximation to the shortest route in The Traveling Salesman Problem by always going to the nearest unvisited neighbor (greedy algorithm).
As you can see by running this simulation a few times, the routes that are found are not completely unreasonable. Except for a few times when the lines cross perhaps, especially towards the end of the algorithm, the resulting route is a lot shorter than we would get by choosing the next city at random.
Finding a near-optimal solution to the Traveling Salesman Problem using the nearest-neighbor algorithm (greedy):
Other Algorithms That Find Near-Optimal Solutions to The Traveling Salesman Problem
In addition to using a greedy algorithm to solve the Traveling Salesman Problem, there are also other algorithms that can find approximations to the shortest route.
These algorithms are popular because they are much more effective than to actually check all possible solutions, but as with the greedy algorithm above, they do not find the overall shortest route.
Algorithms used to find a near-optimal solution to the Traveling Salesman Problem include:
- 2-opt Heuristic: An algorithm that improves the solution step-by-step, in each step removing two edges and reconnecting the two paths in a different way to reduce the total path length.
- Genetic Algorithm: This is a type of algorithm inspired by the process of natural selection and use techniques such as selection, mutation, and crossover to evolve solutions to problems, including the TSP.
- Simulated Annealing: This method is inspired by the process of annealing in metallurgy. It involves heating and then slowly cooling a material to decrease defects. In the context of TSP, it's used to find a near-optimal solution by exploring the solution space in a way that allows for occasional moves to worse solutions, which helps to avoid getting stuck in local minima.
- Ant Colony Optimization: This algorithm is inspired by the behavior of ants in finding paths from the colony to food sources. It's a more complex probabilistic technique for solving computational problems which can be mapped to finding good paths through graphs.
Time Complexity for Solving The Traveling Salesman Problem
To get a near-optimal solution fast, we can use a greedy algorithm that just goes to the nearest unvisited city in each step, like in the second simulation on this page.
Solving The Traveling Salesman Problem in a greedy way like that, means that at each step, the distances from the current city to all other unvisited cities are compared, and that gives us a time complexity of \(O(n^2) \).
But finding the shortest route of them all requires a lot more operations, and the time complexity for that is \(O(n!)\), like mentioned earlier, which means that for 4 cities, there are 4! possible routes, which is the same as \(4 \cdot 3 \cdot 2 \cdot 1 = 24\). And for just 12 cities for example, there are \(12! = 12 \cdot 11 \cdot 10 \cdot \; ... \; \cdot 2 \cdot 1 = 479,001,600\) possible routes!
See the time complexity for the greedy algorithm \(O(n^2)\), versus the time complexity for finding the shortest route by comparing all routes \(O(n!)\), in the image below.

But there are two things we can do to reduce the number of routes we need to check.
In the Traveling Salesman Problem, the route starts and ends in the same place, which makes a cycle. This means that the length of the shortest route will be the same no matter which city we start in. That is why we have chosen a fixed city to start in for the simulation above, and that reduces the number of possible routes from \(n!\) to \((n-1)!\).
Also, because these routes go in cycles, a route has the same distance if we go in one direction or the other, so we actually just need to check the distance of half of the routes, because the other half will just be the same routes in the opposite direction, so the number of routes we need to check is actually \( \frac{(n-1)!}{2}\).
But even if we can reduce the number of routes we need to check to \( \frac{(n-1)!}{2}\), the time complexity is still \( O(n!)\), because for very big \(n\), reducing \(n\) by one and dividing by 2 does not make a significant change in how the time complexity grows when \(n\) is increased.
To better understand how time complexity works, go to this page .
Actual Traveling Salesman Problems Are More Complex
The edge weight in a graph in this context of The Traveling Salesman Problem tells us how hard it is to go from one point to another, and it is the total edge weight of a route we want to minimize.
So far on this page, the edge weight has been the distance in a straight line between two points. And that makes it much easier to explain the Traveling Salesman Problem, and to display it.
But in the real world there are many other things that affects the edge weight:
- Obstacles: When moving from one place to another, we normally try to avoid obstacles like trees, rivers, houses for example. This means it is longer and takes more time to go from A to B, and the edge weight value needs to be increased to factor that in, because it is not a straight line anymore.
- Transportation Networks: We usually follow a road or use public transport systems when traveling, and that also affects how hard it is to go (or send a package) from one place to another.
- Traffic Conditions: Travel congestion also affects the travel time, so that should also be reflected in the edge weight value.
- Legal and Political Boundaries: Crossing border for example, might make one route harder to choose than another, which means the shortest straight line route might be slower, or more costly.
- Economic Factors: Using fuel, using the time of employees, maintaining vehicles, all these things cost money and should also be factored into the edge weights.
As you can see, just using the straight line distances as the edge weights, might be too simple compared to the real problem. And solving the Traveling Salesman Problem for such a simplified problem model would probably give us a solution that is not optimal in a practical sense.
It is not easy to visualize a Traveling Salesman Problem when the edge length is not just the straight line distance between two points anymore, but the computer handles that very well.

COLOR PICKER

Contact Sales
If you want to use W3Schools services as an educational institution, team or enterprise, send us an e-mail: [email protected]
Report Error
If you want to report an error, or if you want to make a suggestion, send us an e-mail: [email protected]
Top Tutorials
Top references, top examples, get certified.

- Data Structures & Algorithms
- DSA - Overview
- DSA - Environment Setup
- DSA - Algorithms Basics
- DSA - Asymptotic Analysis
- Data Structures
- DSA - Data Structure Basics
- DSA - Data Structures and Types
- DSA - Array Data Structure
- Linked Lists
- DSA - Linked List Data Structure
- DSA - Doubly Linked List Data Structure
- DSA - Circular Linked List Data Structure
- Stack & Queue
- DSA - Stack Data Structure
- DSA - Expression Parsing
- DSA - Queue Data Structure
- Searching Algorithms
- DSA - Searching Algorithms
- DSA - Linear Search Algorithm
- DSA - Binary Search Algorithm
- DSA - Interpolation Search
- DSA - Jump Search Algorithm
- DSA - Exponential Search
- DSA - Fibonacci Search
- DSA - Sublist Search
- DSA - Hash Table
- Sorting Algorithms
- DSA - Sorting Algorithms
- DSA - Bubble Sort Algorithm
- DSA - Insertion Sort Algorithm
- DSA - Selection Sort Algorithm
- DSA - Merge Sort Algorithm
- DSA - Shell Sort Algorithm
- DSA - Heap Sort
- DSA - Bucket Sort Algorithm
- DSA - Counting Sort Algorithm
- DSA - Radix Sort Algorithm
- DSA - Quick Sort Algorithm
- Graph Data Structure
- DSA - Graph Data Structure
- DSA - Depth First Traversal
- DSA - Breadth First Traversal
- DSA - Spanning Tree
- Tree Data Structure
- DSA - Tree Data Structure
- DSA - Tree Traversal
- DSA - Binary Search Tree
- DSA - AVL Tree
- DSA - Red Black Trees
- DSA - B Trees
- DSA - B+ Trees
- DSA - Splay Trees
- DSA - Tries
- DSA - Heap Data Structure
- DSA - Recursion Algorithms
- DSA - Tower of Hanoi Using Recursion
- DSA - Fibonacci Series Using Recursion
- Divide and Conquer
- DSA - Divide and Conquer
- DSA - Max-Min Problem
- DSA - Strassen's Matrix Multiplication
- DSA - Karatsuba Algorithm
- Greedy Algorithms
- DSA - Greedy Algorithms
- DSA - Travelling Salesman Problem (Greedy Approach)
- DSA - Prim's Minimal Spanning Tree
- DSA - Kruskal's Minimal Spanning Tree
- DSA - Dijkstra's Shortest Path Algorithm
- DSA - Map Colouring Algorithm
- DSA - Fractional Knapsack Problem
- DSA - Job Sequencing with Deadline
- DSA - Optimal Merge Pattern Algorithm
- Dynamic Programming
- DSA - Dynamic Programming
- DSA - Matrix Chain Multiplication
- DSA - Floyd Warshall Algorithm
- DSA - 0-1 Knapsack Problem
- DSA - Longest Common Subsequence Algorithm
- DSA - Travelling Salesman Problem (Dynamic Approach)
- Approximation Algorithms
- DSA - Approximation Algorithms
- DSA - Vertex Cover Algorithm
- DSA - Set Cover Problem
- DSA - Travelling Salesman Problem (Approximation Approach)
- Randomized Algorithms
- DSA - Randomized Algorithms
- DSA - Randomized Quick Sort Algorithm
- DSA - Karger’s Minimum Cut Algorithm
- DSA - Fisher-Yates Shuffle Algorithm
- DSA Useful Resources
- DSA - Questions and Answers
- DSA - Quick Guide
- DSA - Useful Resources
- DSA - Discussion
- Selected Reading
- UPSC IAS Exams Notes
- Developer's Best Practices
- Questions and Answers
- Effective Resume Writing
- HR Interview Questions
- Computer Glossary
Travelling Salesman Problem (Dynamic Approach)
Travelling salesman dynamic programming algorithm, implementation.
Travelling salesman problem is the most notorious computational problem. We can use brute-force approach to evaluate every possible tour and select the best one. For n number of vertices in a graph, there are (n−1)! number of possibilities. Thus, maintaining a higher complexity.
However, instead of using brute-force, using the dynamic programming approach will obtain the solution in lesser time, though there is no polynomial time algorithm.
Let us consider a graph G = (V,E) , where V is a set of cities and E is a set of weighted edges. An edge e(u, v) represents that vertices u and v are connected. Distance between vertex u and v is d(u, v) , which should be non-negative.
Suppose we have started at city 1 and after visiting some cities now we are in city j . Hence, this is a partial tour. We certainly need to know j , since this will determine which cities are most convenient to visit next. We also need to know all the cities visited so far, so that we don't repeat any of them. Hence, this is an appropriate sub-problem.
For a subset of cities S $\epsilon$ {1,2,3,...,n} that includes 1 , and j $\epsilon$ S, let C(S, j) be the length of the shortest path visiting each node in S exactly once, starting at 1 and ending at j .
When |S|> 1 , we define 𝑪 C(S,1)= $\propto$ since the path cannot start and end at 1.
Now, let express C(S, j) in terms of smaller sub-problems. We need to start at 1 and end at j . We should select the next city in such a way that
$$C\left ( S,j \right )\, =\, min\, C\left ( S\, -\, \left\{j \right\},i \right )\, +\, d\left ( i,j \right )\: where\: i\: \epsilon \: S\: and\: i\neq j$$
There are at the most 2 n .n sub-problems and each one takes linear time to solve. Therefore, the total running time is O(2 n .n 2 ) .
In the following example, we will illustrate the steps to solve the travelling salesman problem.

From the above graph, the following table is prepared.
$$Cost\left ( 2,\Phi ,1 \right )\, =\, d\left ( 2,1 \right )\,=\,5$$
$$Cost\left ( 3,\Phi ,1 \right )\, =\, d\left ( 3,1 \right )\, =\, 6$$
$$Cost\left ( 4,\Phi ,1 \right )\, =\, d\left ( 4,1 \right )\, =\, 8$$
$$Cost(i,s)=min\left\{Cos\left ( j,s-(j) \right )\, +\,d\left [ i,j \right ] \right\}$$
$$Cost(2,\left\{3 \right\},1)=d[2,3]\, +\, Cost\left ( 3,\Phi ,1 \right )\, =\, 9\, +\, 6\, =\, 15$$
$$Cost(2,\left\{4 \right\},1)=d[2,4]\, +\, Cost\left ( 4,\Phi ,1 \right )\, =\, 10\, +\, 8\, =\, 18$$
$$Cost(3,\left\{2 \right\},1)=d[3,2]\, +\, Cost\left ( 2,\Phi ,1 \right )\, =\, 13\, +\, 5\, =\, 18$$
$$Cost(3,\left\{4 \right\},1)=d[3,4]\, +\, Cost\left ( 4,\Phi ,1 \right )\, =\, 12\, +\, 8\, =\, 20$$
$$Cost(4,\left\{3 \right\},1)=d[4,3]\, +\, Cost\left ( 3,\Phi ,1 \right )\, =\, 9\, +\, 6\, =\, 15$$
$$Cost(4,\left\{2 \right\},1)=d[4,2]\, +\, Cost\left ( 2,\Phi ,1 \right )\, =\, 8\, +\, 5\, =\, 13$$
$$Cost(2,\left\{3,4 \right\},1)=min\left\{\begin{matrix} d\left [ 2,3 \right ]\,+ \,Cost\left ( 3,\left\{ 4\right\},1 \right )\, =\, 9\, +\, 20\, =\, 29 \\ d\left [ 2,4 \right ]\,+ \,Cost\left ( 4,\left\{ 3\right\},1 \right )\, =\, 10\, +\, 15\, =\, 25 \\ \end{matrix}\right.\, =\,25$$
$$Cost(3,\left\{2,4 \right\},1)=min\left\{\begin{matrix} d\left [ 3,2 \right ]\,+ \,Cost\left ( 2,\left\{ 4\right\},1 \right )\, =\, 13\, +\, 18\, =\, 31 \\ d\left [ 3,4 \right ]\,+ \,Cost\left ( 4,\left\{ 2\right\},1 \right )\, =\, 12\, +\, 13\, =\, 25 \\ \end{matrix}\right.\, =\,25$$
$$Cost(4,\left\{2,3 \right\},1)=min\left\{\begin{matrix} d\left [ 4,2 \right ]\,+ \,Cost\left ( 2,\left\{ 3\right\},1 \right )\, =\, 8\, +\, 15\, =\, 23 \\ d\left [ 4,3 \right ]\,+ \,Cost\left ( 3,\left\{ 2\right\},1 \right )\, =\, 9\, +\, 18\, =\, 27 \\ \end{matrix}\right.\, =\,23$$
$$Cost(1,\left\{2,3,4 \right\},1)=min\left\{\begin{matrix} d\left [ 1,2 \right ]\,+ \,Cost\left ( 2,\left\{ 3,4\right\},1 \right )\, =\, 10\, +\, 25\, =\, 35 \\ d\left [ 1,3 \right ]\,+ \,Cost\left ( 3,\left\{ 2,4\right\},1 \right )\, =\, 15\, +\, 25\, =\, 40 \\ d\left [ 1,4 \right ]\,+ \,Cost\left ( 4,\left\{ 2,3\right\},1 \right )\, =\, 20\, +\, 23\, =\, 43 \\ \end{matrix}\right.\, =\, 35$$
The minimum cost path is 35.
Start from cost {1, {2, 3, 4}, 1}, we get the minimum value for d [1, 2]. When s = 3, select the path from 1 to 2 (cost is 10) then go backwards. When s = 2, we get the minimum value for d [4, 2]. Select the path from 2 to 4 (cost is 10) then go backwards.
When s = 1, we get the minimum value for d [4, 2] but 2 and 4 is already selected. Therefore, we select d [4, 3] (two possible values are 15 for d [2, 3] and d [4, 3], but our last node of the path is 4). Select path 4 to 3 (cost is 9), then go to s = ϕ step. We get the minimum value for d [3, 1] (cost is 6).

Following are the implementations of the above approach in various programming languages −
- Data Structures
- Linked List
- Binary Tree
- Binary Search Tree
- Segment Tree
- Disjoint Set Union
- Fenwick Tree
- Red-Black Tree
- Advanced Data Structures
- Greedy Algorithms
- Greedy Algorithm Tutorial - Examples, Application and Practice Problem
- Greedy Algorithms (General Structure and Applications)
- Difference between Greedy Algorithm and Divide and Conquer Algorithm
- Greedy approach vs Dynamic programming
- Comparison among Greedy, Divide and Conquer and Dynamic Programming algorithm
Standard Greedy algorithms
- Activity Selection Problem | Greedy Algo-1
- Job Sequencing Problem
- Huffman Coding | Greedy Algo-3
- Huffman Decoding
- Water Connection Problem
- Greedy Algorithm for Egyptian Fraction
- Policemen catch thieves
- Fitting Shelves Problem
- Assign Mice to Holes
Greedy algorithm on Array
- Minimum product subset of an array
- Maximize array sum after K negations using Sorting
- Minimum sum of product of two arrays
- Minimum sum of absolute difference of pairs of two arrays
- Minimum increment/decrement to make array non-Increasing
- Sorting array with reverse around middle
- Sum of Areas of Rectangles possible for an array
- Largest lexicographic array with at-most K consecutive swaps
- Partition into two subsets of lengths K and (N - k) such that the difference of sums is maximum
Greedy algorithm on Operating System
- Program for First Fit algorithm in Memory Management
- Program for Best Fit algorithm in Memory Management
- Program for Worst Fit algorithm in Memory Management
- Program for Shortest Job First (or SJF) CPU Scheduling | Set 1 (Non- preemptive)
- Job Scheduling with two jobs allowed at a time
- Optimal Page Replacement Algorithm
Greedy algorithm on Graph
- Prim’s Algorithm for Minimum Spanning Tree (MST)
- Boruvka's algorithm | Greedy Algo-9
- Dial's Algorithm (Optimized Dijkstra for small range weights)
- Minimum cost to connect all cities
- Number of single cycle components in an undirected graph
Approximate Greedy algorithm for NP complete problems
- Greedy Approximate Algorithm for Set Cover Problem
- Bin Packing Problem (Minimize number of used Bins)
- Graph Coloring Using Greedy Algorithm
- Greedy Approximate Algorithm for K Centers Problem
Approximate solution for Travelling Salesman Problem using MST
Greedy algorithm for special cases of dp.
- Fractional Knapsack Problem
- Greedy Algorithm to find Minimum number of Coins
Some easy problems on Greedy algorithm
- Split n into maximum composite numbers
- Buy Maximum Stocks if i stocks can be bought on i-th day
- Find the minimum and maximum amount to buy all N candies
- Find maximum equal sum of every three stacks
- Divide cuboid into cubes such that sum of volumes is maximum
- Maximum number of customers that can be satisfied with given quantity
- Minimum rotations to unlock a circular lock
- Minimum rooms for m events of n batches with given schedule
- Minimum cost to make array size 1 by removing larger of pairs
- Minimum cost for acquiring all coins with k extra coins allowed with every coin
- Minimum increment by k operations to make all elements equal
- Find minimum number of currency notes and values that sum to given amount
- Smallest subset with sum greater than all other elements
Some medium level problems on Greedy algorithm
- Maximum trains for which stoppage can be provided
- Minimum Fibonacci terms with sum equal to K
- Divide 1 to n into two groups with minimum sum difference
- Paper Cut into Minimum Number of Squares
- Minimum difference between groups of size two
- Minimum Number of Platforms Required for a Railway/Bus Station
- Minimum initial vertices to traverse whole matrix with given conditions
- Largest palindromic number by permuting digits
- Find smallest number with given number of digits and sum of digits
- Lexicographically largest subsequence such that every character occurs at least k times
Some hard problems on Greedy algorithm
- Maximum elements that can be made equal with k updates
- Minimize Cash Flow among a given set of friends who have borrowed money from each other
- Minimum Cost to cut a board into squares
- Minimum cost to process m tasks where switching costs
- Find minimum time to finish all jobs with given constraints
- Minimize the maximum difference between the heights
- Minimum edges to reverse to make path from a source to a destination
- Find the Largest Cube formed by Deleting minimum Digits from a number
- Rearrange characters in a String such that no two adjacent characters are same
- Rearrange a string so that all same characters become d distance away
- Top 20 Greedy Algorithms Interview Questions
We introduced Travelling Salesman Problem and discussed Naive and Dynamic Programming Solutions for the problem in the previous post ,. Both of the solutions are infeasible. In fact, there is no polynomial time solution available for this problem as the problem is a known NP-Hard problem. There are approximate algorithms to solve the problem though. The approximate algorithms work only if the problem instance satisfies Triangle-Inequality.
Triangle-Inequality: The least distant path to reach a vertex j from i is always to reach j directly from i, rather than through some other vertex k (or vertices), i.e., dis(i, j) is always less than or equal to dis(i, k) + dist(k, j). The Triangle-Inequality holds in many practical situations.
When the cost function satisfies the triangle inequality, we can design an approximate algorithm for TSP that returns a tour whose cost is never more than twice the cost of an optimal tour. The idea is to use M inimum S panning T ree (MST). Following is the MST based algorithm.
Algorithm:
- Let 1 be the starting and ending point for salesman.
- Construct MST from with 1 as root using Prim’s Algorithm .
- List vertices visited in preorder walk of the constructed MST and add 1 at the end.
Let us consider the following example. The first diagram is the given graph. The second diagram shows MST constructed with 1 as root. The preorder traversal of MST is 1-2-4-3. Adding 1 at the end gives 1-2-4-3-1 which is the output of this algorithm.
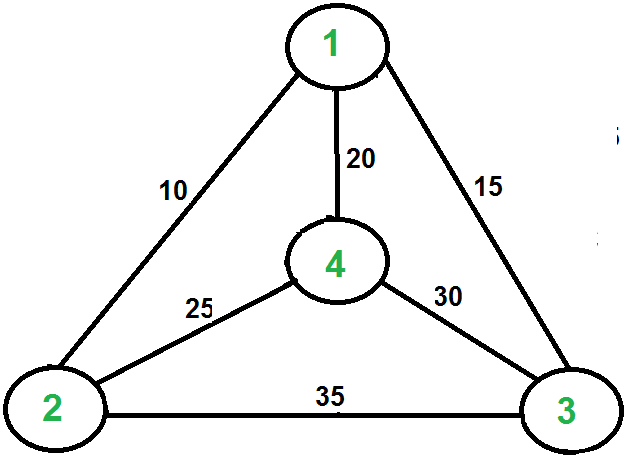
In this case, the approximate algorithm produces the optimal tour, but it may not produce optimal tour in all cases.
How is this algorithm 2-approximate?
The cost of the output produced by the above algorithm is never more than twice the cost of best possible output. Let us see how is this guaranteed by the above algorithm.
Let us define a term full walk to understand this. A full walk is lists all vertices when they are first visited in preorder, it also list vertices when they are returned after a subtree is visited in preorder. The full walk of above tree would be 1-2-1-4-1-3-1.
Following are some important facts that prove the 2-approximateness.
- The cost of best possible Travelling Salesman tour is never less than the cost of MST. (The definition of MST says, it is a minimum cost tree that connects all vertices).
- The total cost of full walk is at most twice the cost of MST (Every edge of MST is visited at-most twice)
- The output of the above algorithm is less than the cost of full walk. In above algorithm, we print preorder walk as output. In preorder walk, two or more edges of full walk are replaced with a single edge. For example, 2-1 and 1-4 are replaced by 1 edge 2-4. So if the graph follows triangle inequality, then this is always true.
From the above three statements, we can conclude that the cost of output produced by the approximate algorithm is never more than twice the cost of best possible solution.
We have discussed a very simple 2-approximate algorithm for the travelling salesman problem. There are other better approximate algorithms for the problem. For example Christofides algorithm is 1.5 approximate algorithm. We will soon be discussing these algorithms as separate posts.
Please Login to comment...
Similar reads.
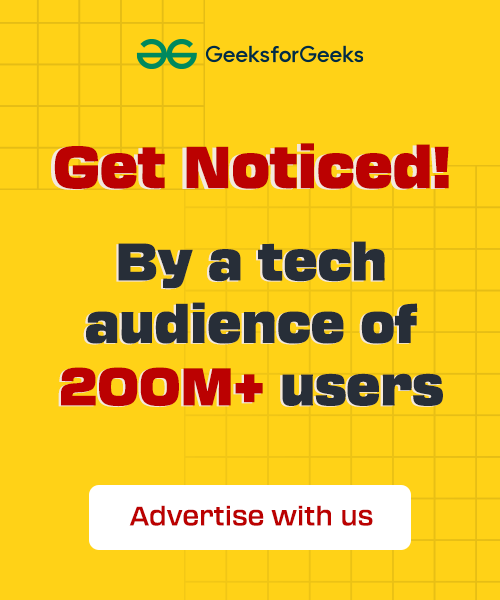
Improve your Coding Skills with Practice
What kind of Experience do you want to share?
TSP Data for the Traveling Salesperson Problem
TSP , a dataset directory which contains some examples of data for the traveleing salesperson problem.
Most of these examples come from TSPLIB, a collection of traveling salesman problem datasets maintained by Gerhard Reinelt at "http://comopt.ifi.uni-heidelberg.de/software/TSPLIB95/"
In the traveling salesperson problem, a salesperson, who lives in one of the cities, is expected to make a round trip visiting all the other cities and returning home. (It doesn't actually matter which city is the starting point.) The requirement is that the total distance traveled be as small as possible.
It turns out that this simple problem is actually remarkably hard to solve, particularly if one insists on finding the absolutely best answer. Approaches to solving this problem have used simple enumeration, Monte Carlo estimation, minimal spanning trees, linear programming, simulated annealing, Tabu searches, the branch and bound procedure, the integer assignment problem, the convex hull, genetic algorithms, ant colony algorithms, and the cross-entropy method,
Thus, this simple problem has been the inspiration for many new ways of trying to solve not just the traveling salesperson problem but related "combinatorial optimization" problems, in which a discrete set of choices result in a payoff which is to be maximized or a cost which is to be minimized.
In the simplest version of the traveling salesperson problem, it is possible to travel from any city A to any city B, and the distance is the same both ways. This might be imagined to correspond to travel by air.
In a variation of the problem, it might not be possible to travel directly between certain cities. This is essentially equivalent to setting the corresponding intercity distance to be infinite. This could be considered an attempt to include the realities of highway travel.
To specify the problem, we may provide the spatial coordinates of the N points. Here again, there can be complications. It would be natural to expect coordinates on a plane, with the Euclidean distance, but the data might be more accurately described as lying on a sphere, with travel constrained to the surface; or we might have locations in a gridded city, in which case distance is measured with the L1 norm rather than the Euclidean norm, and there are further obvious variations.
If we are given a set of coordinates and a description of the distance function, we can compute the distance matrix. So we might suppose that any distance matrix comes from a set of point coordinates. But that's not true. If we start with the distance matrix, we are free to put down numbers that violate the triangle inequality, for instance. Problems in which the data does not come from an underlying set of point coordinates and a norm turn out to be much harder, since certain geometric facts can no longer be used to estimate the solution.
For the data considered here, we will expect symmetry, that is, that the distance is the same coming and going. We will allow problems to be specified either by node coordinates and a norm, (usually 2D planar points and the Euclidean norm) or by an abstract distance matrix.
The computer code and data files described and made available on this web page are distributed under the GNU LGPL license.
Related Data and Programs:
CITIES , a dataset directory which contains sets of information about cities and the distances between them;
CITIES , a FORTRAN90 library which handles various problems associated with a set of "cities" on a map.
CITIES , a MATLAB library which handles various problems associated with a set of "cities" on a map.
DISTANCE_TO_POSITION , a FORTRAN90 program which estimates the positions of cities based on a city-to-city distance table.
DISTANCE_TO_POSITION , a MATLAB program which estimates the positions of cities based on a city-to-city distance table.
DISTANCE_TO_POSITION_SPHERE , a MATLAB program which estimates the positions of cities on a sphere (such as the earth) based on a city-to-city distance table.
LAU_NP , a FORTRAN90 library which implements heuristic algorithms for various NP-hard combinatorial problems.
- Gerhard Reinelt, TSPLIB - A Traveling Salesman Problem Library, ORSA Journal on Computing, Volume 3, Number 4, Fall 1991, pages 376-384.
- att48.tsp , the TSP specification of the data.
- att48_d.txt , the intercity distance table.
- att48_xy.txt , the xy coordinates of each city.
- att48_s.txt , an itinerary that minimizes the total distance.
- dantzig42.tsp , the TSP specification of the data.
- dantzig42_d.txt , the intercity distance table.
- five_d.txt , the intercity distance table.
- five_s.txt , an itinerary that minimizes the total distance.
- fri26.tsp , the TSP specification of the data.
- fri26_d.txt , the intercity distance table.
- fri26_s.txt , an itinerary that minimizes the total distance.
- gr17.tsp , the TSP specification of the data.
- gr17_d.txt , the intercity distance table.
- gr17_s.txt , an itinerary that minimizes the total distance.
- p01.tsp , the TSP specification of the data.
- p01_d.txt , the intercity distance table.
- p01_s.txt , an itinerary that minimizes the total distance.
- p01_sxy.txt , the XY coordinates of the minimal itinerary.
- p01_sxy.png , an image of the minimal itinerary.
- p01_xy.txt , a set of XY coordinates for the cities, inferred from the distances.
- p01_xy.png , an image of the XY coordinates.

- Interview Q
DAA Tutorial
Asymptotic analysis, analysis of sorting, divide and conquer, lower bound theory, sorting in linear time, binary search trees, red black tree, dynamic programming, greedy algorithm, backtracking, shortest path, all-pairs shortest paths, maximum flow, sorting networks, complexity theory, approximation algo, string matching.
Interview Questions
- Send your Feedback to [email protected]
Help Others, Please Share

Learn Latest Tutorials

Transact-SQL

Reinforcement Learning

R Programming

React Native

Python Design Patterns

Python Pillow

Python Turtle

Preparation

Verbal Ability

Company Questions
Trending Technologies

Artificial Intelligence

Cloud Computing

Data Science

Machine Learning

B.Tech / MCA

Data Structures

Operating System

Computer Network

Compiler Design

Computer Organization

Discrete Mathematics

Ethical Hacking

Computer Graphics

Software Engineering

Web Technology

Cyber Security

C Programming

Control System

Data Mining

Data Warehouse

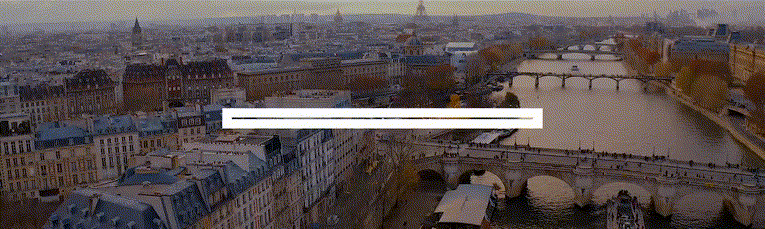
IMAGES
VIDEO
COMMENTS
A TSP tour in the graph is 1-2-4-3-1. The cost of the tour is 10+25+30+15 which is 80. The problem is a famous NP-hard problem. There is no polynomial-time known solution for this problem. Examples: Output of Given Graph: minimum weight Hamiltonian Cycle : 10 + 25 + 30 + 15 := 80.
The traveling salesperson problem has many applications. The obvious ones are in the transportation space. Planning delivery routes or flight patterns, for example, would benefit immensly from breakthroughs is this problem or in the P versus NP problem. However, this same logic can be applied to many facets of planning as well.
For example, branch A in the tree diagram has a sum of 10 + 2 + 11 + 13 = 36 10 + 2 + 11 + 13 = 36. Figure 12.187 Points Along Different Paths. ... Now let's focus our attention on the graph theory application known as the traveling salesperson problem (TSP) ...
Solution of a travelling salesperson problem: the black line shows the shortest possible loop that connects every red dot. The travelling salesman problem, also known as the travelling salesperson problem (TSP), asks the following question: "Given a list of cities and the distances between each pair of cities, what is the shortest possible route that visits each city exactly once and returns ...
Examples of Traveling Salesman Problems I Here are several examples of weighted complete graphs with 5 vertices. I In each case, we're going to perform the Repetitive Nearest-Neighbor Algorithm and Cheapest-Link Algorithm, then see if the results are optimal. I Since N = 5, (N 1)! = 24, so it is feasible to nd the
History Solution to 48 States Traveling Salesman Problem. The origins of the traveling salesman problem are obscure; it is mentioned in an 1832 manual for traveling salesman, which included example tours of 45 German cities but gave no mathematical consideration. 2 W. R. Hamilton and Thomas Kirkman devised mathematical formulations of the problem in the 1800s. 2
This problem is called the Traveling salesman problem (TSP) because the question can be framed like this: Suppose a salesman needs to give sales pitches in four cities. He looks up the airfares between each city, and puts the costs in a graph. ... Going back to our first example, how could we improve the outcome? One option would be to redo the ...
The origin of the traveling salesman problem is not very clear; it is mentioned in an 1832 manual for traveling salesman, which included example tours of 45 German cities but was not formulated as a mathematical problem. However, in the 1800s, mathematicians William Rowan Hamilton and Thomas Kirkman devised mathematical formulations of the problem.
The Traveling Salesman Problem, as we know and love it, was. rst studied in the 1930's in Vienna and Harvard as explained in [3]. Richard M. Karp showed in 1972 that the Hamiltonian cycle problem was NP-complete, which implies the NP-hardness of TSP (see the next section regarding complexity). This supplied.
Output -. Here, the TSP Tour is 0-2-1-3-0 and the cost of the tour is 48. Example 2: Travelling Salesman Problem . Input - Output - Minimum weight Hamiltonian Cycle: EACBDE= 32. Wondering how the Hamiltonian Cycle Problem and the Traveling Salesman Problem differ? The Hamiltonian Cycle problem is to find out if there exists a tour that visits each city exactly once.
Travelling salesman problem takes a graph G {V, E} as an input and declare another graph as the output (say G') which will record the path the salesman is going to take from one node to another. The algorithm begins by sorting all the edges in the input graph G from the least distance to the largest distance. The first edge selected is the ...
Traveling Salesperson Problem. This section presents an example that shows how to solve the Traveling Salesperson Problem (TSP) for the locations shown on the map below. The following sections present programs in Python, C++, Java, and C# that solve the TSP using OR-Tools.
The traveling salesman problem is a classic problem in combinatorial optimization. This problem is finding the shortest path a salesman should take to traverse a list of cities and return to the origin city. ... For example, TSP with 120 cities can be solved in less than 5 seconds on the intel core i7 using this method. Here, "solved" means ...
For example, branch A in the tree diagram has a sum of 10 + 2 + 11 + 13 = 36 10 + 2 + 11 + 13 = 36. Figure 12.214 Points Along Different Paths. ... When the brute force method is impractical for solving a traveling salesperson problem, an alternative is a greedy algorithm known as the nearest neighbor method, which always visit the closest or ...
The Travelling Salesman Problem (TSP) is a very well known problem in theoretical computer science and operations research. The standard version of TSP is a hard problem to solve and belongs to the NP-Hard class. In this tutorial, we'll discuss a dynamic approach for solving TSP. Furthermore, we'll also present the time complexity analysis ...
Example 2 of Travelling Salesman Problem. Input: Output: Minimum Weight Hamiltonian Cycle: EACBDE = 32 Solution of the Travelling Salesman Problem. In the following section, we will discuss different approaches that we will use to solve the problem and their implementations in different programming languages like C, C++, Java, and Python.
Travelling Salesman Problem (TSP) is a classic combinatorics problem of theoretical computer science. The problem asks to find the shortest path in a graph with the condition of visiting all the nodes only one time and returning to the origin city. The problem statement gives a list of cities along with the distances between each city.
The Traveling Salesman Problem states that you are a salesperson and you must visit a number of cities or towns. The Traveling Salesman Problem. Rules: Visit every city only once, then return back to the city you started in. Goal: Find the shortest possible route. Except for the Held-Karp algorithm (which is quite advanced and time consuming ...
Travelling salesman problem is the most notorious computational problem. We can use brute-force approach to evaluate every possible tour and select the best one. For n number of vertices in a graph, there are (n−1)! number of possibilities. Thus, maintaining a higher complexity. However, instead of using brute-force, using the dynamic ...
We have discussed a very simple 2-approximate algorithm for the travelling salesman problem. There are other better approximate algorithms for the problem. For example Christofides algorithm is 1.5 approximate algorithm. We will soon be discussing these algorithms as separate posts. Recommended Problems.
In the simplest version of the traveling salesperson problem, it is possible to travel from any city A to any city B, and the distance is the same both ways. This might be imagined to correspond to travel by air. In a variation of the problem, it might not be possible to travel directly between certain cities.
In order to solve the problem using branch n bound, we use a level order. First, we will observe in which order, the nodes are generated. While creating the node, we will calculate the cost of the node simultaneously. If we find the cost of any node greater than the upper bound, we will remove that node.