
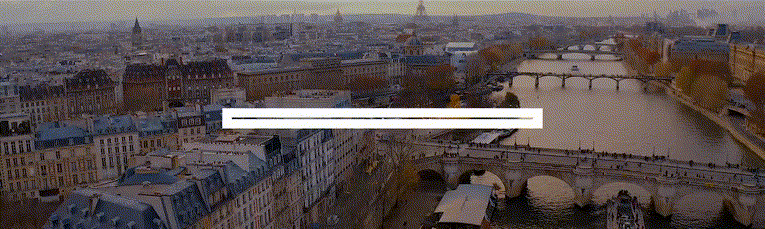
Integrating Operations into Planning and Programming
Communicating tsmo, analysis and performance measurement, regional collaboration and coordination, organizing for operations, mainstreaming tsmo, transportation systems management and operations (tsmo) plans, congestion management process (cmp).
- Regional Concept for Transportation Operations (RCTO)
- How Does TSMO Relate To...
- Brochures and Infographics
- Case Studies and Examples
- Guidance, Primers, and Desk References
- Guides, Reports, and White Papers
- Webinar Archives
Policy and Regulations
- Business Process Frameworks
- Establishing Operations Goals and Objectives
- Setting Operations Objectives
- TSMO Strategies
- Programming and Funding
- Communicating with Decisionmakers
- Communicating with Other Programs
- Analysis and Simulation Tools
- Benefit-Cost Analysis
- Tool for Operations Benefit Cost Analysis
- Performance Measures and System Monitoring
- Operations Data for Planning
How Does TSMO Relate To ...
Performance-based planning, designing for operations, regional its architecture and its strategic plans, systems engineering and its project development.
- Livability, Sustainability and Smart Cities
Traffic Signal Programs
Corridor management, active transportation and demand management, transportation demand management, traffic incident management.
- Travel Time Reliability
All Resources
Resource type, resources helpful to ....
- Metropolitan Planning Organizations
- State Departments of Transportations
- Transit Agencies
- Transportation Decisionmakers
- Local Transportation Agencies
- FHWA and FTA Field Offices
Livability and Sustainability
Reliability.
Home / Transportation Demand Management
Transportation demand management (TDM), or simply demand management, is defined a set of strategies aimed at maximizing traveler choices. Traditionally, TDM has been narrowly defined as commuter ridesharing and its planning application restricted to air quality mitigation (conformity analysis), development mitigation (reducing trip generation rates and parking needs), or efforts to increase multi-modalism in transportation plans. A more contemporary definition of TDM consists of maximizing travel choices, as stated in the definition provided in an FHWA report on TDM:
Managing demand is about providing travelers, regardless of whether they drive alone, with travel choices, such as work location, route, time of travel and mode. In the broadest sense, demand management is defined as providing travelers with effective choices to improve travel reliability. [1]
While transportation systems management and operations is an umbrella term for a set of strategies that includes transportation demand management, it is helpful to focus on specific needs for integrating TDM into existing activities that are carried out under the transportation planning process by States, metropolitan planning organizations, and local agencies. TDM can be effectively integrated into the planning processes at all levels using an objectives-driven, performance-based approach that includes a process for setting specific, measurable objectives for TDM.
OTHER RESOURCES
- Publications and Reference Materials
- Advancing Mode Options in Managed Lane Projects
- Advancing Transportation Demand Management in Resort Towns and Communities
- TSMO and Demand Management
[1] FHWA, Mitigating Traffic Congestion-The Role of Demand-Side Strategies, prepared by ACT, Report No. FHWA-HOP-05-001, October 2004. [ Return to Note 1 ]

- US DOT Home |
- FHWA Home |
- FHWA Operations Home |
- FHWA Planning Home |
- FTA Planning and Environment Home |
- Privacy Policy |

Traffic Demand Management (TDM). Insights, Experiences and Strategies

The old paradigm
A corollary of the “good life” is improved transportation. As in all countries worldwide, accessibility and mobility afforded by transportation are driven mainly by the growth in private car ownership. Correspondingly, the share for public transportation has fallen. In industrialized countries, car dependency is so deeply ingrained that, in the U.S. in particular, car ownership rates exceed an average of one car per licensed driver in many urban areas (Stopher 2004).
Two examples of developing countries are: (1) the number of motorcycles and private passenger cars increased at an average rate of 4.5 percent and 10 percent, respectively in Malaysia from 2000 to 2005 (Kasipillai et Chan, 2008); (2) between 1962 and 1973, the average annual growth rate of motorized vehicles in Singapore was 8.8 % (Chew, 2016).
Road traffic congestion is a critical challenge in many major cities across the world. As congestion is the result of an imbalance between “supply” and “demand”. Congestion mitigation solutions can be divided into two categories: supply-side and demand-side strategies. Supply-side strategies include investments in new transportation infrastructure, such as road network extensions to accommodate travel demand. Previous experiences (Broaddus et al., 2009; Asian Development Bank, 2017) suggest that investing in transportation infrastructure to handle the ever-increasing number of private vehicles would not sufficiently alleviate the congestion problem in the long run. As more road space is taken up by private automobiles, the performance of public transportation will suffer, and non-motorized traffic will be forced out (Broaddus et al., 2009).
A shift of paradigm
Demand-side strategies, on the other hand, employ a variety of tools to manage travel demand in accordance with the capacity of available facilities. In other words, travel demand management focuses on maintaining a balance between the supply (capacity) of the transport system and the demand for travel, thus it provides high potential for reducing traffic congestion, improving environmental conditions, and increasing traffic safety for sustainable urban growth. Many successful cities have alleviated traffic congestion by promoting travel demand management, which reduces demand for travel by private vehicles and encourages non-motorized transport or transit use (Suzuki et al., 2013).
Cities in developing countries often face rapid urbanization and motorization process resulting in many negative externalities (Diao, 2019). Given the constrained living space and limited financial resources, it is not feasible to meet the increased demand via purely investment in the transport infrastructure. Developing nations can avoid most of the urban transport problems through better policies and planning practices that use TDM to maintain a balanced and efficient transportation system. Investment in alternative modes of transportation and TDM initiatives is usually significantly more cost-effective than continuing public investments in growing road and parking facilities, therefore TDM should be a vital and strategic solution for cities in developing countries to alleviate traffic congestion (Federal Highway Administration, 2012).
TDM. Some Definitions
Travel demand management (TDM) is defined as strategies to maximize system efficiency of urban transport by discouraging the use of private transport and promoting more effective, healthy and environmentally friendly modes of transport, including public transport and non-motorized transport (NMT) (Broaddus et al., 2009).
TDM regulates travel demand by influencing people’s travel behavior and providing options to reduce the need for travel, or reduce the number of vehicles. TDM has the potential to deliver an array of benefits across a range of economic, environmental and social objectives such as reducing congestion, saving travel costs, reducing accidents and pollution (Federal Highway Administration, 2012).
TDM has evolved as the policy choice for solving transportation and traffic congestion problems by reducing or restricting travel demand, rather than by providing more transportation facilities (Giuliano & Wachs, 1992). It could be defined as any activity, method or program that reduces vehicle trips, resulting in more efficient use of transportation resources (Dorsey, 2005). IEAust defined TDM as “… an intervention (excluding provision of major infrastructure) to modify travel decisions so that more desirable transport, social, economic and/or environmental objectives can be achieved, and the adverse impacts of travel can be reduced” (Barter, Kenworthy & Laube, 2001).
Some lessons and advice to implement a successful TDM plan
Asian Development Bank in 2017 introduced a comprehensive strategy model of TDM that deployed a three-legged approach: improvement of mobility options, implementation of economic incentives, and enforcement of smart growth – land use policy.
Having clear and realistic objectives is the key to policy success, since policies without clear objectives may lose their way in the long run. Other prerequisites to success of demand management policies include political will and support, strong leadership to coordinate efforts from various jurisdictions and organizations, integrated transport strategies, institutional capacity, and public acceptance (Carran-Fletcher et al., 2020).
To create a successful TDM program, proper policies must be designed at all levels, from strategy to implementation, to coordinate efforts from multiple jurisdictions and organizations toward development objectives while allowing for flexible intervention adaptable to local conditions (Carran-Fletcher et al., 2020).
The following lessons can be drawn from reviewing previous works on TDM policies:
a) No single measure can be effective if used in isolation. Combination of the measures can provide the synergetic effect (Carran-Fletcher et al., 2020).
b) The most effective demand-side techniques are financial incentives and disincentives. Financial disincentives, on the other hand, should be used in tandem with financial incentives to promote strict enforcement (Federal Highway Administration, 2004).
c) Demand management policies should be part of a broader package of transportation policies that includes capital investment and institutional strengthening (Federal Highway Administration, 2004).
d) Only those instruments that are likely to be effective in attaining predefined objectives should be examined. The selected instruments should be politically acceptable and implementable at a reasonable cost (UN-HABITAT, 1995).
Classification of strategies and measures
Factors that affect travel demands include demographics, economic activity, the quality and availability of transport options, land use patterns, cost of travel, and demand management strategies (Litman, 2013).
Broaddus et al. (2009) classified TDM strategies into two main groups: incentives (pull options) that improve travel options like public transport and NMT, and disincentives (push options) that make driving less attractive through economic measures such as high taxes and fees, congestion pricing, and parking management.
Carran-Fletcher et al., (2020) classified the TDM strategies into four categories: improved transportation options, financial incentives, land use planning, and outreach and implementation programs.
TDM strategies use various tools (instruments) to derive TDM measures (solutions). There is a broad range of TDM measures, which can be categorized into: (i) measures that improve mobility options (public transport, active transport modes, ride sharing); (ii) economic measures (congestion pricing, tax increases, parking management); and (iii) smart growth and land-use policies (transit-oriented development, urban compact, integrated transportation planning) (Broaddus et al, 2009).
UN-HABITAT (1995) classified the policy mechanisms for TDM into economic, regulatory and physical categories. Economic instruments are essentially market-based interventions that influence the travel behavior using price incentives or disincentives such as fiscal measures (import taxes, fees, etc. on vehicle ownership, fuel taxes, congestion pricing), or the choice of transport mode (parking fees, parking management). Regulatory measures influence the operations of the transport sector using laws and regulations such as area license schemes, parking controls, and public transport promotions. Physical measures include measures that promote development of physical infrastructures for public transport, walking and cycling (Santos et al., 2010) or measures that induce conscious reduction of road capacity in order to reduce traffic volume such as reduced carriageway widths, traffic calming, road humps. Other measures include staggering of work hours, ride sharing/carpooling, intelligent vehicle systems and enhanced ITS.
List of TDM strategies
Changing behavior
TDM strategies include shifting solo drivers to carpools or transit, allowing more employees to work at home, or adjusting work schedules to avoid peak period auto travel (Giuliano & Wachs, 1992).
The core TDM strategies such as carpooling, vanpooling, transit, bicycling, walking, as well as the promotion of tele-working are supported by strategies such as parking management, rideshare matching, marketing and promotions, incentives and subsidies, and other services (Regional Municipality of Peel, 2004).
Support strategies
TDM support strategies include parking management and parking fees, employee transportation coordinators at area employers, rideshare matching, incentives and subsidies, marketing and promotions, guaranteed ride home, intelligent transportation systems, on-site information and amenities, TDM-friendly site design, High Occupancy Vehicle (HOV ) lanes, Bus Rapid Transit (BRT)/Transit priority and carpool parking lots ( Regional Municipality of Peel , 2004).
Transit related TDM strategies emphasize increasing transit ridership, improving the efficiency of existing services and identifying potential new services to make publicly provided alternatives to single-occupant vehicle travel, including services and facilities that encourage and support other travel modes. Employer-based TDM strategies include private-sector programs and services that encourage employees to change their commuting practices, incentives that make publicly provided travel modes more attractive, disincentives to solo commuting and employer management policies that offer employees flexibility in travel mode choices. Taxing and pricing related TDM strategies affect the cost of transportation and thereby provide monetary disincentives to some travel behaviors (Mahmood et al., 2009).
Smart growth
TDM strategies include smart growth which influences the timing, location, pattern, intensity and budgeting of urban development s (OKI 2030 Regional Transportation Plan, 2004).
Road Taxes, Car Insurance and Polluter Pays Principle
Transportation as a good is essentially under-priced; 32% of the total costs of automobile use are external costs (e.g., pollution) and are paid not by drivers but by society. 24% constitute internal fixed costs (e.g., depreciation) that are not affected by driving distances (Litman 2007).
A pertinent point to be made in quantifying costs associated with automobile usage is that consumers frequently underestimate the cost of running cars by considering only ownership and operating costs without considering other associated external costs (Gardner and Abraham 2006).
A starting point in the way individual perception of road user charging can be altered prior to the imposition of congestion charging involves road tax and car insurance. Altering the charging of both road tax and car insurance from a fixed yearly cost to a per-journey payment is a way of applying the Polluter Pays Principle (PPP). Important to notice that a flat charge that does not address the issue of distance traveled. In fact, flat charges encourage greater usage as, psychologically, users want to lower the per-unit cost of travel (Kasipillai and Chai, 2008).
Elimination of Fuel Subsidies
It is clear that subsidies should be gradually eliminated, as their associated costs, including regulatory and compliance costs on the part of the government and distortions created in the economy, are disproportionately high compared to the benefits garnered. The fuel subsidy mentality dents competitiveness and encourages a consumption ethic (Oxford Business Group, 2006). Fuel taxes encourage a conservation ethic, leading to prudent energy management, greater economic efficiency, and lower pollution emissions (Litman, 2007).
Road pricing
Roa d pricing means that motorists pay directly for the privilege of driving on a particular road or in a particular area ( Victoria Transport Policy Institute, 2007). Co ngestion pricing is a particular type of road pricing where variable fees are charged with an explicit purpose to reduce congestion (Litman 2007a). One policy direction that should be seriously contemplated by the government includes road pricing on a national basis as a way for charging for external costs such as congestion, accidents, and environmental impacts. It is generally accepted that, in line with Pigouvian principles, road pricing should be based on marginal costing principles (Nash, 2007).
Asian Development Bank. (2017). Travel demand management options in Beijing. pp 68.
Barter, P., Kenworthy, J., & Laube. (2001). Lessons from Asia on sustainable urban transport. In N. Low & B.
Gleeson (Eds.), Earth on the Move. London, UK: Palgrave.
Broaddus, A., Litman, T., & Menon G. (2009). Transportation demand management – training document. GTZ, Transport Policy Advisory Services.
Carran-Fletcher, A., Joseph, C., & Thomas, F. (2020). Travel demand management: strategies and outcomes. NZ Transport Agency Research Report 661.
Chew, N. (2016). Walkable and Bikeable Cities: Lessons from Seoul and Singapore. Centre for Liveable Cities Singapore.
Diao, M. (2019). Towards sustainable urban transport in Singapore: Policies and mobility trends. Transp. Policy, 81, 320–330. https://doi.org/10.1016/j.tranpol.2018.05.005.
Dorsey, B. (2005). Mass transit trends and the role of unlimited access in transportation demand management. Journal of Transport Geography, 13, 235-246.
Federal Highway Administration. (2004). Mitigating traffic congestion - the role of demand-side strategies.
Federal Highway Administration. (2012). Integrating demand management into the transportation planning process. Repot No. FHWA-HOP-12-035.
Gardner, B., & Abraham, C. (2006). What drives car use? A grounded theory analysis of commuters’ reasons for driving. Transportation Research Part F, 10(2007), 87-200.
Giuliano, G., & Wachs, M. (1992). Transportation Demand Management as Part of Growth Management. In J. M. Stein (Ed.), Growth Management: The Planning Challenge Of The 1990s. London: Sage Publications.
Kasipillai, J., & Chan, P. (2008). Travel demand management: lessons for Malaysia. Journal of Public Transportation, 11(3), 41-55.
Litman, T.A. (2007). Win-win transportation solutions: Mobility management strategies that provide economic, social and environmental benefits. Victoria Transport Policy Institute.
Litman, T.A. (2007a). Evaluating rail transit benefits: A comment. Transport Policy, 14, 94-97.
Litman, T.A. (2013). Understanding transport demands and elasticities: how prices and other factors affect travel behavior. Victoria Transp. Policy Inst. 1–76.
Mahmood, M., Bashar, M. A., & Akhter, S. (2009). Traffic management system and travel demand management (tdm) strategies: suggestions for urban cities in Bangladesh. Asian Journal of Management and Humanity Sciences, 4(2-3), 161-178.
McKinsey Report. (2020). Comprehensive assessment of public perception on transport systems of 24 cities world-wide.
Nash, C. (2007). Road pricing in Britain. Journal of Transport Economics and Policy, 41(1), 135-147.
Oxford Business Group. (2006). Emerging Malaysia 2006. Oxford Business Group.
OKI 2030 Regional Transportation Plan. (2004). Regional Transportation Plan. Ohio: USA.
Regional Municipality of Peel. (2004). Transportation Demand Management Study. Report No. 1235, Canada.
Santos, G., Behrendt, H., & Teytelboym, A. (2010). Part II: Policies for sustainable road transport. Res. Transp. Econ., 28, 46–91.
Stopher, P.R. (2004). Reducing road congestion: a reality check. Transport Policy, 11, pp.117-131.
Suzuki, H., Cervero, R., & Iuchi, K. (2013). Transforming cities with transit. transit and land use integration for sustainable urban development. 10.1596/978-0-8213-9745-9.
UN-Habitat. (1995). Economic instruments and regulatory measures for demand management of urban transport.
Victoria Transport Policy Institute. (2007). Road pricing: Congestion pricing, value pricing, toll roads and HOT lanes. Online TDM Encyclopedia.

The path of Pontevedra towards a city for people

El Camino de Pontevedra hacia una Ciudad para las Personas
Reordenación del Transporte Público: Una Metodología Estructurada para ciudades medianas
Public Transport Reorganization: A Structured Methodology for medium-sized cities
El Modelo Push, Pull y Mooring aplicado en la Movilidad Urbana
The Push, Pull and Mooring Model applied in Urban Mobility
Strategies and Technologies for the Successful Implementation of Low Emission Zones (LEZ)
Estrategias y Tecnologías para la Implantación Exitosa de las Zonas de Bajas Emisiones (ZBE)
Mobility Payment Systems and MaaS
Sistemas de Pago para la Movilidad Urbana y MaaS
Málaga, Spain
Quality and Environmental Policy
Copyright © Sanvi Consulting Mobility S.L. All rights reserved.
What is Transportation Demand Management
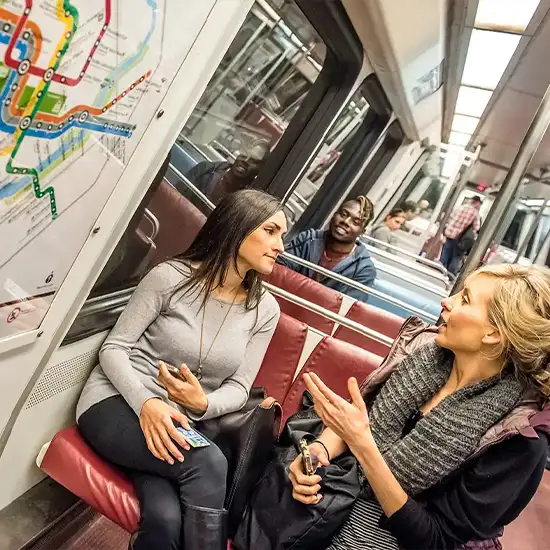
Transportation demand management (TDM) is the successful complement to infrastructure.
TDM focuses on understanding how people make their transportation decisions and influencing people’s behavior to use existing infrastructure in more efficient ways, like reducing single occupancy vehicle trips and getting people to use transit, ridesharing, walking, biking, and telework. TDM is cost effective in guiding the design of transportation and physical infrastructure so that options other than driving are naturally encouraged and transportation systems are better balanced.
How is TDM implemented?
Information, encouragement, incentives.
TDM is a program designed to help people know about and use all their transportation options to optimize all modes in the system and to counterbalance the incentives to drive that are so prevalent in subsidies of parking and roads.
Designing transportation and physical infrastructure
TDM is a principle that should guide everything we do in designing our transportation and physical infrastructure so that alternatives to driving are naturally encouraged and our systems are better balanced.
Traditional and innovative technology based services
TDM is a suite of services that encourage people to use transit, ridesharing, walking, biking, and telework. This includes building and maintaining partnerships with employers and comprehensive promotional programs.
What makes up a robust TDM strategy?
Mobility Lab believes there are seven basic parts of a robust TDM strategy that have the power to shift trips:
- Information
- Marketing business benefits to employers
- Comprehensive programs with mutually reinforcing services, such as transit, carpool/vanpool, bike, walk, transit stores
- Incentives for transit and alternate modes
- Disincentives for driving, which is where parking supply and pricing, tolls, and congestion pricing come in
- Ordinances and development conditions
- Trip caps or maximum average vehicle occupancy
Where do you see the impacts of TDM?
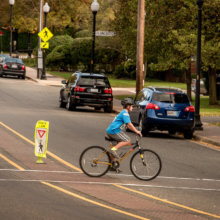
Measuring the benefits of TDM?
Learn how to estimate the benefits of your TDM program with the TDM ROI Calculator or planned expansions of service with TRIMMS 4.0 . Both tools will be valuable additions to your evaluation methods.
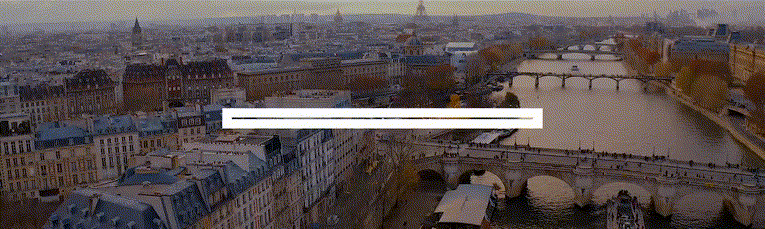
Want to learn more about TDM?
As a transportation policy maker, funder, planner, TDM practitioner, employer, or real estate developer, quantifying costs and benefits of TDM programming to justify the investment and compare it to other transportation improvements can be complex. Here are some resources to help explain:
- List of the top TDM strategies
- 5 ways TDM is invisible (and why that should change)
- Rebranding “TDM” could fix the industry’s communications struggle
- TDM is not scary: A 101 on transportation demand management
- Inside Arlington County’s TDM Bureau
- Overview of Arlington County TDM Commuter Programs
- Victoria Transport Policy Institute’s TDM Encyclopedia
- Association for Commuter Transportation
- Center for Urban Transportation Research at the University of South Florida
- U.S. DOT Federal Highway Administration TDM Strategies
- And, of course, Wikipedia
The Mobility Lab team greatly appreciates your feedback.
If you would like to read our Express Newsletter featuring our latest TDM research and commentary sent directly to your inbox, click to Subscribe.
Mobility Lab
1501 Wilson Boulevard, Suite 1100
Arlington, Virginia 22209
703.228.6558
For all other general inquiries,
please email:
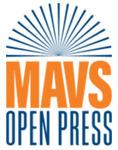
Want to create or adapt books like this? Learn more about how Pressbooks supports open publishing practices.
Part III: Travel Demand Modeling
9 Introduction to Transportation Modeling: Travel Demand Modeling and Data Collection
Chapter overview.
Chapter 9 serves as an introduction to travel demand modeling, a crucial aspect of transportation planning and policy analysis. As explained in previous chapters, the spatial distribution of activities such as employment centers, residential areas, and transportation systems mutually influence each other. The utilization of travel demand forecasting techniques leads to dynamic processes in urban areas. A comprehensive grasp of travel demand modeling is imperative for individuals involved in transportation planning and implementation.
This chapter covers the fundamentals of the traditional four-step travel demand modeling approach. It delves into the necessary procedures for applying the model, including establishing goals and criteria, defining scenarios, developing alternatives, collecting data, and conducting forecasting and evaluation.
Following this chapter, each of the four steps will be discussed in detail in Chapters 10 through 13.
Learning Objectives
- Describe the need for travel demand modeling in urban transportation and relate it to the structure of the four-step model (FSM).
- Summarize each step of FSM and the prerequisites for each in terms of data requirement and model calibration.
- Summarize the available methods for each of the first three steps of FSM and compare their reliability.
- Identify assumptions and limitations of each of the four steps and ways to improve the model.
Introduction
Transportation planning and policy analysis heavily rely on travel demand modeling to assess different policy scenarios and inform decision-making processes. Throughout our discussion, we have primarily explored the connection between urban activities, represented as land uses, and travel demands, represented by improvements and interventions in transportation infrastructure. Figure 9.1 provides a humorous yet insightful depiction of the transportation modeling process. In preceding chapters, we have delved into the relationship between land use and transportation systems, with the houses and factories in the figure symbolizing two crucial inputs into the transportation model: households and jobs. The output of this model comprises transportation plans, encompassing infrastructure enhancements and programs. Chapter 9 delves into a specific model—travel demand modeling. For further insights into transportation planning and programming, readers are encouraged to consult the UTA OERtransport book, “Transportation Planning, Policies, and History.”
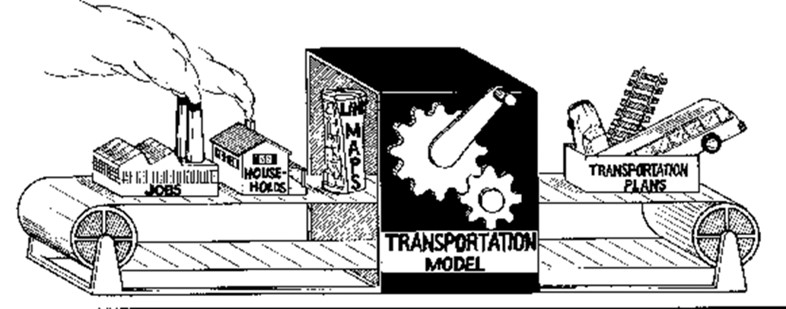
Travel demand models forecast how people will travel by processing thousands of individual travel decisions. These decisions are influenced by various factors, including living arrangements, the characteristics of the individual making the trip, available destination options, and choices regarding route and mode of transportation. Mathematical relationships are used to represent human behavior in these decisions based on existing data.
Through a sequential process, transportation modeling provides forecasts to address questions such as:
- What will the future of the area look like?
- What is the estimated population for the forecasting year?
- How are job opportunities distributed by type and category?
- What are the anticipated travel patterns in the future?
- How many trips will people make? ( Trip Generation )
- Where will these trips end? ( Trip Distribution )
- Which transportation mode will be utilized? ( Mode Split )
- What will be the demand for different corridors, highways, and streets? ( Traffic Assignment )
- Lastly, what impact will this modeled travel demand have on our area? (Rahman, 2008).
9.2 Four-step Model
According to the questions above, Transportation modeling consists of two main stages, regarding the questions outlined above. Firstly, addressing the initial four questions involves demographic and land use analysis, which incorporates the community vision collected through citizen engagement and input. Secondly, the process moves on to the four-step travel demand modeling (FSM), which addresses questions 5 through 8. While FSM is generally accurate for aggregate calculations, it may occasionally falter in providing a reliable test for policy scenarios. The limitations of this model will be explored further in this chapter.
In the first stage, we develop an understanding of the study area from demographic information and urban form (land-use distribution pattern). These are important for all the reasons we discussed in this book. For instance, we must obtain the current age structure of the study area, based on which we can forecast future birth rates, death, and migrations (Beimborn & Kennedy, 1996).
Regarding economic forecasts, we must identify existing and future employment centers since they are the basis of work travel, shopping travel, or other travel purposes. Empirically speaking, employment often grows as the population grows, and the migration rate also depends on a region’s economic growth. A region should be able to generate new employment while sustaining the existing ones based upon past trends and form the basis for judgment for future trends (Mladenovic & Trifunovic, 2014).
After forecasting future population and employment, we must predict where people go (work, shop, school, or other locations). Land-use maps and plans are used in this stage to identify the activity concentrations in the study area. Future urban growth and land use can follow the same trend or change due to several factors, such as the availability of open land for development and local plans and zoning ordinances (Beimborn & Kennedy, 1996). Figure 9.3 shows different possible land-use patterns frequently seen in American cities.
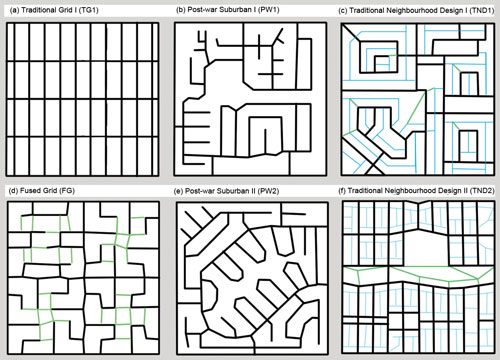
Land-use pattern can also be forecasted through the integration of land use and transportation as we explored in previous chapters.
Figure 9.3 above shows a simple structure of the second stage of FSM.
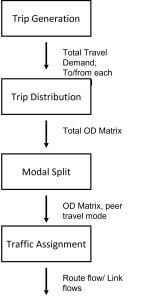
Once the number and types of trips are predicted, they are assigned to various destinations and modes. In the final step, these trips are allocated to the transportation network to compute the total demand for each road segment. During this second stage, additional choices such as the time of travel and whether to travel at all can be modeled using choice models (McNally, 2007). Travel forecasting involves simulating human behavior through mathematical series and calculations, capturing the sequence of decisions individuals make within an urban environment.
The first attempt at this type of analysis in the U.S. occurred during the post-war development period, driven by rapid economic growth. The influential study by Mitchell and Rapkin (1954) emphasized the need to establish a connection between travel and activities, highlighting the necessity for a comprehensive framework. Initial development models for trip generation, distribution, and diversion emerged in the 1950s, leading to the application of the four-step travel demand modeling (FSM) approach in a transportation study in the Chicago area. This model was primarily highway-oriented, aiming to compare new facility development and improved traffic engineering. In the 1960s, federal legislation mandated comprehensive and continuous transportation planning, formalizing the use of FSM. During the 1970s, scholars recognized the need to revise the model to address emerging concerns such as environmental issues and the rise of multimodal transportation systems. Consequently, enhancements were made, leading to the development of disaggregate travel demand forecasting and equilibrium assignment methods that complemented FSM. Today, FSM has been instrumental in forecasting travel demand for over 50 years (McNally, 2007; Weiner, 1997).
Initially outlined by Mannheim (1979), the basic structure of FSM was later expanded by Florian, Gaudry, and Lardinois (1988). Figure 9.3 illustrates various influential components of travel demand modeling. In this representation, “T” represents transportation, encompassing all elements related to the transportation system and its services. “A” denotes the activity system, defined according to land-use patterns and socio-demographic conditions. “P” refers to transportation network performance. “D,” which stands for demand, is generated based on the land-use pattern. According to Florian, Gaudry, and Lardinois (1988), “L” and “S” (location and supply procedures) are optional parts of FSM and are rarely integrated into the model.
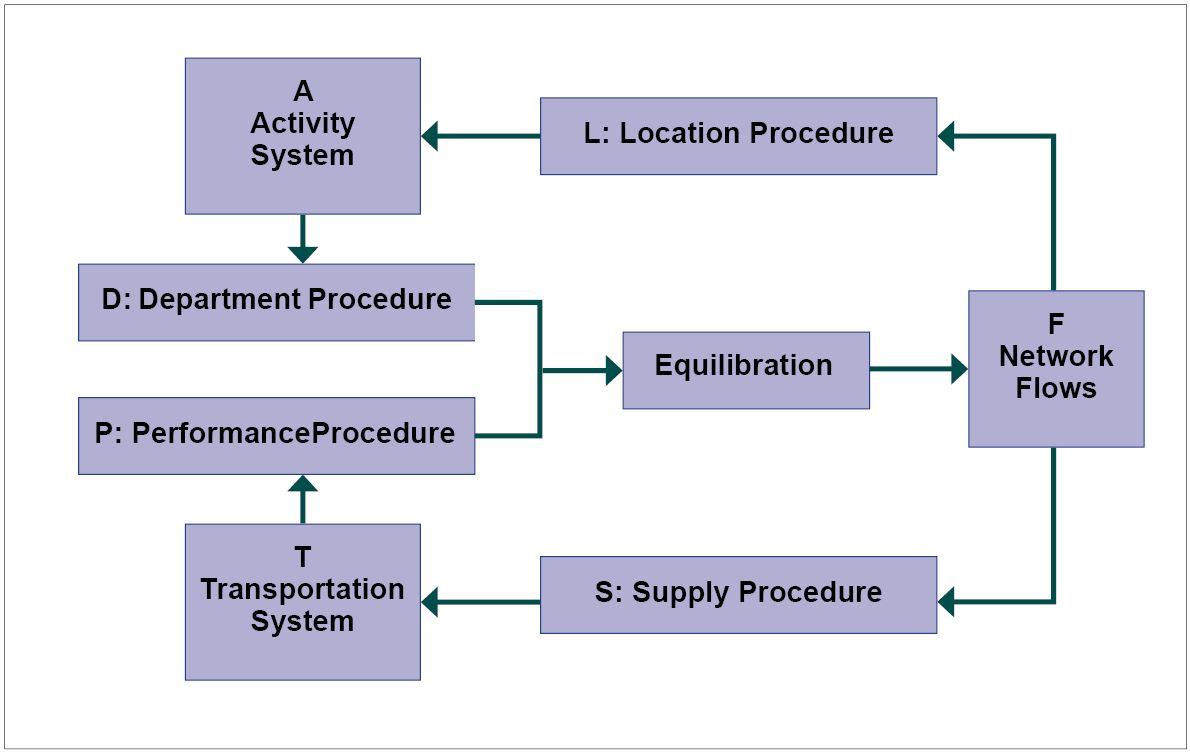
A crucial aspect of the process involves understanding the input units, which are defined both spatially and temporally. Demand generates person trips, which encompass both time and space (e.g., person trips per household or peak-hour person trips per zone). Performance typically yields a level of service, defined as a link volume capacity ratio (e.g., freeway vehicle trips per hour or boardings per hour for a specific transit route segment). Demand is primarily defined at the zonal level, whereas performance is evaluated at the link level.
It is essential to recognize that travel forecasting models like FSM are continuous processes. Model generation takes time, and changes may occur in the study area during the analysis period.
Before proceeding with the four steps of FSM, defining the study area is crucial. Like most models discussed, FSM uses traffic analysis zones (TAZs) as the geographic unit of analysis. However, a higher number of TAZs generally yield more accurate results. The number of TAZs in the model can vary based on its purpose, data availability, and vintage. These zones are characterized or categorized by factors such as population and employment. For modeling simplicity, FSM assumes that trip-making begins at the center of a zone (zone centroid) and excludes very short trips that start and end within a TAZ, such as those made by bike or on foot.
Furthermore, highway systems and transit systems are considered as networks in the model. Highway or transit line segments are coded as links, while intersections are represented as nodes. Data regarding network conditions, including travel times, speeds, capacity, and directions, are utilized in the travel simulation process. Trips originate from trip generation zones, traverse a network of links and nodes, and conclude at trip attraction zones.
Trip Generation
Trip generation is the first step in the FSM model. This step defines the magnitude of daily travel in the study area for different trip purposes. It will also provide us with an estimate of the total trips to and from each zone, creating a trip production and attraction matrix for each trip’s purpose. Trip purposes are typically categorized as follows:
- Home-based work trips (work trips that begin or end at home),
- Home-based shopping trips,
- Home-based other trips,
- School trips,
- Non-home-based trips (trips that neitherbeginnorendathome),
- Trucktrips,and
- Taxitrips(Ahmed,2012).
Trip attractions are based on the level of employment in a zone. In the trip generation step, the assumptions and limitations are listed below:
- Independent decisions: Travel behavior is affected by many factors generated within a household; the model ignores most of these factors. For example, childcare may force people to change their travel plans.
- Limited trip purposes: This model consists of a limited number of trip purposes for simplicity, giving rise to some model limitations. Take shopping trips, for example; they are all considered in the same weather conditions. Similarly, we generate home-based trips for various purposes (banking, visiting friends, medical reasons, or other purposes), all of which are affected by factors ignored by the model.
- Trip combinations: Travelers are often willing to combine various trips into a chain of short trips. While this behavior creates a complex process, the FSM model treats this complexity in a limited way.
- Feedback, cause, and effect problems: Trip generation often uses factors that are a function of the number of trips. For instance, for shopping trip attractions in the FSM model, we assume they are a retail employment function. However, it is logical to assume how many customers these retail centers attract. Alternatively, we can assume that the number of trips a household makes is affected by the number of private cars they own. Nevertheless, the activity levels of families determine the total number of cars.
As mentioned, trip generation process estimations are done separately for each trip purpose. Equations 1 and 2 show the function of trip generation and attraction:

where Oi and Dj trip are generated and attracted respectively, x refers to socio-economic characteristics, and y refers to land-use properties.
Generally, FSM aggregates different trip purposes previously listed into three categories: home-based work trips (HBW) , home-based other (or non-work) trips (HBO) , and non-home-based trips (NHB) . Trip ends are either the origin (generation) or destination (attraction), and home-end trips comprise most trips in a study area. We can also model trips at different levels, such as zones, households, or person levels (activity-based models). Household-level models are the most common scale for trip productions, and zonal-level models are appropriate for trip attractions (McNally, 2007).
There are three main methods for a trip generation or attraction.
- The first method is multiple regression based on population, jobs, and income variables.
- The second method in this step is experience-based analysis, which can show us the ratio of trips generated frequently.
- The third method is cross-classification . Cross-classification is like the experience-based analysis in that it uses trip rates but in an extended format for different categories of trips (home-based trips or non-home-based trips) and different attributes of households, such as car ownership or income.
Elaborating on the differences between these methods, category analysis models are more common for the trip generation model, while regression models demonstrate better performance for trip attractions (Meyer, 2016). Production models are recognized to be influenced by a range of explanatory and policy-sensitive variables (e.g., car ownership, household income, household size, and the number of workers). However, estimation is more problematic for attraction models because regional travel surveys are at the household level (thus providing more accurate data for production models) and not for nonresidential land uses (which is important for trip attraction). Additionally, estimation can be problematic because explanatory trip attraction variables may usually underperform (McNally, 2007). For these reasons, survey data factoring is required prior to relating sample trips to population-level attraction variables, typically achieved via regression analysis. Table 9.1 shows the advantages and disadvantages of each of these two models.
Trip Distribution
Thus far, the number of trips beginning or ending in a particular zone have been calculated. The second step explores how trips are distributed between zones and how many trips are exchanged between two zones. Imagine a shopping trip. There are multiple options for accessible shopping malls accessible. However, in the end, only one will be selected for the destination. This information is modeled in the second step as a distribution of trips. The second step results are usually a very large Origin-Destination (O-D) matrix for each trip purpose. The O-D matrix can look like the table below (9.2), in which sum of Tij by j shows us the total number of trips attracted in zone J and the sum of Tij by I yield the total number of trips produced in zone I.
Up to this point, we have calculated the number of trips originating from or terminating in a specific zone. The next step involves examining how these trips are distributed across different zones and how many trips are exchanged between pairs of zones. To illustrate, consider a shopping trip: there are various options for reaching shopping malls, but ultimately, only one option is chosen as the destination. This process is modeled in the second step as the distribution of trips. The outcome of this step typically yields a large Origin-Destination (O-D) matrix for each trip purpose. An O-D matrix might resemble the table below (9.2), where the sum of Tij by j indicates the total number of trips attracted to zone J, and the sum of Tij by I represents the total number of trips originating from zone I.
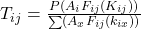
T ij = trips produced at I and attracted at j
P i = total trip production at I
A j = total trip attraction at j
F ij = a calibration term for interchange ij , (friction factor) or travel
time factor ( F ij =C/t ij n )
C= calibration factor for the friction factor
K ij = a socioeconomic adjustment factor for interchange ij
i = origin zone
n = number of zones
Different methods (units) in the gravity model can be used to perform distance measurements. For instance, distance can be represented by time, network distance, or travel costs. For travel costs, auto travel cost is the most common and straightforward way of monetizing distance. A combination of different costs, such as travel time, toll payments, parking payments, etc., can also be used. Alternatively, a composite cost of both car and transit costs can be used (McNally, 2007).
Generalized travel costs can be a function of time divided into different segments. For instance, public transit time can be divided into the following segments: in-vehicle time, walking time, waiting time, interchange time, fare, etc. Since travelers perceive time value differently for each segment (like in-vehicle time vs. waiting time), weights are assigned based on the perceived value of time (VOT). Similarly, car travel costs can be categorized into in-vehicle travel time or distance, parking charge, tolls, etc.
As with the first step in the FSM model, the second step has assumptions and limitations that are briefly explained below.
- Constant trip times: In order to utilize the model for prediction, it assumes that the duration of trips remains constant. This means that travel distances are measured by travel time, and the assumption is that enhancements in the transportation system, which reduce travel times, are counterbalanced by the separation of origins and destinations.
- Automobile travel times to represent distance: We utilize travel time as a proxy for travel distance. In the gravity model, this primarily relies on private car travel time and excludes travel times via other modes like public transit. This leads to a broader distribution of trips.
- Limited consideration of socio-economic and cultural factors: Another drawback of the gravity model is its neglect of certain socio-economic or cultural factors. Essentially, this model relies on trip production and attraction rates along with travel times between them for predictions. Consequently, it may overestimate trip rates between high-income groups and nearby low-income Traffic Analysis Zones (TAZs). Therefore, incorporating more socio-economic factors into the model would enhance accuracy.
- Feedback issues: The gravity model’s reliance on travel times is heavily influenced by congestion levels on roads. However, measuring congestion proves challenging, as discussed in subsequent sections. Typically, travel times are initially assumed and later verified. If the assumed values deviate from actual values, they require adjustment, and the calculations need to be rerun.
Mode choice
FSM model’s third step is a mode-choice estimation that helps identify what types of transportation travelers use for different trip purposes to offer information about users’ travel behavior. This usually results in generating the share of each transportation mode (in percentages) from the total number of trips in a study area using the utility function (Ahmed, 2012). Performing mode-choice estimations is crucial as it determines the relative attractiveness and usage of various transportation modes, such as public transit, carpooling, or private cars. Modal split analysis helps evaluate improvement programs or proposals (e.g., congestion pricing or parking charges) aimed at enhancing accessibility or service levels. It is essential to identify the factors contributing to the utility and disutility of different modes for different travel demands (Beimborn & Kennedy, 1996). Comparing the disutility of different modes between two points aids in determining mode share. Disutility typically refers to the burdens of making a trip, such as time, costs (fuel, parking, tolls, etc.). Once disutility is modeled for different trip purposes between two points, trips can be assigned to various modes based on their utility. As discussed in Chapter 12, a mode’s advantage in terms of utility over another can result in a higher share of trips using that mode.
The assumptions and limitations for this step are outlined as follows:
- Choices are only affected by travel time and cost: This model assumes that changes in mode choices occur solely if transportation cost or travel time in the transportation network or transit system is altered. For instance, a more convenient transit mode with the same travel time and cost does not affect the model’s results.
- Omitted factors: Certain factors like crime, safety, and security, which are not included in the model, are assumed to have no effect, despite being considered in the calibration process. However, modes with different attributes regarding these omitted factors yield no difference in the results.
- Simplified access times: The model typically overlooks factors related to the quality of access, such as neighborhood safety, walkability, and weather conditions. Consequently, considerations like walkability and the impact of a bike-sharing program on the attractiveness of different modes are not factored into the model.
- Constant weights: The model assumes that the significance of travel time and cost remains constant for all trip purposes. However, given the diverse nature of trip purposes, travelers may prioritize travel time and cost differently depending on the purpose of their trip.
The most common framework for mode choice models is the nested logit model, which can accommodate various explanatory variables. However, before the final step, results need to be aggregated for each zone (Koppelman & Bhat, 2006).
A generalized modal split chart is depicted in Figure 9.5.
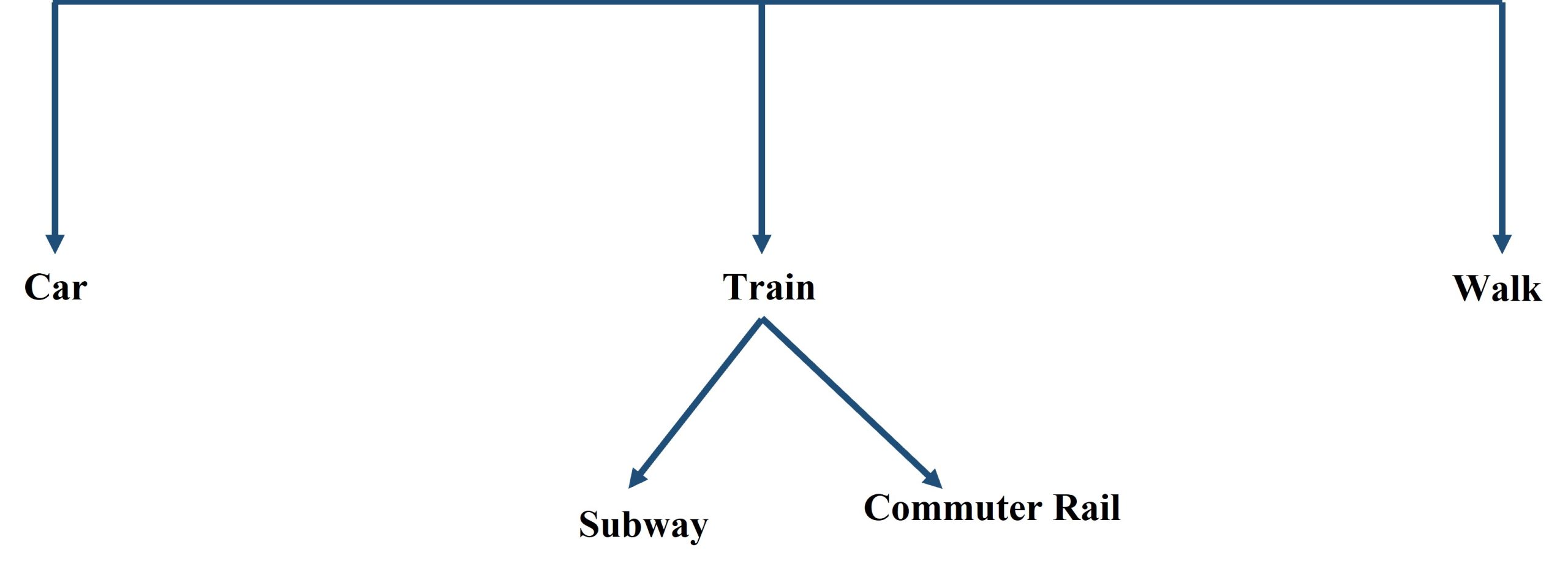
In our analysis, we can use binary logit models (dummy variable for dependent variable) if we have two modes of transportation (like private cars and public transit only). A binary logit model in the FSM model shows us if changes in travel costs would occur, such as what portion of trips changes by a specific mode of transport. The mathematical form of this model is:

where: P_ij 1= The proportion of trips between i and j by mode 1 . Tij 1= Trips between i and j by mode 1.
Cij 1= Generalized cost of travel between i and j by mode 1 .
Cij^2= Generalized cost of travel between i and j by mode 2 .
b= Dispersion Parameter measuring sensitivity to cost.
It is also possible to have a hierarchy of transportation modes for using a binary logit model. For instance, we can first conduct the analysis for the private car and public transit and then use the result of public transit to conduct a binary analysis between rail and bus.
Trip assignment
After breaking down trip counts by mode of transportation, we analyze the routes commuters take from their starting point to their destination, especially for private car trips. This process is known as trip assignment and is the most intricate stage within the FSM model. Initially, the minimum path assigns trips for each origin-destination pair based on either travel costs or time. Subsequently, the assigned volume of trips is compared to the capacity of the route to determine if congestion would occur. If congestion does happen (meaning that traffic volume exceeds capacity), the speed of the route needs to be decreased, resulting in increased travel costs or time. When the Volume/Capacity ratio (v/c ratio) changes due to congestion, it can lead to alterations in both speed and the shortest path. This characteristic of the model necessitates an iterative process until equilibrium is achieved.
The process for public transit is similar, but with one distinction: instead of adjusting travel times, headways are adjusted. Headway refers to the time between successive arrivals of a vehicle at a stop. The duration of headways directly impacts the capacity and volume for each transit vehicle. Understanding the concept of equilibrium in the trip assignment step is crucial because it guides the iterative process of the model. The conclusion of this process is marked by equilibrium, a concept known as Wardrop equilibrium. In Wardrop equilibrium, traffic naturally organizes itself in congested networks so that individual commuters do not switch routes to reduce travel time or costs. Additionally, another crucial factor in this step is the time of day.
Like previous steps, the following assumptions and limitations are pertinent to the trip assignment step:
1. Delays on links: Most traffic assignment models assume that delays occur on the links, not the intersections. For highways with extensive intersections, this can be problematic because intersections involve highly complex movements. Intersections are excessively simplified if the assignment process does not modify control systems to reach an equilibrium.
2. Points and links are only for trips: This model assumes that all trips begin and finish at a single point in a zone (centroids), and commuters only use the links considered in the model network. However, these points and links can vary in the real world, and other arterials or streets might be used for commutes.
3. Roadway capacities: In this model, a simple assumption helps determine roadways’ capacity. Capacity is found based on the number of lanes a roadway provides and the type of road (highway or arterial).
4. Time of the day variations: Traffic volume varies greatly throughout the day and week. In this model, a typical workday of the week is considered and converted to peak hour conditions. A factor used for this step is called the hour adjustment factor. This value is critical because a small number can result in a massive difference in the congestion level forecasted on the model.
5. Emphasis on peak hour travel: The model forecasts for the peak hour but does not forecast for the rest of the day. The models make forecasts for a typical weekday but neglect specific conditions of that time of the year. After completing the fourth step, precise approximations of travel demand or traffic count on each road are achieved. Further models can be used to simulate transportation’s negative or positive externalities. These externalities include air pollution, updated travel times, delays, congestion, car accidents, toll revenues, etc. These need independent models such as emission rate models (Beimborn & Kennedy, 1996).
The basic equilibrium condition point calculation is an algorithm that involves the computation of minimum paths using an all-or-nothing (AON) assignment model to these paths. However, to reach equilibrium, multiple iterations are needed. In AON, it is assumed that the network is empty, and a free flow is possible. The first iteration of the AON assignment requires loading the traffic by finding the shortest path. Due to congestion and delayed travel times, the
previous shortest paths may no longer be the best minimum path for a pair of O-D. If we observe a notable decrease in travel time or cost in subsequent iterations, then it means the equilibrium point has not been reached, and we must continue the estimation. Typically, the following factors affect private car travel times: distance, free flow speed on links, link capacity, link speed capacity, and speed flow relationship .
The relationship between the traffic flow and travel time equation used in the fourth step is:

t= link travel time per length unit
t 0 =free-flow travel time
v=link flow
c=link capacity
a, b, and n are model (calibrated) parameters
Model improvement
Improvements to FSM continue to generate more accurate results. Since transportation dynamics in urban and regional areas are under the complex influence of various factors, the existing models may not be able to incorporate all of them. These can be employer-based trip reduction programs, walking and biking improvement schemes, a shift in departure (time of the day), or more detailed information on socio-demographic and land-use-related factors. However, incorporating some of these variables is difficult and can require minor or even significant modifications to the model and/or computational capacities or software improvements. The following section identifies some areas believed to improve the FSM model performance and accuracy.
• Better data: An effective way of improving the model accuracy is to gather a complete dataset that represents the general characteristics of the population and travel pattern. If the data is out- of-date or incomplete, we will get poor results.
• Better modal split: As you saw in previous sections, the only modes incorporated into the model are private car and public transit trips, while in some cities, a considerable fraction of trips are made by bicycle or by walking. We can improve our models by producing methods to consider these trips in the first and third steps.
• Auto occupancy: In contemporary transportation planning practices, especially in the US, some new policies are emerging for carpooling. We can calculate auto occupancy rates using different mode types, such as carpooling, sensitive to private car trips’ disutility, parking costs, or introducing a new HOV lane.
• Time of the day: In this chapter, the FSM framework discussed is oriented toward peak hour (single time of the day) travel patterns. Nonetheless, understanding the nature of congestion in other hours of the day is also helpful for understanding how travelers choose their travel time.
• A broader trip purpose: Additional trip purposes may provide a better understanding of the
factors affecting different trip purposes and trip-chaining behaviors. We can improve accuracy by having more trip purposes (more disaggregate input and output for the model).
- The concept of access: As discussed, land-use policies that encourage public transit use or create amenities for more convenient walking are not present in the model. Developing factors or indices that reflect such improvements in areas with high demand for non-private vehicles and incorporating them in choice models can be a good improvement.
- Land use feedback: To better understand interactions between land use and travel demand, a land-use simulation model can be added to these steps to determine how a proposed transportation change will lead to a change in land use.
- Intersection delays: As mentioned in the fourth step, intersections in major highways create significant delays. Incorporating models that calculate delays at these intersections, such as stop signs, could be another improvement to the model.
A Simple Example of the FSM model
An example of FSM is provided in this section to illustrate a typical application of this model in the U.S. In the first phase, the specifications about the transportation network and household data are needed. In this hypothetical example, 5 percent of households in each TAZ were sampled and surveyed, which generated 1,955 trips in 200 households. As a hypothetical case study, this sample falls below the standard required for statistical significance but is relevant to demonstrate FSM.
A home interview survey was carried out to gather data from a five percent sample of households in each TAZ. This survey resulted in 1,852 trips from 200 households. It is important to note that the sample size in this example falls below the minimum required for statistical significance, as it is intended for learning purposes only.
Table 9.3 provides network information such as speed limits, number of lanes, and capacity. Table 9.4 displays the total number of households and jobs in three industry sectors for each zone. Additionally, Table 9.5 breaks down the household data into three car ownership groups, which is one of the most significant factors influencing trip making.
In the first step (trip generation), a category model (i.e., cross-classification) helped estimate trips. The sampled population’s sociodemographic and trip data for different purposes helped calculate this estimate. Since research has shown the significant effect of auto ownership on private car trip- making (Ben-Akiva & Lerman, 1974), disaggregating the population based on the number of private cars generates accurate results. Table 9.7 shows the trip-making rate for different income and auto ownership groups.
Also, as mentioned in previous sections, multiple regression estimation analysis can be used to generate the results for the attraction model. Table 9.7 shows the equations for each of the trip purposes.
After estimating production and attraction, the models are used for population data to generate results for the first step. Next, comparing the results of trip production and attraction, we can observe that the total number of trips for each purpose is different. This can be due to using different methods for production and attraction. Since the production method is more reliable, attraction is typically normalized by production. Also, some external zones in our study area are either attracting trips from our zones or generating them. In this case, another alternative is to extend the boundary of the study area and include more zones.
As mentioned, the total number of trips produced and attracted are different in these results. To address this mismatch, we can use a balance factor to come up with the same trip generation and attraction numbers if we want to keep the number of zones within our study area. Alternatively, we can consider some external stations in addition to designated zones. In this example, using the latter seems more rational because, as we saw in Table 9.4, there are more jobs than the number of households aggregately, and our zone may attract trips from external locations.
For the trip distribution step, we use the gravity model. For internal trips, the gravity model is:

and f(tij) is some function of network level of service (LOS)
To apply the gravity model, we need to calculate the impedance function first, which is represented here by travel cost. Table 9.9 shows the minimum travel path between each pair of zones ( skim tree ) in a matrix format in which each cell represents travel time required to travel between the corresponding row and column of that cell.
Table 9.9-Travel cost table (skim tree)
Note. Table adapted from “The Four-Step Model” by M. McNally, In D. A. Hensher, & K. J. Button (Eds.), Handbook of transport modelling , Volume1, p. 5, Bingley, UK: Emerald Publishing. Copyright 2007 by Emerald Publishing.
With having minimum travel costs between each pair of zones, we can calculate the impedance function for each trip purpose using the formula

Table 9.10 shows the model parameters for calculating the impedance function for different trip purposes:
After calculating the impedance function , we can calculate the result of the trip distribution. This stage generates trip matrices since we calculate trips between each zone pair. These matrices are usually in “Origin-Destination” (OD) format and can be disaggregated by the time of day. Field surveys help us develop a base-year trip distribution for different periods and trip purposes. Later, these empirical results will help forecast trip distribution. When processing the surveys, the proportion of trips from the production zone to the attraction zone (P-A) is also generated. This example can be seen in Table 9.11. Looking at a specific example, the first row in table is for the 2-hour morning peak commute time period. The table documents that the production to attraction factor for the home-based work trip is 0.3. Unsurprisingly, the opposite direction, attraction to production zone is 0.0 for this time of day. Additionally, the table shows that the factor for HBO and NHB trips are low but do occur during this time period. This could represent shopping trips or trips to school. Table 9.11 table also contains the information for average occupancy levels of vehicles from surveys. This information can be used to convert person trips to vehicle trips or vice versa.
Table 9.11 Trip distribution rates for different time of the day and trip purposes
The O-D trip table is calculated by adding the multiplication of the P-to-A factor by corresponding cell of the P-A trip table and adding the corresponding cell of the transposed P-A trip table multiplied by the A-to-P factor. These results, which are the final output of second step, are shown in Table 9.12.
Once the Production-Attraction (P-A) table is transformed into Origin-Destination (O-D) format and the complete O-D matrix is computed, the outcomes will be aggregated for mode choice and traffic assignment modeling. Further elaboration on these two steps will be provided in Chapters 11 and 12.
In this chapter, we provided a comprehensive yet concise overview of four-step travel demand modeling including the process, the interrelationships and input data, modeling part and extraction of outputs. The complex nature of cities and regions in terms of travel behavior, the connection to the built environment and constantly growing nature of urban landscape, necessitate building models that are able to forecast travel patterns for better anticipate and prepare for future conditions from multiple perspectives such as environmental preservation, equitable distribution of benefits, safety, or efficiency planning. As we explored in this book, nearly all the land-use/transportation models embed a transportation demand module or sub model for translating magnitude of activities and interconnections into travel demand such as VMT, ridership, congestion, toll usage, etc. Four-step models can be categorized as gravity-based, equilibrium-based models from the traditional approaches. To improve these models, several new extensions has been developed such as simultaneous mode and destination choice, multimodality (more options for mode choice with utility), or microsimulation models that improve granularity of models by representing individuals or agents rather than zones or neighborhoods.
Travel demand modeling are models that predicts the flow of traffic or travel demand between zones in a city using a sequence of steps.
- Intermodality refers to the concept of utilizing two or more travel modes for a trip such as biking to a transit station and riding the light rail.
- Multimodality is a type of transportation network in which a variety of modes such as public transit, rail, biking networks, etc. are offered.
Zoning ordinances is legal categorization of land use policies that permits or prohibits certain built environment factors such as density.
Volume capacity ratio is ratio that divides the demand on a link by the capacity to determine the level of service.
- Zone centroid is usually the geometric center of a zone in modeling process where all trips originate and end.
Home-based work trips (HBW) are the trips that originates from home location to work location usually in the AM peak.
- Home-based other (or non-work) trips (HBO) are the trips that originates from home to destinations other than work like shopping or leisure.
Non-home-based trips (NHB) are the trips that neither origin nor the destination are home or they are part of a linked trip.
Cross-classification is a method for trip production estimation that disaggregates trip rates in an extended format for different categories of trips like home-based trips or non-home-based trips and different attributes of households such as car ownership or income.
- Generalized travel costs is a function of time divided into sections such as in vehicle time vs. waiting time or transfer time in a transit trip.
Binary logit models is a type of logit model where the dependent variable can take only a value of 0 or 1.
- Wardrop equilibrium is a state in traffic assignment model where are drivers are reluctant to change their path because the average travel time is at a minimum.
All-or-nothing (AON) assignment model is a model that assumes all trips between two zones uses the shortest path regardless of volume.
Speed flow relationship is a function that determines the speed based on the volume (flow)
skim tree is structure of travel time by defining minimum cost path for each section of a trip.
Key Takeaways
In this chapter, we covered:
- What travel demand modeling is for and what the common methods are to do that.
- How FSM is structured sequentially, what the relationships between different steps are, and what the outputs are.
- What the advantages and disadvantages of different methods and assumptions in each step are.
- What certain data collection and preparation for trip generation and distribution are needed through a hypothetical example.
Prep/quiz/assessments
- What is the need for regular travel demand forecasting, and what are its two major components?
- Describe what data we require for each of the four steps.
- What are the advantages and disadvantages of regression and cross-classification methods for a trip generation?
- What is the most common modeling framework for mode choice, and what result will it provide us?
- What are the main limitations of FSM, and how can they be addressed? Describe the need for travel demand modeling in urban transportation and relate it to the structure of the four-step model (FSM).
Ahmed, B. (2012). The traditional four steps transportation modeling using a simplified transport network: A case study of Dhaka City, Bangladesh. International Journal of Advanced Scientific Engineering and Technological Research , 1 (1), 19–40. https://discovery.ucl.ac.uk/id/eprint/1418961/
ALMEC, C . (2015). The Project for capacity development on transportation planning and database management in the republic of the Philippines: MMUTIS update and enhancement project (MUCEP) : Project Completion Report . Japan International Cooperation Agency. (JICA) Department of Transportation and Communications (DOTC) . https://books.google.com/books?id=VajqswEACAAJ .
Beimborn, E., and Kennedy, R. (1996). Inside the black box: Making transportation models work for livable communities . Washington, DC: Citizens for a Better Environment and the Environmental Defense Fund. https://www.piercecountywa.gov/DocumentCenter/View/755/A-GuideToModeling?bidId
Ben-Akiva, M., & Lerman, S. R. (1974). Some estimation results of a simultaneous model of auto ownership and mode choice to work. Transportation , 3 (4), 357–376. https://doi.org/10.1007/bf00167966
Ewing, R., & Cervero, R. (2010). Travel and the built environment: A meta-analysis. Journal of the American Planning Association , 76 (3), 265–294. https://doi.org/10.1080/01944361003766766
Florian, M., Gaudry, M., & Lardinois, C. (1988). A two-dimensional framework for the understanding of transportation planning models. Transportation Research Part B: Methodological , 22 (6), 411–419. https://doi.org/10.1016/0191-2615(88)90022-7
Hadi, M., Ozen, H., & Shabanian, S. (2012). Use of dynamic traffic assignment in FSUTMS in support of transportation planning in Florida. Florida International University Lehman Center for Transportation Research. https://rosap.ntl.bts.gov/view/dot/24925
Hansen, W. (1959). How accessibility shapes land use.” Journal of the American Institute of Planners 25 (2): 73–76. https://doi.org/10.1080/01944365908978307
Gavu, E. K. (2010). Network based indicators for prioritising the location of a new urban transport connection: Case study Istanbul, Turkey (Master’s thesis, University of Twente). International Institute for Geo-Information Science and Earth Observation Enschede. http://essay.utwente.nl/90752/1/Emmanuel%20Kofi%20Gavu-22239.pdf
Karner, A., London, J., Rowangould, D., & Manaugh, K. (2020). From transportation equity to transportation justice: Within, through, and beyond the state. Journal of Planning Literature , 35 (4), 440–459. https://doi.org/10.1177/0885412220927691
Kneebone, E., & Berube, A. (2013). Confronting suburban poverty in America . Brookings Institution Press.
Koppelman, Frank S, and Chandra Bhat. (2006). A self instructing course in mode choice modeling: multinomial and nested logit models. U.S. Department of Transportation Federal Transit Administration https://www.caee.utexas.edu/prof/bhat/COURSES/LM_Draft_060131Final-060630.pdf
Manheim, M. L. (1979). Fundamentals of transportation systems analysis. Volume 1: Basic Concepts . The MIT Press https://mitpress.mit.edu/9780262632898/fundamentals-of-transportation-systems-analysis/
McNally, M. G. (2007). The four step model. In D. A. Hensher, & K. J. Button (Eds.), Handbook of transport modelling , Volume1 (pp.35–53). Bingley, UK: Emerald Publishing.
Meyer, M. D., & Institute Of Transportation Engineers. (2016). Transportation planning handbook . Wiley.
Mladenovic, M., & Trifunovic, A. (2014). The shortcomings of the conventional four step travel demand forecasting process. Journal of Road and Traffic Engineering , 60 (1), 5–12.
Mitchell, R. B., and C. Rapkin. (1954). Urban traffic: A function of land use . Columbia University Press. https://doi.org/10.7312/mitc94522
Rahman, M. S. (2008). “ Understanding the linkages of travel behavior with socioeconomic characteristics and spatial Environments in Dhaka City and urban transport policy applications .” Hiroshima: (Master’s thesis, Hiroshima University.) Graduate School for International Development and Cooperation. http://sr-milan.tripod.com/Master_Thesis.pdf
Rodrigue, J., Comtois, C., & Slack, B. (2020). The geography of transport systems . London ; New York Routledge.
Shen, Q. (1998). Location characteristics of inner-city neighborhoods and employment accessibility of low-wage workers. Environment and Planning B: Planning and Design , 25 (3), 345–365.
Sharifiasl, S., Kharel, S., & Pan, Q. (2023). Incorporating job competition and matching to an indicator-based transportation equity analysis for auto and transit in Dallas-Fort Worth Area. Transportation Research Record , 03611981231167424. https://doi.org/10.1177/03611981231167424
Weiner, Edward. 1997. Urban transportation planning in the United States: An historical overview . US Department of Transportation. https://rosap.ntl.bts.gov/view/dot/13691
Xiongbing, J, Grammenos, F. (2013, May, 21) . Taking the Guesswork out of Designing for Walkability. Planetizen . https://www.planetizen.com/node/63248
Home-based other (or non-work) trips (HBO) are the trips that originates from home to destinations other than work like shopping or leisure.
gravity model is a type of accessibility measurement in which the employment in destination and population in the origin defines thee degree of accessibility between the two zones.
Impedance function is a function that convert travel costs (usually time or distance) to the level of difficulty of getting from one location to the other.
Transportation Land-Use Modeling & Policy Copyright © by Mavs Open Press. All Rights Reserved.
Share This Book
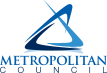
- Meetings and Committees
- Data & Maps
- Contracting Opportunities
- Grants & Funding
- News & Events
- Publications & Documents
- Contact Staff
- Council Fact Sheets
Regional Travel Demand Management Study
The region’s transportation system should be designed to meet the travel needs of all the residents in our growing region. TDM strategies can help meet that need. The Metropolitan Council hired a team led by ICF for the Regional Travel Demand Management Study to evaluate TDM in the region and prepare an Action Plan that will feed into the 2050 Transportation Policy Plan and guide an expanded set of regional TDM activities.
Study goals
The study aimed to understand:
- How TDM can support regional planning goals and objectives
- Short and long-term opportunities and challenges for TDM in the region
- How the Met Council, its planning and implementation partners, and the private and nonprofit sectors can better support TDM
The study outcomes help the Met Council and these implementation partners prepare for leveraging federal investments, and support TDM strategies that are essential to the livability and prosperity of the Twin Cities metropolitan region.
Stakeholder perspectives were critical in making sure that the region can act on the selected TDM strategies. These views helped study outcomes align with the needs and interests of all transportation system users. The study team reached out to a broad group of stakeholders through surveys, interviews, focus groups, and workshops, to hear from voices throughout the region.
A technical advisory team also provided strategic direction and input. The team included a mix of TDM practitioners including planners and policymakers, to provide a range of perspectives. Members included representatives from the Met Council, transit providers, Minnesota Department of Transportation, the four regional transportation management organizations, local governments, and other transportation providers and organizations.
Action plan
The final deliverable of the study was an Action Plan to guide implementation of expanded regional TDM activities.
Regional TDM Action Plan (PDF)
The Action Plan will be summarized in the 2050 Transportation Policy Plan . Implementation of the Action Plan will begin in early 2024.
Study deliverables
The Regional TDM Study prepared a number of memorandums prior to and in support of the Action Plan deliverable. These memorandums describe the study process, outcomes of each phase, and any engagement that was conducted during the different phases of the study.
- Existing Conditions Technical Memorandum (PDF)
- State of Practice Technical Memorandum (PDF)
- Travel Demand Management Framework Technical Memorandum (PDF)
- Future Mobility Considerations and Strengths, Weaknesses, Opportunities, and Threats Analysis Technical Memorandum (PDF)
- Appendix B: Potential TDM Strategy Evaluation Spreadsheet (XLSX)
On request, these documents can be made available in alternative formats. Call Metropolitan Council public information at 651-602-1140 or TTY 651-291-0904.
Previous TDM study
- TDM Evaluation and Implementation Study, August 2010 (PDF, 84 pages)
Search form
Travel demand management.
Travel Demand Management (TDM) is the application of a focused, data-led strategy that seeks to change demand on transport networks by redistributing journeys to other modes, times, routes, or by removing the journey altogether. It is most effectively applied when there is an impetus or catalyst for behaviour change.
A Travel Plan is a management strategy for delivering long-term behavioural change and sustainable travel patterns across an organisation or precinct. It is about understanding how people make their transport decisions and using this to influence behaviours that lead to better customer outcomes, while reducing adverse impacts such as congestion.
Travel Plans work because they are unique, evidence-based and tailored to the specific circumstances of an organisation or site. They lead to real benefits for the organisation, employees and broader community by developing sustainable and commonly agreed solutions.
Transport for NSW has developed a Travel Plan Toolkit designed for people or groups responsible for developing and implementing a Travel Plan. This toolkit provides the steps, templates and resources for developing a comprehensive Travel Plan. Throughout the Toolkit there are links to resources and more detailed information which can also be accessed individually through the links below
Key Documents
Supporting documents, developing your travel plan.
- Travel choices home
- Travel Access Guide - How to
- Travel Access Guide - Checklist
- Travel Access Guide - Sample
- Travel Plan Toolkit
- Travel Plan Toolkit - Hospital Precincts
- Travel Plan Template
- Site audit checklist
- Transport and policy assessment checklist
- Open Data Hub and Developer Portal information
- Survey methods for organisations
- Online staff travel survey
- Tips for analysing survey results
- Sample functions and skills of a travel plan coordinator
- Potential engagement techniques
- Travel Plan review: tips, timing and questions
- Travel plan summary template
- Sample travel plan summaries
- Considerations for hospital travel plans
- Considerations for higher education institution travel plans
- Considerations for large shopping centre travel plans
- Possible travel plan outcomes
- Tips for designing your package of activities
- Examples of ‘hard’ activities
- Examples of ‘soft’ activities
- Travel Choices flexible working toolkit
- Additional references
Questions or comments about the use of this Toolkit, including requests for soft copies of templates, can be directed to [email protected]
Additional resources are also available on the Travel Choices website to help your organisation promote sustainable travel behaviour changes.
Note: If your Travel Plan is a condition of planning consent, you should consult with your relevant planning authority for advice on any additional specific requirements.
RUC FOR ELECTRIC VEHICLES: Road user charges now apply to EVs and plug-in hybrids
SCAM ALERTS: We’ve seen an increase in scam texts and emails claiming to be from NZTA, asking for payments. Learn more about the latest phishing scams or report a phishing scam to us

Online services
- Renew vehicle licence (rego)
- Book, change or cancel a driving test
- Bought a vehicle
- Sold a vehicle
- Change your address
- Buy road user charges (RUC)
- Order replacement labels
- Report a phishing scam
- Access all online services
- Research reports
Research Report 661 Travel demand management: strategies and outcomes
Published: April 2020 | Category: Inclusive access , Research programme , Research & reports | Audience: General
Travel demand management (TDM) is a rapidly changing field. This research drew lessons from international practice to inform New Zealand planning and policy decisions.
The research findings show that a wide range of strategies are in use internationally and the report summarises some key insights from international TDM practice.
Keywords: behaviour change case study, cycling, incentives, mobility management, parking, public transport, transport, travel demand management, walking

Publication details
- Author: A Carran-Fletcher, C Joseph, F Thomas – MRCagney; S Philbin – Traffic Planning Consultants
- Published: April 2020
- Reference: 661
- ISBN / ISSN : 978-1-98-856156-1
- Sign up to receive updates about research reports
Browse section/chapter
- Research Report 661 Travel demand management: strategies and outcomes — 188 pages, 4.4 MB
- Research Report 661 Travel demand management: strategies and outcomes – summary — 3 pages, 599 KB

Transportation Demand Management (TDM)
What is transportation demand management.
Transportation demand management (or TDM) is the discipline of encouraging and facilitating traveler behavior that makes more efficient use of the transportation network. Ultimately, this means providing people with more choice about how, where, when, and if they travel: giving them more freedom and flexibility in their work hours and location, for instance, or making it more convenient, feasible, and attractive to take transit, ride a bike, or share their trip with other travelers.
Why is transportation demand management important for Colorado?
Population Growth
Colorado's population is projected to increase by over 600,000 residents by 2030. Facilitating more efficient travel choices will be an essential component of the strategy to maintain the reliability of the travel network as it accommodates more travelers.
Public Finance
Colorado cannot afford to “build our way out” of congestion. By making the most of our existing network, we can maximize the impact of tax dollars and reduce future maintenance bills.
Climate Change
While the state has made good progress toward our 2030 GHG targets, additional transportation strategies are needed to close the remaining gap. Emissions-reducing TDM strategies will be needed in addition to zero emission vehicle transitions.
Air Quality
The negative health impacts of vehicle emissions are well documented. TDM can be critical in reducing ozone and criteria pollutants from transportation.
The pandemic, changing workplace attitudes and policies, labor market shortages and recent transportation policy and investment changes present a critical opportunity.
Landscape of Transportation Demand Management in Colorado
Colorado has a strong tradition of commuter-focused transportation demand management programming, supported by a mixture of regional initiatives - like the Denver Regional Council of Governments' Way to Go Partnership - and programs championed by local governments, transit agencies, and other community and quasi-governmental organizations.
However, many audiences across the state have historically not been part of the conversation, and this has often caused the range of TDM strategies to be constrained by the actors at the table, especially in regard to developing solutions that work for audiences who have not been well-served by traditional TDM efforts - including rural residents, shift workers, caregivers and recreational travelers.
Furthermore, ongoing changes to travel patterns and behaviors in response to the COVID-19 pandemic continue to create fresh challenges for established approaches, and the emergence of new technologies - such as shared micromobility, electric bicycles, and advancements in data analytics - also present invaluable new opportunities for practitioners across the state to tackle varied (and growing) congestion and mobility issues through context-appropriate tools and approaches.
TDM Conference
The Association for Commuter Transportation (ACT) is the premier organization and leading advocate for commuter transportation and transportation demand management (TDM) professionals. With a vision of "A Better Journey for Everyone," ACT strives to create an efficient multimodal transportation system by empowering the people, places, and organizations working to advance TDM in order to improve the quality of life of commuters, enhance the livability of communities, and stimulate economic activity.
The Colorado Department of Transportation (CDOT) Office of Innovative Mobility (OIM) has two TDM funding opportunities under the OIM Grant Program. The OIM Grant Program includes TDM Seed Funding Grants and TDM Innovation Grants.
OIM Grant Program
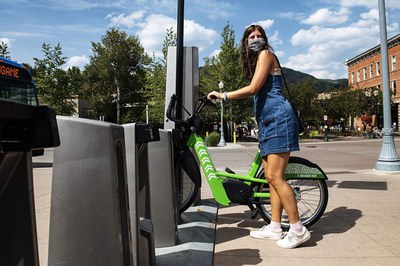
The Colorado Department of Transportation (CDOT) Division of Transit and Rail (DTR) is issuing a Notice of Funding Availability (NOFA) for Federal Transit Administration (FTA) and State transit funds including TDM Seed Funding Grants and TMO Support grants.
Congestion mitigation and air quality improvement program (CMAQ) is designed to assist non-attainment and maintenance areas in attaining the national ambient air quality standards by funding transportation projects and programs that will improve air quality.
Congestion Mitigation Air Quality (CMAQ)
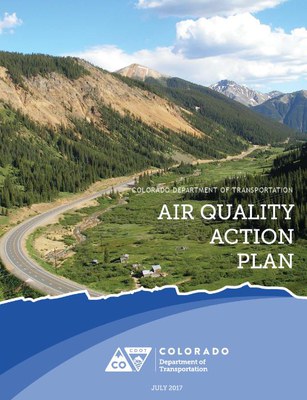
The Revitalizing Main Streets Program began as a part of Colorado’s COVID-19 Recovery Plan , with a $30 million allocation from the state legislature in March 2021. In June 2021, Senate Bill 260 provided $85 million in additional funding for the program over the next 10 years. This program is intended to help communities across the state implement transportation-related projects that improve safety and yield long-term benefits to community main streets. When defining a main street, CDOT is aiming to support areas in or adjacent to community-focused, downtowns where people work, dine and shop. These routes help form a specific region’s identity and act as the major economic hub in many towns and cities across Colorado.
The Colorado Department of Transportation (CDOT) is a multimodal transportation agency which supports a wide variety of alternatives to single occupant vehicle use. Phase 1 of this report details a statewide TDM strategy encompassing core strategies, support strategies emerging technologies TDM for specific travel markets and TDM programs. The 2019 Statewide TDM Plan is available upon request.
Email [email protected] for the TDM Plan.
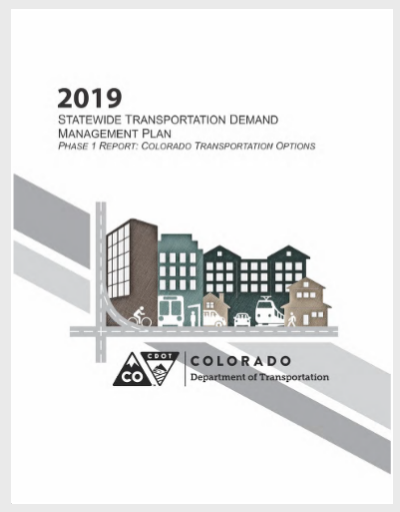
2019 Colorado Transportation Demand Management Plan - an important part of CDOT’s responsibility is to maintain and operate the State Highway System, CDOT is not a highway agency but instead a multimodal transportation agency that supports a wide variety of alternatives to single-occupant vehicle use. Phase 1 of this study was an inventory of existing Colorado TDM programs; phase 2 examines where and how CDOT can use TDM to address near-term mobility needs. This document is available upon request. Please click the button below to request a copy.
Request How to Create a TDM Plan
As part of the CDOT Procedural Directive 1601 approval process for new interchanges or for modification of an existing interchange, applicants will be required to create a Transportation Demand Management Plan. For questions reach out to [email protected] .
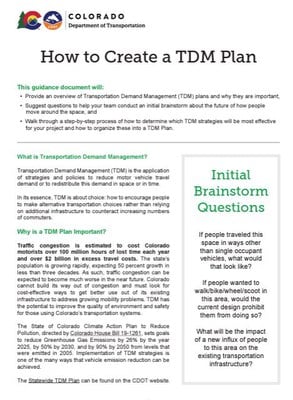
Americans’ dependence on cars and trucks as the dominant means of personal transportation is built on a foundation of dispersed and isolated land use patterns and automobile-centric urban street design, both of which have in turn been created by a complex array of policy and investment decisions. Untangling this complex web of rules and regulations requires a systematic approach to updating policy incentives to align with broadly held public goals—for example, the goal of creating transportation systems that provide equitable; affordable; and sustainable access to jobs, education, healthy food, recreation, and community for all.
The New Transportation Demand Management: An Implementation Guide for City Officials
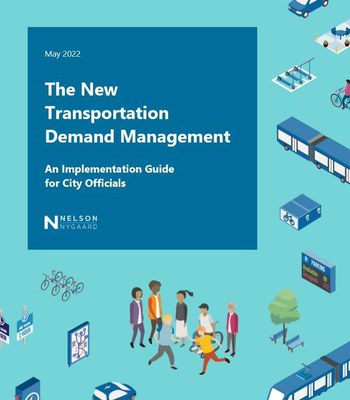
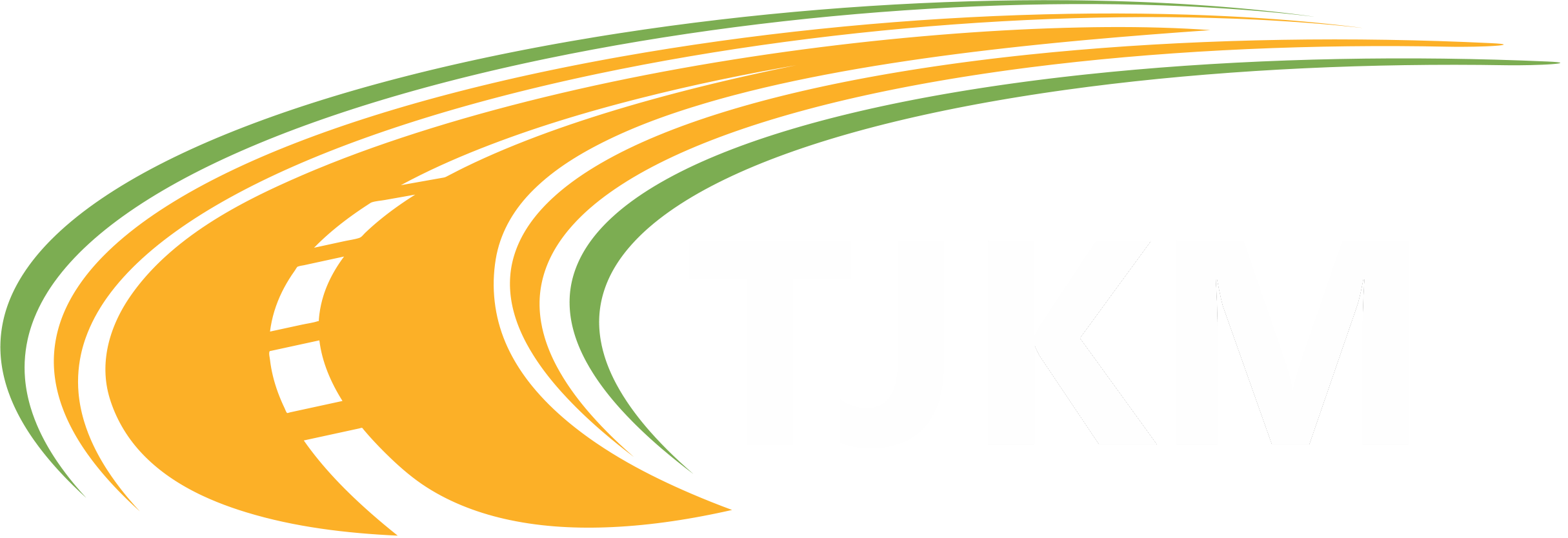
- Safe Routes to School
- Toll Studies
- Adaptive Signal Systems
- Bicycle and Pedestrian
- Complete Streets
- Congestion Management
- Corridor Studies
- Freeway Operations
- On-Call Services
- Signal Timing
- Traffic Calming
- Traffic Impact Fees
- Traffic Impact Studies
- Traffic Signal Design
- Travel Demand Modeling
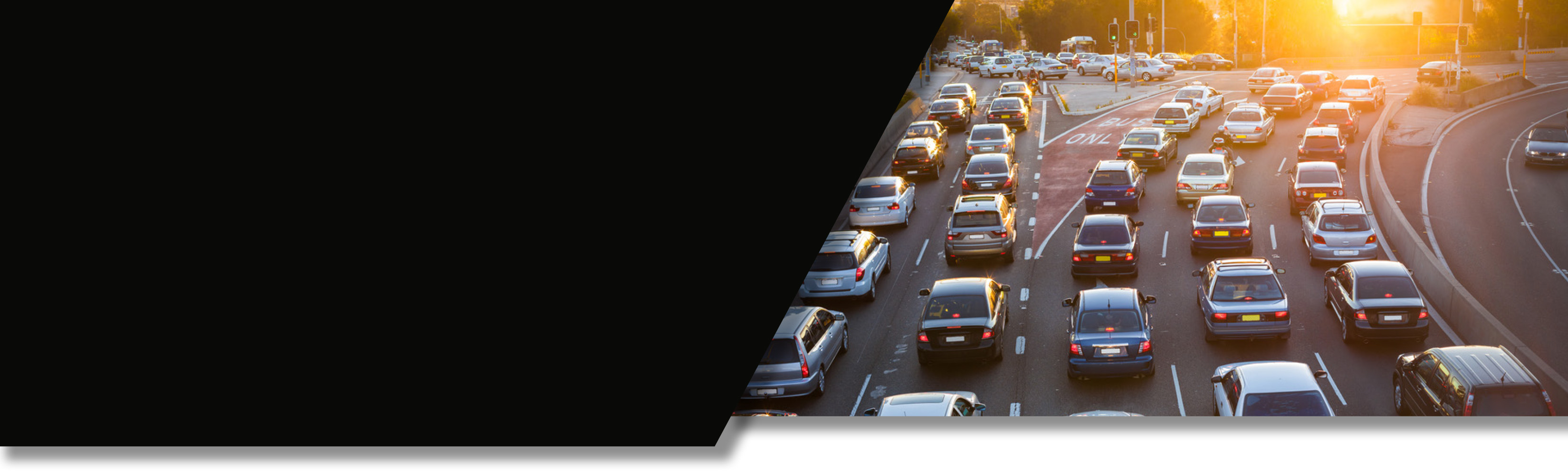
Transportation Demand Management
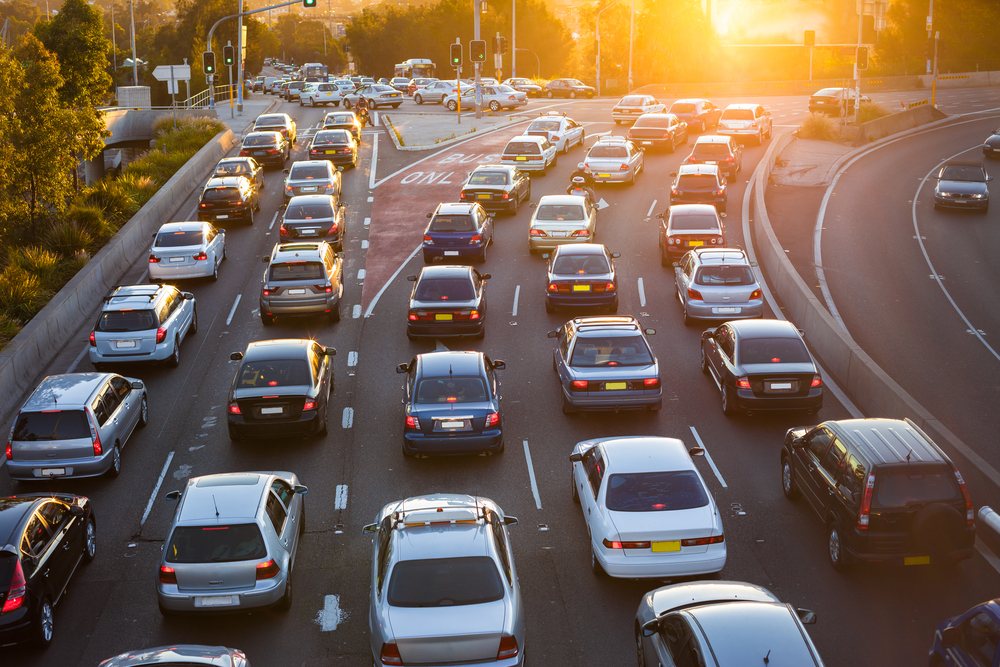
To reduce the number of vehicle trips generated and thereby the traffic impacts of development, TJKM often prepares transportation demand management (TDM) plans for local agencies and developers. TJKM assisted the City of Pleasanton in developingn the City’s TDM program, which has been a key feature in the successful development of the large Hacienda Business Park area of the City over the past 30 years. Additionally, TJKM has created TDM plans for many specific development projects, including the 600 West California Avenue R&D/Office complex in Sunnyvale, San Francisco Premium Outlets in Livermore, and Terraces at San Joaquin Gardens Senior Housing in Fresno.
Relevant Projects
- Transportation Demand Management Plan for the Paragon Retail Outlets, Livermore
- TDM Plan for the Terraces at San Joaquin Gardens Senior Residential Development, Fresno
Click here for more information
- Careers Opportunities
- Benefits and Perks
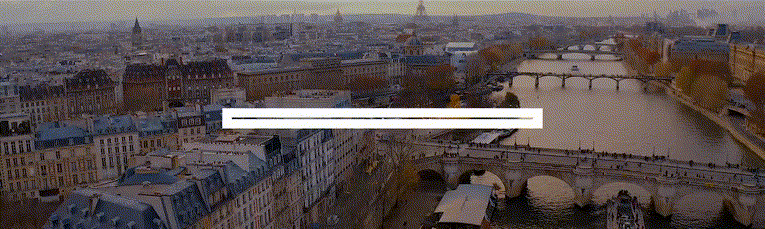
COMMENTS
Transportation Demand Management. Transportation demand management (TDM), or simply demand management, is defined a set of strategies aimed at maximizing traveler choices. ... Managing demand is about providing travelers, regardless of whether they drive alone, with travel choices, such as work location, route, time of travel and mode. In the ...
Transportation Demand Management (TDM) Plan with a focus on how TDM can support goals within the region's 2040 regional transportation plan (PLAN 2040). ... Beyond Day-to-Day Travel Conditions: Active Demand Management Beyond day-to-day travel conditions, the transportation system is often impacted by a range of
Travel demand management is an integral part of multimodal transportation planning. It is important that planners understand the strategies they can use to influence the transportation modes citizens use. These policies and strategies, at their core, aim to increase the efficiency of transportation systems and influence citizen decisions when ...
Evening traffic on the A1 freeway in Slovenia. Transportation demand management or travel demand management (TDM) is the application of strategies and policies to increase the efficiency of transportation systems, that reduce travel demand, or to redistribute this demand in space or in time.. In transport, as in any network, managing demand can be a cost-effective alternative to increasing ...
A: Yes, A Traffic Impact Study (TIS), often currently required of developments, and a Travel Demand Management (TDM) study are both related to transportation planning, but they serve different purposes. Q: What is the cost of a TDM study and plan? A: Each TDM study and plan will be as unique as its development. The scope of the study, plan,
Some Definitions. Travel demand management (TDM) is defined as strategies to maximize system efficiency of urban transport by discouraging the use of private transport and promoting more effective, healthy and environmentally friendly modes of transport, including public transport and non-motorized transport (NMT) (Broaddus et al., 2009).
This Travel Demand Management (TDM) Toolkit sets out a framework for Combined and Local Transport Authorities (LTAs) in England to develop and implement efective TDM plans. This is a standalone document which should be used to help you navigate the efects of the COVID-19 pandemic on the travelling public and your transport networks.
Transportation demand management (TDM) is the successful complement to infrastructure. TDM focuses on understanding how people make their transportation decisions and influencing people's behavior to use existing infrastructure in more efficient ways, like reducing single occupancy vehicle trips and getting people to use transit, ridesharing, walking, biking, and telework.
The eight strategies are organized in two categories: Strategies to manage travel demand: 1. Multi-Service Trip Planner and Transportation Wallet (Mobility as a Service) 2. Free Feeder Services to High-Capacity Transit 3. Ridesharing and Teleworking 4.
This chapter focuses on the different types of strategies and actions to influence the demand for transportation. It is important first, however, to place the use of these strategies in the institutional and planning contexts most often found in the United States and Canada. Three major contexts are of most interest to planners: statewide or ...
Travel Demand Management Plan Guidance. TDM strategies enhance the existing regional transportation infrastructure to provide more convenient opportunities for employees to utilize sustainable forms of transportation rather than single occupant vehicle trips. TDM includes a mix of strategies, as further described in this plan, to encourage ...
The utilization of travel demand forecasting techniques leads to dynamic processes in urban areas. A comprehensive grasp of travel demand modeling is imperative for individuals involved in transportation planning and implementation. This chapter covers the fundamentals of the traditional four-step travel demand modeling approach.
Seattle Urban Mobility Plan January 2008 7A-1 Transportation Demand Management, or TDM, is a general term for strategies that increase over-all system efficiency by encouraging a shift from single-occupant vehicle (SOV) trips to non-SOV modes, or shifting auto trips out of peak periods. This supports the Urban Mobility Plan's focus on
Travel Demand Management (TDM) describes a wide range of strategies that support the most efficient use of the transportation system by making personal travel options more flexible, clear, or convenient. ... a team led by ICF for the Regional Travel Demand Management Study to evaluate TDM in the region and prepare an Action Plan that will feed ...
Travel Demand Management (TDM) is the application of a focused, data-led strategy that seeks to change demand on transport networks by redistributing journeys to other modes, times, routes, or by removing the journey altogether. It is most effectively applied when there is an impetus or catalyst for behaviour change. A Travel Plan is a ...
The overall objective is to ensure that travel demand management principles are considered and applied across all the Waka Kotahi NZ Transport Agency's work. It also informs our transport partners, developers and the public of our position on and approach to managing the demand for travel. Travel demand management manual.
555.1310. Buildings and uses subject to a travel demand management plan. (a) In general.Buildings and uses subject to a travel demand management plan are listed in Table 555-10, Buildings and Uses Subject to a Travel Demand Management Plan, along with the required minimum number of points required for each building or use.
The state's Transportation Demand Management Strategic Plan 2019-2023 (PDF 1.3MB) explains how the state and its partner organizations are using Practical Solutions and multimodal strategies to expand travel options across Washington state. For more general information on transportation demand management, view the following resources:
Travel demand management (TDM) is a rapidly changing field. This research drew lessons from international practice to inform New Zealand planning and policy decisions. The research findings show that a wide range of strategies are in use internationally and the report summarises some key insights from international TDM practice.
Transportation demand management (or TDM) is the discipline of encouraging and facilitating traveler behavior that makes more efficient use of the transportation network. Ultimately, this means providing people with more choice about how, where, when, and if they travel: giving them more freedom and flexibility in their work hours and location ...
The NJTPA developed a Transportation Demand Management (TDM) and Mobility Plan that identifies regional policies and strategies that will increase traveler choices while also minimizing the negative impacts of single-occupant vehicle travel on congestion, air quality, and safety.
Travel Demand Study & Management Plan To arrive at a TDM Plan, a Transportation Demand Study may be necessary. This would combine information and analysis typically found in a TIS with more detailed consideration of the specific site location and form as well as the specific uses and users, to help inform the best management strategies to plan.
Transportation Demand Management. To reduce the number of vehicle trips generated and thereby the traffic impacts of development, TJKM often prepares transportation demand management (TDM) plans for local agencies and developers. TJKM assisted the City of Pleasanton in developingn the City's TDM program, which has been a key feature in the ...
travel and way-finding whether a person is in a car, on foot, or on a bike. As part of the development of the NRP, all buildings will be clearly identified to ensure easy way finding. Building identification will be ... Demand Management Plan, acknowledging that parking will not remain free in subsequent phases of