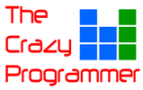
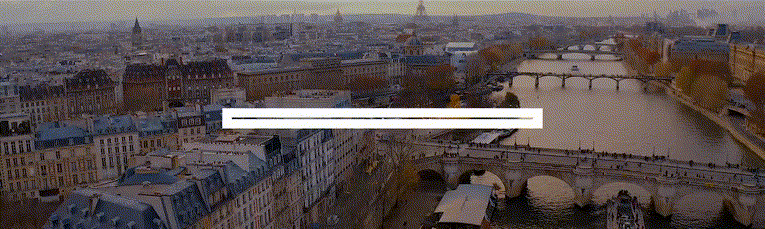
Travelling Salesman Problem in C and C++
Here you will learn about Travelling Salesman Problem (TSP) with example and also get a program that implements Travelling Salesman Problem in C and C++.
Let say there are some villages (1, 2, 3, 4, 5). To work with worst case let assume each villages connected with every other villages. And there is a Salesman living in village 1 and he has to sell his things in all villages by travelling and he has to come back to own village 1.
He has to travel each village exactly once, because it is waste of time and energy that revisiting same village. This is same as visiting each node exactly once, which is Hamiltonian Circuit . But our problem is bigger than Hamiltonian cycle because this is not only just finding Hamiltonian path, but also we have to find shortest path.
Finally the problem is we have to visit each vertex exactly once with minimum edge cost in a graph.
Brute Force Approach takes O (n n ) time, because we have to check (n-1)! paths (i.e all permutations) and have to find minimum among them.
The correct approach for this problem is solving using Dynamic Programming.
Dynamic Programming can be applied only if main problem can be divided into sub-problems. Let’s check that.
Travelling Salesman Problem (TSP) Using Dynamic Programming
Example problem.
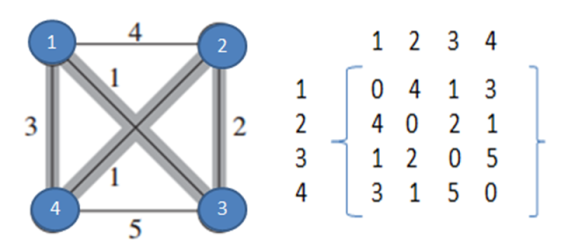
Above we can see a complete directed graph and cost matrix which includes distance between each village. We can observe that cost matrix is symmetric that means distance between village 2 to 3 is same as distance between village 3 to 2.
Here problem is travelling salesman wants to find out his tour with minimum cost.
Say it is T (1,{2,3,4}), means, initially he is at village 1 and then he can go to any of {2,3,4}. From there to reach non-visited vertices (villages) becomes a new problem. Here we can observe that main problem spitted into sub-problem, this is property of dynamic programming.
Note: While calculating below right side values calculated in bottom-up manner. Red color values taken from below calculations.
T ( 1, {2,3,4} ) = minimum of
= { (1,2) + T (2, {3,4} ) 4+ 6 =10
= { (1,3) + T (3, {2,4} ) 1+ 3 =4
= { (1,4) + T (4, {2,3} ) 3+ 3 =6
Here minimum of above 3 paths is answer but we know only values of (1,2) , (1,3) , (1,4) remaining thing which is T ( 2, {3,4} ) …are new problems now. First we have to solve those and substitute here.
T (2, {3,4} ) = minimum of
= { (2,3) + T (3, {4} ) 2+ 5 =7
= { (2,4) + T {4, {3} ) 1+ 5 =6
T (3, {2,4} ) = minimum of
= { (3,2) + T (2, {4} ) 2+ 1 =3
= { (3,4) + T {4, {2} ) 5+ 1 =6
T (4, {2,3} ) = minimum of
= { (4,2) + T (2, {3} ) 1+ 2 =3
= { (4,3) + T {3, {2} ) 5+ 2 =7
T ( 3, {4} ) = (3,4) + T (4, {} ) 5+0=5
T ( 4, {3} ) = (4,3) + T (3, {} ) 5+0=5
T ( 2, {4} ) = (2,4) + T (4, {} ) 1+0=1
T ( 4, {2} ) = (4,2) + T (2, {} ) 1+0 = 1
T ( 2, {3} ) = (2,3) + T (3, {} ) 2+0 = 2
T ( 3, {2} ) = (3,2) + T (2, {} ) 2+0=2
Here T ( 4, {} ) is reaching base condition in recursion, which returns 0 (zero ) distance.
This is where we can find final answer,
= { (1,2) + T (2, {3,4} ) 4+ 6 =10 in this path we have to add +1 because this path ends with 3. From there we have to reach 1 so 3->1 distance 1 will be added total distance is 10+1=11
= { (1,3) + T (3, {2,4} ) 1+ 3 =4 in this path we have to add +3 because this path ends with 3. From there we have to reach 1 so 4->1 distance 3 will be added total distance is 4+3=7
= { (1,4) + T (4, {2,3} ) 3+ 3 =6 in this path we have to add +1 because this path ends with 3. From there we have to reach 1 so 3->1 distance 1 will be added total distance is 6+1=7
Minimum distance is 7 which includes path 1->3->2->4->1.
After solving example problem we can easily write recursive equation.
Recursive Equation
T (i , s) = min ( ( i , j) + T ( j , S – { j }) ) ; S!= Ø ; j € S ;
S is set that contains non visited vertices
= ( i, 1 ) ; S=Ø, This is base condition for this recursive equation.
T (i, S) means We are travelling from a vertex “i” and have to visit set of non-visited vertices “S” and have to go back to vertex 1 (let we started from vertex 1).
( i, j ) means cost of path from node i to node j
If we observe the first recursive equation from a node we are finding cost to all other nodes (i,j) and from that node to remaining using recursion ( T (j , {S-j}))
But it is not guarantee that every vertex is connected to other vertex then we take that cost as infinity. After that we are taking minimum among all so the path which is not connected get infinity in calculation and won’t be consider.
If S is empty that means we visited all nodes, we take distance from that last visited node to node 1 (first node). Because after visiting all he has to go back to initial node.
Time Complexity
Since we are solving this using Dynamic Programming, we know that Dynamic Programming approach contains sub-problems.
Here after reaching i th node finding remaining minimum distance to that i th node is a sub-problem.
If we solve recursive equation we will get total (n-1) 2 (n-2) sub-problems, which is O (n2 n ) .
Each sub-problem will take O (n) time (finding path to remaining (n-1) nodes).
Therefore total time complexity is O (n2 n ) * O (n) = O (n 2 2 n )
Space complexity is also number of sub-problems which is O (n2 n )
Program for Travelling Salesman Problem in C
Enter the number of villages: 4
Enter the Cost Matrix
Enter Elements of Row: 1 0 4 1 3
Enter Elements of Row: 2 4 0 2 1
Enter Elements of Row: 3 1 2 0 5
Enter Elements of Row: 4 3 1 5 0 The cost list is: 0 4 1 3 4 0 2 1 1 2 0 5 3 1 5 0
The Path is: 1—>3—>2—>4—>1
Minimum cost is 7
Program for Travelling Salesman Problem in C++
Comment below if you found any information incorrect or have doubts regarding Travelling Salesman Problem algorithm.
Related Posts
Quick sort in c [program & algorithm], evaluation of postfix expression in c [algorithm and program], infix to postfix conversion in c [program and algorithm], dfs in c [program+algorithm], 49 thoughts on “travelling salesman problem in c and c++”.
Nicely explained. I was just trying to understand the code to implement this. What I was not able to understand is why we are adding the return to the same node as well for the minimum comparison. Will the below changed least code not work for all situation ?
int least(int c) { int i,nc=999; int min=999,kmin;
for(i=0;i < n;i++) { if((ary[c][i]!=0)&&(completed[i]==0)) if(ary[c][i] < min) /* REPLACED */ { min=ary[c][i]; /* REPLACED */ kmin=ary[c][i]; nc=i; } } if(min!=999) cost+=kmin; return nc; }
Sir can u please explain this function
hellow mam your code is not work properly (for selecting minimum path) because i insert a cost matrix input 0 7 3 4 0 2 9 1 0 this cost matrix currect answer is==>8 and also travel a vertex in 1–>3–>2–>1 But your code is only work with a order wise selection example it will travel only with 1–>2–>3–>1. etc…………….
Are you for real? Are you that dumb?
this is an undirected graph. 1->3->2->1 is exactly the same as 1->2->3->1.
go ahead, bob your head.
Hi Good explanation (: But… is it posible to do TSP problem in C without the recursion?
Your Dynamic TSP-Code might not work correctly for more than 4 cities. Looping over all subsets of a set is a challenge for Programmers.
Quote: Your Dynamic TSP-Code might not work correctly for more than 4 cities.
It doesn’t. I tried it for 6 and it fails to find the minimum path. Example cost matrix and found path:
The cost list is: 0 5 9 12 4 8 5 0 4 7 9 7 9 4 0 5 5 11 12 7 5 0 10 14 4 9 5 10 0 12 8 7 11 14 12 0
The Path is: 1—>5—>3—>2—>6—>4—>1 (cost 46)
But the path 1->2->3->4->5->6->1 has cost 44
This code is NOT correct. Also every other site has this same exact code. Some one please share the link to a correct working code for solving TSP using Dynamic Programming approach.
Actually this is TSP code,he is making us fool.Watch Tushar Roy video for real Dp implementation.
Thank you so much for this resource. Why do these sites go on copying I don’t understand.
Yes, you are correct…..
min=ary[i][0]+ary[c][i]; has to be min=ary[i][c]+ary[c][i]; this migth solve the issue with the above code
Thannnnnnnnnnnnnks
it didnt solve the code…
Is the code written using dynamic approach?
NO,it is greedy ,this not for TSP,it for MST
Isn’t this the branch and bound method?
what if I do not want him to go back to starting node ?
I’m pretty sure that this is just another implementation of the nearest neighbor algorithm….
it will be better if you could add more explanation about these above functions such as takeInput(), least(), minCost(). I am really hard to understand your code.
Nice..can i ask you something..how we want to assign a value of the array with specific value..is that possible for an array consists 2 value..its more like we put the coordinate in one array..
Thank you friend. I was trying to implement one here and yours came to save my work. Now I’m sorry in the heuristic way. hugs Att. Anderson Itacoatiara – Amazonas – Brazil
I ran this for 10 cities. It ran fine, but total cost for my matrix of random costs was 138, which is higher than the 125 cost with another program which gave a result of 1 10 9 8 7 6 5 4 3 2 1, which is clearly not a valid calculation. Sigh…
Well, the thought was there, just not carried to correct completion.
The explanation is solid but the code is wrong. In each recursion step only the closest next hop in regards to the starting city is calculated, but you really have to check ALL sub-problems. The recursion doesn’t do anything special here and could as well have been a for-loop. Just check the following matrix where the start point 1 has a large cost to the furthest city 4:
“The cost list is: 0 1 1 99 1 0 1 1 1 1 0 1 99 1 1 0
The Path is: 1—>2—>3—>4—>1
Minimum cost is 102”
When obviously this could have been just 4 cost with 1->2->4->3->1
Dude checkout your code it does not work for all case; int adj_matx[4][4] = {{0,10,15,20},{10,0,35,25},{15,35,0,30},{20,25,30,0}}; //ans: 80 int adj_matx[4][4] = {{0,4,1,3},{4,0,2,1},{1,2,0,5},{3,1,5,0}}; //ans: 7 int adj_matx[5][5] = {{0,100,300,100,75},{100,0,50,75,125},{300,50,0,100,125},{100,75,100,0,50},{75,125,125,50,0}}; //ans: 375 int adj_matx[4][4] = {{0,2,1,3},{2,0,4,100},{1,4,0,2},{3,100,2,0}}; //ans: 11 int adj_matx[4][4] = {{0,2,1,4},{2,0,4,3},{1,4,0,2},{4,3,2,0}}; //ans: 8 int adj_matx[4][4] = {{0,5,6,3},{5,0,3,6},{6,3,0,7},{3,6,7,0}}; //ans: 18 int adj_matx[5][5] = {{0,6,9,100,10},{6,0,11,100,100},{9,11,0,100,14},{100,100,100,0,8},{10,100,14,8,0}}; //ans:57
for the last case if starting node is 1 then path is 1-5-4-3-2-1 and cost is 135
I second that. Saurabh is correct.
———————-T ( 1,{ 2 3 4 5 })——————— Pairwise cost { 6 9 100 10 } Subproblem cost { 129 128 39 125 } Sum cost { 135 137 139 135 } Sub Paths Printing Matrix 5 4 3 2 2 4 5 3 2 3 5 4 2 3 4 5 Choosing subpath 0 Path Vector { 5 4 3 2 1 }
Time complexity of given code is n!
Your Program is good but it is not working for more than 4 cities.
I have never commented on any website. But i was compelled to do so this time. I have been reading your blog for a long time and i find explanations and code far easier than other websites. It’s amazing and very helpful.
U r finding this code for TSP simple bczz it is completely wrong.This is code of MST,using greedy.
0 9 8 8 12 0 13 6 10 9 0 5 20 15 10 0
for this matrix the solution should be 35 (1-2-4-3-1)but by using this code it give 40(1-3-4-2-1). and also this approach is not dynamic it is greedy.
Can any one write code to display all possible paths and their respective sum of that path
Why we declare a value 999 ?
Replace: min=ary[i][0]+ary[c][i]; to: min=ary[i][c]+ary[c][i];
now works :))
No it will not
hello can you pls give program travelling sales man using branch and bound
The Algorithm has this result : The cost list is: 0 10 15 20 10 0 35 25 15 35 0 30 20 25 30 0
The Path is: 1—>;2—>;3—>;4—>;1
Minimum cost is 95 But the correct minimum cost is 80 and the correct path is 1–>2–>4–>3–>1
not showing optimal path.
Function least should have a prototype error occurs here so pls check it out
The code is totally wrong and all the explanation is being plagarized.
It is not working correctly for testcase 4 0 5 15 15 5 0 3 7 15 3 0 10 15 7 10 0 Output is : 1—>2—>4—>3—>1 cost 37 Output should be: 1—>2—>3—>4—>1 cost 33
Crazy bro.. Code is not working bro….
can any one know program of TSP then pls share
Hi, I’m sorry but your code is wrong. Please make the required changes or at least remove the code.
I mean how can you be so wrong?You wrote the explanation so nicely(probably you copied that too) and then this shit code which is present all over the internet just for wasting our time.Dont create such rubbish posts if you dont know how to implement the algorithm.
The code is really helpful but I request you to add comments. A commented code helps a way better.
Leave a Comment Cancel Reply
Your email address will not be published. Required fields are marked *
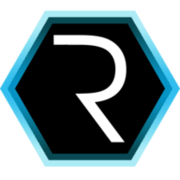
- ComputerSceince
Travelling Salesman Problem , with C Program Example
August 02, 2017.
Reading time ~2 minutes
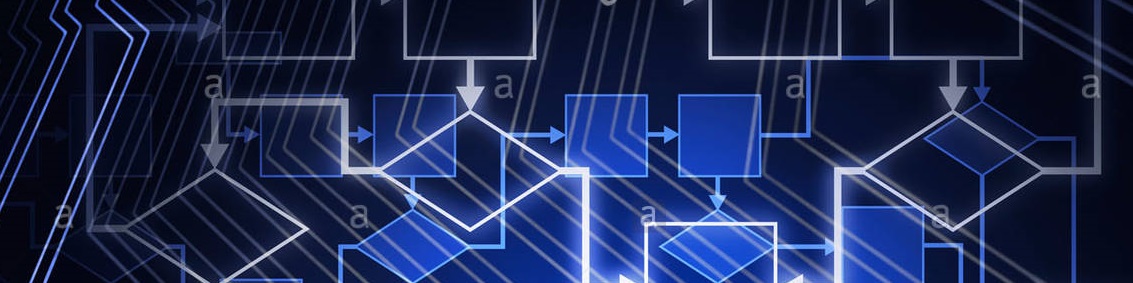
Travelling Salesman Problem is defined as “Given a list of cities and the distances between each pair of cities, what is the shortest possible route that visits each city exactly once and returns to the origin city?” It is an NP-hard problem.
Bellman–Held–Karp algorithm: Compute the solutions of all subproblems starting with the smallest. Whenever computing a solution requires solutions for smaller problems using the above recursive equations, look up these solutions which are already computed. To compute a minimum distance tour, use the final equation to generate the 1st node, and repeat for the other nodes. For this problem, we cannot know which subproblems we need to solve, so we solve them all.
Program in C :
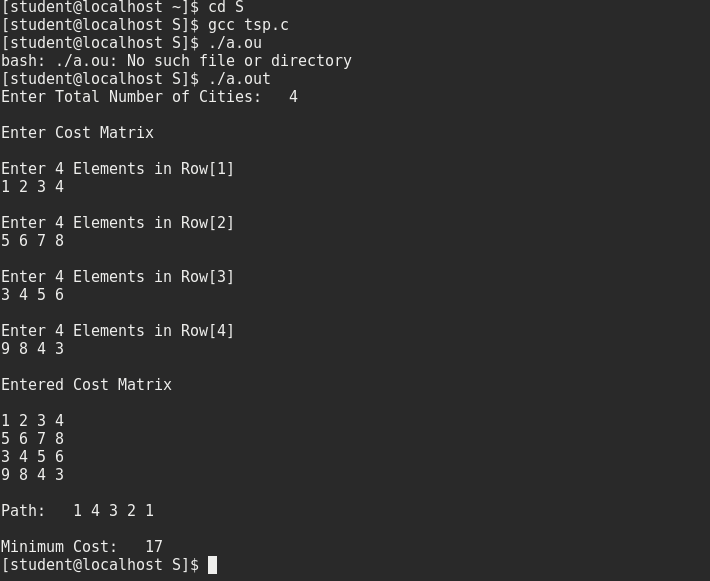
Please comment below in case of any problem found during running the code or any other doubts.
Saheb Ghosh
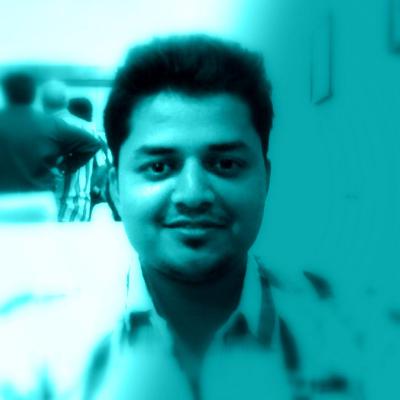
How to Change Service Fabric replicator log size and drive
How to modify Service Fabric replicator log size and also how to change Service Fabric Local cluster installtion directory or log directory. Continue reading
How to fix Dota 2 Crash or freeze Windows 10
Maximum flow ford-fulkarson’s algorithm, with c program example.

- Design Pattern
- Interview Q
C Control Statements
C functions, c dynamic memory, c structure union, c file handling, c preprocessor, c command line, c programming test, c interview.
- Send your Feedback to [email protected]
Help Others, Please Share

Learn Latest Tutorials

Transact-SQL

Reinforcement Learning

R Programming

React Native

Python Design Patterns

Python Pillow

Python Turtle

Preparation

Verbal Ability

Interview Questions

Company Questions
Trending Technologies

Artificial Intelligence

Cloud Computing

Data Science

Machine Learning

B.Tech / MCA

Data Structures

Operating System

Computer Network

Compiler Design

Computer Organization

Discrete Mathematics

Ethical Hacking

Computer Graphics

Software Engineering

Web Technology

Cyber Security

C Programming

Control System

Data Mining

Data Warehouse

Broad-Hurst In Mart
Software development courses in C, C++, Python, C# , Java for Linux and Windows
- 804-644-1856
A Comprehensive Guide: Travelling Salesman Problem in C
Previously, the author delved into three distinct greedy algorithms for the Traveling Salesman Problem: the Cheapest-Link, Nearest-Neighbour, and Repetitive-Nearest Neighbour. Although these methods provide approximate results, an accurate solution for the NP-complete Traveling Salesman Problem would demand exponential computational time, assuming P doesn’t equal NP. Nevertheless, backtracking offers a promising way to prune the search space, making it more manageable.
Table of Contents
Traveling Salesman Problem with Backtracking
To delve into the mechanics, they provide a C code that applies backtracking to solve the Traveling Salesman Problem. This solution uses the idea of permuting vertices while maintaining the starting point at vertex 0. As a result, the last edge invariably links the penultimate vertex back to vertex 0, eliminating the need to locate this edge via permutations .
The primary function, `traveling_salesman()`, ingests a matrix of distances (represented as adjmat), the total number of vertices (represented as order), and a pointer’s address to an unsigned integer array (best_tour). In return, it outputs the best tour’s cost and an array with the vertices arranged sequentially.
Sample Implementation
To better grasp this algorithm’s operation, a sample program is presented below:
When executed, the given program generates the following output:
This result showcases the tour with the minimum possible cost, alongside the corresponding vertices and edge weights. Through the presented backtracking approach, efficient solutions can be attained even for such complex problems as the Traveling Salesman. The Traveling Salesman Problem (TSP) stands as a prominent puzzle in the realm of optimization and computer science. Historically, it has served as a touchstone for algorithmic approaches and a testament to the complexity of real-world logistical challenges. The scenario is simple yet profound: A salesman wishes to visit a set of cities and return to the starting point while minimizing the total distance traveled. But don’t be fooled by its simplicity. The underlying combinatorial nature of the problem means that as the number of cities increases, the number of possible routes grows factorially. This exponential growth renders brute-force solutions impractical even for modest numbers of cities.
A Glimpse into its History
The roots of the TSP stretch back into the 1800s. Mathematicians like W.R. Hamilton and Thomas Kirkman began pondering about round trips through certain sites that would touch each point only once. Fast forward to the 20th century, and this problem found itself at the heart of operational research, especially during World War II, where optimization became crucial for logistical planning.
However, it was only in the latter half of the 20th century that the TSP was formally defined and recognized as an NP-hard problem. This classification implies that no polynomial-time solution exists for the TSP (assuming P ≠ NP, a major unsolved question in computer science).
Greedy Algorithms: A Starting Point
The Cheapest-Link, Nearest-Neighbour, and Repetitive-Nearest Neighbour algorithms are all greedy algorithms. They work on the principle of making the locally optimal choice at each step. For instance, the Nearest-Neighbour algorithm simply picks the closest unvisited city as the next destination. While such methods are fast and intuitive, they often don’t guarantee the best solution. In many instances, these algorithms can be misled by their myopic views, ignoring the broader structure of the problem.
Backtracking: A Ray of Hope
Backtracking offers a methodical way to navigate the solution space of the TSP. Instead of committing to decisions prematurely, backtracking allows for exploration and, when necessary, retreat. By systematically considering all possibilities but discarding those that can’t lead to a better solution early on, backtracking can often drastically reduce the effective search space.
The code provided earlier exemplifies backtracking in action. It starts with a fixed vertex (0 in this case) and explores permutations of the remaining vertices. Whenever a partial route’s cost exceeds the best known, the algorithm discards it, ensuring resources aren’t wasted on suboptimal paths.
Challenges and Real-world Implications
The TSP isn’t merely an academic exercise. Its real-world implications span a plethora of industries. From route planning for delivery trucks to manufacturing circuit boards, the essence of the TSP permeates many logistical and design challenges. Solutions to the TSP can lead to reduced fuel consumption, faster delivery times, and more efficient manufacturing processes.
However, real-world scenarios often come with their own set of challenges. Cities might have varying constraints, like specific time windows for deliveries or restrictions on vehicle types. These add layers of complexity to an already challenging problem. In these cases, heuristics and metaheuristics, like genetic algorithms or simulated annealing, might come into play. These methods, while not always guaranteeing the best solution, provide good enough solutions in a reasonable time frame.
The Traveling Salesman Problem, with its deceptive simplicity and underlying complexity, serves as a powerful reminder of the challenges and mysteries in optimization and computer science. Whether it’s through greedy algorithms that prioritize speed over accuracy, or more methodical approaches like backtracking that strive for the best, the journey to solve the TSP is as intriguing as the problem itself.
As computational power grows and algorithms become more sophisticated, we inch closer to more efficient solutions to the TSP. However, its NP-hard nature ensures that it will continue to captivate and challenge mathematicians, logisticians, and computer scientists for years to come.
Leave a Reply Cancel reply
You must be logged in to post a comment.
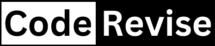
Learn Coding For Free
C program to perform Travelling Salesman Problem
Perform travelling salesman problem.
Here, you will know source code to perform Travelling salesman problem in C language .
Define traveling salesman problem
The Traveling Salesman Problem (TSP) is a problem in Computer Science , where you need to find the shortest possible route that visits a list of cities exactly once and returns to the starting city. It’s used in logistics and optimization, and finding the best solution can be very hard for large datasets.
Enter number of cities: 5 Enter cost of matrix: 23 45 67 56 89 23 45 16 87 56 34 65 47 87 90 12 34 32 56 73 53 12 50 30 25
0 0 1 2 3 0 Minimum Cost: 183
0 0 1 4 3 0 Minimum Cost: 166
0 0 3 1 2 0 Minimum Cost: 163
Find Minimum Spanning Tree using Kruskal’s Algorithm
Implement N Queens Problem using Backtracking
Knapsack Problem Using Dynamic Programming in C
Social Share
Insertion sort in c, linear search in c, binary search in c, merge sort in c using function, leave a comment cancel reply.
Your email address will not be published. Required fields are marked *
Save my name, email, and website in this browser for the next time I comment.
Forgot password? New user? Sign up
Existing user? Log in
Traveling Salesperson Problem
Already have an account? Log in here.
A salesperson needs to visit a set of cities to sell their goods. They know how many cities they need to go to and the distances between each city. In what order should the salesperson visit each city exactly once so that they minimize their travel time and so that they end their journey in their city of origin?
The traveling salesperson problem is an extremely old problem in computer science that is an extension of the Hamiltonian Circuit Problem . It has important implications in complexity theory and the P versus NP problem because it is an NP-Complete problem . This means that a solution to this problem cannot be found in polynomial time (it takes superpolynomial time to compute an answer). In other words, as the number of vertices increases linearly, the computation time to solve the problem increases exponentially.
The following image is a simple example of a network of cities connected by edges of a specific distance. The origin city is also marked.
Network of cities
Here is the solution for that network, it has a distance traveled of only 14. Any other path that the salesman can takes will result in a path length that is more than 14.
Relationship to Graphs
Special kinds of tsp, importance for p vs np, applications.
The traveling salesperson problem can be modeled as a graph . Specifically, it is typical a directed, weighted graph. Each city acts as a vertex and each path between cities is an edge. Instead of distances, each edge has a weight associated with it. In this model, the goal of the traveling salesperson problem can be defined as finding a path that visits every vertex, returns to the original vertex, and minimizes total weight.
To that end, many graph algorithms can be used on this model. Search algorithms like breadth-first search (BFS) , depth-first search (DFS) , and Dijkstra's shortest path algorithm can certainly be used, however, they do not take into consideration that fact that every vertex must be visited.
The Traveling Salesperson Problem (TSP), an NP-Complete problem, is notoriously complicated to solve. That is because the greedy approach is so computational intensive. The greedy approach to solving this problem would be to try every single possible path and see which one is the fastest. Try this conceptual question to see if you have a grasp for how hard it is to solve.
For a fully connected map with \(n\) cities, how many total paths are possible for the traveling salesperson? Show Answer There are (n-1)! total paths the salesperson can take. The computation needed to solve this problem in this way grows far too quickly to be a reasonable solution. If this map has only 5 cities, there are \(4!\), or 24, paths. However, if the size of this map is increased to 20 cities, there will be \(1.22 \cdot 10^{17}\) paths!
The greedy approach to TSP would go like this:
- Find all possible paths.
- Find the cost of every paths.
- Choose the path with the lowest cost.
Another version of a greedy approach might be: At every step in the algorithm, choose the best possible path. This version might go a little quicker, but it's not guaranteed to find the best answer, or an answer at all since it might hit a dead end.
For NP-Hard problems (a subset of NP-Complete problems) like TSP, exact solutions can only be implemented in a reasonable amount of time for small input sizes (maps with few cities). Otherwise, the best approach we can do is provide a heuristic to help the problem move forward in an optimal way. However, these approaches cannot be proven to be optimal because they always have some sort of downside.
Small input sizes
As described, in a previous section , the greedy approach to this problem has a complexity of \(O(n!)\). However, there are some approaches that decrease this computation time.
The Held-Karp Algorithm is one of the earliest applications of dynamic programming . Its complexity is much lower than the greedy approach at \(O(n^2 2^n)\). Basically what this algorithm says is that every sub path along an optimal path is itself an optimal path. So, computing an optimal path is the same as computing many smaller subpaths and adding them together.
Heuristics are a way of ranking possible next steps in an algorithm in the hopes of cutting down computation time for the entire algorithm. They are often a tradeoff of some attribute - such as completeness, accuracy, or precision - in favor of speed. Heuristics exist for the traveling salesperson problem as well.
The most simple heuristic for this problem is the greedy heuristic. This heuristic simply says, at each step of the network traversal, choose the best next step. In other words, always choose the closest city that you have not yet visited. This heuristic seems like a good one because it is simple and intuitive, and it is even used in practice sometimes, however there are heuristics that are proven to be more effective.
Christofides algorithm is another heuristic. It produces at most 1.5 times the optimal weight for TSP. This algorithm involves finding a minimum spanning tree for the network. Next, it creates matchings for the cities of an odd degree (meaning they have an odd number of edges coming out of them), calculates an eulerian path , and converts back to a TSP path.
Even though it is typically impossible to optimally solve TSP problems, there are cases of TSP problems that can be solved if certain conditions hold.
The metric-TSP is an instance of TSP that satisfies this condition: The distance from city A to city B is less than or equal to the distance from city A to city C plus the distance from city C to city B. Or,
\[distance_{AB} \leq distance_{AC} + distance_{CB}\]
This is a condition that holds in the real world, but it can't always be expected to hold for every TSP problem. But, with this inequality in place, the approximated path will be no more than twice the optimal path. Even better, we can bound the solution to a \(3/2\) approximation by using Christofide's Algorithm .
The euclidean-TSP has an even stricter constraint on the TSP input. It states that all cities' edges in the network must obey euclidean distances . Recent advances have shown that approximation algorithms using euclidean minimum spanning trees have reduced the runtime of euclidean-TSP, even though they are also NP-hard. In practice, though, simpler heuristics are still used.
The P versus NP problem is one of the leading questions in modern computer science. It asks whether or not every problem whose solution can be verified in polynomial time by a computer can also be solved in polynomial time by a computer. TSP, for example, cannot be solved in polynomial time (at least that's what is currently theorized). However, TSP can be solved in polynomial time when it is phrased like this: Given a graph and an integer, x, decide if there is a path of length x or less than x . It's easy to see that given a proposed answer to this question, it is simple to check if it is less than or equal to x.
The traveling salesperson problem, like other problems that are NP-Complete, are very important to this debate. That is because if a polynomial time solution can be found to this problems, then \(P = NP\). As it stands, most scientists believe that \(P \ne NP\).
The traveling salesperson problem has many applications. The obvious ones are in the transportation space. Planning delivery routes or flight patterns, for example, would benefit immensly from breakthroughs is this problem or in the P versus NP problem .
However, this same logic can be applied to many facets of planning as well. In robotics, for instance, planning the order in which to drill holes in a circuit board is a complex task due to the sheer number of holes that must be drawn.
The best and most important application of TSP, however, comes from the fact that it is an NP-Complete problem. That means that its practical applications amount to the applications of any problem that is NP-Complete. So, if there are significant breakthroughs for TSP, that means that those exact same breakthrough can be applied to any problem in the NP-Complete class.
Problem Loading...
Note Loading...
Set Loading...

- Data Structures & Algorithms
- DSA - Overview
- DSA - Environment Setup
- DSA - Algorithms Basics
- DSA - Asymptotic Analysis
- Data Structures
- DSA - Data Structure Basics
- DSA - Data Structures and Types
- DSA - Array Data Structure
- Linked Lists
- DSA - Linked List Data Structure
- DSA - Doubly Linked List Data Structure
- DSA - Circular Linked List Data Structure
- Stack & Queue
- DSA - Stack Data Structure
- DSA - Expression Parsing
- DSA - Queue Data Structure
- Searching Algorithms
- DSA - Searching Algorithms
- DSA - Linear Search Algorithm
- DSA - Binary Search Algorithm
- DSA - Interpolation Search
- DSA - Jump Search Algorithm
- DSA - Exponential Search
- DSA - Fibonacci Search
- DSA - Sublist Search
- DSA - Hash Table
- Sorting Algorithms
- DSA - Sorting Algorithms
- DSA - Bubble Sort Algorithm
- DSA - Insertion Sort Algorithm
- DSA - Selection Sort Algorithm
- DSA - Merge Sort Algorithm
- DSA - Shell Sort Algorithm
- DSA - Heap Sort
- DSA - Bucket Sort Algorithm
- DSA - Counting Sort Algorithm
- DSA - Radix Sort Algorithm
- DSA - Quick Sort Algorithm
- Graph Data Structure
- DSA - Graph Data Structure
- DSA - Depth First Traversal
- DSA - Breadth First Traversal
- DSA - Spanning Tree
- Tree Data Structure
- DSA - Tree Data Structure
- DSA - Tree Traversal
- DSA - Binary Search Tree
- DSA - AVL Tree
- DSA - Red Black Trees
- DSA - B Trees
- DSA - B+ Trees
- DSA - Splay Trees
- DSA - Tries
- DSA - Heap Data Structure
- DSA - Recursion Algorithms
- DSA - Tower of Hanoi Using Recursion
- DSA - Fibonacci Series Using Recursion
- Divide and Conquer
- DSA - Divide and Conquer
- DSA - Max-Min Problem
- DSA - Strassen's Matrix Multiplication
- DSA - Karatsuba Algorithm
- Greedy Algorithms
- DSA - Greedy Algorithms
- DSA - Travelling Salesman Problem (Greedy Approach)
- DSA - Prim's Minimal Spanning Tree
- DSA - Kruskal's Minimal Spanning Tree
- DSA - Dijkstra's Shortest Path Algorithm
- DSA - Map Colouring Algorithm
- DSA - Fractional Knapsack Problem
- DSA - Job Sequencing with Deadline
- DSA - Optimal Merge Pattern Algorithm
- Dynamic Programming
- DSA - Dynamic Programming
- DSA - Matrix Chain Multiplication
- DSA - Floyd Warshall Algorithm
- DSA - 0-1 Knapsack Problem
- DSA - Longest Common Subsequence Algorithm
- DSA - Travelling Salesman Problem (Dynamic Approach)
- Approximation Algorithms
- DSA - Approximation Algorithms
- DSA - Vertex Cover Algorithm
- DSA - Set Cover Problem
- DSA - Travelling Salesman Problem (Approximation Approach)
- Randomized Algorithms
- DSA - Randomized Algorithms
- DSA - Randomized Quick Sort Algorithm
- DSA - Karger’s Minimum Cut Algorithm
- DSA - Fisher-Yates Shuffle Algorithm
- DSA Useful Resources
- DSA - Questions and Answers
- DSA - Quick Guide
- DSA - Useful Resources
- DSA - Discussion
- Selected Reading
- UPSC IAS Exams Notes
- Developer's Best Practices
- Questions and Answers
- Effective Resume Writing
- HR Interview Questions
- Computer Glossary
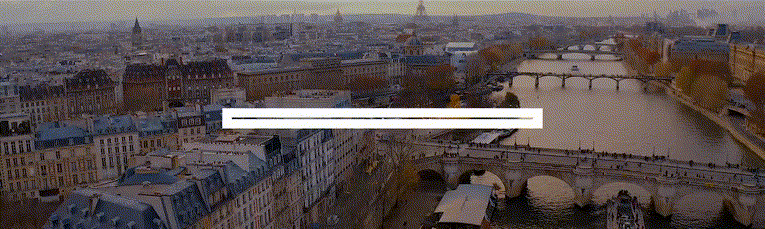
Travelling Salesman Problem (Dynamic Approach)
Travelling salesman dynamic programming algorithm, implementation.
Travelling salesman problem is the most notorious computational problem. We can use brute-force approach to evaluate every possible tour and select the best one. For n number of vertices in a graph, there are (n−1)! number of possibilities. Thus, maintaining a higher complexity.
However, instead of using brute-force, using the dynamic programming approach will obtain the solution in lesser time, though there is no polynomial time algorithm.
Let us consider a graph G = (V,E) , where V is a set of cities and E is a set of weighted edges. An edge e(u, v) represents that vertices u and v are connected. Distance between vertex u and v is d(u, v) , which should be non-negative.
Suppose we have started at city 1 and after visiting some cities now we are in city j . Hence, this is a partial tour. We certainly need to know j , since this will determine which cities are most convenient to visit next. We also need to know all the cities visited so far, so that we don't repeat any of them. Hence, this is an appropriate sub-problem.
For a subset of cities S $\epsilon$ {1,2,3,...,n} that includes 1 , and j $\epsilon$ S, let C(S, j) be the length of the shortest path visiting each node in S exactly once, starting at 1 and ending at j .
When |S|> 1 , we define 𝑪 C(S,1)= $\propto$ since the path cannot start and end at 1.
Now, let express C(S, j) in terms of smaller sub-problems. We need to start at 1 and end at j . We should select the next city in such a way that
$$C\left ( S,j \right )\, =\, min\, C\left ( S\, -\, \left\{j \right\},i \right )\, +\, d\left ( i,j \right )\: where\: i\: \epsilon \: S\: and\: i\neq j$$
There are at the most 2 n .n sub-problems and each one takes linear time to solve. Therefore, the total running time is O(2 n .n 2 ) .
In the following example, we will illustrate the steps to solve the travelling salesman problem.

From the above graph, the following table is prepared.
$$Cost\left ( 2,\Phi ,1 \right )\, =\, d\left ( 2,1 \right )\,=\,5$$
$$Cost\left ( 3,\Phi ,1 \right )\, =\, d\left ( 3,1 \right )\, =\, 6$$
$$Cost\left ( 4,\Phi ,1 \right )\, =\, d\left ( 4,1 \right )\, =\, 8$$
$$Cost(i,s)=min\left\{Cos\left ( j,s-(j) \right )\, +\,d\left [ i,j \right ] \right\}$$
$$Cost(2,\left\{3 \right\},1)=d[2,3]\, +\, Cost\left ( 3,\Phi ,1 \right )\, =\, 9\, +\, 6\, =\, 15$$
$$Cost(2,\left\{4 \right\},1)=d[2,4]\, +\, Cost\left ( 4,\Phi ,1 \right )\, =\, 10\, +\, 8\, =\, 18$$
$$Cost(3,\left\{2 \right\},1)=d[3,2]\, +\, Cost\left ( 2,\Phi ,1 \right )\, =\, 13\, +\, 5\, =\, 18$$
$$Cost(3,\left\{4 \right\},1)=d[3,4]\, +\, Cost\left ( 4,\Phi ,1 \right )\, =\, 12\, +\, 8\, =\, 20$$
$$Cost(4,\left\{3 \right\},1)=d[4,3]\, +\, Cost\left ( 3,\Phi ,1 \right )\, =\, 9\, +\, 6\, =\, 15$$
$$Cost(4,\left\{2 \right\},1)=d[4,2]\, +\, Cost\left ( 2,\Phi ,1 \right )\, =\, 8\, +\, 5\, =\, 13$$
$$Cost(2,\left\{3,4 \right\},1)=min\left\{\begin{matrix} d\left [ 2,3 \right ]\,+ \,Cost\left ( 3,\left\{ 4\right\},1 \right )\, =\, 9\, +\, 20\, =\, 29 \\ d\left [ 2,4 \right ]\,+ \,Cost\left ( 4,\left\{ 3\right\},1 \right )\, =\, 10\, +\, 15\, =\, 25 \\ \end{matrix}\right.\, =\,25$$
$$Cost(3,\left\{2,4 \right\},1)=min\left\{\begin{matrix} d\left [ 3,2 \right ]\,+ \,Cost\left ( 2,\left\{ 4\right\},1 \right )\, =\, 13\, +\, 18\, =\, 31 \\ d\left [ 3,4 \right ]\,+ \,Cost\left ( 4,\left\{ 2\right\},1 \right )\, =\, 12\, +\, 13\, =\, 25 \\ \end{matrix}\right.\, =\,25$$
$$Cost(4,\left\{2,3 \right\},1)=min\left\{\begin{matrix} d\left [ 4,2 \right ]\,+ \,Cost\left ( 2,\left\{ 3\right\},1 \right )\, =\, 8\, +\, 15\, =\, 23 \\ d\left [ 4,3 \right ]\,+ \,Cost\left ( 3,\left\{ 2\right\},1 \right )\, =\, 9\, +\, 18\, =\, 27 \\ \end{matrix}\right.\, =\,23$$
$$Cost(1,\left\{2,3,4 \right\},1)=min\left\{\begin{matrix} d\left [ 1,2 \right ]\,+ \,Cost\left ( 2,\left\{ 3,4\right\},1 \right )\, =\, 10\, +\, 25\, =\, 35 \\ d\left [ 1,3 \right ]\,+ \,Cost\left ( 3,\left\{ 2,4\right\},1 \right )\, =\, 15\, +\, 25\, =\, 40 \\ d\left [ 1,4 \right ]\,+ \,Cost\left ( 4,\left\{ 2,3\right\},1 \right )\, =\, 20\, +\, 23\, =\, 43 \\ \end{matrix}\right.\, =\, 35$$
The minimum cost path is 35.
Start from cost {1, {2, 3, 4}, 1}, we get the minimum value for d [1, 2]. When s = 3, select the path from 1 to 2 (cost is 10) then go backwards. When s = 2, we get the minimum value for d [4, 2]. Select the path from 2 to 4 (cost is 10) then go backwards.
When s = 1, we get the minimum value for d [4, 2] but 2 and 4 is already selected. Therefore, we select d [4, 3] (two possible values are 15 for d [2, 3] and d [4, 3], but our last node of the path is 4). Select path 4 to 3 (cost is 9), then go to s = ϕ step. We get the minimum value for d [3, 1] (cost is 6).

Following are the implementations of the above approach in various programming languages −

- Coding Problems
Travelling Salesman Problem (TSP)
Problem statement, example 1: travelling salesman problem, example 2: travelling salesman problem, 1. simple approach, c++ code implementation, java code implementation, python code implementation, 2. travelling salesman problem using dynamic programming, c code implementation, 3. greedy approach, practice questions, frequently asked questions, 1. which algorithm is used for the travelling salesman problem, 2. what is the complexity of the travelling salesman problem, 3. how is this problem modelled as a graph problem, 4: what is the difficulty level of the travelling salesman problem.
Travelling Salesman Problem (TSP) – Given a set of cities and the distance between every pair of cities as an adjacency matrix, the problem is to find the shortest possible route that visits every city exactly once and returns to the starting point. The ultimate goal is to minimize the total distance travelled, forming a closed tour or circuit.
The TSP is referred to as an NP-hard problem, meaning there is no known algorithm to solve it in polynomial time for large instances. As the number of cities increases, the number of potential solutions grows exponentially, making an exhaustive search unfeasible. This complexity is one of the reasons why the TSP remains a popular topic of research. Learn More .
Input –
Confused about your next job?
Output –
Here, the TSP Tour is 0-2-1-3-0 and the cost of the tour is 48.
Minimum weight Hamiltonian Cycle : EACBDE= 32
Wondering how the Hamiltonian Cycle Problem and the Traveling Salesman Problem differ? The Hamiltonian Cycle problem is to find out if there exists a tour that visits each city exactly once. Here, we know that the Hamiltonian Tour exists (due to the graph being complete), and there are indeed many such tours. The problem is to find a minimum weight Hamiltonian Cycle.
There are various approaches to finding the solution to the travelling salesman problem- simple (naïve) approach, dynamic programming approach, and greedy approach. Let’s explore each approach in detail:
- Consider city 1 as the starting and ending point. Since the route is cyclic, we can consider any point as a starting point.
- Now, we will generate all possible permutations of cities which are (n-1)!.
- Find the cost of each permutation and keep track of the minimum cost permutation.
- Return the permutation with minimum cost.
- Time complexity: O(N!), Where N is the number of cities.
- Space complexity: O(1).
In the travelling salesman problem algorithm, we take a subset N of the required cities that need to be visited, the distance among the cities dist, and starting cities s as inputs. Each city is identified by a unique city id which we say like 1,2,3,4,5………n
Here we use a dynamic approach to calculate the cost function Cost(). Using recursive calls, we calculate the cost function for each subset of the original problem.
To calculate the cost(i) using Dynamic Programming , we need to have some recursive relation in terms of sub-problems.
We start with all subsets of size 2 and calculate C(S, i) for all subsets where S is the subset, then we calculate C(S, i) for all subsets S of size 3 and so on.
There are at most O(n2^n) subproblems, and each one takes linear time to solve. The total running time is, therefore, O(n^22^n). The time complexity is much less than O(n!) but still exponential. The space required is also exponential.
- Time Complexity: O(N^2*2^N).
- First of them is a list that can hold the indices of the cities in terms of the input matrix of distances between cities
- And the Second one is the array which is our result
- Perform traversal on the given adjacency matrix tsp[][] for all the cities and if the cost of reaching any city from the current city is less than the current cost update the cost.
- Generate the minimum path cycle using the above step and return their minimum cost.
- Time complexity: O(N^2*logN), Where N is the number of cities.
- Space complexity: O(N).
- City Tour Problem
- Shortest Common Substring
Ans . Travelling Salesman Problem uses Dynamic programming with a masking algorithm.
Ans.: The complexity of TSP using Greedy will be O(N^2 LogN) and using DP will be O(N^2 2^N).
Ans .: The TSP can be modelled as a graph problem by considering a complete graph G = (V, E). A tour is then a circuit in G that meets every node. In this context, tours are sometimes called Hamiltonian circuits.
Ans.: It is an NP-hard problem.
- Travelling Salesman Problem
Previous Post
Longest common subsequence, minimum spanning tree – kruskal algorithm.
- Data Structures
- Linked List
- Binary Tree
- Binary Search Tree
- Segment Tree
- Disjoint Set Union
- Fenwick Tree
- Red-Black Tree
- Advanced Data Structures
- Traveling Salesman Problem using Genetic Algorithm
- Find if there is a path of more than k length from a source
- Minesweeper Solver
- Unique paths covering every non-obstacle block exactly once in a grid
- Minimum colors required such that edges forming cycle do not have same color
- Number of pairs such that path between pairs has the two vertices A and B
- Difference Between sum of degrees of odd and even degree nodes in an Undirected Graph
- Find the maximum value permutation of a graph
- Relabel-to-front Algorithm
- Check if a cycle exists between nodes S and T in an Undirected Graph with only S and T repeating | Set - 2
- Count the number of walks of length N where cost of each walk is equal to a given number
- Shortest Path Faster Algorithm
- Count number of ways to reach destination in a maze
- Maximum number of nodes which can be reached from each node in a graph.
- Program to generate all possible valid IP addresses from given string | Set 2
- Shortest Path Properties
- Count all possible Paths between two Vertices
- Samsung Semiconductor Institute of Research(SSIR Software) intern/FTE | Set-3
- Maximal Independent Set in an Undirected Graph
Travelling Salesman Problem implementation using BackTracking
Travelling Salesman Problem (TSP): Given a set of cities and distance between every pair of cities, the problem is to find the shortest possible route that visits every city exactly once and returns back to the starting point. Note the difference between Hamiltonian Cycle and TSP. The Hamiltonian cycle problem is to find if there exist a tour that visits every city exactly once. Here we know that Hamiltonian Tour exists (because the graph is complete) and in fact many such tours exist, the problem is to find a minimum weight Hamiltonian Cycle. For example, consider the graph shown in the figure. A TSP tour in the graph is 1 -> 2 -> 4 -> 3 -> 1. The cost of the tour is 10 + 25 + 30 + 15 which is 80. The problem is a famous NP hard problem. There is no polynomial time know solution for this problem.

Output of Given Graph: Minimum weight Hamiltonian Cycle : 10 + 25 + 30 + 15 = 80
Approach: In this post, implementation of simple solution is discussed.
- Consider city 1 (let say 0th node) as the starting and ending point. Since route is cyclic, we can consider any point as starting point.
- Start traversing from the source to its adjacent nodes in dfs manner.
- Calculate cost of every traversal and keep track of minimum cost and keep on updating the value of minimum cost stored value.
- Return the permutation with minimum cost.
Below is the implementation of the above approach:
Time Complexity: O(N!), As for the first node there are N possibilities and for the second node there are n – 1 possibilities. For N nodes time complexity = N * (N – 1) * . . . 1 = O(N!) Auxiliary Space: O(N)
Please Login to comment...
Similar reads.
- Backtracking
- 10 Ways to Use Microsoft OneNote for Note-Taking
- 10 Best Yellow.ai Alternatives & Competitors in 2024
- 10 Best Online Collaboration Tools 2024
- 10 Best Autodesk Maya Alternatives for 3D Animation and Modeling in 2024
- 30 OOPs Interview Questions and Answers (2024)
Improve your Coding Skills with Practice
What kind of Experience do you want to share?
Travelling Salesperson Problem using Dynamic Approach in C++
In this tutorial, we will learn about the TSP(Travelling Salesperson problem) problem in C++. In this tutorial, we will learn about what is TSP. Next, what are the ways there to solve it and at last we will solve with the C++, using Dynamic Approach. This is also known as Travelling Salesman Problem in C++.
Let’s take a scenario. Suppose you want to travel by car from your home to 4 places and at the end of it you want to return back to your home. Taking the problem as a worst case, let’s think all the 4 places are connected with each other [we are taking the worst case because we don’t know in details about the places ].
TSP USING DYNAMIC APPROACH in C++
To solve the problem we have some exact conditions :
- You can only visit each place only once. Once visited you can’t visit the place.
- Most importantly you have to find the shortest path. [This condition will differentiate the problem with Hamiltonian Problem.
We know what are the conditions we have to follow. Now we are going to see what are the process we can use in this problem.
Approaches to solve TSP :
- Dynamic Approach
- Brute Force Approach
- Backtracking Approach
- Branch and Bound
- Genetic Algorithm
- Simulated Annealing
– We are not going to use every approach to solve the problem. We are going to pick up the Dynamic Approach to solve the problem.
Now the question is why Dynamic approach?
Brute Force (or we can tell Backtracking Approach ) solves the problem, checking all the possible solutions to solve it. That will take O(n^n) time to solve it. But in the Dynamic Approach, we can divide the problem into subproblems.
Let’s check the coding of TSP using Dynamic Approach.
Travelling Salesperson Problem in C++
Time Complexity :
Thus we have learned How to solve Travelling Salesperson Problem in C++.
Put your doubts and questions in the below comment section.
You may also learn:
- Activity Selection Problem using Greedy method in C++
4 responses to “Travelling Salesperson Problem using Dynamic Approach in C++”
Thanks A lot !! alert(“Thank You So Much”) !!!!!!!!!!!!!!!!!!!!!!!!!!!!!!!!!!!!!!!!!!!!!!!!!!!!!!!!!!!!!!!!!!!!!!!!!!!!!
I would like to implement dynamic programming for crossover to generate new generation solutions in genetic algorithms. Can anyone suggest how to approach.
The code is well-formatted but the logical part is still not clear. Can anyone please explain the lines of code clearly in brief?
int completed_visit = (1<<n) -1; what dose this line of code means?
Leave a Reply Cancel reply
Your email address will not be published. Required fields are marked *
Please enable JavaScript to submit this form.
Related Posts
- Word Break Problem in C++
- Sort elements on basis of frequency in a string in C++
- Solution of Tower Of Hanoi Problem in C++
Search code, repositories, users, issues, pull requests...
Provide feedback.
We read every piece of feedback, and take your input very seriously.
Saved searches
Use saved searches to filter your results more quickly.
To see all available qualifiers, see our documentation .
traveling-salesman-problem
Here are 14 public repositories matching this topic..., alberto-santini / concorde-easy-build.
Fork of the Concorde TSP solver with an easier build procedure
- Updated Mar 17, 2024
deno750 / TSP_Optimization
Repository for the course Operations Research 2
- Updated Dec 30, 2021
vinaychourasiya / TSP-INDIA
Travelling salesman problem for India
- Updated Dec 14, 2020
walnut07 / GoogleSTEP
This repository has homework that I submitted during Google STEP where I learned the basics of of Computer Science.
- Updated Jul 5, 2022
agusmontero / tsprd
TSP-rd code and instances
- Updated Dec 10, 2023
LogiFoxy / TSP
Final assignment on the Traveling Salesman Problem with Branch and Bound technique for my Graph Theory course.
- Updated Nov 13, 2021
sal-versij / TravelingSalesmanProblem-GPU
TSP Bruteforce on GPU
- Updated Oct 3, 2022
lay-bs / Travelling-Salesman-Problem
Solving the traveling salesman problem using tree and graph algorithms and providing their respective analyses.
- Updated Mar 19, 2024
Angel-Karasu / traveling-salesman-problem
Various methods to find the (pseudo-)optimal solution for the TSP.
- Updated Apr 10, 2024
Vishnu44d / graph_algorithms
Graph algorithms in c
- Updated Nov 18, 2019
HannanI7 / TSPwithBruteForce
Brute Force Approach to finding the solution to the Traveling Salesman Problem (TSP). Done Serially and in Parallel.
- Updated Mar 26, 2024
lazy-dude / TSP
Traveling Sales Person (TSP) problem
- Updated Apr 5, 2023
esha411 / Traveling_Salesman_Problem
Given a list of cities and the distances between them, to find the shortest possible route that visit each city and return to the origin city.
- Updated Feb 8, 2023
enricobolzonello / TravellingSalesmanOptimization
Solving the Traveling Salesman Problem with different algorithms, project repository of the Operations Research 2 course 2023/24 @ UniPD
- Updated Apr 16, 2024
Improve this page
Add a description, image, and links to the traveling-salesman-problem topic page so that developers can more easily learn about it.
Curate this topic
Add this topic to your repo
To associate your repository with the traveling-salesman-problem topic, visit your repo's landing page and select "manage topics."
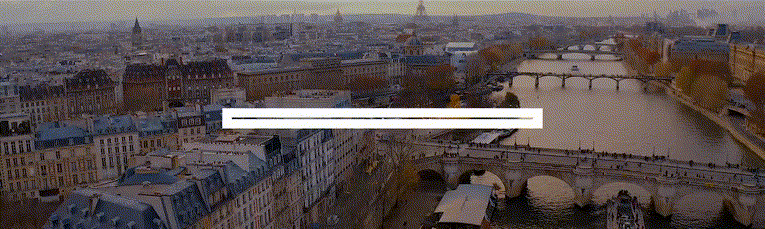
IMAGES
VIDEO
COMMENTS
Here problem is travelling salesman wants to find out his tour with minimum cost. Say it is T (1,{2,3,4}), means, initially he is at village 1 and then he can go to any of {2,3,4}. From there to reach non-visited vertices (villages) becomes a new problem.
Travelling Salesman Problem (TSP) : Given a set of cities and distances between every pair of cities, the problem is to find the shortest possible route that visits every city exactly once and returns to the starting point. Note the difference between Hamiltonian Cycle and TSP. The Hamiltonian cycle problem is to find if there exists a tour that visits every city exactly once.
Reading time ~2 minutes. Travelling Salesman Problem is defined as "Given a list of cities and the distances between each pair of cities, what is the shortest possible route that visits each city exactly once and returns to the origin city?". It is an NP-hard problem. Bellman-Held-Karp algorithm: Compute the solutions of all subproblems ...
Travelling Salesman Problem in C. The Travelling Salesman Problem (TSP) is a well-known optimization issue in the areas of mathematics and computer science.One way to put it is as follows: Find the shortest route that visits each city exactly once, travels the distance between each pair of cities, and then returns to the starting city. Numerous practical applications of the issue exist ...
The Traveling Salesman Problem (TSP) stands as a prominent puzzle in the realm of optimization and computer science. Historically, it has served as a touchstone for algorithmic approaches and a testament to the complexity of real-world logistical challenges. The scenario is simple yet profound: A salesman wishes to visit a set of cities and ...
Travelling salesman problem takes a graph G {V, E} as an input and declare another graph as the output (say G') which will record the path the salesman is going to take from one node to another. The algorithm begins by sorting all the edges in the input graph G from the least distance to the largest distance. The first edge selected is the ...
The problem is a famous NP-hard problem. There is no polynomial-time know solution for this problem. The following are different solutions for the traveling salesman problem. Naive Solution: 1) Consider city 1 as the starting and ending point. 2) Generate all (n-1)!
Define traveling salesman problem. The Traveling Salesman Problem (TSP) is a problem in Computer Science, where you need to find the shortest possible route that visits a list of cities exactly once and returns to the starting city. It's used in logistics and optimization, and finding the best solution can be very hard for large datasets.
The Travelling Salesman Problem (TSP) is a very well known problem in theoretical computer science and operations research. The standard version of TSP is a hard problem to solve and belongs to the NP-Hard class. In this tutorial, we'll discuss a dynamic approach for solving TSP. Furthermore, we'll also present the time complexity analysis ...
A C program to solve the Travelling Salesman Problem with multiple threads (and communication via MPI). This was a project for Data structures & algorithms 3 and won the contest for the fastest implementation of the year 2013. This was achieved by implementing a complex algorithm to calculate the Held-Karp lower bound and optimizing the whole ...
The traveling salesperson problem can be modeled as a graph. Specifically, it is typical a directed, weighted graph. Each city acts as a vertex and each path between cities is an edge. Instead of distances, each edge has a weight associated with it. In this model, the goal of the traveling salesperson problem can be defined as finding a path ...
We introduced Travelling Salesman Problem and discussed Naive and Dynamic Programming Solutions for the problem in the previous post. Both of the solutions are infeasible. In fact, there is no polynomial-time solution available for this problem as the problem is a known NP-Hard problem. There are approximate algorithms to solve the problem though.
we will get all out (n-1) 2(n-2) sub-problems, which is O (n2n). Each sub-problem will take O (n) time (discovering way to outstanding (n-1) hubs). In this manner all-out time unpredictability is O (n2n) * O (n) = O (n22n) Space multifaceted nature is likewise number of sub-problems which is O (n2n) Program for Traveling Salesman Problem in C
Travelling Salesman Problem (Dynamic Approach) - Travelling salesman problem is the most notorious computational problem. We can use brute-force approach to evaluate every possible tour and select the best one. For n number of vertices in a graph, there are (n−1)! number of possibilities. Thus, maintaining a higher complexity.
There are various approaches to finding the solution to the travelling salesman problem- simple (naïve) approach, dynamic programming approach, and greedy approach. Let's explore each approach in detail: 1. Simple Approach. Consider city 1 as the starting and ending point. Since the route is cyclic, we can consider any point as a starting point.
TSP problem is one of the most famous hard combinatorial optimization problems. It belongs to the class of NP-hard optimization problems. This means that no polynomial time algorithm is known to guarantee its global optimal solution. Consider a salesman who has to visit cities. The TSP problem consists of finding the shortest tour through all ...
A TSP tour in the graph is 0-1-3-2-0. The cost of the tour is 10+25+30+15 which is 80. We have discussed following solutions. 1) Naive and Dynamic Programming. 2) Approximate solution using MST. Branch and Bound Solution. As seen in the previous articles, in Branch and Bound method, for current node in tree, we compute a bound on best possible ...
The Travelling Salesman Problem in C++. c-plus-plus nearest-neighbor-search tsp time-windows travelling-salesman tsp-problem tsp-tw travelling-salesman-problem tsp-solver tsp-approximation simulated-annealing-algorithm opt2 compressed-annealing Updated Apr 1, 2024; C++; artix41 / traveler Star 3. Code ...
The problem involves determining the sequence in which the cities should be visited by a salesperson so that the resulting trip covers the shortest possible distance and each city is visited exactly once. Solution of a traveling salesman problem: the black line shows the shortest possible loop that connects every red dot. Source: Wikipedia.
Travelling Salesman Problem (TSP): Given a set of cities and distance between every pair of cities, the problem is to find the shortest possible route that visits every city exactly once and returns back to the starting point. Note the difference between Hamiltonian Cycle and TSP. The Hamiltonian cycle problem is to find if there exist a tour that visits every city exactly once.
Output : Minimum Distance Travelled by you is 80. Time Complexity : O(n^2 * 2^n) Thus we have learned How to solve Travelling Salesperson Problem in C++. Put your doubts and questions in the below comment section. You may also learn: Activity Selection Problem using Greedy method in C++.
In this project, the Traveling Salesman Problem is addressed. There are several approaches implemented. The project was done in C++ 17 and OpenMP 5.1. cpp17 ant-colony-optimization openmp-parallelization traveling-salesman-problem intel-compiler. Updated on Jan 24, 2021.
The traveling salesman problem (TSP) is a very famous and popular classic algorithmic problem in the field of computer science and operations research. There are a lot of algorithms able to solve the problem such as Dijkstra's algorithm, prim's algorithm, breadth-first search algorithm, and so on and so forth, but they are not the ...